Bold Goals Require Bold Funding Levels. The FY25 Requests for the U.S. Bioeconomy Fall Short
Over the past year, there has been tremendous momentum in policy for the U.S. bioeconomy – the collection of advanced industry sectors, like pharmaceuticals, biomanufacturing, and others, with biology at their core. This momentum began in part with the Bioeconomy Executive Order (EO) and the programs authorized in CHIPS and Science, and continued with the Office of Science and Technology Policy (OSTP) release of the Bold Goals for U.S. Biotechnology and Biomanufacturing (Bold Goals) report. The report highlighted ambitious goals that the Department of Energy (DOE), Department of Commerce (DOC), Human Health Services (HHS), National Science Foundation (NSF), and the Department of Agriculture (USDA) have committed to in order to further the U.S. bioeconomical enterprise.
However, these ambitious goals set by various agencies in the Bold Goals report will also require directed and appropriate funding, and this is where we have been falling short. Multiple bioeconomy-related programs were authorized through the bipartisan CHIPS & Science legislation but have yet to receive anywhere near their funding targets. Underfunding and the resulting lack of capacity has also led to a delay in the tasks under the Bioeconomy EO. In order for the bold goals outlined in the report to be realized, it will be imperative for the U.S. to properly direct and fund the many different endeavors under the U.S. bioeconomy.
Despite this need for funding for the U.S. bioeconomy, the recently-completed FY2024 (FY24) appropriations were modest for some science agencies but abysmal for others, with decreases seen across many different scientific endeavors across agencies. The DOC, and specifically the National Institute of Standards and Technology (NIST), saw massive cuts in funding base program funding, with earmarks swamping core activities in some accounts.
There remains some hope that the FY2025 (FY25) budget will alleviate some of the cuts that have been seen to science endeavors, and in turn, to programs related to the bioeconomy. But the strictures of the Fiscal Responsibility Act, which contributed to the difficult outcomes in FY24, remain in place for FY25 as well.
Bioeconomy in the FY25 Request
With this difficult context in mind, the Presidential FY25 Budget was released as well as the FY25 budgets for DOE, DOC, HHS, NSF, and USDA.
The President’s Budget makes strides toward enabling a strong bioeconomy by prioritizing synthetic biology metrology and standards within NIST and by directing OSTP to establish the Initiative Coordination Office to support the National Engineering Biology Research and Development Initiative. However, beyond these two instances, the President’s budget only offers limited progress for the bioeconomy because of mediocre funding levels.
The U.S. bioeconomy has a lot going on, with different agencies prioritizing different areas and programs depending on their jurisdiction. This makes it difficult to properly grasp all the activity that is ongoing (but we’re working on it, stay tuned!). However, we do know that the FY25 budget requests from the agencies themselves have been a mix bag for bioeconomy activities related to the Bold Goals Report. Some agencies are asking for large appropriations, while some agencies are not investing enough to support these goals:
Department of Energy supports Bold Goals Report efforts in biotech & biomanufacturing R&D to further climate change solutions
The increase in funding levels requested for FY25 for BER and MESC will enable increased biotech and biomanufacturing R&D, supporting DOE efforts to meet its proposed objectives in the Bold Goals Report.
- INCREASE: $945M for Biological and Environmental Research (BER) in FY25, a substantial increase of 5% from FY24 appropriations of $900M
- INCREASE: $113M for Manufacturing & Energy Supply Chains (MESC) in FY25, a substantial expansion from FY24 appropriations of $18 million
Department of Commerce falls short in support of biotech & biomanufacturing R&D supply chain resilience
One budgetary increase request is offset by two flat funding levels.
- INCREASE: $645M for the International Trade Administration in FY25, a 6% increase from the FY24 enacted amount
- Specifically they are asking for $103M for the Supply Chain Resiliency Program. This is important because, as seen during the COVID-19 pandemic, our supply chain currently is not resilient. Ensuring that we have the proper inputs, outputs, and materials required to continue our day-to-day endeavors will be vital in maintaining and leading a strong bioeconomy.
- FLAT: $1.5B for NIST in FY25, a 3% increase from FY24 enacted of $1.46 billion
- $37M for NIST’s Manufacturing USA program and $175M for the Manufacturing Extension Partnership, both flat from the FY24 appropriations levels. Flat funding won’t keep pace with the amount of work that needs to be done to create a resilient supply chain and programs like BioMADE and NIIMBL need to guide their industries forward.
Department of Agriculture falls short in support of biotech & biomanufacturing R&D to further food & Ag innovation
- INCREASE: $1.74B for the National Institute of Food and Agriculture, a 3.5% increase from the FY24 enacted amount
- DECREASE: $1.78B for the Agricultural Research Service program, a 3% decrease from the FY24 appropriated $1.85B
- NO FUNDING: Once again the Agriculture Advanced Research and Development Authority (AgARDA) has not been funded at all in FY25, despite being authorized at $50M a year through FY23 through the 2018 Farm Bill – this is a missed opportunity to build much needed agriculture innovation
Human Health Services falls short in support of biotech & biomanufacturing R&D to further human health
- DECREASE: $47.9B for the National Institutes of Health, a 1.4% decrease from the FY24 appropriated $48.6B
- Specifically, the Advanced Research Projects Agency for Health (ARPA-H) would receive $1.5B, maintaining funding levels seen in FY24
- DECREASE: $970M for the Biomedical Advanced Research & Development Authority (BARDA), a 4% decrease from the FY24 appropriated $1B
National Science Foundation supports Bold Goals Report efforts in biotech & biomanufacturing R&D to further cross-cutting advances
- INCREASE: $8B for Research and Related Activities within NSF, a 12% increase from the FY24 enacted amounts
- $900M for Technology, Innovation, and Partnerships (TIP), where in FY24 Congress supported the program without specifying funds for it
- $258M for the Established Program to Stimulate Competitive Research (EPSCoR), a 3% increase from the FY24 enacted amount
- INCREASE: $387M for Advanced Manufacturing across NSF, a 9% increase from FY23 amounts*
- INCREASE: $421M for Biotechnology across NSF, a 9.4% increase from FY23 amounts*
- The NSF Engines will be vital in shaping regional microbioeconomies and furthering cross-cutting advances and information on their FY25 budgets can be found here.
* FY23 amounts are listed due to FY24 appropriations not being finalized at the time that this document was created.
Overall, the DOE and NSF have asked for FY25 budgets that could potentially achieve the goals stated in the Bold Goals Report, while the DOC, USDA and HHS have unfortunately limited their budgets and it remains questionable if they will be able to achieve the goals listed with the funding levels requested. The DOC, and specifically NIST, faces one of the biggest challenges this upcoming year. NIST has to juggle tasks assigned to it from the AI EO as well as the Bioeconomy EO and the Presidential Budget. The 8% decrease in funding for NIST does not paint a promising picture for either the Bioeconomy EO and should be something that Congress rectifies when they enact their appropriation bills. Furthermore, the USDA faces cuts in funding for vital programs related to their goals and AgARDA continues to be unfunded. In order for USDA to achieve the goals listed in the Bold Goals report, it will be imperative that Congress prioritize these areas for the benefit of the U.S. bioeconomy.
Predicting Progress: A Pilot of Expected Utility Forecasting in Science Funding
Read more about expected utility forecasting and science funding innovation here.
The current process that federal science agencies use for reviewing grant proposals is known to be biased against riskier proposals. As such, the metascience community has proposed many alternate approaches to evaluating grant proposals that could improve science funding outcomes. One such approach was proposed by Chiara Franzoni and Paula Stephan in a paper on how expected utility — a formal quantitative measure of predicted success and impact — could be a better metric for assessing the risk and reward profile of science proposals. Inspired by their paper, the Federation of American Scientists (FAS) collaborated with Metaculus to run a pilot study of this approach. In this working paper, we share the results of that pilot and its implications for future implementation of expected utility forecasting in science funding review.
Brief Description of the Study
In fall 2023, we recruited a small cohort of subject matter experts to review five life science proposals by forecasting their expected utility. For each proposal, this consisted of defining two research milestones in consultation with the project leads and asking reviewers to make three forecasts for each milestone:
- the probability of success;
- The scientific impact of the milestone, if it were reached; and
- The social impact of the milestone, if it were reached.
These predictions can then be used to calculate the expected utility, or likely impact, of a proposal and design and compare potential portfolios.
Key Takeaways for Grantmakers and Policymakers
The three main strengths of using expected utility forecasting to conduct peer review are
- For reviewers, it’s a relatively light-touch approach that encourages rigor and reduces anti-risk bias in scientific funding.
- The review criteria allow program managers to better understand the risk-reward profile of their grant portfolios and more intentionally shape them according to programmatic goals.
- Quantitative forecasts are resolvable, meaning that program officers can compare the actual outcomes of funded proposals with reviewers’ predictions. This generates a feedback/learning loop within the peer review process that incentivizes reviewers to improve the accuracy of their assessments over time.
Despite the apparent complexity of this process, we found that first-time users were able to successfully complete their review according to the guidelines without any additional support. Most of the complexity occurs behind-the-scenes, and either aligns with the responsibilities of the program manager (e.g., defining milestones and their dependencies) or can be automated (e.g., calculating the total expected utility). Thus, grantmakers and policymakers can have confidence in the user friendliness of expected utility forecasting.
How Can NSF or NIH Run an Experiment on Expected Utility Forecasting?
An initial pilot study could be conducted by NSF or NIH by adding a short, non-binding expected utility forecasting component to a selection of review panels. In addition to the evaluation of traditional criteria, reviewers would be asked to predict the success and impact of select milestones for the proposals assigned to them. The rest of the review process and the final funding decisions would be made using the traditional criteria.
Afterwards, study facilitators could take the expected utility forecasting results and construct an alternate portfolio of proposals that would have been funded if that approach was used, and compare the two portfolios. Such a comparison would yield valuable insights into whether—and how—the types of proposals selected by each approach differ, and whether their use leads to different considerations arising during review. Additionally, a pilot assessment of reviewers’ prediction accuracy could be conducted by asking program officers to assess milestone achievement and study impact upon completion of funded projects.
Findings and Recommendations
Reviewers in our study were new to the expected utility forecasting process and gave generally positive reactions. In their feedback, reviewers said that they appreciated how the framing of the questions prompted them to think about the proposals in a different way and pushed them to ground their assessments with quantitative forecasts. The focus on just three review criteria–probability of success, scientific impact, and social impact–was seen as a strength because it simplified the process, disentangled feasibility from impact, and eliminated biased metrics. Overall, reviewers found this new approach interesting and worth investigating further.
In designing this pilot and analyzing the results, we identified several important considerations for planning such a review process. While complex, engaging with these considerations tended to provide value by making implicit project details explicit and encouraging clear definition and communication of evaluation criteria to reviewers. Two key examples are defining the proposal milestones and creating impact scoring systems. In both cases, reducing ambiguities in terms of the goals that are to be achieved, developing an understanding of how outcomes depend on one another, and creating interpretable and resolvable criteria for assessment will help ensure that the desired information is solicited from reviewers.
Questions for Further Study
Our pilot only simulated the individual review phase of grant proposals and did not simulate a full review committee. The typical review process at a funding agency consists of first, individual evaluations by assigned reviewers, then discussion of those evaluations by the whole review committee, and finally, the submission of final scores from all members of the committee. This is similar to the Delphi method, a structured process for eliciting forecasts from a panel of experts, so we believe that it would work well with expected utility forecasting. The primary change would therefore be in the definition and approach for eliciting criterion scores, rather than the structure of the review process. Nevertheless, future implementations may uncover additional considerations that need to be addressed or better ways to incorporate forecasting into a panel environment.
Further investigation into how best to define proposal milestones is also needed. This includes questions such as, who should be responsible for determining the milestones? If reviewers are involved, at what part(s) of the review process should this occur? What is the right balance between precision and flexibility of milestone definitions, such that the best outcomes are achieved? How much flexibility should there be in the number of milestones per proposal?
Lastly, more thought should be given to how to define social impact and how to calibrate reviewers’ interpretation of the impact score scale. In our report, we propose a couple of different options for calibrating impact, in addition to describing the one we took in our pilot.
Interested grantmakers, both public and private, and policymakers are welcome to reach out to our team if interested in learning more or receiving assistance in implementing this approach.
Introduction
The fundamental concern of grantmakers, whether governmental or philanthropic, is how to make the best funding decisions. All funding decisions come with inherent uncertainties that may pose risks to the investment. Thus, a certain level of risk-aversion is natural and even desirable in grantmaking institutions, especially federal science agencies which are responsible for managing taxpayer dollars. However, without risk, there is no reward, so the trade-off must be balanced. In mathematics and economics, expected utility is the common metric assumed to underlie all rational decision making. Expected utility has two components: the probability of an outcome occurring if an action is taken and the value of that outcome, which roughly corresponds with risk and reward. Thus, expected utility would seem to be a logical choice for evaluating science funding proposals.
In the debates around funding innovation though, expected utility has largely flown under the radar compared to other ideas. Nevertheless, Chiara Franzoni and Paula Stephan have proposed using expected utility in peer review. Building off of their paper, the Federation of American Scientists (FAS) developed a detailed framework for how to implement expected utility into a peer review process. We chose to frame the review criteria as forecasting questions, since determining the expected utility of a proposal inherently requires making some predictions about the future. Forecasting questions also have the added benefit of being resolvable–i.e., the true outcome can be determined after the fact and compared to the prediction–which provides a learning opportunity for reviewers to improve their abilities and identify biases. In addition to forecasting, we incorporated other unique features, like an exponential scale for scoring impact, that we believe help reduce biases against risky proposals.
With the theory laid out, we conducted a small pilot in fall of 2023. The pilot was run in collaboration with Metaculus, a crowd forecasting platform and aggregator, to leverage their expertise in designing resolvable forecasting questions and to use their platform to collect forecasts from reviewers. The purpose of the pilot was to test the mechanics of this approach in practice, see if there are any additional considerations that need to be thought through, and surface potential issues that need to be solved for. We were also curious if there would be any interesting or unexpected results that arise based on how we chose to calculate impact and total expected utility. It is important to note that this pilot was not an experiment, so we did not have a control group to compare the results of the review with.
Since FAS is not a grantmaking institution, we did not have a ready supply of traditional grant proposals to use. Instead, we used a set of two-page research proposals for Focused Research Organizations (FROs) that we had sourced through separate advocacy work in that area.1 With the proposal authors’ permission, we recruited a cohort of twenty subject matter experts to each review one of five proposals. For each proposal, we defined two research milestones in consultation with the proposal authors. Reviewers were asked to make three forecasts for each milestone:
- The probability of success;
- The scientific impact, conditional on success; and
- The social impact, conditional on success.
Reviewers submitted their forecasts on Metaculus’ platform; in a separate form they provided explanations for their forecasts and responded to questions about their experience and impression of this new approach to proposal evaluation. (See Appendix A for details on the pilot study design.)
Insights from Reviewer Feedback
Overall, reviewers liked the framing and criteria provided by the expected utility approach, while their main critique was of the structure of the research proposals. Excluding critiques of the research proposal structure, which are unlikely to apply to an actual grant program, two thirds of the reviewers expressed positive opinions of the review process and/or thought it was worth pursuing further given drawbacks with existing review processes. Below, we delve into the details of the feedback we received from reviewers and their implications for future implementation.
Feedback on Review Criteria
Disentangling Impact from Feasibility
Many of the reviewers said that this model prompted them to think differently about how they assess the proposals and that they liked the new questions. Reviewers appreciated that the questions focused their attention on what they think funding agencies really want to know and nothing more: “can it occur?” and “will it matter?” This approach explicitly disentangles impact from feasibility: “Often, these two are taken together, and if one doesn’t think it is likely to succeed, the impact is also seen as lower.” Additionally, the emphasis on big picture scientific and social impact “is often missing in the typical review process.” Reviewers also liked that this approach eliminates what they consider biased metrics, such as the principal investigator’s reputation, track record, and “excellence.”
Reducing Administrative Burden
The small set of questions was seen as more efficient and less burdensome on reviewers. One reviewer said, “I liked this approach to scoring a proposal. It reduces the effort to thinking about perceived impact and feasibility.” Another reviewer said, “On the whole it seems a worthwhile exercise as the current review processes for proposals are onerous.”
Quantitative Forecasting
Reviewers saw benefits to being asked to quantify their assessments, but also found it challenging at times. A number of reviewers enjoyed taking a quantitative approach and thought that it helped them be more grounded and explicit in their evaluations of the proposals. However, some reviewers were concerned that it felt like guesswork and expressed low confidence in their quantitative assessments, primarily due to proposals lacking details on their planned research methods, which is an issue discussed in the section “Feedback on Proposals.” Nevertheless, some of these reviewers still saw benefits to taking a quantitative approach: “It is interesting to try to estimate probabilities, rather than making flat statements, but I don’t think I guess very well. It is better than simply classically reviewing the proposal [though].” Since not all academics have experience making quantitative predictions, we expect that there will be a learning curve for those new to the practice. Forecasting is a skill that can be learned though, and we think that with training and feedback, reviewers can become better, more confident forecasters.
Defining Social Impact
Of the three types of questions that reviewers were asked to answer, the question about social impact seemed the harder one for reviewers to interpret. Reviewers noted that they would have liked more guidance on what was meant by social impact and whether that included indirect impacts. Since questions like these are ultimately subjective, the “right” definition of social impact and what types of outcomes are considered most valuable will depend on the grantmaking institution, their domain area, and their theory of change, so we leave this open to future implementers to clarify in their instructions.
Calibrating Impact
While the impact score scale (see Appendix A) defines the relative difference in impact between scores, it does not define the absolute impact conveyed by a score. For this reason, a calibration mechanism is necessary to provide reviewers with a shared understanding of the use and interpretation of the scoring system. Note that this is a challenge that rubric-based peer review criteria used by science agencies also face. Discussion and aggregation of scores across a review committee helps align reviewers and average out some of this natural variation.2
To address this, we surveyed a small, separate set of academics in the life sciences about how they would score the social and scientific impact of the average NIH R01 grant, which many life science researchers apply to and review proposals for. We then provided the average scores from this survey to reviewers to orient them to the new scale and help them calibrate their scores.
One reviewer suggested an alternative approach: “The other thing I might change is having a test/baseline question for every reviewer to respond to, so you can get a feel for how we skew in terms of assessing impact on both scientific and social aspects.” One option would be to ask reviewers to score the social and scientific impact of the average grant proposal for a grant program that all reviewers would be familiar with; another would be to ask reviewers to score the impact of the average funded grant for a specific grant program, which could be more accessible for new reviewers who have not previously reviewed grant proposals. A third option would be to provide all reviewers on a committee with one or more sample proposals to score and discuss, in a relevant and shared domain area.
When deciding on an approach for calibration, a key consideration is the specific resolution criteria that are being used — i.e., the downstream measures of impact that reviewers are being asked to predict. One option, which was used in our pilot, is to predict the scores that a comparable, but independent, panel of reviewers would give the project some number of years following its successful completion. For a resolution criterion like this one, collecting and sharing calibration scores can help reviewers get a sense for not just their own approach to scoring, but also those of their peers.
Making Funding Decisions
In scoring the social and scientific impact of each proposal, reviewers were asked to assess the value of the proposal to society or to the scientific field. That alone would be insufficient to determine whether a proposal should be funded though, since it would need to be compared with other proposals in conjunction with its feasibility. To do so, we calculated the total expected utility of each proposal (see Appendix C). In a real funding scenario, this final metric could then be used to compare proposals and determine which ones get funded. Additionally, unlike a traditional scoring system, the expected utility approach allows for the detailed comparison of portfolios — including considerations like the expected proportion of milestones reached and the range of likely impacts.
In our pilot, reviewers were not informed that we would be doing this additional calculation based on their submissions. As a result, one reviewer thought that the questions they were asked failed to include other important questions, like “should it occur?” and “is it worth the opportunity cost?” Though these questions were not asked of reviewers explicitly, we believe that they would be answered once the expected utility of all proposals is calculated and considered, since the opportunity cost of one proposal would be the expected utility of the other proposals. Since each reviewer only provided input on one proposal, they may have felt like the scores they gave would be used to make a binary yes/no decision on whether to fund that one proposal, rather than being considered as a part of a larger pool of proposals, as it would be in a real review process.
Feedback on Proposals
Missing Information Impedes Forecasting
The primary critique that reviewers expressed was that the research proposals lacked details about their research plans, what methods and experimental protocols would be used, and what preliminary research the author(s) had done so far. This hindered their ability to properly assess the technical feasibility of the proposals and their probability of success. A few reviewers expressed that they also would have liked to have had a better sense of who would be conducting the research and each team member’s responsibilities. These issues arose because the FRO proposals used in our pilot had not originally been submitted for funding purposes, and thus lacked the requirements of traditional grant proposals, as we noted above. We assume this would not be an issue with proposals submitted to actual grantmakers.3
Improving Milestone Design
A few reviewers pointed out that some of the proposal milestones were too ambiguous or were not worded specifically enough, such that there were ways that researchers could technically say that they had achieved the milestone without accomplishing the spirit of its intent. This made it more challenging for reviewers to assess milestones, since they weren’t sure whether to focus on the ideal (i.e., more impactful) interpretation of the milestone or to account for these “loopholes.” Moreover, loopholes skew the forecasts, since they increase the probability of achieving a milestone, while lowering the impact of doing so if it is achieved through a loophole.
One reviewer suggested, “I feel like the design of milestones should be far more carefully worded – or broken up into sub-sentences/sub-aims, to evaluate the feasibility of each. As the questions are currently broken down, I feel they create a perverse incentive to create a vaguer milestone, or one that can be more easily considered ‘achieved’ for some ‘good enough’ value of achieved.” For example, they proposed that one of the proposal milestones, “screen a library of tens of thousands of phage genes for enterobacteria for interactions and publish promising new interactions for the field to study,” could be expanded to
- “Generate a library of tens of thousands of genes from enterobacteria, expressed in E. coli
- “Validate their expression under screenable conditions
- “Screen the library for their ability to impede phage infection with a panel of 20 type phages
- “Publish …
- “Store and distribute the library, making it as accessible to the broader community”
We agree with the need for careful consideration and design of milestones, given that “loopholes” in milestones can detract from their intended impact and make it harder for reviewers to accurately assess their likelihood. In our theoretical framework for this approach, we identified three potential parties that could be responsible for defining milestones: (1) the proposal author(s), (2) the program manager, with or without input from proposal authors, or (3) the reviewers, with or without input from proposal authors. This critique suggests that the first approach of allowing proposal authors to be the sole party responsible for defining proposal milestones is vulnerable to being gamed, and the second or third approach would be preferable. Program managers who take on the task of defining milestones should have enough expertise to think through the different potential ways of fulfilling a milestone and make sure that they are sufficiently precise for reviewers to assess.
Benefits of Flexibility in Milestones
Some flexibility in milestones may still be desirable, especially with respect to the actual methodology, since experimentation may be necessary to determine the best technique to use. For example, speaking about the feasibility of a different proposal milestone – “demonstrate that Pro-AG technology can be adapted to a single pathogenic bacterial strain in a 300 gallon aquarium of fish and successfully reduce antibiotic resistance by 90%” – a reviewer noted that
“The main complexity and uncertainty around successful completion of this milestone arises from the native fish microbiome and whether a CRISPR delivery tool can reach the target strain in question. Due to the framing of this milestone, should a single strain be very difficult to reach, the authors could simply switch to a different target strain if necessary. Additionally, the mode of CRISPR delivery is not prescribed in reaching this milestone, so the authors have a host of different techniques open to them, including conjugative delivery by a probiotic donor or delivery by engineered bacteriophage.”
Peer Review Results
Sequential Milestones vs. Independent Outcomes
In our expected utility forecasting framework, we defined two different ways that a proposal could structure its outcomes: as sequential milestones where each additional milestone builds off of the success of the previous one, or as independent outcomes where the success of one is not dependent on the success of the other(s). For proposals with sequential milestones in our pilot, we would expect the probability of success of milestone 2 to be less than the probability of success of milestone 1 and for the opposite to be true of their impact scores. For proposals with independent outcomes, we do not expect there to be a relationship between the probability of success and the impact scores of milestones 1 and 2. There are different equations for calculating the total expected utility, depending on the relationship between outcomes (see Appendix C).
For each of the proposals in our study, we categorized them based on whether they had sequential milestones or independent outcomes. This information was not shared with reviewers. Table 1 presents the average reviewer forecasts for each proposal. In general, milestones received higher scientific impact scores than social impact scores, which makes sense given the primarily academic focus of research proposals. For proposals 1 to 3, the probability of success of milestone 2 was roughly half of the probability of success of milestone 1; reviewers also gave milestone 2 higher scientific and social impact scores than milestone 1. This is consistent with our categorization of proposals 1 to 3 as sequential milestones.
Further Discussion on Designing and Categorizing Milestones
We originally categorized proposal 4’s milestones as sequential, but one reviewer gave milestone 2 a lower scientific impact score than milestone 1 and two reviewers gave it a lower social impact score. One reviewer also gave milestone 2 roughly the same probability of success as milestone 1. This suggests that proposal 4’s milestones can’t be considered strictly sequential.
The two milestones for proposal 4 were
- Milestone 1: Develop a tool that is able to perturb neurons in C. elegans and record from all neurons simultaneously, automated w/ microfluidics, and
- Milestone 2: Develop a model of the C. elegans nervous system that can predict what every neuron will do when stimulating one neuron with R2 > 0.8
The reviewer who gave milestone 2 a lower scientific impact score explained: “Given the wording of the milestone, I do not believe that if the scientific milestone was achieved, it would greatly improve our understanding of the brain.” Unlike proposals 1-3, in which milestone 2 was a scaled-up or improved-upon version of milestone 1, these milestones represent fundamentally different categories of output (general-purpose tool vs specific model). Thus, despite the necessity of milestone 1’s tool for achieving milestone 2, the reviewer’s response suggests that the impact of milestone 2 was being considered separately rather than cumulatively.
To properly address this case of sequential milestones with different types of outputs, we recommend that for all sequential milestones, latter milestones should be explicitly defined as inclusive of prior milestones. In the above example, this would imply redefining milestone 2 as “Complete milestone 1 and develop a model of the C. elegans nervous system…” This way, reviewers know to include the impact of milestone 1 in their assessment of the impact of milestone 2.
To help ensure that reviewers are aligned with program managers in how they interpret the proposal milestones (if they aren’t directly involved in defining milestones), we suggest that either reviewers be informed of how program managers are categorizing the proposal outputs so they can conduct their review accordingly or allow reviewers to decide the category (and thus how the total expected utility is calculated), whether individually or collectively or both.
We chose to use only two of the goals that proposal authors provided because we wanted to standardize the number of milestones across proposals. However, this may have provided an incomplete picture of the proposals’ goals, and thus an incomplete assessment of the proposals. We recommend that future implementations be flexible and allow the number of milestones to be determined based on each proposal’s needs. This would also help accommodate one of the reviewers’ suggestion that some milestones should be broken down into intermediary steps.
Importance of Reviewer Explanations
As one can tell from the above discussion, reviewers’ explanation of their forecasts were crucial to understanding how they interpreted the milestones. Reviewers’ explanations varied in length and detail, but the most insightful responses broke down their reasoning into detailed steps and addressed (1) ambiguities in the milestone and how they chose to interpret ambiguities if they existed, (2) the state of the scientific field and the maturity of different techniques that the authors propose to use, and (3) factors that improve the likelihood of success versus potential barriers or challenges that would need to be overcome.
Exponential Impact Scales Better Reflect the Real Distribution of Impact
The distribution of NIH and NSF proposal peer review scores tends to be skewed such that most proposals are rated above the center of the scale and there are few proposals rated poorly. However, other markers of scientific impact, such as citations (even with all of its imperfections), tend to suggest a long tail of studies with high impact. This discrepancy suggests that traditional peer review scoring systems are not well-structured to capture the nonlinearity of scientific impact, resulting in score inflation. The aggregation of scores at the top end of the scale also means that very negative scores have a greater impact than very positive scores when averaged together, since there’s more room between the average score and the bottom end of the scale. This can generate systemic bias against more controversial or risky proposals.
In our pilot, we chose to use an exponential scale with a base of 2 for impact to better reflect the real distribution of scientific impact. Using this exponential impact scale, we conducted a survey of a small pool of academics in the life sciences about how they would rate the impact of the average funded NIH R01 grant. They responded with an average scientific impact score 5 and an average social impact score of 3, which are much lower on our scale compared to traditional peer review scores4, suggesting that the exponential scale may be beneficial for avoiding score inflation and bunching at the top. In our pilot, the distribution of scientific impact scores was centered higher than 5, but still less skewed than NIH peer review scores for significance and innovation typically are. This partially reflects the fact that proposals were expected to be funded at one to two orders of magnitude more than NIH R01 proposals are, so impact should also be greater. The distribution of social impact scores exhibits a much wider spread and lower center.
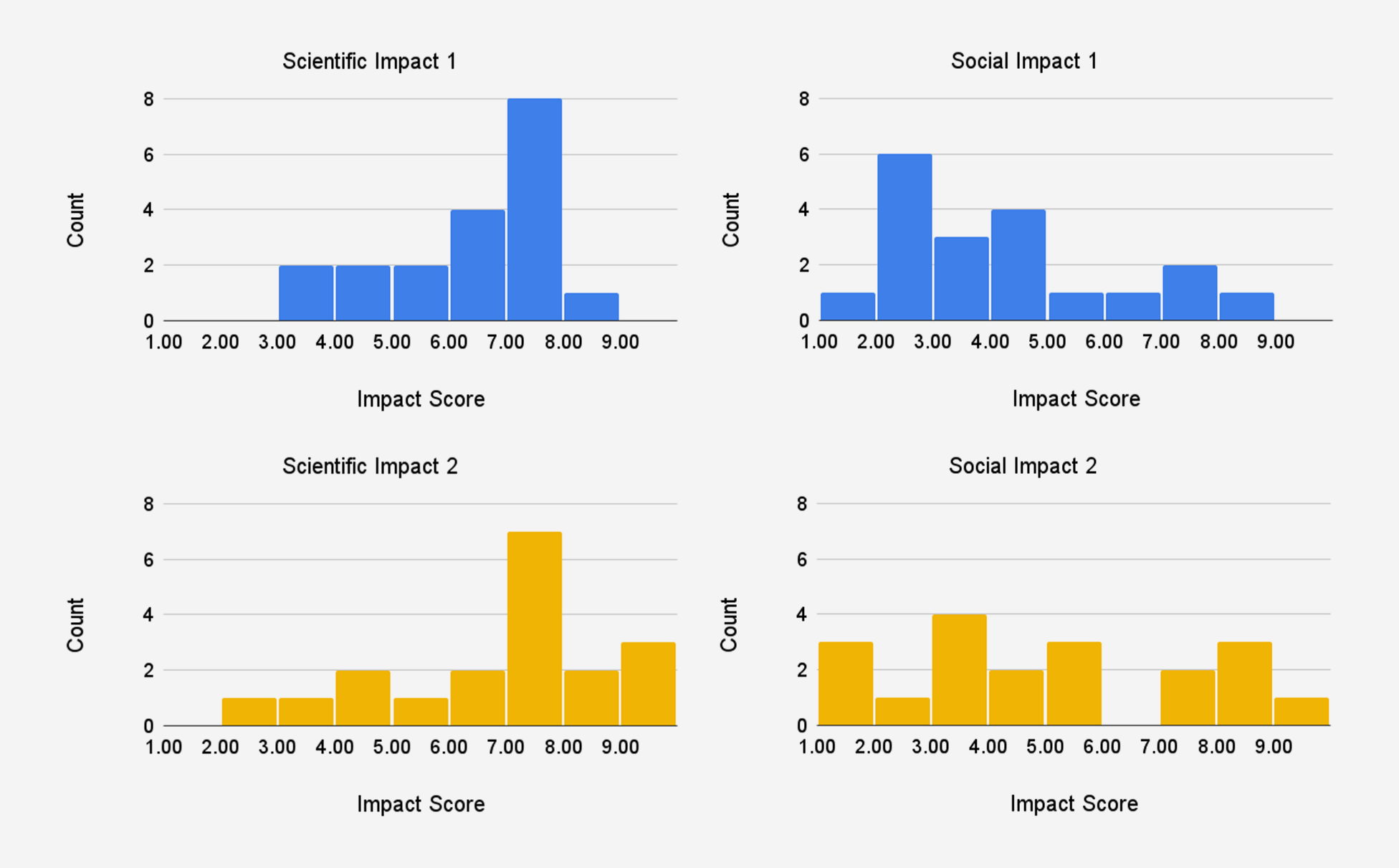
Conclusion
In summary, expected utility forecasting presents a promising approach to improving the rigor of peer review and quantitatively defining the risk-reward profile of science proposals. Our pilot study suggests that this approach can be quite user-friendly for reviewers, despite its apparent complexity. Further study into how best to integrate forecasting into panel environments, define proposal milestones, and calibrate impact scales will help refine future implementations of this approach.
More broadly, we hope that this pilot will encourage more grantmaking institutions to experiment with innovative funding mechanisms. Reviewers in our pilot were more open-minded and quick-to-learn than one might expect and saw significant value in this unconventional approach. Perhaps this should not be so much of a surprise given that experimentation is at the heart of scientific research.
Interested grantmakers, both public and private, and policymakers are welcome to reach out to our team if interested in learning more or receiving assistance in implementing this approach.
Acknowledgements
Many thanks to Jordan Dworkin for being an incredible thought partner in designing the pilot and providing meticulous feedback on this report. Your efforts made this project possible!
Appendix A: Pilot Study Design
Our pilot study consisted of five proposals for life science-related Focused Research Organizations (FROs). These proposals were solicited from academic researchers by FAS as part of our advocacy for the concept of FROs. As such, these proposals were not originally intended as proposals for direct funding, and did not have as strict content requirements as traditional grant proposals typically do. Researchers were asked to submit one to two page proposals discussing (1) their research concept, (2) the motivation and its expected social and scientific impact, and (3) the rationale for why this research can not be accomplished through traditional funding channels and thus requires a FRO to be funded.
Permission was obtained from proposal authors to use their proposals in this study. We worked with proposal authors to define two milestones for each proposal that reviewers would assess: one that they felt confident that they could achieve and one that was more ambitious but that they still thought was feasible. In addition, due to the brevity of the proposals, we included an additional 1-2 pages of supplementary information and scientific context. Final drafts of the milestones and supplementary information were provided to authors to edit and approve. Because this pilot study could not provide any actual funding to proposal authors, it was not possible to solicit full length research proposals from proposal authors.
We recruited four to six reviewers for each proposal based on their subject matter expertise. Potential participants were recruited over email with a request to help review a FRO proposal related to their area of research. They were informed that the review process would be unconventional but were not informed of the study’s purpose. Participants were offered a small monetary compensation for their time.
Confirmed participants were sent instructions and materials for the review process on the same day and were asked to complete their review by the same deadline a month and a half later. Reviewers were told to assume that, if funded, each proposal would receive $50 million in funding over five years to conduct the research, consistent with the proposed model for FROs. Each proposal had two technical milestones, and reviewers were asked to answer the following questions for each milestone:
- Assuming that the proposal is funded by 2025, will the milestone be achieved before 2031?
- What will be the average scientific impact score, as judged in 2032, of accomplishing the milestone?
- What will be the average social impact score, as judged in 2032, of accomplishing the milestone?
The impact scoring system was explained to reviewers as follows:
Please consider the following in determining the impact score: the current and expected long-term social or scientific impact of a funded FRO’s outputs if a funded FRO accomplishes this milestone before 2030.
The impact score we are using ranges from 1 (low) to 10 (high). It is base 2 exponential, meaning that a proposal that receives a score of 5 has double the impact of a proposal that receives a score of 4, and quadruple the impact of a proposal that receives a score of 3. In a small survey we conducted of SMEs in the life sciences, they rated the scientific and social impact of the average NIH R01 grant — a federally funded research grant that provides $1-2 million for a 3-5 year endeavor — on this scale to be 5.2 ± 1.5 and 3.1 ± 1.3, respectively. The median scores were 4.75 and 3.00, respectively.
Below is an example of how a predicted impact score distribution (left) would translate into an actual impact distribution (right). You can try it out yourself with this interactive version (in the menu bar, click Runtime > Run all) to get some further intuition on how the impact score works. Please note that this is meant solely for instructive purposes, and the interface is not designed to match Metaculus’ interface.
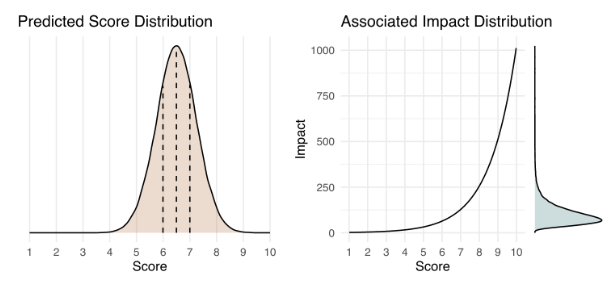
The choice of an exponential impact scale reflects the tendency in science for a small number of research projects to have an outsized impact. For example, studies have shown that the relationship between the number of citations for a journal article and its percentile rank scales exponentially.
Scientific impact aims to capture the extent to which a project advances the frontiers of knowledge, enables new discoveries or innovations, or enhances scientific capabilities or methods. Though each is imperfect, one could consider citations of papers, patents on tools or methods, or users of software or datasets as proxies of scientific impact.
Social impact aims to capture the extent to which a project contributes to solving important societal problems, improving well-being, or advancing social goals. Some proxy metrics that one might use to assess a project’s social impact are the value of lives saved, the cost of illness prevented, the number of job-years of employment generated, economic output in terms of GDP, or the social return on investment.
You may consider any or none of these proxy metrics as a part of your assessment of the impact of a FRO accomplishing this milestone.
Reviewers were asked to submit their forecasts on Metaculus’ website and to provide their reasoning in a separate Google form. For question 1, reviewers were asked to respond with a single probability. For questions 2 and 3, reviewers were asked to provide their median, 25th percentile, and 75th percentile predictions, in order to generate a probability distribution. Metaculus’ website also included information on the resolution criteria of each question, which provided guidance to reviewers on how to answer the question. Individual reviewers were blind to other reviewers’ responses until after the submission deadline, at which point the aggregated results of all of the responses were made public on Metaculus’ website.
Additionally, in the Google form, reviewers were asked to answer a survey question about their experience: “What did you think about this review process? Did it prompt you to think about the proposal in a different way than when you normally review proposals? If so, how? What did you like about it? What did you not like? What would you change about it if you could?”
Some participants did not complete their review. We received 19 complete reviews in the end, with each proposal receiving three to six reviews.
Study Limitations
Our pilot study had certain limitations that should be noted. Since FAS is not a grantmaking institution, we could not completely reproduce the same types of research proposals that a grantmaking institution would receive nor the entire review process. We will highlight these differences in comparison to federal science agencies, which are our primary focus.
- Review Process: There are typically two phases to peer review at NIH and NSF. First, at least three individual reviewers with relevant subject matter expertise are assigned to read and evaluate a proposal independently. Then, a larger committee of experts is convened. There, the assigned reviewers present the proposal and their evaluation, and then the committee discusses and determines the final score for the proposal. Our pilot study only attempted to replicate the first phase of individual review.
- Sample Size: In our pilot, the sample size was quite small, since only five proposals were reviewed, and they were all in different subfields, so different reviewers were assigned to each proposal. NIH and NSF peer review committees typically focus on one subfield and review on the order of twenty or so proposals. The number of reviewers per proposal–three to six–in our pilot was consistent with the number of reviewers typically assigned to a proposal by NIH and NSF. Peer review committees are typically larger, ranging from six to twenty people, depending on the agency and the field.
- Proposals: The FRO proposals plus supplementary information were only two to four pages long, which is significantly shorter than the 12 to 15 page proposals that researchers submit for NIH and NSF grants. Proposal authors were asked to generally describe their research concept, but were not explicitly required to describe the details of the research methodology they would use or any preliminary research. Some proposal authors volunteered more information on this for the supplementary information, but not all authors did.
- Grant Size: For the FRO proposals, reviewers were asked to assume that funded proposals would receive $50 million over five years, which is one to two orders of magnitude more funding than typical NIH and NSF proposals.
Appendix B: Feedback on Study-Specific Implementation
In addition to feedback about the review framework, we received feedback on how we implemented our pilot study, specifically the instructions and materials for the review process and the submission platforms. This feedback isn’t central to this paper’s investigation of expected value forecasting, but we wanted to include it in the appendix for transparency.
Reviewers were sent instructions over email that outlined the review process and linked to Metaculus’ webpage for this pilot. On Metaculus’ website, reviewers could find links to the proposals on FAS’ website and the supplementary information in Google docs. Reviewers were expected to read those first and then read through the resolution criteria for each forecasting question before submitting their answers on Metaculus’ platform. Reviewers were asked to submit the explanations behind their forecasts in a separate Google form.
Some reviewers had no problem navigating the review process and found Metaculus’ website easy to use. However, feedback from other reviewers suggested that the different components necessary for the review were spread out over too many different websites, making it difficult for reviewers to keep track of where to find everything they needed.
Some had trouble locating the different materials and pieces of information needed to conduct the review on Metaculus’ website. Others found it confusing to have to submit their forecasts and explanations in two separate places. One reviewer suggested that the explanation of the impact scoring system should have been included within the instructions sent over email rather than in the resolution criteria on Metaculus’ website so that they could have read it before reading the proposal. Another reviewer suggested that it would have been simpler to submit their forecasts through the same Google form that they used to submit their explanations rather than through Metaculus’ website.
Based on this feedback, we would recommend that future implementations streamline their submission process to a single platform and provide a more extensive set of instructions rather than seeding information across different steps of the review process. Training sessions, which science funding agencies typically conduct, would be a good supplement to written instructions.
Appendix C: Total Expected Utility Calculations
To calculate the total expected utility, we first converted all of the impact scores into utility by taking two to the exponential of the impact score, since the impact scoring system is base 2 exponential:
Utility=2Impact Score.
We then were able to average the utilities for each milestone and conduct additional calculations.
To calculate the total utility of each milestone, ui, we averaged the social utility and the scientific utility of the milestone:
ui = (Social Utility + Scientific Utility)/2.
The total expected utility (TEU) of a proposal with two milestones can be calculated according to the general equation:
TEU = u1P(m1 ∩ not m2) + u2P(m2 ∩ not m1) + (u1+u2)P(m1m2),
where P(mi) represents the probability of success of milestone i and
P(m1 ∩ not m2) = P(m1) – P(m1 ∩ m2)
P(m2 ∩ not m1) = P(m2) – P(m1 ∩ m2).
For sequential milestones, milestone 2 is defined as inclusive of milestone 1 and wholly dependent on the success of milestone 1, so this means that
u2, seq = u1+u2
P(m2) = Pseq(m1 ∩ m2)
P(m2 ∩ not m1) = 0.
Thus, the total expected utility of sequential milestones can be simplified as
TEU = u1P(m1)-u1P(m2) + (u2, seq)P(m2)
TEU = u1P(m1) + (u2, seq-u1)P(m2)
This can be generalized to
TEUseq = Σi(ui, seq-ui-1, seq)P(mi).
Otherwise, the total expected utility can be simplified to
TEU = u1P(m1) + u2P(m2) – (u1+u2)P(m1 ∩ m2).
For independent outcomes, we assume
Pind(m1 ∩ m2) = P(m1)P(m2),
so
TEUind = u1P(m1) + u2P(m2) – (u1+u2)P(m1)P(m2).
To present the results in Tables 1 and 2, we converted all of the utility values back into the impact score scale by taking the log base 2 of the results.
Risk and Reward in Peer Review
This article was written as a part of the FRO Forecasting project, a partnership between the Federation of American Scientists and Metaculus. This project aims to conduct a pilot study of forecasting as an approach for assessing the scientific and societal value of proposals for Focused Research Organizations. To learn more about the project, see the press release here. To participate in the pilot, you can access the public forecasting tournament here.
The United States federal government is the single largest funder of scientific research in the world. Thus, the way that science agencies like the National Science Foundation and the National Institutes of Health distribute research funding has a significant impact on the trajectory of science as a whole. Peer review is considered the gold standard for evaluating the merit of scientific research proposals, and agencies rely on peer review committees to help determine which proposals to fund. However, peer review has its own challenges. It is a difficult task to balance science agencies’ dual mission of protecting government funding from being spent on overly risky investments while also being ambitious in funding proposals that will push the frontiers of science, and research suggests that peer review may be designed more for the former rather than the latter. We at FAS are exploring innovative approaches to peer review to help tackle this challenge.
Biases in Peer Review
A frequently echoed concern across the scientific and metascientific community is that funding agencies’ current approach to peer review of science proposals tends to be overly risk-averse, leading to bias against proposals that entail high risk or high uncertainty about the outcomes. Reasons for this conservativeness include reviewer preferences for feasibility over potential impact, contagious negativity, and problems with the way that peer review scores are averaged together.
This concern, alongside studies suggesting that scientific progress is slowing down, has led to a renewed effort to experiment with new ways of conducting peer review, such as golden tickets and lottery mechanisms. While golden tickets and lottery mechanisms aim to complement traditional peer review with alternate means of making funding decisions — namely individual discretion and randomness, respectively — they don’t fundamentally change the way that peer review itself is conducted.
Traditional peer review asks reviewers to assess research proposals based on a rubric of several criteria, which typically include potential value, novelty, feasibility, expertise, and resources. These criteria are given a score based on a numerical scale; for example, the National Institutes of Health uses a scale from 1 (best) to 9 (worst). Reviewers then provide an overall score that need not be calculated in any specific way based on the criteria scores. Next, all of the reviewers convene to discuss the proposal and submit their final overall scores, which may be different from what they submitted prior to the discussion. The final overall scores are averaged across all of the reviewers for a specific proposal. Proposals are then ranked based on their average overall score and funding is prioritized for those ranked before a certain cutoff score, though depending on the agency, some discretion by program administrators is permitted.
The way that this process is designed allows for the biases mentioned at the beginning—reviewer preferences for feasibility, contagious negativity, and averaging problems—to influence funding decisions. First, reviewer discretion in deciding overall scores allows them to weigh feasibility more heavily than potential impact and novelty in their final scores. Second, when evaluations are discussed reviewers tend to adjust their scores to better align with their peers. This adjustment tends to be greater when correcting in the negative direction than in the positive direction, resulting in a stronger negative bias. Lastly, since funding tends to be quite limited, cutoff scores tend to be quite close to the best score. This means that even if almost all of the reviewers rate a proposal positively, one very negative review can potentially bring the average below the cutoff.
Designing a New Approach to Peer Review
In 2021, the researchers Chiara Franzoni and Paula Stephan published a working paper arguing that risk in science results from three sources of uncertainty: uncertainty of research outcomes, uncertainty of the probability of success, and uncertainty of the value of the research outcomes. To comprehensively and consistently account for these sources of uncertainty, they proposed a new expected utility approach to peer review evaluations, in which reviewers are asked to
- Identify the primary expected outcome of a research proposal and, optionally, a potential secondary outcome;
- Assess the probability between 0 to 1 of achieving each expected outcome (P(j); and
- Assess the value of achieving each expected outcome (uj) on a numerical scale (e.g., 0 to 100).
From this, the total expected utility can be calculated for each proposal and used to rank them.1 This systematic approach addresses the first bias we discussed by limiting the extent to which reviewers’ preferences for more feasible proposals would impact the final score of each proposal.
We at FAS see a lot of potential in Franzoni and Stephan’s expected value approach to peer review, and it inspired us to design a pilot study using a similar approach that aims to chip away at the other biases in review.
To explore potential solutions for negativity bias, we are taking a cue from forecasting by complementing the peer review process with a resolution and scoring process. This means that at a set time in the future, reviewers’ assessments will be compared to a ground truth based on the actual events that have occurred (i.e., was the outcome actually achieved and, if so, what was its actual impact?). Our theory is that if implemented in peer review, resolution and scoring could incentivize reviewers to make better, more accurate predictions over time and provide empirical estimates of a committee’s tendency to provide overly negative (or positive) assessments, thus potentially countering the effects of contagion during review panels and helping more ambitious proposals secure support.
Additionally, we sought to design a new numerical scale for assessing the value or impact of a research proposal, which we call an impact score. Typically, peer reviewers are free to interpret the numerical scale for each criteria as they wish; Franzoni and Stephan’s design also did not specify how the numerical scale for the value of the research outcome should work. We decided to use a scale ranging from 1 (low) to 10 (high) that was base 2 exponential, meaning that a proposal that receives a score of 5 has double the impact of a proposal that receives a score of 4, and quadruple the impact of a proposal that receives a score of 3.
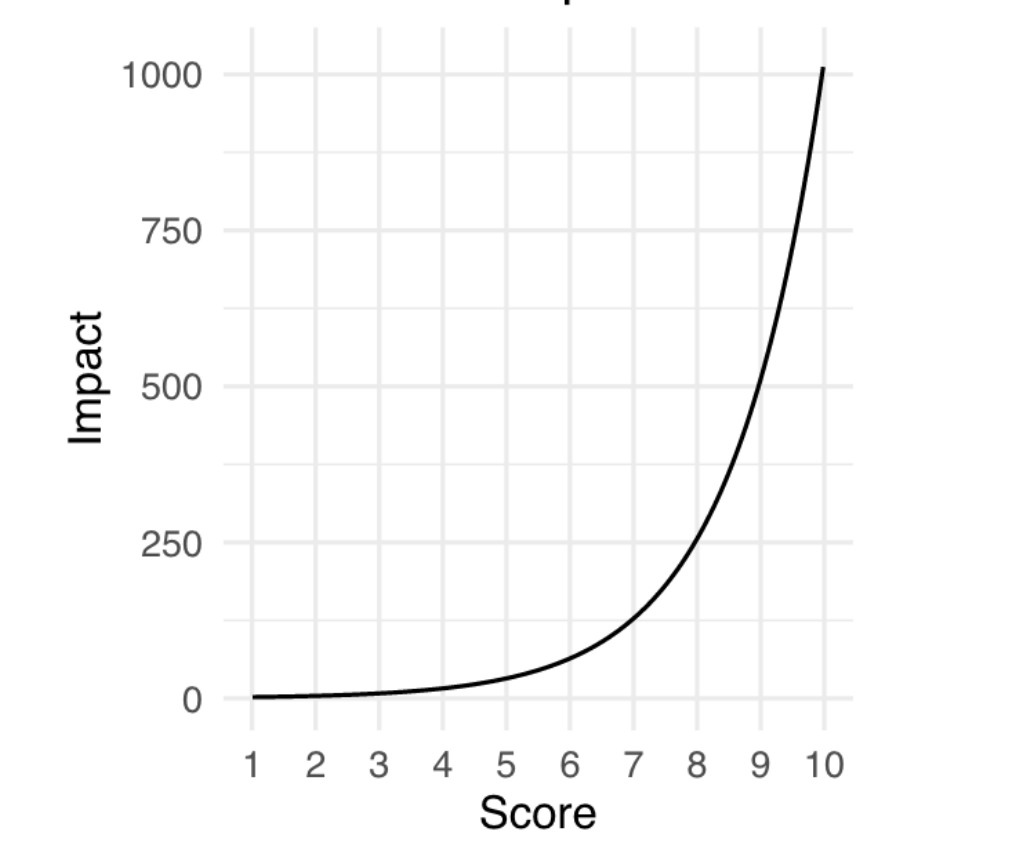
The choice of an exponential scale reflects the tendency in science for a small number of research projects to have an outsized impact (Figure 2), and provides more room at the top end of the scale for reviewers to increase the rating of the proposals that they believe will have an exceptional impact. We believe that this could help address the last bias we discussed, which is that currently, bad scores are more likely to pull a proposal’s average below the cutoff than good scores are likely to pull a proposal’s average above the cutoff.
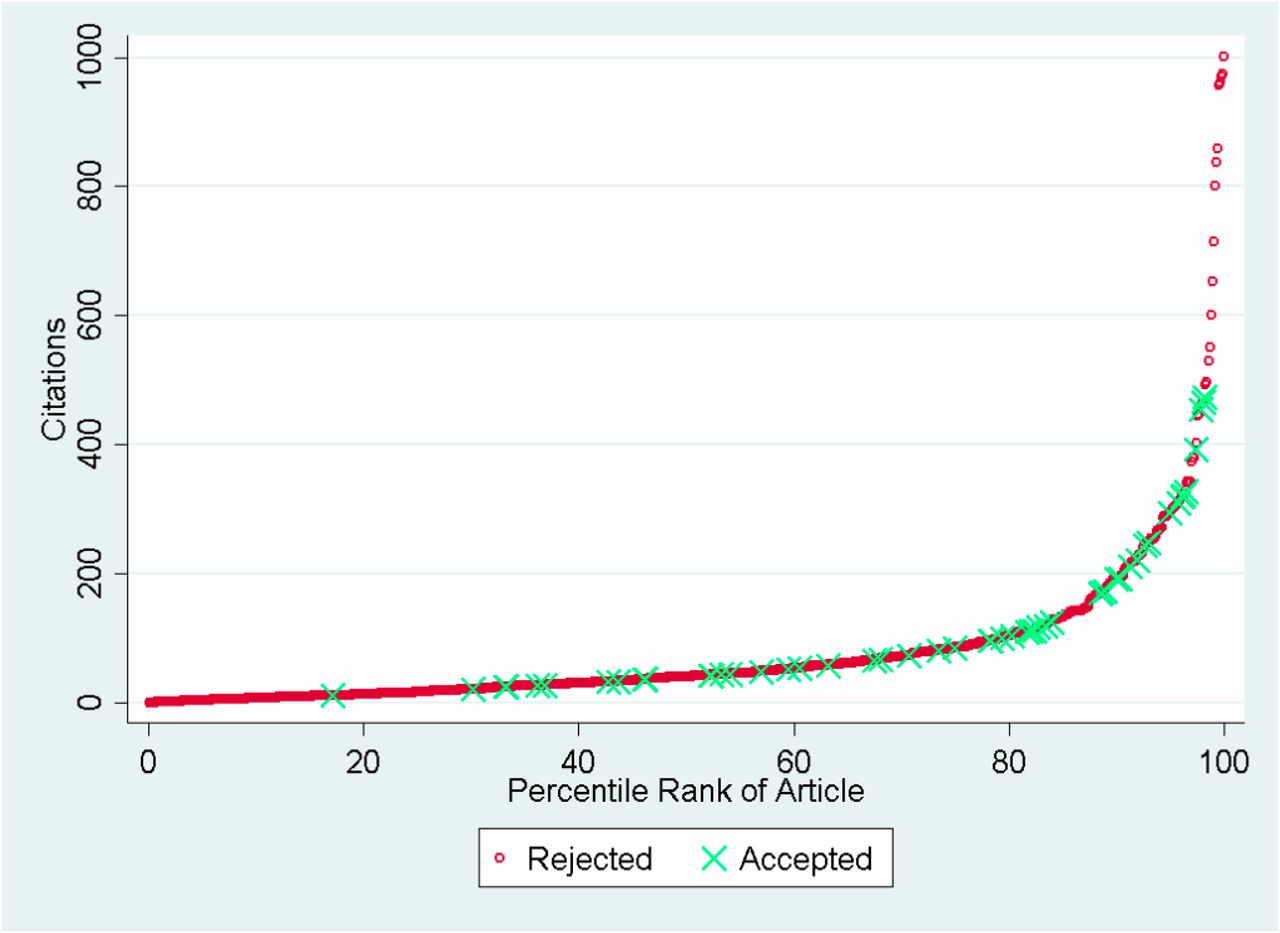
Source: Siler, Lee, and Bero (2014)
We are now piloting this approach on a series of proposals in the life sciences that we have collected for Focused Research Organizations, a new type of non-profit research organization designed to tackle challenges that neither academia or industry is incentivized to work on. The pilot study was developed in collaboration with Metaculus, a forecasting platform and aggregator, and will be hosted on their website. We welcome subject matter experts in the life sciences — or anyone interested! — to participate in making forecasts on these proposals here. Stay tuned for the results of this pilot, which we will publish in a report early next year.
FY24 NDAA AI Tracker
As both the House and Senate gear up to vote on the National Defense Authorization Act (NDAA), FAS is launching this live blog post to track all proposals around artificial intelligence (AI) that have been included in the NDAA. In this rapidly evolving field, these provisions indicate how AI now plays a pivotal role in our defense strategies and national security framework. This tracker will be updated following major updates.
Senate NDAA. This table summarizes the provisions related to AI from the version of the Senate NDAA that advanced out of committee on July 11. Links to the section of the bill describing these provisions can be found in the “section” column. Provisions that have been added in the manager’s package are in red font. Updates from Senate Appropriations committee and the House NDAA are in blue.
House NDAA. This table summarizes the provisions related to AI from the version of the House NDAA that advanced out of committee. Links to the section of the bill describing these provisions can be found in the “section” column.
Funding Comparison. The following tables compare the funding requested in the President’s budget to funds that are authorized in current House and Senate versions of the NDAA. All amounts are in thousands of dollars.
On Senate Approps Provisions
The Senate Appropriations Committee generally provided what was requested in the White House’s budget regarding artificial intelligence (AI) and machine learning (ML), or exceeded it. AI was one of the top-line takeaways from the Committee’s summary of the defense appropriations bill. Particular attention has been paid to initiatives that cut across the Department of Defense, especially the Chief Digital and Artificial Intelligence Office (CDAO) and a new initiative called Alpha-1. The Committee is supportive of Joint All-Domain Command and Control (JADC2) integration and the recommendations of the National Security Commission on Artificial Intelligence (NSCAI).
On House final bill provisions
Like the Senate Appropriations bill, the House of Representatives’ final bill generally provided or exceeded what was requested in the White House budget regarding AI and ML. However, in contract to the Senate Appropriations bill, AI was not a particularly high-priority takeaway in the House’s summary. The only note about AI in the House Appropriations Committee’s summary of the bill was in the context of digital transformation of business practices. Program increases were spread throughout the branches’ Research, Development, Test, and Evaluation budgets, with a particular concentration of increased funding for the Defense Innovation Unit’s AI-related budget.
How to Replicate the Success of Operation Warp Speed
Operation Warp Speed (OWS) was a public-private partnership that produced COVID-19 vaccines in the unprecedented timeline of less than one year. This unique success among typical government research and development (R&D) programs is attributed to OWS’s strong public-private partnerships, effective coordination, and command leadership structure. Policy entrepreneurs, leaders of federal agencies, and issue advocates will benefit from understanding what policy interventions were used and how they can be replicated. Those looking to replicate this success should evaluate the stakeholder landscape and state of the fundamental science before designing a portfolio of policy mechanisms.
Challenge and Opportunity
Development of a vaccine to protect against COVID-19 began when China first shared the genetic sequence in January 2020. In May, the Trump Administration announced OWS to dramatically accelerate development and distribution. Through the concerted efforts of federal agencies and private entities, a vaccine was ready for the public in January 2021, beating the previous record for vaccine development by about three years. OWS released over 63 million doses within one year, and to date more than 613 million doses have been administered in the United States. By many accounts, OWS was the most effective government-led R&D effort in a generation.
Policy entrepreneurs, leaders of federal agencies, and issue advocates are interested in replicating similarly rapid R&D to solve problems such as climate change and domestic manufacturing. But not all challenges are suited for the OWS treatment. Replicating its success requires an understanding of the unique factors that made OWS possible, which are addressed in Recommendation 1. With this understanding, the mechanisms described in Recommendation 2 can be valuable interventions when used in a portfolio or individually.
Plan of Action
Recommendation 1. Assess whether (1) the majority of existing stakeholders agree on an urgent and specific goal and (2) the fundamental research is already established.
Criteria 1. The majority of stakeholders—including relevant portions of the public, federal leaders, and private partners—agree on an urgent and specific goal.
The OWS approach is most appropriate for major national challenges that are self-evidently important and urgent. Experts in different aspects of the problem space, including agency leaders, should assess the problem to set ambitious and time-bound goals. For example, OWS was conceptualized in April and announced in May, and had the specific goal of distributing 300 million vaccine doses by January.
Leaders should begin by assessing the stakeholder landscape, including relevant portions of the public, other federal leaders, and private partners. This assessment must include adoption forecasts that consider the political, regulatory, and behavioral contexts. Community engagement—at this stage and throughout the process—should inform goal-setting and program strategy. Achieving ambitious goals will require commitment from multiple federal agencies and the presidential administration. At this stage, understanding the private sector is helpful, but these stakeholders can be motivated further with mechanisms discussed later. Throughout the program, leaders must communicate the timeline and standards for success with expert communities and the public.
Example Challenge: Building Capability for Domestic Rare Earth Element Extraction and Processing |
Rare earth elements (REEs) have unique properties that make them valuable across many sectors, including consumer electronics manufacturing, renewable and nonrenewable energy generation, and scientific research. The U.S. relies heavily on China for the extraction and processing of REEs, and the U.S. Geological Survey reports that 78% of our REEs were imported from China from 2017-2020. Disruption to this supply chain, particularly in the case of export controls enacted by China as foreign policy, would significantly disrupt the production of consumer electronics and energy generation equipment critical to the U.S. economy. Export controls on REEs would create an urgent national problem, making it suitable for an OWS-like effort to build capacity for domestic extraction and processing. |
Criteria 2. Fundamental research is already established, and the goal requires R&D to advance for a specific use case at scale.
Efforts modeled after OWS should require fundamental research to advance or scale into a product. For example, two of the four vaccine platforms selected for development in OWS were mRNA and replication-defective live vector platforms, which had been extensively studied despite never being used in FDA-licensed vaccines. Research was advanced enough to give leaders confidence to bet on these platforms as candidates for a COVID-19 vaccine. To mitigate risk, two more-established platforms were also selected.
Technology readiness levels (TRLs) are maturity level assessments of technologies for government acquisition. This framework can be used to assess whether a candidate technology should be scaled with an OWS-like approach. A TRL of at least five means the technology was successfully demonstrated in a laboratory environment as part of an integrated or partially integrated system. In evaluating and selecting candidate technologies, risk is unavoidable, but decisions should be made based on existing science, data, and demonstrated capabilities.
Example Challenge: Scaling Desalination to Meet Changing Water Demand |
Increases in efficiency and conservation efforts have largely kept the U.S.’s total water use flat since the 1980s, but drought and climate variability are challenging our water systems. Desalination, a well-understood process to turn seawater into freshwater, could help address our changing water supply. However, all current desalination technologies applied in the U.S. are energy intensive and may negatively impact coastal ecosystems. Advanced desalination technologies—such as membrane distillation, advanced pretreatment, and advanced membrane cleaning, all of which are at technology readiness levels of 5–6—would reduce the total carbon footprint of a desalination plant. An OWS for desalination could increase the footprint of efficient and low-carbon desalination plants by speeding up development and commercialization of advanced technologies. |
Recommendation 2: Design a program with mechanisms most needed to achieve the goal: (1) establish a leadership team across federal agencies, (2) coordinate federal agencies and the private sector, (3) activate latent private-sector capacities for labor and manufacturing, (4) shape markets with demand-pull mechanisms, and (5) reduce risk with diversity and redundancy.
Design a program using a combination of the mechanisms below, informed by the stakeholder and technology assessment. The organization of R&D, manufacturing, and deployment should follow an agile methodology in which more risk than normal is accepted. The program framework should include criteria for success at the end of each sprint. During OWS, vaccine candidates were advanced to the next stage based on the preclinical or early-stage clinical trial data on efficacy; the potential to meet large-scale clinical trial benchmarks; and criteria for efficient manufacturing.
Mechanism 1: Establish a leadership team across federal agencies
Establish an integrated command structure co-led by a chief scientific or technical advisor and a chief operating officer, a small oversight board, and leadership from federal agencies. The team should commit to operate as a single cohesive unit despite individual affiliations. Since many agencies have limited experience in collaborating on program operations, a chief operating officer with private-sector experience can help coordinate and manage agency biases. Ideally, the team should have decision-making authority and report directly to the president. Leaders should thoughtfully delegate tasks, give appropriate credit for success, hold themselves and others accountable, and empower others to act.
The OWS team was led by personnel from the Department of Health and Human Services (HHS), the Department of Defense (DOD), and the vaccine industry. It included several HHS offices at different stages: the Centers for Disease Control and Prevention (CDC), the Food and Drug Administration (FDA), the National Institutes of Health (NIH), and the Biomedical Advanced Research and Development Authority (BARDA). This structure combined expertise in science and manufacturing with the power and resources of the DOD. The team assigned clear roles to agencies and offices to establish a chain of command.
Example Challenge: Managing Wildland Fire with Uncrewed Aerial Systems (UAS) |
Wildland fire is a natural and normal ecological process, but the changing climate and our policy responses are causing more frequent, intense, and destructive fires. Reducing harm requires real-time monitoring of fires with better detection technology and modernized equipment such as UAS. Wildfire management is a complex policy and regulatory landscape with functions spanning multiple federal, state, and local entities. Several interagency coordination bodies exist, including the National Wildfire Coordinating Group, Wildland Fire Leadership Council, and the Wildland Fire Mitigation and Management Commission, but much of these efforts are consensus-based coordination models. The status quo and historical biases against agencies have created silos of effort and prevent technology from scaling to the level required. An OWS for wildland fire UAS would establish a public-private partnership led by experienced leaders from federal agencies, state and local agencies, and the private sector to advance this technology development. The team would motivate commitment to the challenge across government, academia, nonprofits, and the private sector to deliver technology that meets ambitious goals. Appropriate teams across agencies would be empowered to refocus their efforts during the duration of the challenge. |
Mechanism 2: Coordinate federal agencies and the private sector
Coordinate agencies and the private sector on R&D, manufacturing, and distribution, and assign responsibilities based on core capabilities rather than political or financial considerations. Identify efficiency improvements by mapping processes across the program. This may include accelerating regulatory approval by facilitating communication between the private sector and regulators or by speeding up agency operations. Certain regulations may be suspended entirely if the risks are considered acceptable relative to the urgency of the goal. Coordinators should identify processes that can occur in parallel rather than sequentially. Leaders can work with industry so that operations occur under minimal conditions to ensure worker and product safety.
The OWS team worked with the FDA to compress traditional approval timelines by simultaneously running certain steps of the clinical trial process. This allowed manufacturers to begin industrial-scale vaccine production before full demonstration of efficacy and safety. The team continuously sent data to FDA while they completed regulatory procedures in active communication with vaccine companies. Direct lines of communication permitted parallel work streams that significantly reduced the normal vaccine approval timeline.
Example Challenge: Public Transportation and Interstate Rail |
Much of the infrastructure across the United States needs expensive repairs, but the U.S. has some of the highest infrastructure construction costs for its GDP and longest construction times. A major contributor to costs and time is the approval process with extensive documentation, such as preparing an environmental impact study to comply with the National Environmental Policy Act. An OWS-like coordinating body could identify key pieces of national infrastructure eligible for support, particularly for near-end-of-lifespan infrastructure or major transportation arteries. Reducing regulatory burden for selected projects could be achieved by coordinating regulatory approval in close collaboration with the Department of Transportation, the Environmental Protection Agency, and state agencies. The program would need to identify and set a precedent for differentiating between expeditable regulations and key regulations, such as structural reviews, that could serve as bottlenecks. |
Mechanism 3: Activate latent private-sector capacities for labor and manufacturing
Activate private-sector capabilities for production, supply chain management, deployment infrastructure, and workforce. Minimize physical infrastructure requirements, establish contracts with companies that have existing infrastructure, and fund construction to expand facilities where necessary. Coordinate with the Department of State to expedite visa approval for foreign talent and borrow personnel from other agencies to fill key roles temporarily. Train staff quickly with boot camps or accelerators. Efforts to build morale and ensure commitment are critical, as staff may need to work holidays or perform higher than normally expected. Map supply chains, identify critical components, and coordinate supply. Critical supply chain nodes should be managed by a technical expert in close partnership with suppliers. Use the Defense Production Act sparingly to require providers to prioritize contracts for procurement, import, and delivery of equipment and supplies. Map the distribution chain from the manufacturer to the endpoint, actively coordinate each step, and anticipate points of failure.
During OWS, the Army Corps of Engineers oversaw construction projects to expand vaccine manufacturing capacity. Expedited visa approval brought in key technicians and engineers for installing, testing, and certifying equipment. Sixteen DOD staff also served in temporary quality-control positions at manufacturing sites. The program established partnerships between manufacturers and the government to address supply chain challenges. Experts from BARDA worked with the private sector to create a list of critical supplies. With this supply chain mapping, the DOD placed prioritized ratings on 18 contracts using the Defense Production Act. OWS also coordinated with DOD and U.S. Customs to expedite supply import. OWS leveraged existing clinics at pharmacies across the country and shipped vaccines in packages that included all supplies needed for administration, including masks, syringes, bandages, and paper record cards.
Example Challenge: EV Charging Network |
Electric vehicles (EVs) are becoming increasingly popular due to high gas prices and lower EV prices, stimulated by tax credits for both automakers and consumers in the Inflation Reduction Act. Replacing internal combustion engine vehicles with EVs is aligned with our current climate commitments and reduces overall carbon emissions, even when the vehicles are charged with energy from nonrenewable sources. Studies suggest that current public charging infrastructure has too few functional chargers to meet the demand of EVs currently on the road. Reliable and available public chargers are needed to increase public confidence in EVs as practical replacements for gas vehicles. Leveraging latent private-sector capacity could include expanding the operations of existing charger manufacturers, coordinating the deployment and installation of charging stations and requisite infrastructure, and building a skilled workforce to repair and maintain this new infrastructure. In February 2023 the Biden Administration announced actions to expand charger availability through partnerships with over 15 companies. |
Mechanism 4: Shape markets with demand-pull mechanisms
Use contracts and demand-pull mechanisms to create demand and minimize risks for private partners. Other Transaction Authority can also be used to procure capabilities quickly by bypassing elements of the Federal Acquisition Regulation. The types of demand-pull mechanisms available to agencies are:
- Volume guarantees: Commits the buyer (i.e., a federal agency) to purchase a minimum quantity of an existing product at a set price from multiple vendors.
- Advance purchase agreements: Establishes a contract between a single buyer and a single supplier in which the buyer provides advance funding for resources to manufacture a product or provide a service.
- Advance market commitments: Engages multiple suppliers or producers to produce a product or service by providing advance funds.
- Prize competitions: Solicits the development of creative solutions for a particular, well-defined problem from a wide range of actors, including individuals, companies, academic teams, and more, and rewards them with a cash prize.
- Challenge-based acquisitions: Solicits creative solutions for a well-defined problem and rewards success by purchasing the solution.
- Milestone payments: Provide a series of payments contingent on achieving defined objectives through the contract timeline.
HHS used demand-pull mechanisms to develop the vaccine candidates during OWS. This included funding large-scale manufacturing and committing to purchase successful vaccines. HHS made up to $483 million in support available for Phase 1 trials of Moderna’s mRNA candidate vaccine. This agreement was increased by $472 million for late-stage clinical development and Phase 3 clinical trials. Several months later, HHS committed up to $1.5 billion for Moderna’s large-scale manufacturing and delivery efforts. Ultimately the U.S. government owned the resulting 100 million doses of vaccines and reserved the option to acquire more. Similar agreements were created with other manufacturers, leading to three vaccine candidates receiving FDA emergency use authorization.
Example Challenge: Space Debris |
Low-earth orbit includes dead satellites and other debris that pose risks for existing and future space infrastructure. Increased interest in commercialization of low-earth orbit will exacerbate a debris count that is already considered unstable. Since national space policy generally requires some degree of engagement with commercial providers, the U.S. would need to include the industry in this effort. The cost of active space debris removal, satellite decommissioning and recycling, and other cleanup activities are largely unknown, which dissuades novel business ventures. Nevertheless, large debris objects that pose the greatest collision risks need to be prioritized for decommission. Demand-pull mechanisms could be used to create a market for sustained space debris mitigation, such as an advanced market commitment for the removal of large debris items. Commitments for removal could be paired with a study across the DOD and NASA to identify large, high-priority items for removal. Another mechanism that could be considered is fixed milestone payments, which NASA has used in past partnerships with commercial partners, most notably SpaceX, to develop commercial orbital transportation systems. |
Mechanism 5: Reduce risk with diversity and redundancy
Engage multiple private partners on the same goal to enable competition and minimize the risk of overall program failure. Since resources are not infinite, the program should incorporate evidence-based decision-making with strict criteria and a rubric. A rubric and clear criteria also ensure fair competition and avoid creating a single national champion.
During OWS, four vaccine platform technologies were considered for development: mRNA, replication-defective live-vector, recombinant-subunit-adjuvanted protein, and attenuated replicating live-vector. The first two had never been used in FDA-licensed vaccines but showed promise, while the second two were established in FDA-licensed vaccines. Following a risk assessment, six vaccine candidates using three of the four platforms were advanced. Redundancy was incorporated in two dimensions: three different vaccine platforms and two separate candidates. The manufacturing strategy also included redundancy, as several companies were awarded contracts to produce needles and syringes. Diversifying sources for common vaccination supplies reduced the overall risk of failure at each node in the supply chain.
Example Challenge: Alternative Battery Technology |
Building infrastructure to capture energy from renewable sources requires long-term energy storage to manage the variability of renewable energy generation. Lithium-ion batteries, commonly used in consumer electronics and electric vehicles, are a potential candidate, since research and development has driven significant cost declines since the technology’s introduction in the 1990s. However, performance declines when storing energy over long periods, and the extraction of critical minerals is still relatively expensive and harmful to the environment. The limitations of lithium-ion batteries could be addressed by investing in several promising alternative battery technologies that use cheaper materials such as sodium, sulfur, and iron. This portfolio approach will enable competition and increase the chance that at least one option is successful. |
Conclusion
Operation Warp Speed was a historic accomplishment on the level of the Manhattan Project and the Apollo program, but the unique approach is not appropriate for every challenge. The methods and mechanisms are best suited for challenges in which stakeholders agree on an urgent and specific goal, and the goal requires scaling a technology with established fundamental research. Nonetheless, the individual mechanisms of OWS can effectively address smaller challenges. Those looking to replicate the success of OWS should deeply evaluate the stakeholder and technology landscape to determine which mechanisms are required or feasible.
Acknowledgments
This memo was developed from notes on presentations, panel discussions, and breakout conversations at the Operation Warp Speed 2.0 Conference, hosted on November 17, 2022, by the Federation of American Scientists, 1Day Sooner, and the Institute for Progress to recount the success of OWS and consider future applications of the mechanisms. The attendees included leadership from the original OWS team, agency leaders, Congressional staffers, researchers, and vaccine industry leaders. Thank you to Michael A. Fisher, FAS senior fellow, who contributed significantly to the development of this memo through January 2023. Thank you to the following FAS staff for additional contributions: Dan Correa, chief executive officer; Jamie Graybeal, director, Defense Budgeting Project (through September 2022); Sruthi Katakam, Scoville Peace Fellow; Vijay Iyer, program associate, science policy; Kai Etheridge, intern (through August 2022).
The OWS approach is unlikely to succeed for challenges that are too broad or too politically polarizing. For example, curing cancer: While a cure is incredibly urgent and the goal is unifying, too many variations of cancer exist and they include several unique research and development challenges. Climate change is another example: particular climate challenges may be too politically polarizing to motivate the commitment required.
No topic is immune to politicization, but some issues have existing political biases that will hinder application of the mechanisms. Challenges with bipartisan agreement and public support should be prioritized, but politicization can be managed with a comprehensive understanding of the stakeholder landscape.
The pandemic created an emergency environment that likely motivated behavior change at agencies, but OWS demonstrated that better agency coordination is possible.
In addition to using processes like stakeholder mapping, the leadership team must include experts across the problem space that are deeply familiar with key stakeholder groups and existing power dynamics. The problem space includes impacted portions of the public; federal agencies and offices; the administration; state, local, Tribal, and territorial governments; and private partners.
OWS socialized the vaccination effort through HHS’s Office of Intergovernmental and External Affairs, which established communication with hospitals, healthcare providers, nursing homes, community health centers, health insurance companies, and more. HHS also worked with state, local, Tribal, and territorial partners, as well as organizations representing minority populations, to address health disparities and ensure equity in vaccination efforts. Despite this, OWS leaders expressed that better communication with expert communities was needed, as the public was confused by contradictory statements from experts who were unaware of the program details.
Future efforts should create channels for bottom-up communication from state, local, Tribal, and territorial governments to federal partners. Encouraging feedback through community engagement can help inform distribution strategies and ensure adoption of the solution. Formalized data-sharing protocols may also help gain buy-in and confidence from relevant expert communities.
Possibly, but it would require more coordination and alignment between the countries involved. This could include applying the mechanisms within existing international institutions to achieve existing goals. The mechanisms could apply with revisions, such as coordination among national delegations and nongovernmental organizations, activating nongovernmental capacity, and creating geopolitical incentives for adoption.
The team included HHS Secretary Alex Azar; Secretary of Defense Mark Esper; Dr. Moncef Slaoui, former head of vaccines at GlaxoSmithKline; and General Gustave F. Perna, former commanding general of U.S. Army Materiel Command. This core team combined scientific and technical expertise with military and logistical backgrounds. Dr. Slaoui’s familiarity with the pharmaceutical industry and the vaccine development process allowed OWS to develop realistic goals and benchmarks for its work. This connection was also critical in forging robust public-private partnerships with the vaccine companies.
It depends on the challenge. Determining which mechanism to use for a particular project requires a deep understanding of the particular R&D, manufacturing, supply chain landscapes to diagnose the market gaps. For example, if manufacturing process technologies are needed, prize competitions or challenge-based acquisitions may be most effective. If manufacturing volume must increase, volume guarantees or advance purchase agreements may be more appropriate. Advance market commitments or milestone payments can motivate industry to increase efficiency. OWS used a combination of volume guarantees and advance market commitments to fund the development of vaccine candidates and secure supply.
Enabling Faster Funding Timelines in the National Institutes of Health
Summary
The National Institutes of Health (NIH) funds some of the world’s most innovative biomedical research, but rising administrative burden and extended wait times—even in crisis—have shown that its funding system is in desperate need of modernization. Examples of promising alternative models exist: in the last two years, private “fast science funding” initiatives such as Fast Grants and Impetus Grants have delivered breakthroughs in responding to the coronavirus pandemic and aging research on days to one-month timelines, significantly faster than the yearly NIH funding cycles. In response to the COVID-19 pandemic the NIH implemented a temporary fast funding program called RADx, indicating a willingness to adopt such practices during acute crises. Research on other critical health challenges like aging, the opioid epidemic, and pandemic preparedness deserves similar urgency. We therefore believe it is critical that the NIH formalize and expand its institutional capacity for rapid funding of high-potential research.
Using the learnings of these fast funding programs, this memo proposes actions that the NIH could take to accelerate research outcomes and reduce administrative burden. Specifically, the NIH director should consider pursuing one of the following approaches to integrate faster funding mechanisms into its extramural research programs:
- Reform the existing R21 grant mechanism to bring it more in line with its own goals of funding high-reward, rapid-turnaround research; and
- Direct NIH institutes and centers to independently develop and deploy new research programs with faster funding timelines.
Future efforts by the NIH and other federal policymakers to respond to crises like the COVID-19 pandemic would also benefit from a clearer understanding of the impact of the decision-making process and actions taken by the NIH during the earliest weeks of the pandemic. To that end, we also recommend that Congress initiate a report from the Government Accountability Office to illuminate the outcomes and learnings of fast governmental programs during COVID-19, such as RADx.
Challenge and Opportunity
The urgency of the COVID-19 pandemic created adaptations not only in how we structure our daily lives but in how we develop therapeutics and fund science. Starting in 2020, the public saw a rapid emergence of nongovernmental programs like Fast Grants, Impetus Grants, and Reproductive Grants to fund both big clinical trials and proof-of-concept scientific studies within timelines that were previously thought to be impossible. Within the government, the NIH launched RADx, a program for the rapid development of coronavirus diagnostics with significantly accelerated approval timelines. Though the sudden onset of the pandemic was unique, we believe that an array of other biomedical crises deserve the same sense of urgency and innovation. It is therefore vital that the new NIH director permanently integrate fast funding programs like RADx into the NIH in order to better respond to these crises and accelerate research progress for the future.
To demonstrate why, we must remember that the coronavirus is far from being an outlier—in the last 20 years, humanity has gone through several major pandemics, notably swine flu, SARS CoV-1, and Ebola. Based on the long-observed history of infectious diseases, the risk of pandemics with an impact similar to that of COVID-19 is about two percent in any year. An extension of naturally occurring pandemics is the ongoing epidemic of opioid use and addiction. The rapidly changing landscape of opioid use—with overdose rates growing rapidly and synthetic opioid formulations becoming more common—makes slow, incremental grantmaking ill-suited for the task. The counterfactual impact of providing some awards via faster funding mechanisms in these cases is self-evident: having tests, trials, and interventions earlier saves lives and saves money, without sacrificing additional resources.
Beyond acute crises, there are strong longer-term public health motivations for achieving faster funding of science. In about 10 years, the United States will have more seniors (people aged 65+) than children. This will place substantial stress on the U.S. healthcare system, especially given that two-thirds of seniors suffer from more than one chronic disease. New disease treatments may help, but it often takes years to translate the results of basic research into approved drugs. The idiosyncrasies of drug discovery and clinical trials make them difficult to accelerate at scale, but we can reliably accelerate drug timelines on the front end by reducing the time researchers spend in writing and reviewing grants—potentially easing the long-term stress on U.S. healthcare.
The existing science funding system developed over time with the best intentions, but for a variety of reasons—partly because the supply of federal dollars has not kept up with demand—administrative requirements have become a major challenge for many researchers. According to surveys, working scientists now spend 44% of their research time on administrative activities and compliance, with roughly half of that time spent on pre-award activities. Over 60% of scientists say administrative burden compromises research productivity, and many fear it discourages students from pursuing science careers. In addition, the wait for funding can be extensive: one of the major NIH grants, R01, takes more than three months to write and around 8–20 months to receive (see FAQ). Even proof-of-concept ideas face onerous review processes and take at least a year to fund. This can bottleneck potentially transformative ideas, as with Katalin Kariko famously struggling to get funding for her breakthrough mRNA vaccine work when it was at its early stages. These issues have been of interest for science policymakers for more than two decades, but with little to show for it.
Though several nongovernmental organizations have attempted to address this need, the model of private citizens continuously fundraising to enable fast science is neither sustainable nor substantial enough compared to the impact of the NIH. We believe that a coordinated governmental effort is needed to revitalize American research productivity and ensure a prompt response to national—and international—health challenges like naturally occurring pandemics and imminent demographic pressure from age-related diseases. The new NIH director has an opportunity to take bold action by making faster funding programs a priority under their leadership and a keystone of their legacy.
The government’s own track record with such programs gives grounds for optimism. In addition to the aforementioned RADx program at NIH, the National Science Foundation (NSF) runs the Early-Concept Grants for Exploratory Research (EAGER) and Rapid Response Research (RAPID) programs, which can have response times in a matter of weeks. Going back further in history, during World War II, the National Defense Research Committee maintained a one-week review process.
Faster grant review processes can be either integrated into existing grant programs or rolled out by institutes in temporary grant initiatives responding to pressing needs, as the RADx program was. For example, when faced with data falsification around the beta amyloid hypothesis, the National Institute of Aging (NIA) could leverage fast grant review infrastructure to quickly fund replication studies for key papers, without waiting for the next funding cycle. In case of threats to human health due to toxins, the National Institute of Environmental Health Sciences (NIEHS) could rapidly fund studies on risk assessment and prevention, giving public evidence-based recommendations with no delay. Finally, empowering the National Institute of Allergy and Infectious Diseases (NIAID) to quickly fund science would prepare us for many yet-to-come pandemics.
Plan of Action
The NIH is a decentralized organization, with institutes and centers (ICs) that each have their own mission and focus areas. While the NIH Office of the Director sets general policies and guidelines for research grants, individual ICs have the authority to create their own grant programs and define their goals and scope. The Center for Scientific Review (CSR) is responsible for the peer review process used to review grants across the NIH and recently published new guidelines to simplify the review criteria. Given this organizational structure, we propose that the NIH Office of the Director, particularly the Office of Extramural Research, assess opportunities for both NIH-wide and institute-specific fast funding mechanisms and direct the CSR, institutes, and centers to produce proposed plans for fast funding mechanisms within one year. The Director’s Office should consider the following approaches.
Approach 1. Develop an expedited peer review process for the existing R21 grant mechanism to bring it more in line with the NIH’s own goals of funding high-reward, rapid-turnaround research.
The R21 program is designed to support high-risk, high-reward, rapid-turnaround, proof-of-concept research. However, it has been historically less popular among applicants compared to the NIH’s traditional research mechanism, the R01. This is in part due to the fact that its application and review process is known to be only slightly less burdensome than the R01, despite providing less than half of the financial and temporal support. Therefore, reforming the application and peer review process for the R21 program to make it a fast grant–style award would both bring it more in line with its own goals and potentially make it more attractive to applicants.
All ICs follow identical yearly cycles for major grant programs like the R21, and the CSR centrally manages the peer review process for these grant applications. Thus, changes to the R21 grant review process must be spearheaded by the NIH director and coordinated in a centralized manner with all parties involved in the review process: the CSR, program directors and managers at the ICs, and the advisory councils at the ICs.
The track record of federal and private fast funding initiatives demonstrates that faster funding timelines can be feasible and successful (see FAQ). Among the key learnings and observations of public efforts that the NIH could implement are:
- Pilot monthly or bimonthly study section and advisory council meetings for R21 grant review. CSR has switched to conducting the majority of its meetings virtually since the COVID-19 pandemic and has found that in-person and virtual meetings are of equal quality. CSR should take advantage of the convenience of virtual meetings by piloting shorter, virtual monthly or bimonthly study section meetings to review R21 grants outside of the three regular meetings held each year. By meeting more frequently but for shorter amounts of time, the individual time commitment for each meeting is reduced, which may incentivize more researchers to participate in study sections and prevent reviewer fatigue from the traditional one- to two-day meetings. To match this change, the advisory councils of ICs that review R21 grant applications should also pilot monthly virtual meetings, timed to occur immediately after the corresponding peer review meetings. Together, these changes could reduce the R21 grant review timeline from a minimum of nine months down to just two or three months.
- Explore new approaches for reviewer participation. One obstacle to faster funding timelines is the recruitment of reviewers without a conflict of interest. Previously, the travel and financial burden of in-person study sections kept the standing body of reviewers small; this makes it difficult to find and gather a quorum of knowledgeable and unconflicted experts. With online study sections, the CSR could engage a larger committee of reviewers at lower cost. This would allow them to identify and address conflicts of interest dynamically and to select a small and varying subset of reviewers to meet each month. Scientists may also be more inclined to participate as potential reviewers, knowing that they may not be called upon for every round of reviews.
- Emphasize the potential value of success over risk. Reviewers should be explicitly instructed not to lower their scores for the Approach criterion (or the new Rigor and Feasibility criterion proposed by CSR) solely due to a lack of extensive prior literature or over differences in the applicant’s past area of expertise. (Reviewer suggestions could still be used to help inform the direction of the proposed work in these cases.) Instead, the Significance and Innovation criteria (or the new Importance of Research criterion) should be weighed much more heavily than other criteria in the overall score. The rationale for these changes is evident: novel areas will naturally have less extensive prior literature, while learnings from one area of research can cross-pollinate innovation in an entirely different area of research. Acceptance of high-risk, high-reward proposals could be further facilitated by piloting the “golden ticket” model, in which reviewers are provided the right to unilaterally fund one application per year that they believe holds the most innovation potential.
- Reduce the length of applications. The length of proposals for both Fast Grants and Impetus Grants did not exceed two pages, which, according to reviewers, was more than enough to make well-reasoned judgment calls. The NIH should reduce the page limit from six to three pages for the R21 grant program. This will reduce the administrative burden and save time for both applicants and peer reviewers.
Pending the success of these changes, the NIH should consider applying similar changes to other major research grant programs.
Approach 2. Direct NIH institutes and centers to independently develop and deploy programs with faster funding timelines using Other Transaction Authority (OTA).
Compared to reforming an existing mechanism, the creation of institute-specific fast funding programs would allow for context-specific implementation and cross-institute comparison. This could be accomplished using OTA—the same authority used by the NIH to implement COVID-19 response programs. Since 2020, all ICs at the NIH have had this authority and may implement programs using OTA with approval from the director of NIH, though many have yet to make use of it.
As discussed previously, the NIA, NIDA, and NIAID would be prime candidates for the roll-out of faster funding. In particular, these new programs could focus on responding to time-sensitive research needs within each institute or center’s area of focus—such as health crises or replication of linchpin findings—that would provide large public benefits. To maintain this focus, these programs could restrict investigator-initiated applications and only issue funding opportunity announcements for areas of pressing need.
To enable faster peer review of applications, ICs should establish (a) new study section(s) within their Scientific Review Branch dedicated to rapid review, similar to how the RADx program had its own dedicated review committees. Reviewers who join these study sections would commit to short meetings on a monthly or bimonthly basis rather than meeting three times a year for one to two days as traditional study sections do. Additionally, as recommended above, these new programs should have a three-page limit on applications to reduce the administrative burden on both applicants and reviewers.
In this framework, we propose that the ICs be encouraged to direct at least one percent of their budget to establish new research programs with faster funding processes. We believe that even one percent of the annual budget is sufficient to launch initial fast grant programs funded through National Institutes. For example, the National Institute of Aging had an operating budget of $4 billion in the 2022 fiscal year. One percent of this budget would constitute $40 million for faster funding initiatives, which would be on the order of initial budgets of Impetus and Fast Grants ($25 million and $50 million accordingly).
NIH ICs should develop success criteria in advance of launching new fast funding programs. If the success criteria are met, they should gradually increase the budget and expand the scope of the program by allowing for investigator-initiated applications, making it a real alternative to R01 grants. A precedent for this type of grant program growth is the Maximizing Investigators’ Research Award (MIRA) (R35) grant program within the National Institute of General Medical Sciences (NIGMS), which set the goal of funding 60% of all R01 equivalent grants through MIRA by 2025. In the spirit of fast grants, we recommend setting a deadline on how long each institute can take to establish a fast grants program to ensure that the process does not extend for too many years.
Additional recommendation. Congress should initiate a Government Accountability Office report to illuminate the outcomes and learnings of governmental fast funding programs during COVID-19, such as RADx.
While a number of published papers cite RADx funding, the program’s overall impact and efficiency haven’t yet been assessed. We believe that the agency’s response during the pandemic isn’t yet well-understood but likely played an important role. Illuminating the learnings of these interventions would greatly benefit future emergency fast funding programs.
Conclusion
The NIH should become a reliable agent for quickly mobilizing funding to address emergencies and accelerating solutions for longer-term pressing issues. As present, no funding mechanisms within NIH or its branch institutes enable them to react to such matters rapidly. However, both public and governmental initiatives show that fast funding programs are not only possible but can also be extremely successful. Given this, we propose the creation of permanent fast grants programs within the NIH and its institutes based on learnings from past initiatives.
The changes proposed here are part of a larger effort from the scientific community to modernize and accelerate research funding across the U.S. government. In the current climate of rapidly advancing technology and increasing global challenges, it is more important than ever for U.S. agencies to stay at the forefront of science and innovation. A fast funding mechanism would enable the NIH to be more agile and responsive to the needs of the scientific community and would greatly benefit the public through the advancement of human health and safety.
The NIH released a number of Notices of Special Interest to allow emergency revision to existing grants (e.g., PA-20-135 and PA-18-591) and a quicker path for commercialization of life-saving COVID technologies (NOT-EB-20-008). Unfortunately, repurposing existing grants reportedly took several months, significantly delaying impactful research.
The current scientific review process in NIH involves multiple stakeholders. There are two stages of review at NIH, with the first stage being conducted by a Scientific Review Group that consists primarily of nonfederal scientists. Typically, Center for Scientific Review committees meet three times a year for one or two days. This way, the initial review starts only four months after the proposal submission. Special Emphasis Panel meetings that are not recurring take even longer due to panel recruitment and scheduling. The Institute and Center National Advisory Councils or Boards are responsible for the second stage of review, which usually happens after revision and appeals, taking the total timeline to approximately a year.
Because of the difficulty of empirically studying drivers of scientific impact, there has been little research evaluating peer review’s effects on scientific quality. A Cochrane systematic review from 2007 found no studies directly assessing review’s effects on scientific quality, and a recent Rand review of the literature in 2018 found a similar lack of empirical evidence. A few more recent studies have found modest associations between NIH peer review scores and research impact, suggesting that peer review may indeed successfully identify innovative projects. However, such a relationship still falls short of demonstrating that the current model of grant review reliably leads to better funding outcomes than alternative models. Additionally, some studies have demonstrated that the current model leads to variable and conservative assessments. Taken together, we think that experimentation with models of peer review that are less burdensome for applicants and reviewers is warranted.
Intuitively, it seems that having longer grant applications and longer review processes ensures that both researchers and reviewers expend great effort to address pitfalls and failure modes before research starts. However, systematic reviews of the literature have found that reducing the length and complexity of applications has minimal effects on funding decisions, suggesting that the quality of resulting science is unlikely to be affected.
Historical examples have also suggested that the quality of an endeavor is largely uncorrelated from its planning times. It took Moderna 45 days from COVID-19 genome publication to submit the mRNA-1273 vaccine to the NIH for use in its Phase 1 clinical study. Such examples exist within government too: during World War II, National Defense Research Committee set a record by reviewing and authorizing grants within one week, which led to DUKW, Project Pigeon, Proximity fuze, and Radar.
Recent fast grant initiatives have produced high-quality outcomes. With its short applications and next-day response times, Fast Grants enabled:
- detection of new concerning COVID-19 variants before other sources of funding became available.
- work that showed saliva-based COVID-19 tests can work just as well as those using nasopharyngeal swabs.
- drug-repurposing clinical trials, one of which identified a generic drug reducing hospitalization from COVID-19 by ~40%.
- Research into “Long COVID,” which is now being followed up with a clinical trial on the ability of COVID-19 vaccines to improve symptoms.
Impetus Grants focused on projects with longer timelines but led to a number of important preprints in less than a year from the moment person applied:
- Aging Fly Cell Atlas
- Modular, programmable RNA sensing using ADAR editing in living cells
- Mechanisms of natural rejuvenation in a test tube
- Optogenetic rejuvenation of mitochondrial membrane potential to extend C. elegans lifespan
- Evidence that conserved essential genes are enriched for pro-longevity factors
- Trials on neuroprotective effects of Canagliflozin
With the heavy toll that resource-intensive approaches to peer review take on the speed and innovative potential of science—and the early signs that fast grants lead to important and high-quality work—we feel that the evidentiary burden should be placed on current onerous methods rather than the proposed streamlined approaches. Without strong reason to believe that the status quo produces vastly improved science, we feel there is no reason to add years of grant writing and wait times to the process.
The adoption of faster funding mechanisms would indeed be valuable across a range of federal funding agencies. Here, we focus on the NIH because its budget for extramural research (over $30 billion per year) represents the single largest source of science funding in the United States. Additionally, the NIH’s umbrella of health and medical science includes many domains that would be well-served by faster research timelines for proof-of-concept studies—including pandemics, aging, opioid addiction, mental health, cancer, etc.
Towards a Solution for Broadening the Geography of NSF Funding
Congressional negotiations over the massive bipartisan innovation bill have stumbled over a controversial proposal to expand the geographic footprint of National Science Foundation (NSF) funding. That proposal, in the Senate-passed U.S. Innovation and Competition Act (USICA), mandates that 20% of NSF’s budget be directed to a special program to help institutions in the many states that receive relatively few NSF dollars.
Such a mandate would represent a dramatic expansion of the Established Program to Stimulate Competitive Research (EPSCoR), which currently receives less than 3% of NSF’s budget. Major EPSCoR expansion is popular among legislators who would like to see the research institutions they represent become more competitive within the NSF portfolio. Some legislators have said their support of the overall innovation package is contingent on such expansion.
But the proposed 20% set-aside for EPSCoR is being met with fierce opposition on Capitol Hill. 96 other legislators recently co-authored a letter warning, “Arbitrarily walling off a sizable percentage of a science agency’s budget from a sizable majority of the country’s research institutions would fundamentally reduce the entire nation’s scientific capacity and damage the research profiles of existing institutions.”
Both proponents and opponents of the 20% set-aside make good points. Those in favor want to see more equitable distribution of federal research dollars, while those against are concerned that the mandatory set-aside is too massive and blunt an instrument for achieving that goal. Fortunately, we believe compromise is achievable—and well worth pursuing. Here’s how.
What is EPSCoR?
First, some quick background on the program at the heart of the controversy: ESPCoR. The program was established in 1979 with the admirable goal of broadening the geographic distribution of NSF research dollars, which even then were disproportionately concentrated in a handful of states.
EPSCoR provides eligible jurisdictions with targeted support for research infrastructure, development activities like workshops, and co-funding for project proposals submitted to other parts of NSF. A jurisdiction is eligible to participate in EPSCoR if its most recent five-year level of total NSF funding is equal to or less than 0.75% of the total NSF budget (excluding EPSCoR funding and NSF funding to other federal agencies). Currently, 25 states plus Puerto Rico, Guam, and the U.S. Virgin Islands qualify for EPSCoR. Yet the non-EPSCoR states still accounted for nearly 90% of NSF awards in FY 2021.
Why is expansion controversial?
As mentioned above, the Senate-passed USICA (S. 1260) would require NSF to devote 20% of its budget to EPSCoR (including research consortia led by EPSCoR institutions). The problem is that EPSCoR received only 2.4% of NSF’s FY 2022 appropriation. This means that to achieve the 20% mandate without cutting non-EPSCoR funding, Congress would have to approve nearly $2 billion in new appropriations for NSF in FY 2023, representing a 22% year-over-year increase, devoted entirely to EPSCoR. This is, to be blunt, wildly unlikely.
On the other hand, achieving a 20% budget share for EPSCoR under a more realistic FY 2023 appropriation for NSF would require cutting funding for non-EPSCoR programs on the order of 15%: a cataclysmic proposition for the research community.
Neither pathway for a 20% EPSCoR set-aside seems plausible. Still, key legislators have said that the 20% target is a must-have. So what can be done?
A path forward
We think a workable compromise is possible. The following three revisions to the Senate-proposed set-aside that everyone might accept:
- Specify that the 20% mandate applies to institutions in EPSCoR states rather than the EPSCoR program itself. While specific funding for the EPSCoR program accounts for less than 3% of the total NSF budget, institutions in current EPSCoR states actually receive about 13% of NSF research dollars. In other words, a substantial portion of NSF funding is allocated to EPSCoR institutions through the agency’s normal competitive-award opportunities. Given this fact, there’s a clear case to be made for focusing the 20% ramp-up on EPSCoR-eligible institutions rather than the EPSCoR program.
- Specify that the mandate only applies to extramural funding, not to agency operations and administrative appropriations. This is simply good government. If EPSCoR funding is tied to administrative appropriations, it may create an incentive to bloat the administrative line items. Further, if the mandate is applied to the entirety of the NSF budget and administrative costs must increase for other reasons (for instance, to cover future capital investments at NSF headquarters), then NSF may be forced to “balance the books” by cutting non-EPSCoR extramural funding to maintain the 20% ESPCoR share.
- Establish a multi-year trajectory to achieve the 20% target. As mentioned above, a major year-over-year increase in the proportion of NSF funding directed to either EPSCoR or EPSCoR-eligible institutions could cripple other essential NSF programs from which funding would have to be pulled. Managing the deluge of new dollars could also prove a challenge for EPSCoR-eligible institutions. Phasing in the 20% target over, say, five years would (i) enable federal appropriators to navigate pathways for increasing EPSCoR funding while avoiding drastic cuts elsewhere at NSF, and (ii) give EPSCoR-eligible institutions time to build out the capacities needed to maximize return on new research investments.
Crunching the numbers
To illustrate what this proposed compromise could mean fiscally, let’s say Congress mandates that NSF funding for EPSCoR-eligible institutions rises from its current ~13% share of total research dollars to 20% in five years. To achieve this target, the share of NSF funding received by EPSCoR-eligible states would have to rise by approximately 9% per year for five years.
Under this scenario, if NSF achieves 3% annual increases in appropriations (which is close to what it’s done since the FY 2013 “sequestration” year), then we’d see about 13% annual growth in NSF research dollars funneled to EPSCoR states due to the escalating set-aside. NSF research dollars funneled to non-EPSCoR states would increase by about 1% annually over the same time period. By the end of the five-year period, EPSCoR-eligible institutions would have seen a more than 80% increase in funding.
Annual increases in NSF appropriations of 2% would be enough to achieve the 20% set-aside without cutting funding for institutions in non-EPSCoR states, but wouldn’t allow any growth in funding for those institutions either. In other words, the appropriations increases would have to be entirely directed to the rising EPSCoR set-aside.
Finally, annual increases in NSF appropriations of 5% would be enough to achieve the 20% set-aside for EPSCoR-eligible institutions while also enabling non-EPSCoR-eligible institutions to enjoy continued 3% annual increases in funding growth.
The next step
U.S. strength in innovation is predicated on the scientific contributions from all corners of the nation. There is hence a clear and compelling reason to ensure that all U.S. research institutions have the resources they need to succeed, including those that have historically received a lower share of support from federal agencies.
he bipartisan innovation package offers a chance to achieve this, but it must be done carefully. The three-pronged compromise on EPSCoR outlined above is a prudent way to thread the needle. It should also be supported by sustained, robust increases in NSF funding as a whole. Congress should therefore couple this compromise with an explicit, bipartisan commitment to support long-term appropriations growth for NSF—because such growth would benefit institutions in every state.
The bipartisan innovation package offers enormous potential upside along several dimensions for U.S. science, innovation, and competitiveness. To enable that upside, an EPSCoR compromise is worth pursuing.
Piloting and Evaluating NSF Science Lottery Grants: A Roadmap to Improving Research Funding Efficiencies and Proposal Diversity
This memo was jointly produced by the Federation of American Scientists & the Institute for Progress
Summary
The United States no longer leads the world in basic science. There is growing recognition of a gap in translational activities — the fruits of American research do not convert to economic benefits. As policymakers consider a slew of proposals that aim to restore American competitiveness with once-in-a-generation investments into the National Science Foundation (NSF), less discussion has been devoted to improving our research productivity — which has been declining for generations. Cross-agency data indicates that this is not the result of a decline in proposal merit, nor of a shift in proposer demographics, nor of an increase (beyond inflation) in the average requested funding per proposal, nor of an increase in the number of proposals per investigator in any one year. As the Senate’s U.S. Innovation and Competition Act (USICA) and House’s America COMPETES Act propose billions of dollars to the NSF for R&D activities, there is an opportunity to bolster research productivity but it will require exploring new, more efficient ways of funding research.
The NSF’s rigorous merit review process has long been regarded as the gold standard for vetting and funding research. However, since its inception in the 1950s, emergent circumstances — such as the significant growth in overall population of principal investigators (PIs) — have introduced a slew of challenges and inefficiencies to the traditional peer-review grantmaking process: The tax on research productivity as PIs submit about 2.3 proposals for every award they receive and spend an average of 116 hours grant-writing per NSF proposal (i.e., “grantsmanship”), corresponding to a staggering loss of nearly 45% of researcher time; the orientation of grantsmanship towards incremental research with the highest likelihood of surviving highly-competitive, consensus-driven, and points-based review (versus riskier, novel, or investigator-driven research); rating bias against interdisciplinary research or previously unfunded researchers as well as reviewer fatigue. The result of such inefficiencies is unsettling: as fewer applicants are funded as a percentage of the increasing pool, some economic analysis suggests that the value of the science that researchers forgo for grantsmanship may exceed the value of the science that the funding program supports.
Our nation’s methods of supporting new ideas should evolve alongside our knowledge base.
Our nation’s methods of supporting new ideas should evolve alongside our knowledge base. Science lotteries — when deployed as a complement to the traditional peer review grant process — could improve the systems’ overall efficiency-cost ratio by randomly selecting a small percentage of already-performed, high quality, yet unfunded grant proposals to extract value from. Tested with majority positive feedback from participants in New Zealand, Germany, and Switzerland, science lotteries would introduce an element of randomness that could unlock innovative, disruptive scholarship across underrepresented demographics and geographies.
This paper proposes an experimental NSF pilot of science lotteries and the Appendix provides illustrative draft legislation text. In particular, House and Senate Science Committees should consider the addition of tight language in the U.S. Innovation and Competition Act (Senate) and the America COMPETES Act (House) that authorizes the use of “grant lotteries” across all NSF directorates, including the Directorate of Technology and Innovation. This language should carry the spirit of expanding the geography of innovation and evidence-based reviews that test what works.
Challenge and Opportunity
A recent NSF report pegged the United States as behind China in key scientific metrics, including the overall number of papers published and patents awarded. The numbers are sobering but reflect the growing understanding that America must pick which frontiers of knowledge it seeks to lead. One of these fields should be the science of science — in other words not just what science & technology innovations we hope to pursue, but in discovering new, more efficient ways to pursue them.
Since its inception in 1950, NSF has played a critical role in advancing the United States’ academic research enterprise, and strengthened our leadership in scientific research across the world. In particular, the NSF’s rigorous merit review process has been described as the gold standard for vetting and funding research. However, growing evidence indicates that, while praiseworthy, the peer review process has been stretched to its limits. In particular, the growing overall population of researchers has introduced a series of burdens on the system.
One NSF report rated nearly 70% of proposals as equally meritorious, while only one-third received funding. With a surplus of competitive proposals, reviewing committees often face tough close calls. In fact, empirical evidence has found that award decisions change nearly a quarter of the time when re-reviewed by a new set of peer experts. In response, PIs spend upwards of 116 hours on each NSF proposal to conform to grant expectations and must submit an average of 2.3 proposals to receive an award — a process known as “grantsmanship” that survey data suggests occupies nearly 45% of top researchers’ time. Even worse, this grantsmanship is oriented towards writing proposals on incremental research topics (versus riskier, novel, or investigator-driven research) which has a higher likelihood of surviving a consensus-driven, points-based review. On the reviewer side, data supports a clear rating bias against interdisciplinary research or previously unfunded researchers PIs, while experts increasingly are declining invitations to review proposals in the interests of protecting their winnowing time (e.g., reviewer fatigue).
These tradeoffs in the current system appear quite troubling and merit further investigation of alternative and complementary funding models. At least one economic analysis suggests that as fewer applicants are funded as a percentage of the increasing pool, the value of the science that researchers forgo because of grantsmanship often exceeds the value of the science that the funding program supports. In fact, despite dramatic increases in research effort, America has for generations been facing dramatic declines in research productivity. And empirical analysis suggests this is notnecessarily the result of a decline in proposal merit, nor of a shift in proposer demographics, nor of an increase (beyond inflation) in the average requested funding per proposal, nor of an increase in the number of proposals per investigator in any one year.
As the Senate’s U.S. Innovation and Competition Act (USICA) and House’s America COMPETES Act propose billions of dollars to the NSF for R&D activities, about 96% of which will be distributed via the peer review, meritocratic grant awards process, now is the time to apply the scientific method to ourselves in the experimentation of alternative and complementary mechanisms for funding scientific research.
Science lotteries, an effort tested in New Zealand, Switzerland, and Germany, represent one innovation particularly suited to reduce the overall taxes on research productivity while uncovering new, worthwhile initiatives for funding that might otherwise slip through the cracks. In particular, modified science lotteries, as those proposed here, select a small percentage of well-qualified grant applications at random for funding. By only selecting from a pool of high-value projects, the lottery supports additional, quality research with minimal comparative costs to the researchers or reviewers. In a lottery, the value to the investigator of being admitted to the lottery scales directly with the number of awards available.
These benefits translate to favorable survey data from PIs who have gone through science lottery processes. In New Zealand, for example, the majority of scientists supported a random allocation of 2% total research expenditures. Sunny Collings, chief executive of New Zealand’s Health Research Council, recounted:
“Applications often have statistically indistinguishable scores, and there is a degree of randomness in peer review selection anyway. So why not formalize that and try to get the best of both approaches?”
By establishing conditions for entrance into the lottery — such as selecting for certain less funded or represented regions — NSF could also over-index for those applicants less prepared for “grantsmanship”.
What we propose, specifically, is a modified “second chance” lottery, whereby proposals that are deemed meritorious by the traditional peer-review process, yet are not selected for funding are entered into a lottery as a second stage in the funding process. This modified format ensures a high level of quality in the projects selected by the lottery to receive funding while still creating a randomized baseline to which the current system can be compared.
The use of science lotteries in the United States as a complement to the traditional peer-review process is likely to improve the overall system. However, it is possible that selecting among well-qualified grants at random could introduce unexpected outcomes. Unfortunately, direct, empirical comparisons between the NSF’s peer review process and partial lotteries do not exist. Through a pilot, the NSF has the opportunity to evaluate to what extent the mechanism could supplement the NSF’s traditional merit review process.
By formalizing a randomized selection process to use as a baseline for comparison, we may discover surprising things about the make up of and process that leads to successful or high-leverage research with reduced costs to researchers and reviewers. For instance, it may be the case that younger scholars who come from non-traditional backgrounds end up having as much or more success in terms of research outcomes through the lottery program as the typical NSF grant, but are selected at higher rates when compared to the traditional NSF grantmaking process. If this is the case, then there will be some evidence that something in the selection process is unfairly penalizing non-traditional candidates.
Alternatively, we may discover that the average grant selected through the lottery is mostly indistinguishable from the average grant selected through the traditional meritorious selection, which would provide some evidence that existing administrative burdens to select candidates are too stringent. Or perhaps, we will discover that randomly selected winners, in fact, produce fewernoteworthy results than candidates selected through traditional means, which would be evidence that the existing process is providing tangible value in filtering funding proposals.By providing a baseline for comparison, a lottery would offer an evidence-based means of assessing the efficacy of the current peer-review system. Any pilot program should therefore make full use of a menu of selection criteria to toggle outcomes, while also undergoing evaluations from internal and external, scientific communities.
Plan of Action
Recommendation 1: Congress should direct the NSF to pilot experimental lotteries through America COMPETES and the U.S. Innovation and Competition Act, among other vehicles.
In reconciling the differing House America COMPETES and Senate USICA, Congress should add language that authorizes a pilot program for “lotteries.”
We recommend opting for signaling language and follow-on legislation that adds textual specificity. For example, in latest text of the COMPETES Act, the responsibilities of the Assistant Director of the Directorate for Science and Engineering Solutions could be amended to include “lotteries”:
Sec. 1308(d)(4)(E). developing and testing diverse merit-review models and mechanisms, including lotteries, for selecting and providing awards for use-inspired and translational research and development at different scales, from individual investigator awards to large multi-institution collaborations;
Specifying language should then require the NSF to employ evidence-based evaluation criteria and grant it the flexibility to determine timeline of the lottery intake and award mechanisms, with broader goals of timeliness and supporting the equitable distribution among regional innovation contenders.
The appendix contains one example structure of a science lottery in bill text (incorporated into the new NSF Directorate established by the Senate-passed United States Innovation and Competition Act), which includes the following key policy choices that Congress should consider:
- Limiting eligibility to meritorious proposals;
- Ensuring that proposals are timely;
- Limiting the grant proposal size to provide the maximum number of awards and create a large sample to fairly evaluate the success of a lottery program;
- Rigorous stakeholder feedback mechanisms from the scientific research community;
- Fast-tracking award distribution following a lottery; and
- Regular reports to Congress in accordance with the NSF’s Open Science Policy to ensure transparency; accountability; and rigorous evaluation.
Recommendation 2: Create a “Translational Science of Science” Program within the new NSF Technology, Innovation and Partnerships Directorate that pilots the use of lotteries with evidence-based testing:
First, the NSF Office of Integrative Activities (OIA) should convene a workshop with relevant stakeholders including representatives from each directorate, the research community including NSF grant recipients, non-recipients, and SME’s on programmatic implementation from New Zealand, Germany, and Switzerland in order to temperature- and pressure-test key criteria for implementing piloted science lotteries across directorates.
- The initial goal of the workshop should be to gather feedback and gauge interest from the PI community on this topic. To this end, it would be wise to explore varying elements in science lottery construction to appreciate which are most supported from the PI community. The community, for example, should be involved in the development of baseline parameters for proposal quality and a timely, equitable process, despite varying directorate application deadlines. This might include applicants’ consented sign-off before entrance into the lottery, upfront and consistent communications of timelines, and randomization and selection from a pool with scores of at least [excellent/very good/good] during the peer evaluation process described in the NSF’s “Proposal and Award Policies and Procedures Guide”.
- Another goal of this workshop would be to scope the process of an OIA inter-directorate competition to submit applications in order to receive an award from the Division of Grants and Agreements to pursue pilot science lottery. The workshop should therefore develop a clear sense of opportunities with respect to budget sizing for each directorate and could consider making recommendations about the placement of science lottery pilots across directorates based on willingness to devote experimental resources. To maximize the number of lottery recipients, the proposal must not exceed 200% of the median grant proposal to a given directorate;
- Finally, a third goal of the workshop should be to explore standards and timeframe for evidence-based evaluation mechanisms as described above and in the bill-text below, including stakeholder feedback mechanisms, regular reports to Congress, and transparency requirements. Additional mechanisms might include detailed reports on grants and awardees like demographic and geographic information of awardees, comparison of outcomes from traditional awardees and lottery awardees, and a statistical picture of the entire pool of grant proposals entered into the lottery. If the workshop is based on competitive directorate applications, the General Services Administration’s Office of Evaluation Sciences (OES) should be invited for later-stage workshop convenings to provide technical assistance in designing evaluation criteria. Some unifying criteria include meeting the requirements of the NSF’s Open Science Policy, Public Access Policy, and making grant information public as soon as feasible to facilitate rapid evaluation from external stakeholders — a potential metric to judge directorate applications.
Appendix: Bill Text
Note: Please view attached PDF for the formatted bill text
H. ______
To establish a pilot program for National Science Foundation grant lotteries.
In the House of Representatives of the United States
February 2, 2022
______________________________
A BILL
Title: To establish a pilot program for National Science Foundation grant lotteries.
Be it enacted by the Senate and House of Representatives of the United States of America in Congress assembled,
SEC. _____. Pilot Program to Establish National Science Foundation Grant Lotteries
- Findings.— Congress makes the following findings:
- Over the past seven decades, the National Science Foundation has played a critical role in advancing the United States academic research enterprise by supporting fundamental research and education across all scientific disciplines;
- The National Science Foundation has made remarkable contributions to scientific advancement, economic growth, human health, and national security, and its peer review and merit review processes have identified and funded scientifically and societally relevant basic research;
- Every year, thousands of meritorious grant proposals do not receive National Science Foundation grants, threatening the United States’ leadership in science and technology and harming our efforts to lead translation and development of scientific advances in key technology areas; and
- While Congress reaffirms its belief that the National Science Foundation’s merit-review system is appropriate for evaluating grant proposals, Congress should establish efforts to explore alternative mechanisms for distributing grants and evaluating, objectively, whether it can supplement the merit-review system by funding worthwhile projects that otherwise go unawarded.
- Definitions.—In this section:
- Directorate.— The term “Directorate” refers to the Directorate for Technology and Innovation established in Sec. 2102 of this Act.
- Assistant Director.— The term “Assistant Director” refers to the Assistant Director for the Directorate described in Sec. 2102(d) of this Act.
- Foundation.—The term “Foundation” refers to the National Science Foundation.
- PAPPG.—The term “PAPPG” refers to the document entitled “OMB Control Number 3145-0058,” also known as the Proposal and Award Policies and Procedures Guide, published by the National Science Foundation, also published as NSF 22-1.
- Program.—The term “program” refers to the program established in subparagraph (d) of this section.
- Grant request.—The term “grant request” refers to the amount of funding requested in an individual grant proposal to the National Science Foundation.
- Lottery awardee.—The term “lottery awardee” refers to a grant proposal selected for award during a lottery established by this section.
- Lottery year.—The term “lottery year” refers to the calendar year of eligibility for proposals, as determined by the Assistant Director, for a lottery established under this program.
- Purpose.—It is the purpose of this section to establish a pilot program for merit-based lotteries to award scientific research grants in order to:
- Provide grants to meritorious but unawarded grant proposals;
- Explore “second-look” mechanisms to distribute grants to meritorious but overlooked grant proposals; and
- To evaluate whether alternative mechanisms can supplement the Foundation’s merit-review system.
- Establishment.— No later than 180 days after establishment of the Directorate, the Assistant Director shall establish a lottery program to provide second-look grants for meritorious grant proposals that were declined funding by the Foundation.
- Requirements.
- Eligibility.—A grant proposal shall be eligible for a lottery if:
- It did not receive funding from the Foundation;
- The grant proposal received an overall evaluation score deemed meritorious during the peer review process;
- Meritorious.—The Assistant Director determine a minimum score that a proposal must receive during the peer evaluation process described in Chapter III of the PAPPG to be deemed meritorious.
- The grant request does not exceed 200 percent of the median grant request to a given directorate in the calendar year with the most recently available data;
- The grant was proposed to one of the following directorates within the Foundation:
- Biological Sciences;
- Computer and Information Science and Engineering;
- Engineering;
- Geosciences; or
- Mathematical and Physical Sciences;
- Social, Behavioral, and Economic Sciences;
- Education and Human Resources;
- Environmental Research and Education;
- International Science of Engineering;
- The grant has been deemed timely by a Foundation Program Officer; and
- Any other criteria deemed necessary by the Assistant Director
- Exemptions.—If deemed necessary or worthwhile to further the mission and goals of the Directorate or the Foundation, the Assistant Director may:
- Exempt grant proposals from the requirement in subparagraph (e)(1)(D); and
- Determine an appropriate method to include such exempted proposals in a lottery.
- Stakeholder Feedback.—Prior to finalizing eligibility requirements, the Assistant Director shall, to the extent practicable, ensure that the requirements take into consideration advice and feedback from the scientific research community. The Federal Advisory Committee Act (5 U.S.C. App.) shall not apply whenever such advice or feedback is sought in accordance with this subsection.
- Eligibility.—A grant proposal shall be eligible for a lottery if:
- Implementation.
- Policies and Procedures.—The Assistant Director shall:
- Develop procedures and policies to ensure that each grant lottery:
- Is randomized and affords equal opportunity to all participants; and
- Is not susceptible to fraud;
- Ensure that grant amounts are distributed equitably among the directorates described in subparagraph (e)(1)(D);
- Ensure that relevant external parties have due notice of their obligations with respect to participation in a lottery;
- Ensure that relevant staff and officers of the Foundation are aware of their duties and responsibilities with respect to implementation of the program;
- Ensure that ranked alternative awardees are selected for each lottery in the event that:
- a lottery awardee withdraws their application;
- a lottery awardee receives Foundation funding following an appeals process; or
- is otherwise deemed ineligible for a Foundation grant.
- Grant Approval.—Once a proposal has been selected for an award:
- It shall be submitted to the Division of Grants and Agreements for a review of business, financial, and policy implications and award finalization thereafter, as described in PAPPG Chapter III; and
- It shall not be declined funding by the Division of Grants and Agreements unless granting the award would result in fraud, abuse, or other outcomes deemed egregious and antithetical to the mission of the Foundation.
- Lottery timeline.—For each directorate specified in subparagraph (e)(1)(D), the Assistant Director shall administer a lottery for each calendar year ending in the years [2022, 2023, and 2024].
- Stakeholder Feedback.—Prior to finalizing lottery implementation, and subsequent to conducting each lottery, the Assistant Director shall, to the extent practicable, ensure that lottery implementation takes into consideration advice and feedback from the scientific research community. The Federal Advisory Committee Act (5 U.S.C. App.) shall not apply whenever such advice or feedback is sought in accordance with this subsection.
- Develop procedures and policies to ensure that each grant lottery:
- Deadline of Submission of Grants to the Directorate.—No later than [90 days] following a given lottery year, Foundation Program Officers shall submit all grant proposals that meet the criteria described in subparagraphs (e)(1)(A)—(e)(1)(F) of this section.
- Authorization of Appropriation.— There is authorized to be appropriated to the Foundation [$—,000,000] to carry out this Section.
- Evaluation and Oversight and Public Access.
- Evaluation.—The Assistant Director shall:
- Ensure that awards are evaluated using the same methods and procedures as other grant programs of the Foundation, including as set forth by the Foundation’s Evaluation and Assessment Capability and the Foundation’s values of learning, excellence, inclusion, collaboration, integrity, and transparency; and
- Establish a rapid, empirically-based evaluation program to determine the effectiveness of the lottery program.
- Reports to Congress.—
- Periodic.— No later than 180 days following completion of a lottery, the Assistant Director shall submit a summary report to Congress including:
- A list of all grants awarded;
- Demographic information of the grant awardees;
- Geographic information of the grant awardees;
- Information regarding the institutions receiving grants;
- An assessment comparing lottery grant awardees with those awarded grants through the Foundation’s traditional review process;
- Information and data describing the entire pool of grant proposals deemed eligible for the lottery.
- Any other information deemed necessary or valuable by the Assistant Director;
- Yearly.—Not later than [two years] following the first lottery, the Assistant Director shall submit comprehensive reports on a yearly basis, for a period of five years after the report submission, evaluating awards using the Foundation’s Evaluation and Assessment Capability or other assessment methods used to evaluate grants awarded through the traditional grant process;
- Final report.—Within [3 years] of completion of the final lottery, the Assistant Director shall submit a final report to Congress evaluating the success of the program and assessing whether Congress should make the program permanent.
- Periodic.— No later than 180 days following completion of a lottery, the Assistant Director shall submit a summary report to Congress including:
- Public Access.—The Assistant Director shall:
- Ensure that the program meets the requirements of the Foundation’s:
- Open Science Policy;
- Public Access Policy; and
- General values of learning, transparency, and integrity.
- Make grant information available to the public as soon as is feasible to facilitate rapid, empirically-based evaluation by external stakeholders;
- Ensure that the program meets the requirements of the Foundation’s:
- Evaluation.—The Assistant Director shall:
- Duties, Conditions, Restrictions, and Prohibitions.—
Right to Review.—Nothing in this section shall affect an applicant’s right to review, appeal, or contest an award decision.
Reforming Federal Rules on Corporate-Sponsored Research at Tax-Exempt University Facilities
Improving university/corporate research partnerships is key to advancing U.S. competitiveness. Reform of the IRS rules surrounding corporate sponsored research taking place in university facilities funded by tax-exempt bonds has long been sought by the higher education community and will stimulate more public-private partnerships. With Congress considering new ways to fund research through a new NSF Technology Directorate and the possibility of a large infrastructure package, an opportunity is now open for Congress to address these long-standing reforms in IRS rules.
Challenge and Opportunity
Research partnerships between private companies and universities are critical to U.S. technology competitiveness. China and other countries are creating massive, government-funded research centers in artificial intelligence, robotics, quantum computing, biotechnology, and other critical sectors, threatening our nation’s international technology advantage. The United States has responded with initiatives such as the corporate research and development (R&D) tax credit, the SBIR and STTR programs, Manufacturing USA institutes, and numerous other programs and policies to assist tech development and encourage public-private collaborations. States and cities have mirrored these efforts, helping to build a network of innovation hubs in communities across the nation
The U.S. Innovation and Competition Act recently passed by the Senate is designed to build on this progress. A key provision of the Act is the establishment of a National Science Foundation (NSF) Directorate for Science and Technology that would “identify and develop opportunities to reduce barriers for technology transfer, including intellectual property frameworks between academia and industry, nonprofit entities, and the venture capital communities.”
One such barrier is the suite of “private use” rules surrounding corporate research taking place in university facilities financed with tax-exempt bonds. Tax-exempt bonds are a preferred financing option for university research facilities as they carry lower interest rates and more favorable terms. But the Internal Revenue Service (IRS) prohibition on “private business use“ of facilities financed using tax-exempt bonds have the unfortunate consequence of hamstringing the U.S. research enterprise. Current IRS rules place universities wishing to avoid concerns about sponsoring research from outside organizations to hold the rights to almost all intellectual property (IP) generated within their research facilities, even when the research is sponsored by private corporations. This can lead U.S. corporations wishing to retain IP rights to partner with universities overseas instead of U.S. universities institutions. Small technology companies whose business plans depend on their claim to IP rights may similarly avoid partnerships with universities.
Though the IRS has issued policies that aim to address these problems (e.g., Revenue Procedure 2007-47), such policies are so narrow in scope that most research partnerships between companies and universities are still considered private uses. As a result, universities engaged in cutting-edge, industry-relevant research face an unenviable choice: they must either (i) forego promising partnerships with the many companies unwilling to completely cede claims to IP rights, (ii) dedicate substantial time and administrative resources to track and report all specific instances of corporate-sponsored research occurring in facilities financed by tax-exempt bonds, or (iii) use funding that would otherwise be available for research to finance facilities through taxable bonds.
Forcing this choice upon universities further exacerbates a system of “haves” and “have-nots”. Large and/or well-endowed universities may have the financial resources to avoid relying on tax-exempt financing for research facilities, or to hire sophisticated and expensive legal expertise for help structuring financing in a way that complies with IRS rules. But for many — perhaps most — universities, the more viable solution is to avoid corporate-sponsored research altogether.
Complex federal rules governing intellectual property and private business use are widely acknowledged as an issue. A memo from the Association of American Universities (AAU), which represents the leading research universities in North America, notes that “[m]any universities believe that the remaining [IRS] private use regulations are overly restrictive” and “[limit] their ability to conduct certain cooperative research.” Similarly, the website of the Carnegie Mellon University Office of Sponsored Programs warns:
“While colleges and universities have lobbied the Internal Revenue Service to reconsider its position with respect to sponsored research in bond financed facilities, they have not, as yet, been successful. Consequently, if the University does not receive fair market royalties from the sponsors of sponsored research, it risks having its tax-exempt bonds become taxable, with all of the concomitant consequences.”
At a 2013 hearing on “Improving Technology Transfer at Universities, Research Institute and National Laboratories” before the U.S. House of Representatives Committee on Science, Space and Technology, several university witnesses and members of Congress commented on the complications that federal rules present for cooperative research conducted by universities working in partnership with corporations.
In 2014, Congress introduced H.R. 5829 to amend the Internal Revenue Code to provide an exception from the “business use” test for certain public-private research arrangements, but it did not pass as a stand-alone bill.
In June 2021, the American Council of Education and Association of American Universities released a letter to Congress on behalf of over 20 higher education organizations asking Congress to modernize rules on tax exempt bond financing.
Overly restrictive federal rules may hamstring bipartisan efforts by the new administration and Congress to accelerate tech commercialization and enhance U.S. competitiveness in science and technology (S&T). The recent U.S. Innovation and Competition Act, passed by the Senate, for instance, aims to support public-private partnerships, cross-sectoral innovation hubs, and other multistakeholder initiatives in priority S&T areas. But such initiatives may run afoul of rules on facilities financed by tax-exempt bonds…unless reforms are adopted.
Plan of Action
The administration should implement the following two reforms to clarify and update rules governing use of facilities financed by tax-exempt bonds:
- Eliminate the requirement that universities must retain ownership to all IP generated in university-owned facilities financed by tax-exempt bonds. Instead, universities and corporations should be allowed to negotiate their own terms of IP ownership before entering a research partnership.
- Broaden applicability of IRS safe-harbor provisions. IRS revenue procedures include safe-harbor provisions that exempt “basic research agreements” from restrictions on private business use. The IRS defines basic research as “any original investigation for the advancement of scientific knowledge not having a specific commercial objective.” This definition is too narrow. But especially today, the lines between “basic” and applied research are blurry — and virtually nonexistent when it comes to cutting-edge fields such as digitalization, biosciences, and quantum computing. The IRS should broaden the applicability of its safe-harbor provisions to include all research activities, not just ‘basic research’.
Together, these reforms would support new public-private initiatives by the federal government (such the research hubs funded under the U.S. Innovation and Competition Act); help emerging research universities (including minority-serving institutions such as historically black colleges and universities (HBCUs) and Hispanic-serving institutions (HSIs)) grow their profiles and better compete for talent and resources; and repatriate corporate research to the United States. Moreover — since other countries do not have similarly onerous restrictions on research activities conducted in facilities financed with tax-exempt bonds — these reforms are needed for the U.S. tech economy to remain competitive on an international scale.
These reforms require changes to tax laws, but do not require a direct outlay of federal appropriations. Reforms could be implemented as part of several tech-commercialization legislative packages expected to be considered by this Congress, including the U.S. Innovation and Competition Act or the proposed US Infrastructure bill.
Conclusion
As the Congress and the Administration explore ways to make the U.S. more technologically competitive, ensuring robust university-industry partnerships should be a key factor in any strategy. Reforming the current rules concerning corporate research performed in university facilities needs to be considered, given that the IRS rules have not been updated in over 30 years. The debate over the infrastructure bill or other competitiveness initiatives provides such an opportunity to make these reforms. Now is the time.
A Profile of Defense Science & Tech Spending
Annual spending on defense science and technology has “grown substantially” over the past four decades from $2.3 billion in FY1978 to $13.4 billion in FY2018 or by nearly 90% in constant dollars, according to a new report from the Congressional Research Service.
Defense science and technology refers to the early stages of military research and development, including basic research (known by its budget code 6.1), applied research (6.2) and advanced technology development (6.3).
“While there is little direct opposition to Defense S&T spending in its own right,” the CRS report says, “there is intense competition for available dollars in the appropriations process,” such that sustained R&D spending is never guaranteed.
Still, “some have questioned the effectiveness of defense investments in R&D.”
CRS takes note of a 2012 article published by the Center for American Progress which argued that military spending was an inefficient way to spur innovation and that the growing sophistication of military technology was poorly suited to meet some low-tech threats such as improvised explosive devices (IEDs) in Iraq and Afghanistan (as discussed in an earlier article in the Bulletin of the Atomic Scientists).
The new CRS report presents an overview of the defense science and tech budget, its role in national defense, and questions about its proper size and proportion. See Defense Science and Technology Funding, February 21, 2018,
Other new and updated reports from the Congressional Research Service include the following.
Armed Conflict in Syria: Overview and U.S. Response, updated February 16, 2018
Jordan: Background and U.S. Relations, updated February 16, 2018
Bahrain: Reform, Security, and U.S. Policy, updated February 15, 2018
Potential Options for Electric Power Resiliency in the U.S. Virgin Islands, February 14, 2018
U.S. Manufacturing in International Perspective, updated February 21, 2018
Methane and Other Air Pollution Issues in Natural Gas Systems, updated February 15, 2018
Where Can Corporations Be Sued for Patent Infringement? Part I, CRS Legal Sidebar, February 20, 2018
How Broad A Shield? A Brief Overview of Section 230 of the Communications Decency Act, CRS Legal Sidebar, February 21, 2018
Russians Indicted for Online Election Trolling, CRS Legal Sidebar, February 21, 2018
Hunting and Fishing on Federal Lands and Waters: Overview and Issues for Congress, February 14, 2018