Predicting Progress: A Pilot of Expected Utility Forecasting in Science Funding
Read more about expected utility forecasting and science funding innovation here.
The current process that federal science agencies use for reviewing grant proposals is known to be biased against riskier proposals. As such, the metascience community has proposed many alternate approaches to evaluating grant proposals that could improve science funding outcomes. One such approach was proposed by Chiara Franzoni and Paula Stephan in a paper on how expected utility — a formal quantitative measure of predicted success and impact — could be a better metric for assessing the risk and reward profile of science proposals. Inspired by their paper, the Federation of American Scientists (FAS) collaborated with Metaculus to run a pilot study of this approach. In this working paper, we share the results of that pilot and its implications for future implementation of expected utility forecasting in science funding review.
Brief Description of the Study
In fall 2023, we recruited a small cohort of subject matter experts to review five life science proposals by forecasting their expected utility. For each proposal, this consisted of defining two research milestones in consultation with the project leads and asking reviewers to make three forecasts for each milestone:
- the probability of success;
- The scientific impact of the milestone, if it were reached; and
- The social impact of the milestone, if it were reached.
These predictions can then be used to calculate the expected utility, or likely impact, of a proposal and design and compare potential portfolios.
Key Takeaways for Grantmakers and Policymakers
The three main strengths of using expected utility forecasting to conduct peer review are
- For reviewers, it’s a relatively light-touch approach that encourages rigor and reduces anti-risk bias in scientific funding.
- The review criteria allow program managers to better understand the risk-reward profile of their grant portfolios and more intentionally shape them according to programmatic goals.
- Quantitative forecasts are resolvable, meaning that program officers can compare the actual outcomes of funded proposals with reviewers’ predictions. This generates a feedback/learning loop within the peer review process that incentivizes reviewers to improve the accuracy of their assessments over time.
Despite the apparent complexity of this process, we found that first-time users were able to successfully complete their review according to the guidelines without any additional support. Most of the complexity occurs behind-the-scenes, and either aligns with the responsibilities of the program manager (e.g., defining milestones and their dependencies) or can be automated (e.g., calculating the total expected utility). Thus, grantmakers and policymakers can have confidence in the user friendliness of expected utility forecasting.
How Can NSF or NIH Run an Experiment on Expected Utility Forecasting?
An initial pilot study could be conducted by NSF or NIH by adding a short, non-binding expected utility forecasting component to a selection of review panels. In addition to the evaluation of traditional criteria, reviewers would be asked to predict the success and impact of select milestones for the proposals assigned to them. The rest of the review process and the final funding decisions would be made using the traditional criteria.
Afterwards, study facilitators could take the expected utility forecasting results and construct an alternate portfolio of proposals that would have been funded if that approach was used, and compare the two portfolios. Such a comparison would yield valuable insights into whether—and how—the types of proposals selected by each approach differ, and whether their use leads to different considerations arising during review. Additionally, a pilot assessment of reviewers’ prediction accuracy could be conducted by asking program officers to assess milestone achievement and study impact upon completion of funded projects.
Findings and Recommendations
Reviewers in our study were new to the expected utility forecasting process and gave generally positive reactions. In their feedback, reviewers said that they appreciated how the framing of the questions prompted them to think about the proposals in a different way and pushed them to ground their assessments with quantitative forecasts. The focus on just three review criteria–probability of success, scientific impact, and social impact–was seen as a strength because it simplified the process, disentangled feasibility from impact, and eliminated biased metrics. Overall, reviewers found this new approach interesting and worth investigating further.
In designing this pilot and analyzing the results, we identified several important considerations for planning such a review process. While complex, engaging with these considerations tended to provide value by making implicit project details explicit and encouraging clear definition and communication of evaluation criteria to reviewers. Two key examples are defining the proposal milestones and creating impact scoring systems. In both cases, reducing ambiguities in terms of the goals that are to be achieved, developing an understanding of how outcomes depend on one another, and creating interpretable and resolvable criteria for assessment will help ensure that the desired information is solicited from reviewers.
Questions for Further Study
Our pilot only simulated the individual review phase of grant proposals and did not simulate a full review committee. The typical review process at a funding agency consists of first, individual evaluations by assigned reviewers, then discussion of those evaluations by the whole review committee, and finally, the submission of final scores from all members of the committee. This is similar to the Delphi method, a structured process for eliciting forecasts from a panel of experts, so we believe that it would work well with expected utility forecasting. The primary change would therefore be in the definition and approach for eliciting criterion scores, rather than the structure of the review process. Nevertheless, future implementations may uncover additional considerations that need to be addressed or better ways to incorporate forecasting into a panel environment.
Further investigation into how best to define proposal milestones is also needed. This includes questions such as, who should be responsible for determining the milestones? If reviewers are involved, at what part(s) of the review process should this occur? What is the right balance between precision and flexibility of milestone definitions, such that the best outcomes are achieved? How much flexibility should there be in the number of milestones per proposal?
Lastly, more thought should be given to how to define social impact and how to calibrate reviewers’ interpretation of the impact score scale. In our report, we propose a couple of different options for calibrating impact, in addition to describing the one we took in our pilot.
Interested grantmakers, both public and private, and policymakers are welcome to reach out to our team if interested in learning more or receiving assistance in implementing this approach.
Introduction
The fundamental concern of grantmakers, whether governmental or philanthropic, is how to make the best funding decisions. All funding decisions come with inherent uncertainties that may pose risks to the investment. Thus, a certain level of risk-aversion is natural and even desirable in grantmaking institutions, especially federal science agencies which are responsible for managing taxpayer dollars. However, without risk, there is no reward, so the trade-off must be balanced. In mathematics and economics, expected utility is the common metric assumed to underlie all rational decision making. Expected utility has two components: the probability of an outcome occurring if an action is taken and the value of that outcome, which roughly corresponds with risk and reward. Thus, expected utility would seem to be a logical choice for evaluating science funding proposals.
In the debates around funding innovation though, expected utility has largely flown under the radar compared to other ideas. Nevertheless, Chiara Franzoni and Paula Stephan have proposed using expected utility in peer review. Building off of their paper, the Federation of American Scientists (FAS) developed a detailed framework for how to implement expected utility into a peer review process. We chose to frame the review criteria as forecasting questions, since determining the expected utility of a proposal inherently requires making some predictions about the future. Forecasting questions also have the added benefit of being resolvable–i.e., the true outcome can be determined after the fact and compared to the prediction–which provides a learning opportunity for reviewers to improve their abilities and identify biases. In addition to forecasting, we incorporated other unique features, like an exponential scale for scoring impact, that we believe help reduce biases against risky proposals.
With the theory laid out, we conducted a small pilot in fall of 2023. The pilot was run in collaboration with Metaculus, a crowd forecasting platform and aggregator, to leverage their expertise in designing resolvable forecasting questions and to use their platform to collect forecasts from reviewers. The purpose of the pilot was to test the mechanics of this approach in practice, see if there are any additional considerations that need to be thought through, and surface potential issues that need to be solved for. We were also curious if there would be any interesting or unexpected results that arise based on how we chose to calculate impact and total expected utility. It is important to note that this pilot was not an experiment, so we did not have a control group to compare the results of the review with.
Since FAS is not a grantmaking institution, we did not have a ready supply of traditional grant proposals to use. Instead, we used a set of two-page research proposals for Focused Research Organizations (FROs) that we had sourced through separate advocacy work in that area.1 With the proposal authors’ permission, we recruited a cohort of twenty subject matter experts to each review one of five proposals. For each proposal, we defined two research milestones in consultation with the proposal authors. Reviewers were asked to make three forecasts for each milestone:
- The probability of success;
- The scientific impact, conditional on success; and
- The social impact, conditional on success.
Reviewers submitted their forecasts on Metaculus’ platform; in a separate form they provided explanations for their forecasts and responded to questions about their experience and impression of this new approach to proposal evaluation. (See Appendix A for details on the pilot study design.)
Insights from Reviewer Feedback
Overall, reviewers liked the framing and criteria provided by the expected utility approach, while their main critique was of the structure of the research proposals. Excluding critiques of the research proposal structure, which are unlikely to apply to an actual grant program, two thirds of the reviewers expressed positive opinions of the review process and/or thought it was worth pursuing further given drawbacks with existing review processes. Below, we delve into the details of the feedback we received from reviewers and their implications for future implementation.
Feedback on Review Criteria
Disentangling Impact from Feasibility
Many of the reviewers said that this model prompted them to think differently about how they assess the proposals and that they liked the new questions. Reviewers appreciated that the questions focused their attention on what they think funding agencies really want to know and nothing more: “can it occur?” and “will it matter?” This approach explicitly disentangles impact from feasibility: “Often, these two are taken together, and if one doesn’t think it is likely to succeed, the impact is also seen as lower.” Additionally, the emphasis on big picture scientific and social impact “is often missing in the typical review process.” Reviewers also liked that this approach eliminates what they consider biased metrics, such as the principal investigator’s reputation, track record, and “excellence.”
Reducing Administrative Burden
The small set of questions was seen as more efficient and less burdensome on reviewers. One reviewer said, “I liked this approach to scoring a proposal. It reduces the effort to thinking about perceived impact and feasibility.” Another reviewer said, “On the whole it seems a worthwhile exercise as the current review processes for proposals are onerous.”
Quantitative Forecasting
Reviewers saw benefits to being asked to quantify their assessments, but also found it challenging at times. A number of reviewers enjoyed taking a quantitative approach and thought that it helped them be more grounded and explicit in their evaluations of the proposals. However, some reviewers were concerned that it felt like guesswork and expressed low confidence in their quantitative assessments, primarily due to proposals lacking details on their planned research methods, which is an issue discussed in the section “Feedback on Proposals.” Nevertheless, some of these reviewers still saw benefits to taking a quantitative approach: “It is interesting to try to estimate probabilities, rather than making flat statements, but I don’t think I guess very well. It is better than simply classically reviewing the proposal [though].” Since not all academics have experience making quantitative predictions, we expect that there will be a learning curve for those new to the practice. Forecasting is a skill that can be learned though, and we think that with training and feedback, reviewers can become better, more confident forecasters.
Defining Social Impact
Of the three types of questions that reviewers were asked to answer, the question about social impact seemed the harder one for reviewers to interpret. Reviewers noted that they would have liked more guidance on what was meant by social impact and whether that included indirect impacts. Since questions like these are ultimately subjective, the “right” definition of social impact and what types of outcomes are considered most valuable will depend on the grantmaking institution, their domain area, and their theory of change, so we leave this open to future implementers to clarify in their instructions.
Calibrating Impact
While the impact score scale (see Appendix A) defines the relative difference in impact between scores, it does not define the absolute impact conveyed by a score. For this reason, a calibration mechanism is necessary to provide reviewers with a shared understanding of the use and interpretation of the scoring system. Note that this is a challenge that rubric-based peer review criteria used by science agencies also face. Discussion and aggregation of scores across a review committee helps align reviewers and average out some of this natural variation.2
To address this, we surveyed a small, separate set of academics in the life sciences about how they would score the social and scientific impact of the average NIH R01 grant, which many life science researchers apply to and review proposals for. We then provided the average scores from this survey to reviewers to orient them to the new scale and help them calibrate their scores.
One reviewer suggested an alternative approach: “The other thing I might change is having a test/baseline question for every reviewer to respond to, so you can get a feel for how we skew in terms of assessing impact on both scientific and social aspects.” One option would be to ask reviewers to score the social and scientific impact of the average grant proposal for a grant program that all reviewers would be familiar with; another would be to ask reviewers to score the impact of the average funded grant for a specific grant program, which could be more accessible for new reviewers who have not previously reviewed grant proposals. A third option would be to provide all reviewers on a committee with one or more sample proposals to score and discuss, in a relevant and shared domain area.
When deciding on an approach for calibration, a key consideration is the specific resolution criteria that are being used — i.e., the downstream measures of impact that reviewers are being asked to predict. One option, which was used in our pilot, is to predict the scores that a comparable, but independent, panel of reviewers would give the project some number of years following its successful completion. For a resolution criterion like this one, collecting and sharing calibration scores can help reviewers get a sense for not just their own approach to scoring, but also those of their peers.
Making Funding Decisions
In scoring the social and scientific impact of each proposal, reviewers were asked to assess the value of the proposal to society or to the scientific field. That alone would be insufficient to determine whether a proposal should be funded though, since it would need to be compared with other proposals in conjunction with its feasibility. To do so, we calculated the total expected utility of each proposal (see Appendix C). In a real funding scenario, this final metric could then be used to compare proposals and determine which ones get funded. Additionally, unlike a traditional scoring system, the expected utility approach allows for the detailed comparison of portfolios — including considerations like the expected proportion of milestones reached and the range of likely impacts.
In our pilot, reviewers were not informed that we would be doing this additional calculation based on their submissions. As a result, one reviewer thought that the questions they were asked failed to include other important questions, like “should it occur?” and “is it worth the opportunity cost?” Though these questions were not asked of reviewers explicitly, we believe that they would be answered once the expected utility of all proposals is calculated and considered, since the opportunity cost of one proposal would be the expected utility of the other proposals. Since each reviewer only provided input on one proposal, they may have felt like the scores they gave would be used to make a binary yes/no decision on whether to fund that one proposal, rather than being considered as a part of a larger pool of proposals, as it would be in a real review process.
Feedback on Proposals
Missing Information Impedes Forecasting
The primary critique that reviewers expressed was that the research proposals lacked details about their research plans, what methods and experimental protocols would be used, and what preliminary research the author(s) had done so far. This hindered their ability to properly assess the technical feasibility of the proposals and their probability of success. A few reviewers expressed that they also would have liked to have had a better sense of who would be conducting the research and each team member’s responsibilities. These issues arose because the FRO proposals used in our pilot had not originally been submitted for funding purposes, and thus lacked the requirements of traditional grant proposals, as we noted above. We assume this would not be an issue with proposals submitted to actual grantmakers.3
Improving Milestone Design
A few reviewers pointed out that some of the proposal milestones were too ambiguous or were not worded specifically enough, such that there were ways that researchers could technically say that they had achieved the milestone without accomplishing the spirit of its intent. This made it more challenging for reviewers to assess milestones, since they weren’t sure whether to focus on the ideal (i.e., more impactful) interpretation of the milestone or to account for these “loopholes.” Moreover, loopholes skew the forecasts, since they increase the probability of achieving a milestone, while lowering the impact of doing so if it is achieved through a loophole.
One reviewer suggested, “I feel like the design of milestones should be far more carefully worded – or broken up into sub-sentences/sub-aims, to evaluate the feasibility of each. As the questions are currently broken down, I feel they create a perverse incentive to create a vaguer milestone, or one that can be more easily considered ‘achieved’ for some ‘good enough’ value of achieved.” For example, they proposed that one of the proposal milestones, “screen a library of tens of thousands of phage genes for enterobacteria for interactions and publish promising new interactions for the field to study,” could be expanded to
- “Generate a library of tens of thousands of genes from enterobacteria, expressed in E. coli
- “Validate their expression under screenable conditions
- “Screen the library for their ability to impede phage infection with a panel of 20 type phages
- “Publish …
- “Store and distribute the library, making it as accessible to the broader community”
We agree with the need for careful consideration and design of milestones, given that “loopholes” in milestones can detract from their intended impact and make it harder for reviewers to accurately assess their likelihood. In our theoretical framework for this approach, we identified three potential parties that could be responsible for defining milestones: (1) the proposal author(s), (2) the program manager, with or without input from proposal authors, or (3) the reviewers, with or without input from proposal authors. This critique suggests that the first approach of allowing proposal authors to be the sole party responsible for defining proposal milestones is vulnerable to being gamed, and the second or third approach would be preferable. Program managers who take on the task of defining milestones should have enough expertise to think through the different potential ways of fulfilling a milestone and make sure that they are sufficiently precise for reviewers to assess.
Benefits of Flexibility in Milestones
Some flexibility in milestones may still be desirable, especially with respect to the actual methodology, since experimentation may be necessary to determine the best technique to use. For example, speaking about the feasibility of a different proposal milestone – “demonstrate that Pro-AG technology can be adapted to a single pathogenic bacterial strain in a 300 gallon aquarium of fish and successfully reduce antibiotic resistance by 90%” – a reviewer noted that
“The main complexity and uncertainty around successful completion of this milestone arises from the native fish microbiome and whether a CRISPR delivery tool can reach the target strain in question. Due to the framing of this milestone, should a single strain be very difficult to reach, the authors could simply switch to a different target strain if necessary. Additionally, the mode of CRISPR delivery is not prescribed in reaching this milestone, so the authors have a host of different techniques open to them, including conjugative delivery by a probiotic donor or delivery by engineered bacteriophage.”
Peer Review Results
Sequential Milestones vs. Independent Outcomes
In our expected utility forecasting framework, we defined two different ways that a proposal could structure its outcomes: as sequential milestones where each additional milestone builds off of the success of the previous one, or as independent outcomes where the success of one is not dependent on the success of the other(s). For proposals with sequential milestones in our pilot, we would expect the probability of success of milestone 2 to be less than the probability of success of milestone 1 and for the opposite to be true of their impact scores. For proposals with independent outcomes, we do not expect there to be a relationship between the probability of success and the impact scores of milestones 1 and 2. There are different equations for calculating the total expected utility, depending on the relationship between outcomes (see Appendix C).
For each of the proposals in our study, we categorized them based on whether they had sequential milestones or independent outcomes. This information was not shared with reviewers. Table 1 presents the average reviewer forecasts for each proposal. In general, milestones received higher scientific impact scores than social impact scores, which makes sense given the primarily academic focus of research proposals. For proposals 1 to 3, the probability of success of milestone 2 was roughly half of the probability of success of milestone 1; reviewers also gave milestone 2 higher scientific and social impact scores than milestone 1. This is consistent with our categorization of proposals 1 to 3 as sequential milestones.
Further Discussion on Designing and Categorizing Milestones
We originally categorized proposal 4’s milestones as sequential, but one reviewer gave milestone 2 a lower scientific impact score than milestone 1 and two reviewers gave it a lower social impact score. One reviewer also gave milestone 2 roughly the same probability of success as milestone 1. This suggests that proposal 4’s milestones can’t be considered strictly sequential.
The two milestones for proposal 4 were
- Milestone 1: Develop a tool that is able to perturb neurons in C. elegans and record from all neurons simultaneously, automated w/ microfluidics, and
- Milestone 2: Develop a model of the C. elegans nervous system that can predict what every neuron will do when stimulating one neuron with R2 > 0.8
The reviewer who gave milestone 2 a lower scientific impact score explained: “Given the wording of the milestone, I do not believe that if the scientific milestone was achieved, it would greatly improve our understanding of the brain.” Unlike proposals 1-3, in which milestone 2 was a scaled-up or improved-upon version of milestone 1, these milestones represent fundamentally different categories of output (general-purpose tool vs specific model). Thus, despite the necessity of milestone 1’s tool for achieving milestone 2, the reviewer’s response suggests that the impact of milestone 2 was being considered separately rather than cumulatively.
To properly address this case of sequential milestones with different types of outputs, we recommend that for all sequential milestones, latter milestones should be explicitly defined as inclusive of prior milestones. In the above example, this would imply redefining milestone 2 as “Complete milestone 1 and develop a model of the C. elegans nervous system…” This way, reviewers know to include the impact of milestone 1 in their assessment of the impact of milestone 2.
To help ensure that reviewers are aligned with program managers in how they interpret the proposal milestones (if they aren’t directly involved in defining milestones), we suggest that either reviewers be informed of how program managers are categorizing the proposal outputs so they can conduct their review accordingly or allow reviewers to decide the category (and thus how the total expected utility is calculated), whether individually or collectively or both.
We chose to use only two of the goals that proposal authors provided because we wanted to standardize the number of milestones across proposals. However, this may have provided an incomplete picture of the proposals’ goals, and thus an incomplete assessment of the proposals. We recommend that future implementations be flexible and allow the number of milestones to be determined based on each proposal’s needs. This would also help accommodate one of the reviewers’ suggestion that some milestones should be broken down into intermediary steps.
Importance of Reviewer Explanations
As one can tell from the above discussion, reviewers’ explanation of their forecasts were crucial to understanding how they interpreted the milestones. Reviewers’ explanations varied in length and detail, but the most insightful responses broke down their reasoning into detailed steps and addressed (1) ambiguities in the milestone and how they chose to interpret ambiguities if they existed, (2) the state of the scientific field and the maturity of different techniques that the authors propose to use, and (3) factors that improve the likelihood of success versus potential barriers or challenges that would need to be overcome.
Exponential Impact Scales Better Reflect the Real Distribution of Impact
The distribution of NIH and NSF proposal peer review scores tends to be skewed such that most proposals are rated above the center of the scale and there are few proposals rated poorly. However, other markers of scientific impact, such as citations (even with all of its imperfections), tend to suggest a long tail of studies with high impact. This discrepancy suggests that traditional peer review scoring systems are not well-structured to capture the nonlinearity of scientific impact, resulting in score inflation. The aggregation of scores at the top end of the scale also means that very negative scores have a greater impact than very positive scores when averaged together, since there’s more room between the average score and the bottom end of the scale. This can generate systemic bias against more controversial or risky proposals.
In our pilot, we chose to use an exponential scale with a base of 2 for impact to better reflect the real distribution of scientific impact. Using this exponential impact scale, we conducted a survey of a small pool of academics in the life sciences about how they would rate the impact of the average funded NIH R01 grant. They responded with an average scientific impact score 5 and an average social impact score of 3, which are much lower on our scale compared to traditional peer review scores4, suggesting that the exponential scale may be beneficial for avoiding score inflation and bunching at the top. In our pilot, the distribution of scientific impact scores was centered higher than 5, but still less skewed than NIH peer review scores for significance and innovation typically are. This partially reflects the fact that proposals were expected to be funded at one to two orders of magnitude more than NIH R01 proposals are, so impact should also be greater. The distribution of social impact scores exhibits a much wider spread and lower center.
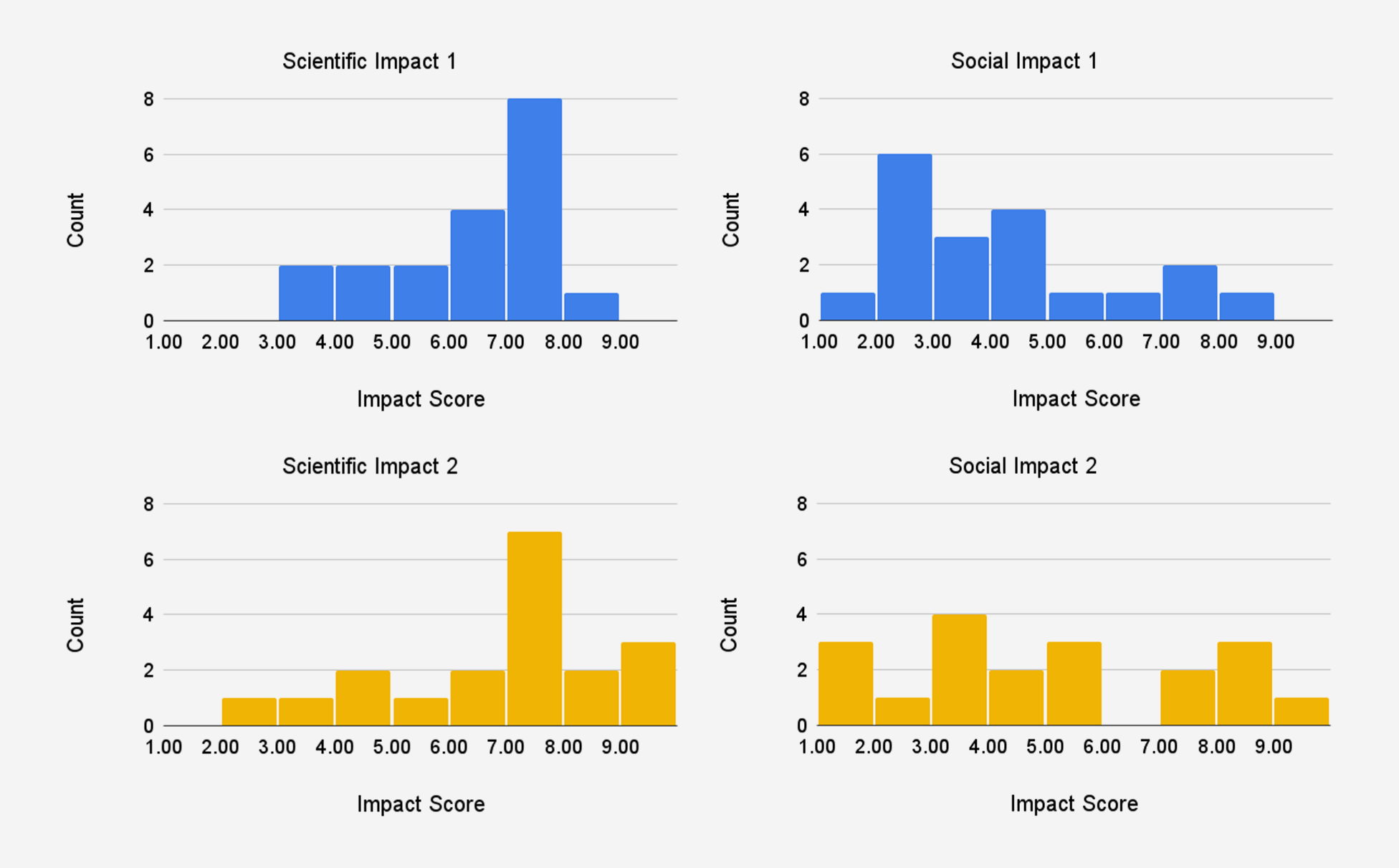
Conclusion
In summary, expected utility forecasting presents a promising approach to improving the rigor of peer review and quantitatively defining the risk-reward profile of science proposals. Our pilot study suggests that this approach can be quite user-friendly for reviewers, despite its apparent complexity. Further study into how best to integrate forecasting into panel environments, define proposal milestones, and calibrate impact scales will help refine future implementations of this approach.
More broadly, we hope that this pilot will encourage more grantmaking institutions to experiment with innovative funding mechanisms. Reviewers in our pilot were more open-minded and quick-to-learn than one might expect and saw significant value in this unconventional approach. Perhaps this should not be so much of a surprise given that experimentation is at the heart of scientific research.
Interested grantmakers, both public and private, and policymakers are welcome to reach out to our team if interested in learning more or receiving assistance in implementing this approach.
Acknowledgements
Many thanks to Jordan Dworkin for being an incredible thought partner in designing the pilot and providing meticulous feedback on this report. Your efforts made this project possible!
Appendix A: Pilot Study Design
Our pilot study consisted of five proposals for life science-related Focused Research Organizations (FROs). These proposals were solicited from academic researchers by FAS as part of our advocacy for the concept of FROs. As such, these proposals were not originally intended as proposals for direct funding, and did not have as strict content requirements as traditional grant proposals typically do. Researchers were asked to submit one to two page proposals discussing (1) their research concept, (2) the motivation and its expected social and scientific impact, and (3) the rationale for why this research can not be accomplished through traditional funding channels and thus requires a FRO to be funded.
Permission was obtained from proposal authors to use their proposals in this study. We worked with proposal authors to define two milestones for each proposal that reviewers would assess: one that they felt confident that they could achieve and one that was more ambitious but that they still thought was feasible. In addition, due to the brevity of the proposals, we included an additional 1-2 pages of supplementary information and scientific context. Final drafts of the milestones and supplementary information were provided to authors to edit and approve. Because this pilot study could not provide any actual funding to proposal authors, it was not possible to solicit full length research proposals from proposal authors.
We recruited four to six reviewers for each proposal based on their subject matter expertise. Potential participants were recruited over email with a request to help review a FRO proposal related to their area of research. They were informed that the review process would be unconventional but were not informed of the study’s purpose. Participants were offered a small monetary compensation for their time.
Confirmed participants were sent instructions and materials for the review process on the same day and were asked to complete their review by the same deadline a month and a half later. Reviewers were told to assume that, if funded, each proposal would receive $50 million in funding over five years to conduct the research, consistent with the proposed model for FROs. Each proposal had two technical milestones, and reviewers were asked to answer the following questions for each milestone:
- Assuming that the proposal is funded by 2025, will the milestone be achieved before 2031?
- What will be the average scientific impact score, as judged in 2032, of accomplishing the milestone?
- What will be the average social impact score, as judged in 2032, of accomplishing the milestone?
The impact scoring system was explained to reviewers as follows:
Please consider the following in determining the impact score: the current and expected long-term social or scientific impact of a funded FRO’s outputs if a funded FRO accomplishes this milestone before 2030.
The impact score we are using ranges from 1 (low) to 10 (high). It is base 2 exponential, meaning that a proposal that receives a score of 5 has double the impact of a proposal that receives a score of 4, and quadruple the impact of a proposal that receives a score of 3. In a small survey we conducted of SMEs in the life sciences, they rated the scientific and social impact of the average NIH R01 grant — a federally funded research grant that provides $1-2 million for a 3-5 year endeavor — on this scale to be 5.2 ± 1.5 and 3.1 ± 1.3, respectively. The median scores were 4.75 and 3.00, respectively.
Below is an example of how a predicted impact score distribution (left) would translate into an actual impact distribution (right). You can try it out yourself with this interactive version (in the menu bar, click Runtime > Run all) to get some further intuition on how the impact score works. Please note that this is meant solely for instructive purposes, and the interface is not designed to match Metaculus’ interface.
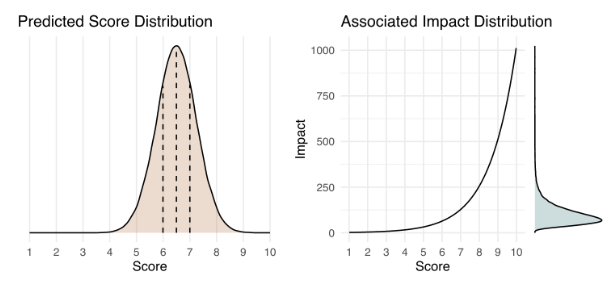
The choice of an exponential impact scale reflects the tendency in science for a small number of research projects to have an outsized impact. For example, studies have shown that the relationship between the number of citations for a journal article and its percentile rank scales exponentially.
Scientific impact aims to capture the extent to which a project advances the frontiers of knowledge, enables new discoveries or innovations, or enhances scientific capabilities or methods. Though each is imperfect, one could consider citations of papers, patents on tools or methods, or users of software or datasets as proxies of scientific impact.
Social impact aims to capture the extent to which a project contributes to solving important societal problems, improving well-being, or advancing social goals. Some proxy metrics that one might use to assess a project’s social impact are the value of lives saved, the cost of illness prevented, the number of job-years of employment generated, economic output in terms of GDP, or the social return on investment.
You may consider any or none of these proxy metrics as a part of your assessment of the impact of a FRO accomplishing this milestone.
Reviewers were asked to submit their forecasts on Metaculus’ website and to provide their reasoning in a separate Google form. For question 1, reviewers were asked to respond with a single probability. For questions 2 and 3, reviewers were asked to provide their median, 25th percentile, and 75th percentile predictions, in order to generate a probability distribution. Metaculus’ website also included information on the resolution criteria of each question, which provided guidance to reviewers on how to answer the question. Individual reviewers were blind to other reviewers’ responses until after the submission deadline, at which point the aggregated results of all of the responses were made public on Metaculus’ website.
Additionally, in the Google form, reviewers were asked to answer a survey question about their experience: “What did you think about this review process? Did it prompt you to think about the proposal in a different way than when you normally review proposals? If so, how? What did you like about it? What did you not like? What would you change about it if you could?”
Some participants did not complete their review. We received 19 complete reviews in the end, with each proposal receiving three to six reviews.
Study Limitations
Our pilot study had certain limitations that should be noted. Since FAS is not a grantmaking institution, we could not completely reproduce the same types of research proposals that a grantmaking institution would receive nor the entire review process. We will highlight these differences in comparison to federal science agencies, which are our primary focus.
- Review Process: There are typically two phases to peer review at NIH and NSF. First, at least three individual reviewers with relevant subject matter expertise are assigned to read and evaluate a proposal independently. Then, a larger committee of experts is convened. There, the assigned reviewers present the proposal and their evaluation, and then the committee discusses and determines the final score for the proposal. Our pilot study only attempted to replicate the first phase of individual review.
- Sample Size: In our pilot, the sample size was quite small, since only five proposals were reviewed, and they were all in different subfields, so different reviewers were assigned to each proposal. NIH and NSF peer review committees typically focus on one subfield and review on the order of twenty or so proposals. The number of reviewers per proposal–three to six–in our pilot was consistent with the number of reviewers typically assigned to a proposal by NIH and NSF. Peer review committees are typically larger, ranging from six to twenty people, depending on the agency and the field.
- Proposals: The FRO proposals plus supplementary information were only two to four pages long, which is significantly shorter than the 12 to 15 page proposals that researchers submit for NIH and NSF grants. Proposal authors were asked to generally describe their research concept, but were not explicitly required to describe the details of the research methodology they would use or any preliminary research. Some proposal authors volunteered more information on this for the supplementary information, but not all authors did.
- Grant Size: For the FRO proposals, reviewers were asked to assume that funded proposals would receive $50 million over five years, which is one to two orders of magnitude more funding than typical NIH and NSF proposals.
Appendix B: Feedback on Study-Specific Implementation
In addition to feedback about the review framework, we received feedback on how we implemented our pilot study, specifically the instructions and materials for the review process and the submission platforms. This feedback isn’t central to this paper’s investigation of expected value forecasting, but we wanted to include it in the appendix for transparency.
Reviewers were sent instructions over email that outlined the review process and linked to Metaculus’ webpage for this pilot. On Metaculus’ website, reviewers could find links to the proposals on FAS’ website and the supplementary information in Google docs. Reviewers were expected to read those first and then read through the resolution criteria for each forecasting question before submitting their answers on Metaculus’ platform. Reviewers were asked to submit the explanations behind their forecasts in a separate Google form.
Some reviewers had no problem navigating the review process and found Metaculus’ website easy to use. However, feedback from other reviewers suggested that the different components necessary for the review were spread out over too many different websites, making it difficult for reviewers to keep track of where to find everything they needed.
Some had trouble locating the different materials and pieces of information needed to conduct the review on Metaculus’ website. Others found it confusing to have to submit their forecasts and explanations in two separate places. One reviewer suggested that the explanation of the impact scoring system should have been included within the instructions sent over email rather than in the resolution criteria on Metaculus’ website so that they could have read it before reading the proposal. Another reviewer suggested that it would have been simpler to submit their forecasts through the same Google form that they used to submit their explanations rather than through Metaculus’ website.
Based on this feedback, we would recommend that future implementations streamline their submission process to a single platform and provide a more extensive set of instructions rather than seeding information across different steps of the review process. Training sessions, which science funding agencies typically conduct, would be a good supplement to written instructions.
Appendix C: Total Expected Utility Calculations
To calculate the total expected utility, we first converted all of the impact scores into utility by taking two to the exponential of the impact score, since the impact scoring system is base 2 exponential:
Utility=2Impact Score.
We then were able to average the utilities for each milestone and conduct additional calculations.
To calculate the total utility of each milestone, ui, we averaged the social utility and the scientific utility of the milestone:
ui = (Social Utility + Scientific Utility)/2.
The total expected utility (TEU) of a proposal with two milestones can be calculated according to the general equation:
TEU = u1P(m1 ∩ not m2) + u2P(m2 ∩ not m1) + (u1+u2)P(m1m2),
where P(mi) represents the probability of success of milestone i and
P(m1 ∩ not m2) = P(m1) – P(m1 ∩ m2)
P(m2 ∩ not m1) = P(m2) – P(m1 ∩ m2).
For sequential milestones, milestone 2 is defined as inclusive of milestone 1 and wholly dependent on the success of milestone 1, so this means that
u2, seq = u1+u2
P(m2) = Pseq(m1 ∩ m2)
P(m2 ∩ not m1) = 0.
Thus, the total expected utility of sequential milestones can be simplified as
TEU = u1P(m1)-u1P(m2) + (u2, seq)P(m2)
TEU = u1P(m1) + (u2, seq-u1)P(m2)
This can be generalized to
TEUseq = Σi(ui, seq-ui-1, seq)P(mi).
Otherwise, the total expected utility can be simplified to
TEU = u1P(m1) + u2P(m2) – (u1+u2)P(m1 ∩ m2).
For independent outcomes, we assume
Pind(m1 ∩ m2) = P(m1)P(m2),
so
TEUind = u1P(m1) + u2P(m2) – (u1+u2)P(m1)P(m2).
To present the results in Tables 1 and 2, we converted all of the utility values back into the impact score scale by taking the log base 2 of the results.
Working with academics: A primer for U.S. government agencies
Collaboration between federal agencies and academic researchers is an important tool for public policy. By facilitating the exchange of knowledge, ideas, and talent, these partnerships can help address pressing societal challenges. But because it is rarely in either party’s job description to conduct outreach and build relationships with the other, many important dynamics are often hidden from view. This primer provides an initial set of questions and topics for agencies to consider when exploring academic partnership.
Why should agencies consider working with academics?
- Accessing the frontier of knowledge: Academics are at the forefront of their fields, and their insights can provide fresh perspectives on agency work.
- Leveraging innovative methods: From data collection to analysis, academics may have access to the new technologies and approaches that can enhance governmental efforts.
- Enhancing credibility: By incorporating research and external expertise, policy decisions gain legitimacy and trust, and align with evidence-based policy guidelines.
- Generating new insights: Collaboration between agencies and outside researchers can lead to discoveries that advance both knowledge and practice..
- Developing human capital: Collaboration can enhance the skills of both public servants and academics, creating a more robust workforce and potentially leading to longer-term talent exchange.
What considerations may arise when working with academics?
- Designing collaborative relationships that are targeted to the incentives of both the agency and the academic partners;
- Navigating different rules and regulations that may impact academic-government collaboration, e.g. rules on external advisory groups, university guidelines, and data/information confidentiality;
- Understanding the different structures and mechanisms that enable academic-government collaboration, such as sabbaticals, fellowships, consultancies, grants, or contracts;
- Identifying and approaching the right academics for different projects and needs.
Academic faculty progress through different stages of professorship — typically assistant, associate, and full — that affect their research and teaching expectations and opportunities. Assistant professors are tenure-track faculty who need to secure funding, publish papers, and meet the standards for tenure. Associate professors have job security and academic freedom, but also more mentoring and leadership responsibilities; associate professors are typically tenured, though this is not always the case. Full professors are senior faculty who have a high reputation and recognition in their field, but also more demands for service and supervision. The nature of agency-academic collaboration may depend on the seniority of the academic. For example, junior faculty may be more available to work with agencies, but primarily in contexts that will lead to traditional academic outputs; while senior faculty may be more selective, but their academic freedom will allow for less formal and more impact-oriented work.
Soft money positions are those that depend largely or entirely on external funding sources, typically research grants, to support the salary and expenses of the faculty. Hard money positions are those that are supported by the academic institution’s central funds, typically tied to more explicit (and more expansive) expectations for teaching and service than soft-money positions. Faculty in soft money positions may face more pressure to secure funding for research, while faculty in hard money positions may have more autonomy in their research agenda but more competing academic activities. Federal agencies should be aware of the funding situation of the academic faculty they collaborate with, as it may affect their incentives and expectations for agency engagement.
A sabbatical is a period of leave from regular academic duties, usually for one or two semesters, that allows faculty to pursue an intensive and unstructured scope of work — this can include research in their own field or others, as well as external engagements or tours of service with non-academic institutions . Faculty accrue sabbatical credits based on their length and type of service at the university, and may apply for a sabbatical once they have enough credits. The amount of salary received during a sabbatical depends on the number of credits and the duration of the leave. Federal agencies may benefit from collaborating with academic faculty who are on sabbatical, as they may have more time and interest to devote to impact-focused work.
Consulting limits & outside activity limits are policies that regulate the amount of time that academic faculty can spend on professional activities outside their university employment. These policies are intended to prevent conflicts of commitment or interest that may interfere with the faculty’s primary obligations to the university, such as teaching, research, and service, and the specific limits vary by university. Federal agencies may need to consider these limits when engaging academic faculty in ongoing or high-commitment collaborations.
Some academic faculty are paid on a 9-month basis, meaning that they receive their annual salary over nine months and have the option to supplement their income with external funding or other activities during the summer months. Other faculty are paid on a 12-month basis, meaning that they receive their annual salary over twelve months and have less flexibility to pursue outside opportunities. Federal agencies may need to consider the salary structure of the academic faculty they work with, as it may affect their availability to engage on projects and the optimal timing with which they can do so.
Informal advising
Advisory relationships consist of an academic providing occasional or periodic guidance to a federal agency on a specific topic or issue, without being formally contracted or compensated. This type of collaboration can be useful for agencies that need access to cutting-edge expertise or perspectives, but do not have a formal deliverable in mind.
Academic considerations
- Career stage: Informal advising can be done by faculty at any level of seniority, as long as they have relevant knowledge and experience. However, junior faculty may be more cautious about engaging in informal advising, as it may not count towards their tenure or promotion criteria. Senior faculty, who have established expertise and secured tenure, may be more willing to engage in impact-focused advisory relationships.
- Incentives: Advisory relationships can offer some benefits for faculty regardless of career stage, such as expanding their network, increasing their visibility, and influencing policy or practice. Informal advising can also stimulate new research questions, and create opportunities for future access to data or resources. Some agencies may also acknowledge the contributions of academic advisors in their reports or publications, which may enhance researchers’ academic reputation.
- Conflicts of interest: Informal advising may pose potential conflicts of interest or commitment for faculty, especially if they have other sources of funding or collaboration related to the same topic or issue. Faculty may need to consult with their department chair or dean before engaging in formal conversations, and should also avoid any activities that may compromise their objectivity, integrity, or judgment in conducting or reporting their university research.
- Timing: Faculty on 9-month salaries might be more willing/able to engage during summer months, when they have minimal teaching requirements and are focused on research and impact outputs.
Regulatory & structural considerations
- Contracting: An advisory relationship may not require a formal agreement or contract between the agency and the academic. For some topics or agencies, however, it may require a non-disclosure agreement or consulting agreement if the agency wants to ensure the exclusivity or confidentiality of the conversation.
- Advisory committee rules: Depending on the scope and scale of the academic engagement, agencies should be sure to abide by Federal Advisory Committee Act regulations. With informal one-on-one conversations that are focused on education and knowledge exchange, this is unlikely to be an issue.
- University approval: An NDA or consulting agreement may require approval from the university’s office of sponsored programs or office of technology transfer before engaging in informal advising. These offices may review and approve the agreement between the agency and the academic institution, ensuring compliance with university policies and regulations.
- Compensation: Informal advising typically does not involve compensation for the academic, but it may involve reimbursement for travel or other expenses related to the advisory role. This work is unlikely to count towards the consulting limit for faculty, but it may count towards the outside professional activity limit, depending on the nature and frequency of the advising.
Federal agencies and academic institutions are subject to various laws and regulations that affect their research collaboration, and the ownership and use of the research outputs. Key legislation includes the Federal Advisory Committee Act (FACA), which governs advisory committees and ensures transparency and accountability; the Federal Acquisition Regulation (FAR), which controls the acquisition of supplies and services with appropriated funds; and the Federal Grant and Cooperative Agreement Act (FGCAA), which provides criteria for distinguishing between grants, cooperative agreements, and contracts. Agencies should ensure that collaborations are structured in accordance with these and other laws.
Federal agencies may use various contracting mechanisms to engage researchers from non-federal entities in collaborative roles. These mechanisms include the IPA Mobility Program, which allows the temporary assignment of personnel between federal and non-federal organizations; the Experts & Consultants authority, which allows the appointment of qualified experts and consultants to positions that require only intermittent and/or temporary employment; and Cooperative Research and Development Agreements (CRADAs), which allow agencies to enter into collaborative agreements with non-federal partners to conduct research and development projects of mutual interest.
Offices of Sponsored Programs are units within universities that provide administrative support and oversight for externally funded research projects. OSPs are responsible for reviewing and approving proposals, negotiating and accepting awards, ensuring compliance with sponsor and university policies and regulations, and managing post-award activities such as reporting, invoicing, and auditing. Federal agencies typically interact with OSPs as the authorized representative of the university in matters related to sponsored research.
When engaging with academics, federal agencies may use NDAs to safeguard sensitive information. Agencies each have their own rules and procedures for using and enforcing NDAs involving their grantees and contractors. These rules and procedures vary, but generally require researchers to sign an NDA outlining rights and obligations relating to classified information, data, and research findings shared during collaborations.
Study groups
A study group is a type of collaboration where an academic participates in a group of experts convened by a federal agency to conduct analysis or education on a specific topic or issue. The study group may produce a report or hold meetings to present their findings to the agency or other stakeholders. This type of collaboration can be useful for agencies that need to gather evidence or insights from multiple sources and disciplines with expertise relevant to their work.
Academic considerations
- Career stage: Faculty at any level of seniority can participate in a study group, but junior faculty may be more selective about joining, as they have limited time and resources to devote to activities that may not count towards their tenure or promotion criteria. Senior faculty may be more willing to join a study group, as they have more established expertise and reputation, and may seek to have more impact on policy or practice.
- Soft vs. hard money: Faculty in soft money positions, where their salary and research expenses depend largely on external funding sources, may be more interested in joining a study group if it provides funding or other resources that support their research. Faculty in hard money positions, where their salary and research expenses are supported by institutional funds, may be less motivated by funding, but more by the recognition and impact that comes from participating.
- Incentives: Study groups can offer some benefits for faculty, such as expanding their network, increasing their visibility, and influencing policy or practice. Study groups can also stimulate new research ideas or questions for faculty, and create opportunities for future access to data or resources. Some study groups may also result in publication of output or other forms of recognition (e.g., speaking engagements) that may enhance the academic reputation of the faculty.
- Conflicts of interest: Study groups may pose potential conflicts of interest or commitment for academics, especially if they have other sources of funding related to the same topic. Faculty may also be cautious about entering into more formal agreements if it may impact their ability to apply for & receive federal research funding in the future. Agencies should be aware of any such impacts of academic participation, and faculty should be encouraged to consult with their department chair or dean before joining a study group.
Regulatory & structural considerations
- Contracting and compensation: The optimal contracting mechanism for a study group will depend on the agency, the topic, and the planned output of the group. Some possible contracting mechanisms are extramural grants, service contracts, cooperative agreements, or memoranda of understanding. The mechanism will determine the amount and type of compensation that participants (or the organizing body) receive, and could include salary support, travel reimbursement, honoraria, or overhead costs.
- Advisory committee rules: When setting up study groups, agencies should work carefully to ensure that the structure abides by Federal Advisory Committee Act regulations. To ensure that study groups are distinct from Advisory Committees , these groups should be limited in size, and should be tasked with providing knowledge, research, and education — rather than specific programmatic guidance — to agency partners.
- University approval: Depending on the contracting mechanism and the compensation involved, academic participants may need to obtain approval from their university’s office of sponsored programs or office of technology transfer before joining a study group. These offices may review the terms and conditions of the agreement between the agency and the academic institution, such as the scope of work, the budget, and the reporting requirements.
Case study
In 2022, the National Science Foundation (NSF) awarded the National Bureau of Economic Research (NBER) a grant to create the EAGER: Place-Based Innovation Policy Study Group. This group, led by two economists with expertise in entrepreneurship, innovation, and regional development — Jorge Guzman from Columbia University and Scott Stern from MIT — aimed to provide “timely insight for the NSF Regional Innovation Engines program.” During Fall 2022, the group met regularly with NSF staff to i) provide an assessment of the “state of knowledge” of place-based innovation ecosystems, ii) identify the insights of this research to inform NSF staff on design of their policies, and iii) surface potential means by which to measure and evaluate place-based innovation ecosystems on a rigorous and ongoing basis. Several of the academic leads then completed a paper synthesizing the opportunities and design considerations of the regional innovation engine model, based on the collaborative exploration and insights developed throughout the year. In this case, the study group was structured as a grant, with funding provided to the organizing institution (NBER) for personnel and convening costs. Yet other approaches are possible; for example, NSF recently launched a broader study group with the Institute for Progress, which is structured as a no-cost Other Transaction Authority contract.
Collaborative research
Active collaboration covers scenarios in which an academic engages in joint research with a federal agency, either as a co-investigator, a subrecipient, a contractor, or a consultant. This type of collaboration can be useful for agencies that need to leverage the expertise, facilities, data, or networks of academics to conduct research that advances their mission, goals, or priorities.
Academic considerations
- Career stage: Collaborative research is likely to be attractive to junior faculty, who are seeking opportunities to access data that might not be otherwise available, and to foster new relationships with partners. This is particularly true if there is a commitment that findings or evaluations will be publishable, and if the collaboration does not interfere with teaching and service obligations. Collaborative projects are also likely to be of interest to senior faculty — if work aligns with their established research agenda — and publication of findings may be (slightly) less of a requirement.
- Soft vs. hard money: Researchers on hard money contracts, where their salary and research expenses are supported by institutional funds, may be more motivated by the opportunity to use and publish internal data from the agency. Researchers on soft money contracts, where their salary and research expenses depend largely on external funding sources, may be more motivated by the availability of grants and financial support from the agency.
- Timing: Depending on the scope of the collaboration, and the availability of funding for the researcher, efforts could be targeted for academics’ summer months or their sabbaticals. Alternatively, collaborative research could be integrated into the regular academic year, as part of the researcher’s ongoing research activities.
- Incentives: As mentioned above, collaborative research can offer some benefits for faculty, such as access to data and information, publication opportunities, funding sources, and partnership networks. Collaborative research can also provide faculty with more direct and immediate impact on policy or practice, as well as recognition from the agency and stakeholders (and, perhaps to a lesser extent, the academic community).
Regulatory & structural considerations
- Contracting: The contracting requirements for collaborative research will vary greatly depending on the structure and scope of the collaboration, the partnering agency, and the use of internal government data or resources. Readers are encouraged to explore agency-specific guidance when considering the ideal mechanism for a given project. Some possible contracting mechanisms are extramural grants, service contracts, or cooperative research and development agreements. Each mechanism has different terms and conditions regarding the scope of work, the budget, the intellectual property rights, the reporting requirements, and the oversight responsibilities.
- Regulatory compliance: Collaborative research involving both governmental and non-governmental partners will require compliance with various laws, regulations, and authorities. These include but are not limited to:
- Federal Acquisition Regulation (FAR), which establishes the policies and procedures for acquiring supplies and services with appropriated funds;
- Federal Grant and Cooperative Agreement Act (FGCAA), which provides criteria for determining whether to use a grant or a cooperative agreement to provide assistance to non-federal entities;
- Other Transaction Authority (OTA), a contracting mechanism that provides (most) agencies with the ability to enter into flexible research & development agreements that are not subject to the regulations on standard contracts, grants, or cooperative agreements
- OMB’s Uniform Guidance, which set forth the administrative requirements, cost principles, and audit requirements for federal awards
- Bayh-Dole Act, which allows academic institutions to retain title to inventions made with federal funding, subject to certain conditions and obligations.
- Collaborative research may also require compliance with ethical standards and guidelines for human subjects research, such as the Belmont Report and the Common Rule.
Case studies
External collaboration between academic researchers and government agencies has repeatedly proven fruitful for both parties. For example, in May 2020, the Rhode Island Department of Health partnered with researchers at Brown University’s Policy Lab to conduct a randomized controlled trial evaluating the effectiveness of different letter designs in encouraging COVID-19 testing. This study identified design principles that improved uptake of testing by 25–60% without increasing cost, and led to follow-on collaborations between the institutions. The North Carolina Office of Strategic Partnerships provides a prime example of how government agencies can take steps to facilitate these collaborations. The office recently launched the North Carolina Project Portal, which serves as a platform for the agency to share their research needs, and for external partners — including academics — to express interest in collaborating. Researchers are encouraged to contact the relevant project leads, who then assess interested parties on their expertise and capacity, extend an offer for a formal research partnership, and initiate the project.
Short-term placements
Short-term placements allow for an academic researcher to work at a federal agency for a limited period of time (typically one year or less), either as a fellow, a scholar, a detailee, or a special government employee. This type of collaboration can be useful for agencies that need to fill temporary gaps in expertise, capacity, or leadership, or to foster cross-sector exchange and learning.
Academic considerations
- Career stage: Short-term placements may be more appealing to senior faculty, who have more established and impact-focused research agendas, and who may seek to influence policy or practice at the highest levels. Junior faculty may be less interested in placements, particularly if they are still progressing towards tenure — unless the position offers opportunities for publication, funding, or recognition that are relevant to their tenure or promotion criteria.
- Soft vs. hard money: Faculty in soft money positions may face more challenges in arranging short-term placement if they have ongoing grants or labs to maintain; but placements where external resources are available (e.g., established fellowships), could be an attractive option when ongoing commitments are manageable. The impact of hard money will depend largely on the type of placement and the expectations for whether institutional support or external resources will cover a faculty member’s time away from the university.
- Timing: Sabbaticals are an ideal time for short-term placements, as they allow faculty to pursue intensive research or external engagement, without interfering with their regular academic duties. However, convincing faculty to use their sabbaticals for short-term placement may require a longer discovery and recruitment period, as well as a strong value proposition that highlights the benefits and incentives of the collaboration. Because most faculty are subject to the academic calendar, June and January tend to be ideal start dates for this type of engagement.
- Incentives: Short-term placements can offer benefits for academics, such as having an impact on policy or practice, gaining access to new data or research areas, and building relationships with agency officials and other stakeholders. However, short-term placements can also involve some costs and/or risks for participating faculty, including logistical complications, relocation, confidentiality constraints, and publication restrictions.
Regulatory & structural considerations
- Contracting: Short-term placements require a formal agreement or contract between the agency and the academic. There are several contracting & hiring mechanisms that can facilitate short-term placement, such as the Intergovernmental Personnel Act (IPA) Mobility Program, the Experts & Consultants authority, Schedule A(r), or the Special Government Employee (SGE) designation. Each mechanism has different eligibility criteria, terms and conditions, and administrative processes. Alternatively, many fellowship programs already exist within agencies or through outside organizations, which can streamline the process and handle logistics on behalf of both the academic institution and the agency.
- Compensation: The payment of salary support, travel, overhead, etc. will depend on the contracting mechanism and the agreement between the agency and the academic institution. Costs are generally covered by the organization that is expected to benefit most from the placement, which is often the agency itself; though some authorities for facilitating cross-sector exchange (e.g., the IPA program and Experts and Consultants authority) allow research institutions to cost-share or cover the expense of an expert’s compensation when appropriate. External fellowship programs also occasionally provide external resources to cover costs.
- Role and expectations: Placements, more so than more informal collaborations, require clear communication and understanding of the role and expectations. The academic should also be prepared to adapt to the agency’s norms and processes, which will differ from those in academia, and to perform work that may not reflect their typical contribution. The academic should also be aware of their rights and obligations as a federal employee or contractor.
- Confidentiality: Placements may involve access to confidential or sensitive information from the agency, such as classified data or personal information. Academics will likely be required to sign a non-disclosure agreement (NDA) that defines the scope and terms of confidentiality, and will often be subject to security clearance or background check procedures before entering their role.
Case studies
Various programs exist throughout government to facilitate short-term rotations of outside experts into federal agencies and offices. One of the most well-known examples is the American Association for the Advancement of Science (AAAS) Science & Technology Policy Fellowship (STPF) program, which places scientists and engineers from various disciplines and career stages in federal agencies for one year to apply their scientific knowledge and skills to inform policy making and implementation. The Schedule A(r) hiring authority tends to be well-suited for these kinds of fellowships; it is used, for example, by the Bureau of Economic Analysis to bring on early career fellows through the American Economic Association’s Summer Economics Fellows Program. In some circumstances, outside experts are brought into government “on loan” from their home institution to do a tour of service in a federal office or agency; in these cases, the IPA program can be a useful mechanism. IPAs are used by the National Science Foundation (NSF) in its Rotator Program, which brings outside scientists into the agency to serve as temporary Program Directors and bring cutting-edge knowledge to the agency’s grantmaking and priority-setting. IPA is also used for more ad-hoc talent needs; for example, the Office of Evaluation Sciences (OES) at GSA often uses it to bring in fellows and academic affiliates.
Long-term rotations
Long-term rotations allow an academic to work at a federal agency for an extended period of time (more than one year), either as a fellow, a scholar, a detailee, or a special government employee. This type of collaboration can be useful for agencies that need to recruit and retain expertise, capacity, or leadership in areas that are critical to their mission, goals, or priorities.
Academic considerations
- Career stage: Long-term rotations may be more feasible for senior faculty, who have more experience in their discipline and are likely to have more flexibility and support from their institutions to take a leave of absence. Junior faculty may face more barriers and risks in pursuing long-term rotations, such as losing momentum in their research productivity, missing opportunities for tenure or promotion, or losing connection with their academic peers and mentors.
- Soft vs. hard money: Faculty in soft money positions may have more ability to seek longer-term rotations, as the provision of external support is more in line with their institutions’ expectations. Faculty in hard money positions may face difficulties seeking long-term rotations, as institutional provision of resources comes with expectations for teaching and service that administrations may be wary of pausing for extended periods of time.
- Timing: Long-term rotations require careful planning and coordination with the academic institution and the federal agency, as it may involve significant changes in the academic’s schedule, workload, and responsibilities. These rotations may be easier to arrange during sabbaticals or other periods of leave from the academic institution, but will often still require approval from the institution’s administration. Because most faculty are subject to the academic calendar, June and January tend to be ideal start dates for sabbatical or secondment engagements.
- Incentives: Long-term rotations offer an opportunity for faculty to gain valuable experience and insight into the impact frontier — both in terms of policy and practice — of their field or discipline. These experiences can yield new skills or competencies that enhance their academic performance or career advancement, can help academics build strong relationships and networks with agency officials and other stakeholders, and can provide a lasting impact on public good. However, long-term roles involve challenges for faculty, such as adjusting to a different organizational structure, balancing expectations from both the agency and the academy, and transitioning back into academic work and productivity following the rotation.
Regulatory & structural considerations
- Regulatory and structural considerations — including contracting, compensation, and expectations — are similar to those of short-term placements, and tend to involve the same mechanisms and processes.
- The desired length of a long-term rotation will affect how agencies select and apply the appropriate mechanism. For example, IPA assignments are initially made for up to two years, and can then be extended for another two years when relevant — yielding a maximum continuous term length of four years.
- Longer time frames typically require additional structural considerations. Specifically, extensions of mechanisms like the IPA may be required, or more formal governmental employment may be prioritized at the outset. Given that these types of placements are often bespoke, these considerations should be explored in depth for the agency’s specific needs and regulatory context.
Case study
One example of a long-term rotation that draws experts from academia into federal agency work is the Advanced Research Projects Agency (ARPA) Program Manager (PM) role. ARPA PMs — across DARPA, IARPA, ARPA-E, and now ARPA-H — are responsible for leading high-risk, high-reward research programs, and have considerable autonomy and authority in defining their research vision, selecting research performers, managing their research budget, and overseeing their research outcomes. PMs are typically recruited from academia, industry, or government for a term of three to five years, and are expected to return to their academic institutions or pursue other career opportunities after their term at the agency. PMs coming from academia or nonprofit organizations are often brought on through the IPA mobility program, and some entities also have unique term-limited, hiring authorities for this purpose. PMs can also be hired as full government employees; this mechanism is primarily used for candidates coming from the private sector.
Incorporate open science standards into the identification of evidence-based social programs
Evidence-based policy uses peer-reviewed research to identify programs that effectively address important societal issues. For example, several agencies in the federal government run clearinghouses that review and assess the quality of peer-reviewed research to identify programs with evidence of effectiveness. However, the replication crisis in the social and behavioral sciences raises concerns that research publications may contain an alarming rate of false positives (rather than true effects), in part due to selective reporting of positive results. The use of open and rigorous practices — like study registration and availability of replication code and data — can ensure that studies provide valid information to decision-makers, but these characteristics are not currently collected or incorporated into assessments of research evidence.
To rectify this issue, federal clearinghouses should incorporate open science practices into their standards and procedures used to identify evidence-based social programs eligible for federal funding.
Details
The federal government is increasingly prioritizing the curation and use of research evidence in making policy and supporting social programs. In this effort, federal evidence clearinghouses—influential repositories of evidence on the effectiveness of programs—are widely relied upon to assess whether policies and programs across various policy sectors are truly “evidence-based.” As one example, the Every Student Succeeds Act (ESSA) directs states, districts, and schools to implement programs with research evidence of effectiveness when using federal funds for K-12 public education; the What Works Clearinghouse—an initiative of the U.S. Department of Education—identifies programs that meet the evidence-based funding requirements of the ESSA. Similar mechanisms exist in the Departments of Health and Human Services (the Prevention Services Clearinghouse and the Pathways to Work Evidence Clearinghouse), Justice (CrimeSolutions), and Labor (the Clearinghouse for Labor and Evaluation Research). Consequently, clearinghouse ratings have the potential to influence the allocation of billions of dollars appropriated by the federal government for social programs.
Clearinghouses generally follow explicit standards and procedures to assess whether published studies used rigorous methods and reported positive results on outcomes of interest. Yet this approach rests on assumptions that peer-reviewed research is credible enough to inform important decisions about resource allocation and is reported accurately enough for clearinghouses to distinguish which reported results represent true effects likely to replicate at scale. Unfortunately, published research often contains results that are wrong, exaggerated, or not replicable. The social and behavioral sciences are experiencing a replication crisis as a result of numerous large-scale collaborative efforts that had difficulty replicating novel findings in published peer-reviewed research. This issue is partly attributed to closed scientific workflows, which hinder reviewers’ and evaluators’ attempts to detect issues that negatively impact the validity of reported research findings—such as undisclosed multiple hypothesis testing and the selective reporting of results.
Research transparency and openness can mitigate the risk of informing policy decisions on false positives. Open science practices like prospectively sharing protocols and analysis plans, or releasing code and data required to replicate key results, would allow independent third parties such as journals and clearinghouses to fully assess the credibility and replicability of research evidence. Such openness in the design, execution, and analysis of studies on program effectiveness is paramount to increasing public trust in the translation of peer-reviewed research into evidence-based policy.
Currently, standards and procedures to measure and encourage open workflows—and facilitate detection of detrimental practices in the research evidence—are not implemented by either clearinghouses or the peer-reviewed journals publishing the research on program effectiveness that clearinghouses review. When these practices are left unchecked, incomplete, misleading, or invalid research evidence may threaten the ability of evidence-based policy to live up to its promise of producing population-level impacts on important societal issues.
Recommendations
Policymakers should enable clearinghouses to incorporate open science into their standards and procedures used to identify evidence-based social programs eligible for federal funding, and increase the funds appropriated to clearinghouse budgets to allow them to take on this extra work. There are several barriers to clearinghouses incorporating open science into their standards and procedures. To address these barriers and facilitate implementation, we recommend that:
- Dedicated funding should be appropriated by Congress and allocated by federal agencies to clearinghouse budgets so they can better incorporate the assessment of open science practices into research evaluation.
- Funding should facilitate the hiring of additional personnel dedicated to collecting data on whether open science practices were used—and if so, whether they were used well enough to assess the comprehensive of reporting (e.g., checking publications on results with prospective protocols) and reproducibility of results (e.g., rerunning analyses using study data and code).
- The Office of Management and Budget should establish a formal mechanism for federal agencies that run clearinghouses to collaborate on shared standards and procedures for reviewing open science practices in program evaluations. For example, an interagency working group can develop and implement updated standards of evidence that include assessment of open science practices, in alignment with the Transparency and Openness Promotion (TOP) Guidelines for Clearinghouses.
- Once funding, standards, and procedures are in place, federal agencies sponsoring clearinghouses should create a roadmap for eventual requirements on open science practices in studies on program effectiveness.
- Other open science initiatives targeting researchers, research funders, and journals are increasing the prevalence of open science practices in newly published research. As open science practices become more common, agencies can introduce requirements on open science practices for evidence-based social programs, similar to research transparency requirements implemented by the Department of Health and Human Services for the marketing and reimbursement of medical interventions.
- For example, evidence-based funding mechanisms often have several tiers of evidence to distinguish the level of certainty that a study produced true results. Agencies with tiered-evidence funding mechanisms can begin by requiring open science practices in the highest tier, with the long-term goal of requiring a program meeting any tier to be based on open evidence.
Conclusion
The momentum from the White House’s 2022 Year of Evidence for Action and 2023 Year of Open Science provides an unmatched opportunity for connecting federal efforts to bolster the infrastructure for evidence-based decision-making with federal efforts to advance open research. Evidence of program effectiveness would be even more trustworthy if favorable results were found in multiple studies that were registered prospectively, reported comprehensively, and computationally reproducible using open data and code. With policymaker support, incorporating these open science practices in clearinghouse standards for identifying evidence-based social programs is an impactful way to connect these federal initiatives that can increase the trustworthiness of evidence used for policymaking.
Develop a Digital Technology Fund to secure and sustain open source software
Open source software (OSS) is a key part of essential digital infrastructure. Recent estimates indicate that 95% of all software relies upon open source, with about 75% of the code being directly open source. Additionally, as our science and technology ecosystem becomes more networked, computational, and interdisciplinary, open source software will increasingly be the foundation on which our discoveries and innovations rest.
However, there remain important security and sustainability issues with open source software, as evidenced by recent incidents such as the Log4j vulnerability that affected millions of systems worldwide.
To better address security and sustainability of open source software, the United States should establish a Digital Technology Fund through multi-stakeholder participation.
Details
Open source software — software whose source code is publicly available and can be modified, distributed, and reused by anyone — has become ubiquitous. OSS offers myriad benefits, including fostering collaboration, reducing costs, increasing efficiency, and enhancing interoperability. It also plays a key role in U.S. government priorities: federal agencies increasingly create and procure open source software by default, an acknowledgement of its technical benefits as well as its value to the public interest, national security, and global competitiveness.
Open source software’s centrality in the technology produced and consumed by the federal government, the university sector, and the private sector highlights the pressing need for these actors to coordinate on ensuring its sustainability and security. In addition to fostering more robust software development practices, raising capacity, and developing educational programs, there is an urgent need to invest in individuals who create and maintain critical open source software components, often without financial support.
The German Sovereign Tech Fund — launched in 2021 to support the development and maintenance of open digital infrastructure — recently announced such support for the maintainers of Log4j, thereby bolstering its prospects for timely, secure production and sustainability. Importantly, this is one example of numerous that require similar support. Cybersecurity and Infrastructure Security (CISA)’s director Jen Easterly has affirmed the importance of OSS while noting its security vulnerabilities as a national security concern. Easterly rightly called upon moving the responsibility and support for critical OSS components away from individuals to the organizations that benefit from those individuals’ efforts.
Recommendations
To address these challenges, the United States should establish a Digital Technology Fund to provide direct and indirect support to OSS projects and communities that are essential for the public interest, national security, and global competitiveness. The Digital Technology Fund would be funded by a coalition of federal, private, academic, and philanthropic stakeholders and would be administered by an independent nonprofit organization.
To better understand the risks and opportunities:
- The Office of the Cyber National Director should publish a synopsis of the feedback to the recent RFI regarding OSS security; it should then commission a comparative analysis of this synopsis and the German Tech Sovereign Fund to identify the gaps and needs within the U.S. context.
To encourage multi-stakeholder participation and support:
- The White House should task the Open-Source Software Security Initiative (OS3I) working group with developing a strategy, draft legislation, and funding proposal for the Digital Technology Fund. The fund should be established as a public-private partnership with a focus on the security and sustainability of OSS; it could be designed to augment the existing Open Technology Fund, which supports internet freedom and digital rights. The strategy should include approaches for encouraging contribution from the private sector, universities, and philanthropy, along with the federal government, to the fund’s resources and organization.
To launch the Digital Tech Fund:
- Congress should appropriate funding in alignment with the proposal developed by the OS3I working group. Legislation could provide relevant agencies — many of which have identified secure OSS as a priority — with initial implementation and oversight responsibility for the fund, after which point a permanent board could be selected.
The realized and potential impact of open source software is transformative in terms of next-generation infrastructure, innovation, workforce development, and artificial intelligence safety. The Digital Tech Fund can play an essential and powerful role in raising our collective capacity to address important security and sustainability challenges by acknowledging and supporting the pioneering individuals who are advancing open source software.
Advance open science through robust data privacy measures
In an era of accelerating advancements in data collection and analysis, realizing the full potential of open science hinges on balancing data accessibility and privacy. As we move towards a more open scientific environment, the volume of sensitive data being shared is swiftly increasing. While open science presents an opportunity to fast-track scientific discovery, it also poses a risk to privacy if not managed correctly.
Building on existing data and privacy efforts, the White House and federal science agencies should collaborate to develop and implement clear standards for research data privacy across the data management and sharing life cycle.
Details
Federal agencies’ open data initiatives are a milestone in the move towards open science. They have the potential to foster greater collaboration, transparency, and innovation in the U.S. scientific ecosystem and lead to a new era of discovery. However, a shift towards open data also poses challenges for privacy, as sharing research data openly can expose personal or sensitive information when done without the appropriate care, methods, and tools. Addressing this challenge requires new policies and technologies that allow for open data sharing while also protecting individual privacy.
The U.S. government has shown a strong commitment to addressing data privacy challenges in various scientific and technological contexts. This commitment is underpinned by laws and regulations such as the Health Insurance Portability and Accountability Act and the regulations for human subjects research (e.g., Code of Federal Regulations Title 45, Part 46). These regulations provide a legal framework for protecting sensitive and identifiable information, which is crucial in the context of open science.
The White House Office of Science and Technology Policy (OSTP) has spearheaded the “National Strategy to Advance Privacy-Preserving Data Sharing and Analytics,” aiming to further the development of these technologies to maximize their benefits equitably, promote trust, and mitigate risks. The National Institutes of Health (NIH) operate an internal Privacy Program, responsible for protecting sensitive and identifiable information within NIH work. The National Science Foundation (NSF) complements these efforts with a multidisciplinary approach through programs like the Secure and Trustworthy Cyberspace program, aiming to develop new ways to design, build, and operate cyber systems, protect existing infrastructure, and motivate and educate individuals about cybersecurity.
Given the unique challenges within the open science context and the wide reach of open data initiatives across the scientific ecosystem, there remains a need for further development of clear policies and frameworks that protect privacy while also facilitating the efficient sharing of scientific data. Coordinated efforts across the federal government could ensure these policies are adaptable, comprehensive, and aligned with the rapidly evolving landscape of scientific research and data technologies.
Recommendations
To clarify standards and best practices for research data privacy:
- The National Institute of Standards and Technology (NIST) should build on its existing Research Data Framework to develop a new framework that is specific to research data privacy and addresses the unique needs of open science communities and practices. This would provide researchers with a clear roadmap for implementing privacy-preserving data sharing in their work.
- This framework should incorporate the principles of Privacy by Design, ensuring that privacy is an integral part of the research life cycle, rather than an afterthought.
- The framework should be regularly updated to stay current with the changes in state, federal, and international data privacy laws, as well as new privacy-preserving methodologies. This will ensure that it remains relevant and effective in the evolving data privacy landscape.
To ensure best practices are used in federally funded research:
- Funding agencies like the NIH and NSF should work with NIST to develop and implement training for Data Management and Sharing Plan applicants and reviewers. This training would equip both parties with knowledge of best practices in privacy-preserving data sharing in open science, thereby ensuring that data privacy measures are effectively integrated into research workflows.
- Agencies should additionally establish programs to foster privacy education, as recommended in the OSTP national strategy.
- Training on open data privacy could additionally be incorporated into agencies’ existing Responsible Conduct of Research requirements.
To catalyze continued improvements in data privacy technologies:
- Science funding agencies should increase funding for domain-specific research and development of privacy-preserving methods for research data sharing. Such initiatives would spur innovation in fields like cryptography and secure computation, leading to the development of new technologies that can broaden the scope of open and secure data sharing.
- To further stimulate innovation, these agencies could also host privacy/security innovation competitions, encouraging researchers and developers to create and implement cutting-edge solutions.
To facilitate inter-agency coordination:
- OSTP should launch a National Science and Technology Council subcommittee on research data privacy within the Committee on Science. This subcommittee should work closely with the Office of Management and Budget, leveraging its expertise in overseeing federal information resources and implementing data management policies. This collaboration would ensure a coordinated and consistent approach to addressing data privacy issues in open science across different federal agencies.
Incorporate open source hardware into Patent and Trademark Office search locations for prior art
Increasingly, scientific innovations reside outside the realm of papers and patents. This is particularly true for open source hardware — hardware designs made freely and publicly available for study, modification, distribution, production, and sale. The shift toward open source aligns well with the White House’s 2023 Year of Open Science and can advance the accessibility and impact of federally funded hardware. Yet as the U.S. government expands its support for open science and open source, it will be increasingly vital that our intellectual property (IP) system is designed to properly identify and protect open innovations. Without consideration of open source hardware in prior art and attribution, these public goods are at risk of being patented over and having their accessibility lost.
Organizations like the Open Source Hardware Association (OSHWA) — a standards body for open hardware — provide verified databases of open source innovations. Over the past six years, for example, OSHWA’s certification program has grown to over 2600 certifications, and the organization has offered educational seminars and training. Despite the availability of such resources, open source certifications and resources have yet to be effectively incorporated into the IP system.
We recommend that the United States Patent and Trademark Office (USPTO) incorporate open source hardware certification databases into the library of resources to search for prior art, and create guidelines and training to build agency capacity for evaluating open source prior art.
Details
Innovative and important hardware products are increasingly being developed as open source, particularly in the sciences, as academic and government research moves toward greater transparency. This trend holds great promise for science and technology, as more people from more backgrounds are able to replicate, improve, and share hardware. A prime example is the 3D printing industry. Once foundational patents in 3D printing were released, there was an explosion of invention in the field that led to desktop and consumer 3D printers, open source filaments, and even 3D printing in space.
For these benefits to be more broadly realized across science and technology, open source hardware must be acknowledged in a way that ensures scientists will have their contributions found and respected by the IP system’s prior art process. Scientists building open source hardware are rightfully concerned their inventions will be patented over by someone else. Recently, a legal battle ensued from open hardware being wrongly patented over. While the patent was eventually overturned, it took time and money, and revealed important holes in the United States’ prior art system. As another example, the Electronic Frontier Foundation found 30+ pieces of prior art that the ArrivalStar patent was violating.
Erroneous patents can harm the validity of open source and limit the creation and use of new open source tools, especially in the case of hardware, which relies on prior art as its main protection. The USPTO — the administrator of intellectual property protection and a key actor in the U.S. science and technology enterprise — has an opportunity to ensure that open source tools are reliably identified and considered. Standardized and robust incorporation of open source innovations into the U.S. IP ecosystem would make science more reproducible and ensure that open science stays open, for the benefits of rapid improvement, testing, citizen science, and general education.
Recommendations
We recommend that the USPTO incorporate open source hardware into prior art searches and take steps to develop education and training to support the protection of open innovation in the patenting process.
- USPTO should add OSHWA’s certification – a known, compliant open source hardware certification program – to its non-patent search library.
- USPTO should put out a request for information (RFI) seeking input on (a) optimal approaches for incorporating open source innovations into searches for prior art, and (b) existing databases, standards, or certification programs that can/should be added to the agency’s non-patent search library.
- Based on the results of the RFI, USPTO’s Scientific and Technical Information Center should create guidelines and educational training programs to build examiners’ knowledge and capacity for evaluating open source prior art.
- USPTO should create clear public guidelines for the submission of new databases into the agency’s prior art library, and the requirements for their consideration and inclusion.
Incorporation of open hardware into prior art searches will signify the importance and consideration of open source within the IP system. These actions have the potential to improve the efficiency of prior art identification, advance open source hardware by assuring institutional actors that open innovations will be reliably identified and protected, and ensure open science stays open.
Improve research through better data management and sharing plans
The United States government spends billions of dollars every year to support the best scientific research in the world. The novel and multidisciplinary data produced by these investments have historically remained unavailable to the broader scientific community and the public. This limits researchers’ ability to synthesize knowledge, make new discoveries, and ensure the credibility of research. But recent guidance from the Office of Science and Technology Policy (OSTP) represents a major step forward for making scientific data more available, transparent, and reusable.
Federal agencies should take coordinated action to ensure that data sharing policies created in response to the 2022 Nelson memo incentivize high-quality data management and sharing plans (DMSPs), include robust enforcement mechanisms, and implement best practices in supporting a more innovative and credible research culture.
Details
The 2022 OSTP memorandum “Ensuring Free, Immediate, and Equitable Access to Federally Funded Research” (the Nelson memo) represents a significant step toward opening up not only the findings of science but its materials and processes as well. By including data and related research outputs as items that should be publicly accessible, defining “scientific data” to include “material… of sufficient quality to validate and replicate research findings” (emphasis added), and specifying that agency plans should cover “scientific data that are not associated with peer-reviewed scholarly publications,” this guidance has the potential to greatly improve the transparency, equity, rigor, and reusability of scientific research.
Yet while the 2022 Nelson memo provides a crucial foundation for open, transparent, and reusable scientific data, preliminary review of agency responses reveals considerable variation on how access to data and research outputs will be handled. Agencies vary by the degree to which policies will be reviewed and enforced and by the degree of specificity by which they define data as being materials needed to “validate and replicate” research findings. Finally, they could and should go further in including plans to fully support a research ecosystem that supports cumulative scientific evidence by enabling the accessibility, discoverability, and citation of researchers’ data sharing plans themselves.
Recommendations
To better incentivize quality and reusability in data sharing, agencies should:
- Make DMSPs publicly available in an easy-to-use interface on their websites where individual grants are listed to increase accountability for stated plans and discoverability of research outputs.
- Additionally, give DMSPs persistent, unique identifiers (e.g., digital object identifiers, or DOIs) so that they can be cited, read, and used.
- Make DMSPs subject to peer review as part of the same process that other aspects of a proposed research project’s intellectual merit are evaluated. This will directly incentivize high standards of planned data sharing practices and enable the diffusion of best practices across the research community.
To better ensure compliance and comprehensive availability, agencies should:
- Coordinate across agencies to create a consistent mechanism for DMSP enforcement to reduce applicant uncertainty about agencies’ expectations and processes.
- Approaches to enforcement should include evaluation of past adherence to DMSPs in future grant applications and should ensure that early career researchers and researchers from lower-resourced institutions are not penalized for a lack of a data-sharing record.
- Assert that data includes all digital materials needed for external researchers to replicate and validate findings
- Work with domain-specific stakeholders to develop guidance for the specific components that should be included as research outputs (e.g., data, codebooks, metadata, protocols, analytic code, preregistrations).
Updates to the Center for Open Science’s efforts to track, curate, and recommend best practices in implementing the Nelson memo will be disseminated through publication and through posting on our website at https://www.cos.io/policy-reform.
Support scientific software infrastructure by requiring SBOMs for federally funded research
Federally funded research relies heavily on software. Despite considerable evidence demonstrating software’s crucial role in research, there is no systematic process for researchers to acknowledge its use, and those building software lack recognition for their work. While researchers want to give appropriate acknowledgment for the software they use, many are unsure how to do so effectively. With greater knowledge of what software is used in research underlying publications, federal research funding agencies and researchers themselves will better be able to make efficient funding decisions, enhance the sustainability of software infrastructure, identify vital yet often overlooked digital infrastructure, and inform workforce development.
All agencies that fund research should require that resulting publications include a Software Bill of Materials (SBOM) listing the software used in the research.
Details
Software is a cornerstone in research. Evidence from numerous surveys consistently shows that a majority of researchers rely heavily on software. Without it, their work would likely come to a standstill. However, there is a striking contrast between the crucial role that software plays in modern research and our knowledge of what software is used, as well as the level of recognition it receives. To bridge this gap, we propose policies to properly acknowledge and support the essential software that powers research across disciplines.
Software citation is one way to address these issues, but citation alone is insufficient as a mechanism to generate software infrastructure insights. In recent years, there has been a push for the recognition of software as a crucial component of scholarly publications, leading to the creation of guidelines and specialized journals for software citation. However, software remains under-cited due to several challenges, including friction with journals’ reference list standards, confusion regarding which or when software should be cited, and opacity of the roles and dependencies among cited software. Therefore, we need a new approach to this problem.
A Software Bill of Materials (SBOM) is a list of the software components that were used in an effort, such as building application software. Executive Order 14028 requires that all federal agencies obtain SBOMs when they purchase software. For this reason, many high-quality open-source SBOM tools already exist and can be straightforwardly used to generate descriptions of software used in research.
SBOM tools can identify and list the stack of software underlying each publication, even when the code itself is not openly shared. If we were able to combine software manifests from many publications together, we would have the insights needed to better advance research. SBOM data can help federal agencies find the right mechanism (funding, in-kind contribution of time) to sustain software critical to their missions. Better knowledge about patterns of software use in research can facilitate better coordination among developers and reduce friction in their development roadmaps. Understanding the software used in research will also promote public trust in government-funded research through improved reproducibility.
Recommendation
We recommend the adoption of Software Bills of Materials (SBOMs) — which are already used by federal agencies for security reasons — to understand the software infrastructure underlying scientific research. Given their mandatory use for software suppliers to the federal government, SBOMs are ideal for highlighting software dependencies and potential security vulnerabilities. The same tools and practices can be used to generate SBOMs for publications. We, therefore, recommend that all agencies that fund research should require resulting publications to include an SBOM listing the software used in the research. Additionally, for research that has already been published with supplementary code materials, SBOMs should be generated retrospectively. This will not only address the issue of software infrastructure sustainability but also enhance the verification of research by clearly documenting the specific software versions used and directing limited funds to software maintenance that most need it.
- The Office of Science and Technology Policy (OSTP) should coordinate with agencies to undertake feasibility studies of this policy, building confidence that it would work as intended.
- Coordination should include funding agencies, federal actors currently applying SBOMs in software procurement, organizations developing SBOM tools and standards, and scientific stakeholders.
- Coordination should include funding agencies, federal actors currently applying SBOMs in software procurement, organizations developing SBOM tools and standards, and scientific stakeholders.
- Based on the results of the study, OSTP should direct funding agencies to design and implement policies requiring that publications resulting from federal funding include an openly accessible, machine-readable SBOM for the software used in the research.
- OSTP and the Office of Management and Budget should additionally use the Multi-Agency Research and Development Budget Priorities to encourage agencies’ collection, integration, and analysis of SBOM data to inform funding and workforce priorities and to catalyze additional agency resource allocations for software infrastructure assessment in follow-on budget processes.
Create an Office of Co-Production at the National Institutes of Health
The National Institutes of Health (NIH) spent $49 billion in fiscal year 2023 on research and development, a significant annual investment in medical treatment discovery and development. Despite NIH’s research investments producing paradigm-shifting therapies, such as CAR-T cancer treatments, CRISPR-enabled gene therapy for sickle cell, and the mRNA vaccine for COVID-19, the agency and medical scientists more broadly are grappling with declining trust. This further compounds decades-long mistrust in medical research by marginalized populations, whom researchers struggle to recruit as participants in medical research. If things do not improve, a lack of representation may lead to lack of access to effective medical interventions, worsen health disparities, and cost hundreds of billions of dollars.
A new paradigm for research is needed to ensure meaningful public engagement and rebuild trust. Co-production —in which researchers, patients, and practitioners work together as collaborators — offers a framework for embedding collaboration and trust into the biomedical enterprise.
The National Institutes of Health should form an Office of Co-Production in the Office of the Director, Division of Program Coordination, Planning, and Strategic Initiatives.
Details
In accordance with Executive Order 13985 and ongoing public access initiatives, science funding and R&D agencies have been seeking ways to embed equity, accessibility, and public participation into their processes. The NIH has been increasingly working to advance publicly engaged and led research, illustrated by trainings and workshops around patient-engaged research, funding resources for community partnerships like RADx Underserved Populations, community-led research programs like Community Partnerships to Advance Science for Society (ComPASS), and support from the new NIH director.
To ensure that public engagement efforts are sustainable, it is critical to invest in lasting infrastructure capable of building and maintaining these ties. Indeed, in their Recommendation on Open Science, the United Nations Educational, Scientific, and Cultural Organization outlined infrastructure that must be built for scientific funding to include those beyond STEMM practitioners in research decision-making. One key approach involves explicitly supporting the co-production of research, a process by which “researchers, practitioners and the public work together, sharing power and responsibility from the start to the end of the project, including the generation of knowledge.”
Co-production provides a framework with which the NIH can advance patient involvement in research, health equity, uptake and promotion of new technologies, diverse participation in clinical trials, scientific literacy, and public health. Doing so effectively would require new models for including and empowering patient voices in the agency’s work.
Recommendations
The NIH should create an Office of Co-Production within the Office of the Director, Division of Program Coordination, Planning, and Strategic Initiatives (DPCPSI). The Center for Co-Production would institutionalize best practices for co-producing research, train NIH and NIH-funded researchers in co-production principles, build patient-engaged research infrastructure, and fund pilot projects to build the research field.
The NIH Office of Co-Production, co-led by patient advocates (PA) and NIH personnel, should be established with the following key programs:
- A Resources and Training Program that trains patient advocates and researchers, both separately and together, so they can understand and work together as collaborators. This work would include helping researchers develop understanding about the communities affected by diseases they are investigating, relationship-building strategies, and ways to address power differentials, and helping patient advocates gain understanding about research processes, including understanding disease pathogenesis, different mechanisms of action and targets for research, and clinical research processes including regulatory requirements and ethical considerations. PAs could also be trained to qualify to serve on Data and Safety Monitoring Boards (DSMB).
- A Patient Advocate Advisors Management Program that would manage the placement of PAs into community advisory bodies, into advisory roles to NIH institutes’ major initiatives, onto ethical advisory bodies, onto DSMBs, onto peer review committees and study sections, and onto key long-range planning bodies, including those determining research prioritization.
- A Co-Production Principles and Practice Program led by a senior team of PAs and advisors that coordinates, organizes, and facilitates cross-disease understanding and solidarity and establishes basic principles for patient advocate engagement, grant requirements, and ongoing assessment of the quality of co-production and relational infrastructure. This program will focus on key principles such as:
- Sharing of power – the research is jointly owned and people work together to achieve a joint understanding
- Including all perspectives and skills – make sure the research team includes all those who can make a contribution
- Respecting and valuing the knowledge of all those working together on the research – everyone is of equal importance
- Reciprocity – everybody benefits from working together
- Building and maintaining relationships – an emphasis on relationships is key to sharing power. There needs to be joint understanding and consensus and clarity over roles and responsibilities. It is also important to value people and unlock their potential.
- A Communications, Planning, and Policy Program that works with the NIH director and institute directors to advocate for mutual goals to advance the public engagement mission of the NIH and its institutes.
- A Grantmaking Program that can pilot the expansion and scaling of NIH-sponsored Co-Production Cores and support the involvement of patient advocates in NIH-funded research across the country through equitable participation and standard compensation policies.
Creating an Office of Co-Production would achieve the following goals:
- It would address the growing gulf between the public, who ultimately fund biomedical research with their tax dollars, and researchers by directly and meaningfully engaging patient advocates in biomedical and clinical science. Co-production builds relationships and trust as it requires that relationships are valued and nurtured and that efforts are made to redress power differentials.
- By working early and often with patient populations around treatments, co-production helps medical scientists better anticipate and address risk early in the research process.
- It would institutionalize a known model for collaborative research that efficiently uses research dollars. During the HIV/AIDS crisis, rapid advances in biomedical and clinical research were made possible by patient advocate involvement in trial design, recruitment, and analysis.
- The NIH Center would create a replicable model of institutional support for co-production that can be scaled across the federal R&D agencies. The NIH should regularly report on the progress made by the Center for Co-Production to encourage replication in other agencies that can benefit from increased public participation.
Make government-funded hardware open source by default
While scientific publications and data are increasingly made publicly accessible, designs and documentation for scientific hardware — another key output of federal funding and driver of innovation — remain largely closed from view. This status quo can lead to redundancy, slowed innovation, and increased costs. Existing standards and certifications for open source hardware provide a framework for bringing the openness of scientific tools in line with that of other research outputs. Doing so would encourage the collective development of research hardware, reduce wasteful parallel creation of basic tools, and simplify the process of reproducing research. The resulting open hardware would be available to the public, researchers, and federal agencies, accelerating the pace of innovation and ensuring that each community receives the full benefit of federally funded research.
Federal grantmakers should establish a default expectation that hardware developed as part of federally supported research be released as open hardware. To retain current incentives for translation and commercialization, grantmakers should design exceptions to this policy for researchers who intend to patent their hardware.
Details
Federal funding plays an important role in setting norms around open access to research. The White House Office of Science and Technology Policy (OSTP)’s recent Memorandum Ensuring Free, Immediate, and Equitable Access to Federally Funded Research makes it clear that open access is a cornerstone of a scientific culture that values collaboration and data sharing. OSTP’s recent report on open access publishing further declares that “[b]road and expeditious sharing of federally funded research is fundamental for accelerating discovery on critical science and policy questions.”
These efforts have been instrumental in providing the public with access to scientific papers and data — two of the foundational outputs of federally funded research. Yet hardware, another key input and output of science and innovation, remains largely hidden from view. To continue the move towards an accessible, collaborative, and efficient scientific enterprise, public access policies should be expanded to include hardware. Specifically, making federally funded hardware open source by default would have a number of specific and immediate benefits:
Reduce Wasteful Reinvention. Researchers are often forced to develop testing and operational hardware that supports their research. In many cases, unbeknownst to those researchers, this hardware has already been developed as part of other projects by other researchers in other labs. However, since that original hardware was not openly documented and licensed, subsequent researchers are not able to learn from and build upon this previous work. The lack of open documentation and licensing is also a barrier to more intentional, collaborative development of standardized testing equipment for research.
Increase Access to Information. As the OSTP memo makes clear, open access to federally funded research allows all Americans to benefit from our collective investment. This broad and expeditious sharing strengthens our ability to be a critical leader and partner on issues of open science around the world. Immediate sharing of research results and data is key to ensuring that benefit. Explicit guidance on sharing the hardware developed as part of that research is the next logical step towards those goals.
Alternative Paths to Recognition. Evaluating a researcher’s impact often includes an assessment of the number of patents they can claim. This is in large part because patents are easy to quantify. However, this focus on patents creates a perverse incentive for researchers to erect barriers to follow on study even if they have no intention of using patents to commercialize their research. Encouraging researchers to open source the hardware developed as part of their research creates an alternative path to evaluate their impact, especially as those pieces of open source hardware are adopted and improved by others. Uptake of researchers’ open hardware could be included in assessments on par with any patented work. This path recognizes the contribution to a collective research enterprise.
Verifiability. Open access to data and research are important steps towards allowing third parties to verify research conclusions. However, these tools can be limited if the hardware used to generate the data and produce the research are not themselves open. Open sourcing hardware simplifies the process of repeating studies under comparable conditions, allowing for third-party validation of important conclusions.
Recommendations
Federal grantmaking agencies should establish a default presumption that recipients of research funds make hardware developed with those funds available on open terms. This policy would apply to hardware built as part of the research process, as well as hardware that is part of the final output. Grantees should be able to opt out of this requirement with regards to hardware that is expected to be patented; such an exception would provide an alternative path for researchers to share their work without undermining existing patent-based development pathways.
To establish this policy, OSTP should conduct a study and produce a report on the current state of federally funded scientific hardware and opportunities for open source hardware policy.
- As part of the study, OSTP should coordinate and convene stakeholders to discuss and align on policy implementation details — including relevant researchers, funding agencies, U.S. Patent and Trademark Office officials, and leaders from university tech transfer offices.
- The report should provide a detailed and widely applicable definition of open source hardware, drawing on definitions established in the community — in particular, the definition maintained by the Open Source Hardware Association, which has been in use for over a decade and is based on the widely recognized definition of open source software maintained by the Open Source Initiative.
- It should also lay out a broadly acceptable policy approach for encouraging open source by default, and provide guidance to agencies on implementation. The policy framework should include recommendations for:
- Minimally burdensome components of the grant application and progress report with which to capture relevant information regarding hardware and to ensure planning and compliance for making outputs open source
- A clear and well-defined opportunity for researchers to opt out of this mandate when they intend to patent their hardware
The Office of Management and Budget (OMB) should issue a memorandum establishing a policy on open source hardware in federal research funding. The memorandum should include:
- The rationale for encouraging open source hardware by default in federally funded scientific research, drawing on the motivation of public access policies for publications and data
- A finalized definition of open source hardware to be used by agencies in policy implementation
- The incorporation of OMB’s Open Source Scientific Hardware Policy, in alignment with the OSTP report and recommendations
Conclusion
The U.S. government and taxpayers are already paying to develop hardware created as part of research grants. In fact, because there is not currently an obligation to make that hardware openly available, the federal government and taxpayers are likely paying to develop identical hardware over and over again.
Grantees have already proven that existing open publication and open data obligations promote research and innovation without unduly restricting important research activities. Expanding these obligations to include the hardware developed under these grants is the natural next step.
Promoting reproducible research to maximize the benefits of government investments in science
Scientific research is the foundation of progress, creating innovations like new treatments for melanoma and providing behavioral insights to guide policy in responding to events like the COVID-19 pandemic. This potential for real-world impact is best realized when research is rigorous, credible, and subject to external confirmation. However, evidence suggests that, too often, research findings are not reproducible or trustworthy, preventing policymakers, practitioners, researchers, and the public from fully capitalizing on the promise of science to improve social outcomes in domains like health and education.
To build on existing federal efforts supporting scientific rigor and integrity, funding agencies should study and pilot new programs to incentivize researchers’ engagement in credibility-enhancing practices that are presently undervalued in the scientific enterprise.
Details
Federal science agencies have a long-standing commitment to ensuring the rigor and reproducibility of scientific research for the purposes of accelerating discovery and innovation, informing evidence-based policymaking and decision-making, and fostering public trust in science. In the past 10 years alone, policymakers have commissioned three National Academies reports, a Government Accountability Office (GAO) study, and a National Science and Technology Council (NSTC) report exploring these and related issues. Unfortunately, flawed, untrustworthy, and potentially fraudulent studies continue to affect the scientific enterprise.
The U.S. government and the scientific community have increasingly recognized that open science practices — like sharing research code and data, preregistering study protocols, and supporting independent replication efforts — hold great promise for ensuring the rigor and replicability of scientific research. Many U.S. science agencies have accordingly launched efforts to encourage these practices in recent decades. Perhaps the most well-known example is the creation of clinicaltrials.gov and the requirements that publicly and privately funded trials be preregistered (in 2000 and 2007, respectively), leading, in some cases, to fewer trials reporting positive results.
More recent federal actions have focused on facilitating sharing of research data and materials and supporting open science-related education. These efforts seek to build on areas of consensus given the diversity of the scientific ecosystem and the resulting difficulty of setting appropriate and generalizable standards for methodological rigor. However, further steps are warranted. Many key practices that could enhance the government’s efforts to increase the rigor and reproducibility of scientific practice — such as the preregistration of confirmatory studies and replication of influential or decision-relevant findings — remain far too rare. A key challenge is the weak incentive to engage in these practices. Researchers perceive them as costly or undervalued given the professional rewards created by the current funding and promotion system, which encourages exploratory searches for new “discoveries” that frequently fail to replicate. Absent structural change to these incentives, uptake is likely to remain limited.
Recommendations
To fully capitalize on the government’s investments in education and infrastructure for open science, we recommend that federal funding agencies launch pilot initiatives to incentivize and reward researchers’ pursuit of transparent, rigorous, and public good-oriented practices. Such efforts could enhance the quality and impact of federally funded research at relatively low cost, encourage alignment of priorities and incentive structures with other scientific actors, and help science and scientists better deliver on the promise of research to benefit society. Specifically, NIH and NSF should:
Establish discipline-specific offices to launch initiatives around rigor and reproducibility
- Use the National Institute for Neurological Disorders and Stroke’s Office of Research Quality (ORQ) as a model; similar ORQs would encourage uptake of under-incentivized practices through both internal initiatives and external funding programs.
- To ensure that programs are tailored to fit the priorities of a single disciplinary context, offices should be established within individual NIH institutes and within individual NSF directorates.
Incorporate assessments of transparent and credible research methods into their learning agendas
- Include questions to better understand existing practices, such as “How frequently and effectively do [agency]-funded researchers across disciplines engage in open science practices — e.g., preregistration, publication of null results, and external replication — and how do these practices relate to future funding and research outcomes?”
- Include questions to inform new policies and initiatives, such as “What steps could [agency] take to incentivize broader uptake of open science practices, and which ones — e.g., funding programs, application questions, standards, and evaluation models — are most effective?”
- To answer these questions, solicit feedback from applicants, reviewers, and program officers, and partner with external “science of science management” researchers to design rigorous prospective and retrospective studies; use the information obtained to develop new processes to incentivize and reward open science practices in funded research.
Expand support for third-party replications
- Allocate a consistent proportion of funds to support independent replications of key findings through the use of non-grant mechanisms — e.g., prizes, cooperative agreements, and contracts. The high value placed on scientific novelty discourages such studies, which could provide valuable information about treatment, policy, regulatory approval, or future scientific inquiry. A combination of agency prioritization and public requests for information should be used to identify topics for which additional supportive or contradictory evidence would provide significant societal and/or scientific benefit.
- The NSF, in partnership with an independent third party organization like the Institute for Replication, should run a pilot study to assess the utility of commissioning targeted and/or randomized replication studies for advancing research rigor and informing future funding.
Build capacity for agency use of open science hardware
When creating, using, and buying tools for agency science, federal agencies rely almost entirely on proprietary instruments. This is a missed opportunity because open source hardware — machines, devices, and other physical things whose design has been released to the public so that anyone can make, modify, distribute, and use them — offer significant benefits to federal agencies, to the creators and users of scientific tools, and to the scientific ecosystem.
In scientific work in the service of agency missions, the federal government should use and contribute to open source hardware.
Details
Open source has transformative potential for science and for government. Open source tools are generally lower cost, promote reuse and customization, and can avoid dependency on a particular vendor for products. Open source engenders transparency and authenticity and builds public trust in science. Open source tools and approaches build communities of technologists, designers, and users, and they enable co-design and public engagement with scientific tools. Because of these myriad benefits, the U.S. government has made significant strides in using open source software for digital solutions. For example, 18F, an office within the General Services Administration (GSA) that acts as a digital services consultancy for agency partners, defaults to open source for software created in-house with agency staff as well as in contracts it negotiates.
Open science hardware, as defined by the Gathering for Open Science Hardware, is any physical tool used for scientific investigations that can be obtained, assembled, used, studied, modified, shared, and sold by anyone. It includes standard lab equipment as well as auxiliary materials, such as sensors, biological reagents, and analog and digital electronic components. Beyond a set of scientific tools, open science hardware is an alternative approach to the scientific community’s reliance on expensive and proprietary equipment, tools, and supplies. Open science hardware is growing quickly in academia, with new networks, journals, publications, and events crossing institutions and disciplines. There is a strong case for open science hardware in the service of the United Nations’ Sustainable Development Goals, as a collaborative solution to challenges in environmental monitoring, and to increase the impact of research through technology transfer. Although limited so far, some federal agencies support open science hardware, such as an open source Build-It-Yourself Rover; the development of infrastructure, including NIH 3D, a platform for sharing 3D printing files and documentation; and programs such as the National Science Foundation’s Pathways to Enable Open-Source Ecosystems.
If federal agencies regularly used and contributed to open science hardware for agency science, it would have a transformative effect on the scientific ecosystem.
Federal agency procurement practices are complex, time-intensive, and difficult to navigate. Like other small businesses and organizations, the developers and users of open science hardware often lack the capacity and specialized staff needed to compete for federal procurement opportunities. Recent innovations demonstrate how the federal government can change how it buys and uses equipment and supplies. Agency Innovation Labs at the Department of Defense, Department of Homeland Security, National Oceanic and Atmospheric Association (NOAA), National Aeronautics and Space Administration, National Institute of Standards and Technology, and the Census Bureau have developed innovative procurement strategies to allow for more flexible and responsive government purchasing and provide in-house expertise to procurement officers on using these models in agency contexts. These teams provide much-needed infrastructure for continuing to expand the understanding and use of creative, mission-oriented procurement approaches, which can also support open science hardware for agency missions.
Agencies such as the Environmental Protection Agency (EPA), NOAA, and the Department of Agriculture (USDA) are well positioned to both benefit greatly from and make essential contributions to the open source ecosystem. These agencies have already demonstrated interest in open source tools; for example, the NOAA Technology Partnerships Office has supported the commercialization of open science hardware that is included in the NOAA Technology Marketplace, including an open source ocean temperature and depth logger and a sea temperature sensor designed by NOAA researchers and partners. These agencies have significant need for scientific instrumentation for agency work, and they often develop and use custom solutions for agency science. Each of these agencies has a demonstrated commitment to broadening public participation in science, which open science hardware supports. For example, EPA’s Air Sensor Loan Programs bring air sensor technology to the public for monitoring and education. Moreover, these agencies’ missions invite public engagement in a way that a commitment to open source instrumentation and tools would build a shared infrastructure for progress in the public good.
Recommendations
We recommend that the GSA take the following steps to build capacity for the use of open science hardware across government:
- Create an Interagency Community of Practice for federal staff working on open source–related topics.
- Direct the Technology Transformation Services to create boilerplate language for procurement of open source hardware that is compliant with the Federal Acquisition Authority and the America COMPETES Reauthorization Act of 2010.
- Conduct training on open source and open science hardware for procurement professionals across government.
We also recommend that EPA, NOAA, and USDA take the following steps to build capacity for agency use of open science hardware:
- Task agency representatives to identify agency scientific instrumentation needs that are most amenable to open source solutions. For example, the EPA Office of Research and Development could use and contribute to open source air quality sensors for research on spatial and temporal variation in air quality, and the USDA could use and contribute to an open source soil testing kit.
- Task agency challenge and prize coordinators with working intra-agency on a challenge or prize competition to create an open source option for one of the identified scientific instruments or sensors above that meets agency quality requirements.
- Support agency staff in using open source approaches when creating and using scientific instrumentation. Include open source scientific instrumentation in internal communication products, highlight staff efforts to create and use open science hardware, and provide training to agency staff on its development and use.
- Integrate open source hardware into Procurement Innovation Labs or agency procurement offices. This may include training for acquisition professionals on the use of open science hardware so that they can understand the benefits and better support agency staff use. This can include options for using open source designs and how to understand and use open source licenses.
Conclusion
Defaulting to open science hardware for agency science will result in an open library of tools for science that are replicable and customizable and result in a much higher return on investment. Beyond that, prioritizing open science hardware in agency science would allow all kinds of institutions, organizations, communities, and individuals to contribute to agency science goals in a way that builds upon each of their efforts.