AI, Energy, and Climate: What’s at Stake? Hint: A lot.
DC’s first-ever Climate Week brought with it many chances to discuss the hottest-button topics in climate innovation and policy. FAS took the opportunity to do just that, by hosting a panel to explore the intersection of artificial intelligence (AI), energy, and climate issues with leading experts. Dr. Oliver Stephenson, FAS’ Associate Director of Artificial Intelligence and Emerging Technology Policy, sat down with Dr. Tanya Das, Dr. Costa Samaras, and Charles Hua to discuss what’s at stake at this critical crossroads moment.
Missed the panel? Don’t fret. Read on to learn the need-to-knows. Here’s how these experts think we can maximize the “good” and minimize the “bad” of AI and data centers, leverage research and development (R&D) to make AI tools more successful and efficient, and how to better align incentives for AI growth with the public good.
First, Some Level Setting
The panelists took their time to make sure the audience understood two key facts regarding this space. First, not all data centers are utilized for AI. The Electric Power Research Institute (EPRI) estimates that AI applications are only used in about 10-20% of data centers. The rest? Data storage, web hosting capabilities, other cloud computing, and more.
Second, load growth due to the energy demand of data centers is happening, but the exact degree still remains unknown. Lawrence Berkeley National Lab (LBNL) models project that data centers in the US will consume anywhere between 6.7% and 12% of US electricity generation by 2028. For a country that consumes roughly 4 trillion kilowatt hours (kWh) of electricity each year, this estimation spans a couple hundred billion kWh/year from the low end to the high. Also, these projections are calculated based on different assumptions that factor in AI energy efficiency improvements, hardware availability, regulatory decisions, modeling advancements, and just how much demand there will be for AI. When each of these conditions are evolving daily, even the most credible projections come with a good amount of uncertainty.
There is also ambiguity in the numbers and in the projections at the local and state levels, as many data center companies shop around to multiple utilities to get the best deal. This can sometimes lead to projects getting counted twice in local projections. Researchers at LBNL have recently said they can confidently make data center energy projections out to 2028. Beyond that, they can’t make reasonable assumptions about data center load growth amid growing load from other sectors working to electrify—like decarbonizing buildings and electric vehicle (EV) adoption.
Maximizing the Good, Minimizing the Bad
As data center clusters continue to proliferate across the United States, their impacts—on energy systems and load growth, water resources, housing markets, and electricity rates—will be most acutely felt at the state and local levels. DC’s nearby neighbor Northern Virginia has become a “data center alley” with more than 200 data centers in Loudoun County alone, and another 117 in the planning stages.
States ultimately hold the power to shape the future of the industry through utility regulation, zoning laws, tax incentives, and grid planning – with specific emphasis on state Public Utility Commissions (PUCs). PUCs have a large influence on where data centers can be connected to the grid and the accompanying rate structure for how each data center pays for its power—whether through tariffs, increasing consumer rates, or other cost agreements. It is imperative that vulnerable ratepayers are not left to shoulder the costs and risks associated with the rapid expansion of data centers, including higher electricity bills, increased grid strain, and environmental degradation.
Panelists emphasized that despite the potential negative impacts of AI and data centers expansion, leaders have a real opportunity to leverage AI to maximize positive outcomes—like improving grid efficiency, accelerating clean energy deployment, and optimizing public services—while minimizing harms like overconsumption of energy and water, or reinforcing environmental injustice. Doing so, however, will require new economic and political incentives that align private investment with public benefit.
Research & Development at the Department of Energy
The U.S. Department of Energy (DOE) is uniquely positioned to help solve the challenges AI and data centers pose, as the agency sits at the critical intersection of AI development, high-performance computing, and energy systems. DOE’s national laboratories have been central to advancing AI capabilities: Oak Ridge National Laboratory (ORNL) was indeed the first to integrate graphics processing units (GPUs) into supercomputers, pioneering a new era of AI training and modeling capacity. DOE also runs two of the world’s most powerful supercomputers – Aurora at Argonne National Lab and Frontier at ORNL – cementing the U.S.’ leadership in high-performance computing.
Beyond computing, DOE plays a key role in modernizing grid infrastructure, advancing clean energy technologies, and setting efficiency standards for energy-intensive operations like data centers. The agency has also launched programs like the Frontiers in Artificial Intelligence for Science, Security and Technology (FASST), overseen by the Office of Critical and Emerging Tech (CET), to coordinate AI-related activities across its programs.
As the intersection of AI and energy deepens—with AI driving data center expansion and offering tools to manage its impact—DOE must remain at the center of this conversation, and it must continue to deliver. The stakes are high: how we manage this convergence will influence not only the pace of technological innovation but also the equity and sustainability of our energy future.
Incentivizing Incentives: Aligning AI Growth with the Public Good
The U.S. is poised to spend a massive amount of carbon to power the next wave of artificial intelligence. From training LLMs to supporting real-time AI applications, the energy intensity of this sector is undeniable—and growing. That means we’re not just investing financially in AI; we’re investing environmentally. To ensure that this investment delivers public value, we must align political and economic incentives with societal outcomes like grid stability, decarbonization, and real benefits for American communities.
One of the clearest opportunities lies in making data centers more responsive to the needs of the electric grid. While these facilities consume enormous amounts of power, they also hold untapped potential to act as flexible loads—adjusting their demand based on grid conditions to support reliability and integrate clean energy. The challenge? There’s currently little economic incentive for them to do so. One panelist noted skepticism that market structures alone will drive this shift without targeted policy support or regulatory nudges.
Instead, many data centers continue to benefit from “sweetheart deals”—generous tax abatements and economic development incentives offered by states and municipalities eager to attract investment. These agreements often lack transparency and rarely require companies to contribute to local energy resilience or emissions goals. For example, in several states, local governments have offered multi-decade property tax exemptions or reduced electricity rates without any accountability for climate impact or grid contributions.
New AI x Energy Policy Ideas Underway
If we’re going to spend gigatons of carbon in pursuit of AI-driven innovation, we must be strategic about where and how we direct incentives. That means:
- Conditioning public subsidies on data center flexibility and efficiency performance.
- Requiring visibility into private energy agreements and emissions footprints.
- Designing market signals—like time-of-use pricing or demand response incentives—that reward facilities for operating in sync with clean energy resources.
We don’t just need more incentives—we need better ones. And we need to ensure they serve public priorities, not just private profit. Through our AI x Energy Policy Sprint, FAS is working with leading experts to develop promising policy solutions for the Trump administration, Congress, and state and local governments. These policy memos will address how to: mitigate the energy and environmental impacts of AI systems and data centers, enhance the reliability and efficiency of energy systems using AI applications, and unlock transformative technological solutions with AI and energy R&D.
Right now, we have a rare opportunity to shape U.S. policy at the critical intersection of AI and energy. Acting decisively today ensures we can harness AI to drive innovation, revolutionize energy solutions, and sustainably integrate transformative technologies into our infrastructure.
Securing American AI Leadership: A Strategic Action Plan for Innovation, Adoption, and Trust
The Federation of American Scientists (FAS) submitted the following response to the Request for Information (RFI) issued by the Office of Science and Technology Policy (OSTP) in February 2025 regarding the development of an Artificial Intelligence (AI) Action Plan.
At a time when AI is poised to transform every sector of the economy, the Trump administration has a critical opportunity to solidify America’s leadership in this pivotal technology. Building on the foundations laid during the first Trump administration, bold and targeted policies can unleash innovation, unlocking AI’s vast potential to stimulate economic growth, revolutionize industries, and strengthen national security. However, innovation alone is insufficient; without public trust, AI adoption will stall. Ensuring AI systems are transparent, reliable, and aligned with American values will accelerate responsible adoption and solidify AI as a cornerstone of America’s economic and technological leadership.
To sustain America’s leadership in AI innovation, accelerate adoption across the economy, and guarantee that AI systems remain secure and trustworthy, we offer a set of actionable policy recommendations. Developed by FAS in partnership with prominent AI experts, industry leaders, and research institutions—including contributors to the recent FAS Day One 2025 Project and the 2024 AI Legislative Sprint—these proposals are structured around four strategic pillars: 1) unleashing AI innovation, 2) accelerating AI adoption, 3) ensuring secure and trustworthy AI, and 4) strengthening existing world-class U.S. government institutions and programs.
1) Unleashing AI Innovation. American AI leadership has been driven by bold private-sector investments and world-class academic research. However, critical high-impact areas remain underfunded. The federal government can catalyze investment and innovation by expanding access to essential data, investing strategically in overlooked areas of AI R&D, defining priority research challenges, promoting public-private partnerships, and attracting and retaining global talent.
2) Accelerating AI Adoption Across the Economy. The United States leads in AI breakthroughs, but these breakthroughs must translate into widespread adoption to maximize their economic and societal benefits. Accelerating adoption—a critical yet often overlooked driver of national competitiveness—requires addressing workforce readiness, expanding government capacity, and managing rising energy demands.
3) Ensuring Secure and Trustworthy AI. Ensuring AI systems are secure and trustworthy is essential not only for fostering public confidence and accelerating widespread adoption, but also for improving government efficiency and ensuring the responsible use of taxpayer resources when AI is deployed by public agencies. While the previous Trump administration recognized the necessity of public trust when promoting AI adoption, concerns persist about AI’s rapid evolution, unpredictable capabilities, and potential for misuse. Future AI accidents could further erode this trust, stalling AI progress. To address these risks and fully harness AI’s potential, the U.S. government must proactively monitor emerging threats, rigorously evaluate AI technologies, and encourage innovation that upholds fundamental American values such as privacy.
4) Strengthening Existing World-Class U.S. Government AI Institutions and Programs. Realizing the Trump Administration’s goals will require building on leading government AI capabilities. Key initiatives—including the NIST AI Safety Institute (AISI), the National AI Research Resource (NAIRR) Pilot, the AI Use Case Inventory, and the Department of Energy’s Office of Critical and Emerging Technologies (CET)—advance AI innovation, security, and transparency. The AISI evaluates AI models with broad industry support, while the NAIRR Pilot expands access to AI resources beyond Big Tech. Federal AI use case inventories enhance government transparency and industry engagement, building public trust. DOE’s CET drives AI-powered advancements in science and national security. Integrating these proven initiatives into the AI Action Plan will solidify America’s AI leadership.
By acting decisively, the administration can ensure American AI remains the gold standard, drive economic competitiveness, and accelerate science and innovation.
Overview of Policy Proposals
Policy Proposals to Unleash AI Innovation
- Recommendation 1: Promote innovation in trustworthy AI through a Public-Private National Initiative for AI Explainability.
- Recommendation 2: Direct the Department of Energy (DOE) to use AI to accelerate the discovery of new materials.
- Recommendation 3: Create AI-ready collaborative datasets to accelerate progress in the life sciences.
- Recommendation 4: Establish a NIST Foundation to amplify public-private collaboration, secure private investment, and accelerate innovation.
- Recommendation 5: Attract top global talent by creating a National Security AI Entrepreneur Visa for elite dual-use technology founders.
Policy Proposals to Accelerate AI Adoption Across the Economy
- Recommendation 1: Streamline procurement processes for government use of AI.
- Recommendation 2: Establish a Federal Center of Excellence to expand state and local government capacity for AI procurement and use.
- Recommendation 3: Pilot an AI Corps at HHS to drive government-wide AI adoption.
- Recommendation 4: Make America’s teacher workforce competitive for the AI era.
- Recommendation 5: Prepare U.S. energy infrastructure for AI growth through standardized measurement and forecasting.
Policy Proposals to Ensure Secure and Trustworthy AI
- Privacy:
- Recommendation 1: Secure third party commercial data for AI through FedRAMP authorization.
- Recommendation 2: Catalyze federal data sharing through privacy enhancing technologies.
- Recommendation 3: Establish data-sharing standards to support AI development in healthcare.
- Security, Safety, and Trustworthiness:
- Recommendation 1: Establish an early warning system for AI-powered threats to national security and public safety.
- Recommendation 2: Create a voluntary AI incident reporting hub to monitor security incidents from AI.
- Recommendation 3: Promote AI trustworthiness by providing a safe harbor for AI researchers.
- Recommendation 4: Build a national digital content authentication technologies research ecosystem.
- Recommendation 5: Strengthen national security by evaluating AI-driven biological threats.
Policy Proposals to Strengthen Existing World-Class U.S. Government AI Institutions and Programs that are Key to the Trump Administration’s AI Agenda
- Recommendation 1: Support the NIST AI Safety Institute as a key pillar of American AI excellence.
- Recommendation 2: Expand the National Artificial Intelligence Research Resource from pilot to full program.
- Recommendation 3: Enhance transparency, accountability, and industry engagement by preserving the AI use case inventory.
- Recommendation 4: Propel U.S. Scientific and Security AI Leadership by Supporting AI and Computing at DOE.
Policy Proposals to Unleash AI Innovation
As artificial intelligence continues transforming industries and reshaping global competition, the United States must take bold, coordinated action to maintain its technological leadership. A multi-agency approach could include launching a National Initiative for AI Explainability, accelerating materials science discovery through AI-powered autonomous laboratories, creating AI-ready datasets for the life sciences, establishing a NIST Foundation to enhance public-private collaboration in AI research, and creating a National Security AI Entrepreneur Visa to attract and retain top global talent. Together, these initiatives would strengthen America’s AI ecosystem by addressing critical challenges in transparency, scientific research, standards development, and talent acquisition—while ensuring the U.S. remains at the forefront of responsible AI innovation.
Recommendation 1. Promote Innovation in Trustworthy AI through a Public-Private National Initiative for AI Explainability
Understanding the inner workings of AI systems is critical not only for reliability and risk mitigation in high-stakes areas such as defense, healthcare, and finance, but also for bolstering American technological leadership and maximizing government accountability and efficiency. However, despite promising progress in fields such as “mechanistic interpretability”, the study of explainability in AI systems is still nascent. A lack of explainability risks undermining trust and inhibiting AI adoption, particularly in safety-critical sectors.
To address the challenge of understanding and improving AI systems, we propose the launch of a Public-Private National Initiative for AI Explainability. Following in the footsteps of government-coordinated research projects like the Human Genome Project, this initiative would unite researchers, industry leaders, standards bodies, and government agencies to map the inner workings of advanced AI systems in a public-private partnership.
Federal precedent for such work already exists: DARPA’s 2017-2021 Explainable AI (XAI) program sought to create machine learning systems capable of explaining their decisions in a way humans could understand. While the program advanced techniques for explainable models and human-friendly translations of complex AI reasoning, the rapid development and scaling of AI technologies in the past five years demand a renewed, more ambitious effort.
The objectives of the initiative would include:
- Creating Open-Access Resources: Developing AI models, datasets, and tools accessible to researchers and practitioners, allowing a larger number of actors to contribute to progress.
- Developing Standardized Metrics and Benchmarks: Establishing clear standards to evaluate the explainability of AI systems in different circumstances, ensuring consistency and reliability across applications.
- Defining Common Tasks: Establishing standardized metrics and open datasets to create “common tasks” in explainability—well-defined challenges that drive innovation and encourage widespread progress as the broader ecosystem competes to improve performance.
- Investigating User-Centric Explanation Needs: Conducting research to identify which types of AI explanations are most effective and meaningful, and which provide appropriate degrees of control, to users across various contexts and applications.
- Developing a Repository of Explainability Techniques: Researching and disseminating explainability methods applicable across various AI domains, including an analysis of the strengths and weaknesses of different approaches and where they can be properly applied.
Implementation Strategy:
To launch this effort, the President should issue an executive order to signal national commitment and assign leadership to key federal agencies, including:
- Office of Science and Technology Policy: Playing a coordinating role across the government.
- Defense Advanced Research Projects Agency (DARPA): Building upon its prior experience with the XAI program to spearhead research efforts.
- National Institute of Standards and Technology (NIST): Developing standards and benchmarks for AI explainability, building on previous work in this area.
- National Science Foundation (NSF): Funding academic research through its National AI Research Institutes.
- Department of Energy (DOE): Leveraging its computational resources and expertise in large-scale research projects.
- Other government agencies with relevant expertise: For example, the National Institutes of Health (NIH) could focus on explainability in AI applications within the healthcare sector.
The White House should leverage its convening power to unite leading AI companies, top academic institutions, and government agencies in formal collaborations. These partnerships could encompass co-funded research, shared datasets and computing resources, collaborative access to advanced AI models, and joint development of open-source tools. Establishing a structured public-private partnership will facilitate coordinated funding, align strategic priorities, and streamline resource sharing, ensuring that advancements in AI explainability directly support both national interests and economic competitiveness. To sustain this initiative, the administration should also secure consistent, multi-year federal funding through appropriations requests to Congress.
DARPA’s XAI program showed that AI explainability requires interdisciplinary collaboration to align technical development with human understanding. Building on these insights, this initiative should include experts from computer science, cognitive science, ethics, law, and domain-specific fields to ensure explanations are clear, useful, and actionable for decision-makers across critical sectors.
By implementing this National Initiative for AI Explainability, the Trump administration can significantly enhance public confidence in AI technologies, accelerate responsible adoption by both the public and private sectors, and solidify America’s global leadership in AI innovation. Critically, a modest investment of government resources in this initiative could unlock substantial private-sector investment, spurring innovation and driving economic growth. This strategic approach will also enhance government accountability, optimize the responsible use of taxpayer resources, and ensure that American industry continues to lead in AI development and deployment.
Recommendation 2. Direct the Department of Energy (DOE) to use AI to Accelerate the Discovery of New Materials (link to full memo >>>)
Innovations in AI and robotics could revolutionize materials science by automating experimental processes and dramatically accelerating the discovery of new materials. Currently, materials science research involves manually testing different combinations of elements to identify promising materials, which limits the pace of discovery. Using AI foundation models for physics and chemistry, scientists could simulate new materials, while robotic “self-driving labs” could run 24/7 to synthesize and evaluate them autonomously. This approach would enable continuous data generation, refining AI models in a feedback loop that speeds up research and lowers costs. Given its expertise in supercomputing, AI, and a vast network of national labs, the Department of Energy (DOE) could lead this transformative initiative, potentially unlocking advancements in critical materials, such as improved battery components, that could have immense economic and technological impacts.
Recommendation 3. Create AI-ready Collaborative Datasets to Accelerate Progress in the Life Sciences (link to full memo >>>)
Large, high-quality datasets could revolutionize life science research by powering AI models that unlock new discoveries in areas like drug development and diagnostics. Currently, researchers often work in silos with limited incentives to collaborate and share meticulously curated data, slowing progress. By launching a government-funded, end-to-end initiative—from identifying critical dataset needs to certifying automated collection methods and hosting robust open repositories—scientists could continuously generate and refine data, fueling AI models in a feedback loop that boosts accuracy and lowers costs. Even a relatively modest government investment could produce vital resources for researchers and startups to spark new industries. This model could also be extended to a range of other scientific fields to accelerate U.S.science and innovation.
Recommendation 4. Create a NIST Foundation to Support the Agency’s AI Mandate (link to full memo >>>)
To maintain America’s competitive edge in AI, NIST needs greater funding, specialized talent, and the flexibility to work effectively with private-sector partners. One solution is creating a “NIST Foundation,” modeled on the DOE’s Foundation for Energy Security and Innovation (FESI), which combines federal and private resources to expand capacity, streamline operations, and spur innovation. Legislation enabling such a foundation was introduced with bipartisan support in the 118th Congress, signaling broad consensus on its value. The Trump administration can direct NIST to study how a nonprofit foundation might boost its AI initiatives and broader mission—just as a similar report helped pave the way for FESI—giving Congress the evidence it needs to formally authorize a NIST Foundation. The administration can also support passage of authorizing legislation through Congress.
Recommendation 5. Attract Top Global Talent by Creating a National Security AI Entrepreneur Visa for Elite Dual-use Technology Founders (link to full memo >>>)
America’s leadership in AI has been driven by the contributions of immigrant entrepreneurs, with companies like NVIDIA, Anthropic, OpenAI, X, and HuggingFace—all of which have at least one immigrant co-founder—leading the charge. To maintain this competitive edge as global competition intensifies, the administration should champion a National Security Startup Visa specifically targeted at high-skilled founders of AI firms. These entrepreneurs are at the forefront of developing dual-use technologies critical for both America’s economic leadership and national security. Although the linked proposal above is targeted at legislative action, the administration can take immediate steps to advance this priority by publicly supporting legislation to establish such a visa, engaging with Congressional allies to underscore its strategic importance, and directing agencies like the Department of Homeland Security and the Department of Commerce to explore ways to streamline pathways for these innovators. This decisive action would send a clear signal that America remains the destination of choice for world-class talent, ensuring that the nation stays ahead in the race for AI dominance.
Policy Proposals to Accelerate AI Adoption Across the Economy
AI has transformative potential to boost economic growth and unlock new levels of prosperity for all. The Trump administration should take bold action to encourage greater adoption of AI technologies and AI expertise by leveraging government procurement, hiring, and standards-setting processes, alongside coordinated support for America’s teachers to prepare students to join the future AI workforce. In government, a coordinated set of federal initiatives is needed to modernize and streamline effective AI adoption in the public sector. These proposals include developing a national digital platform through GSA to streamline AI procurement processes, establishing a federal center of excellence to support state and local governments in AI implementation, and pursuing innovative hiring models to expand AI expertise at HHS. Additionally, NIST should develop voluntary standards for measuring AI energy and resource usage to inform infrastructure planning efforts. Finally, the President should announce a national teacher talent surge and set AI as a competitive priority in American education.
Recommendation 1. Streamline Procurement Processes for Government Use of AI (link to full memo >>>)
The federal government has a critical role in establishing standards for AI systems to enhance public services while ensuring they are implemented ethically and transparently. To streamline this effort and support federal agencies, the administration should direct the General Services Administration (GSA) to create a user-friendly, digital platform for AI procurement. This platform would simplify the acquisition process by providing agencies with clear, up-to-date guidelines, resources, and best practices, all tailored to align with existing procurement frameworks. The platform would empower agencies to make informed decisions that prioritize safety, fairness, and effective use of AI technologies, while demonstrating the administration’s commitment to modernizing government operations and ensuring America leads the way in adopting cutting-edge AI solutions.
Recommendation 2. Establish a Federal Center of Excellence to Expand State and Local Government Capacity for AI Procurement and Use (link to full memo >>>)
State and local governments often face challenges in effectively leveraging AI to enhance their efficiency and service capabilities. To support responsible AI adoption at the state, local, tribal, and territorial (SLTT) levels, the administration should establish a federal AI Center of Excellence. This center would provide hands-on guidance from experts in government, academia, and civil society, helping SLTT agencies navigate complex challenges such as limited technical expertise, budget constraints, privacy concerns, and evolving regulations. It would also translate existing federal AI standards—including Executive Order 13960 and the NIST Risk Management Framework—into practical, actionable advice. By developing in-house procurement and deployment expertise, SLTT governments could independently and confidently implement AI solutions, promoting innovation while ensuring responsible, effective, and efficient use of taxpayer resources.
Recommendation 3. Pilot an AI Corps at HHS to Drive Government-Wide AI Adoption (link to full memo >>>)
Federal agencies often struggle to leverage AI effectively, due to limited technical expertise and complex oversight requirements. Modeled after the Department of Homeland Security’s successful AI Corps, which has improved disaster response and cybersecurity, this pilot would embed AI and machine learning experts within the Department of Health and Human Services’s (HHS) 10 agencies, accelerating responsible AI implementation in healthcare, driving greater efficiency, and demonstrating a scalable model that could be replicated across other federal departments. HHS is uniquely suited for piloting an AI Corps because it oversees critical health infrastructure and massive, sensitive datasets—presenting significant opportunities for AI-driven improvements but also requiring careful management. If successful, this pilot could serve as a strategic blueprint to enhance AI adoption, improve government performance, and maximize the responsible use of taxpayer resources across the federal government.
Recommendation 4. Make America’s Teacher Workforce Competitive for the AI Era (link to full memo >>>)
With America facing a significant shortage of teachers and a growing need for AI and digital skills in the workforce, the Trump administration can rebuild America’s teaching profession by launching a coordinated strategy led by the Office of Science and Technology Policy (OSTP). This initiative should begin with a national teacher talent surge to expand annual teacher graduates by 100,000, addressing both the urgent workforce gap and the imperative to equip students for an AI-driven future. The plan includes a Challenge.gov competition to attract innovative recruitment and retention models, updating Department of Education scholarship programs (like the Graduate Assistance in Areas of National Need) to include AI, data science, and machine learning, convening colleges of education to modernize training, and directing agencies to prioritize AI-focused teacher development. By leveraging existing grants (e.g., Teacher Quality Partnerships, SEED, the STEM Corps, and Robert Noyce Scholarships), the administration can ensure a robust pipeline of educators ready to guide the next generation.
Recommendation 5. Prepare U.S. Energy Infrastructure for AI Growth Through Standardized Measurement and Forecasting
As AI adoption accelerates, America’s energy infrastructure faces a critical challenge: next-generation AI systems could place unprecedented demands on the power grid, yet the lack of standardized measurements, and wide variations in forecasted demand, leaves utilities and policymakers unprepared. Without proactive planning, energy constraints could slow AI innovation and undermine U.S. competitiveness.
To address this, the Administration should direct the National Institute of Standards and Technology (NIST) and the Department of Energy (DOE) to develop a standardized framework for measuring and forecasting AI’s energy and resource demands. This framework should be paired with a voluntary reporting program for AI developers—potentially collected by the Energy Information Administration (EIA)—to provide a clearer picture of AI’s impact on energy consumption. The EIA should also be tasked with forecasting AI-driven energy demand, ensuring that utilities, public utility commissions, and state energy planners have the data needed to modernize the grid efficiently.
Greater transparency will enable both government and industry to anticipate energy needs, drive investment in grid modernization, and prevent AI-related power shortages that could hinder economic growth. The proactive integration of AI and energy planning will strengthen America’s leadership in AI innovation while safeguarding the reliability of its infrastructure. FAS is actively developing policy proposals with the science and technology community at the intersection of AI and energy. We plan to share additional recommendations on this topic in the coming months.
Policy Proposals to Ensure Secure and Trustworthy AI
Privacy
Protecting Americans’ privacy while harnessing the potential of AI requires decisive federal action that prioritizes both individual rights and technological advancement. Strengthening privacy protections while enabling responsible data sharing is crucial for ensuring that AI-driven innovations improve public services without compromising sensitive information. Key initiatives include establishing NIST-led guidelines for secure data sharing and maintaining data integrity, implementing a FedRAMP authorization framework for third-party data sources used by government agencies, and promoting the use of Privacy Enhancing Technologies (PETs). Additionally, the administration should create a “Responsible Data Sharing Corps” to provide agencies with expert guidance and build capacity in responsible data practices.
Recommendation 1. Secure Third Party Commercial Data for AI through FedRAMP Authorization (link to full memo >>>)
The U.S. government is a major customer of commercial data brokers and should require a pre-evaluation process before agencies acquire large datasets, ensuring privacy and security from the outset. Thoroughly vetting data brokers and verifying compliance standards can help avert national security risks posed by compromised or unregulated third-party vendors. To formalize these safeguards, OMB and FedRAMP should create an authorization framework for data brokers that provide commercially available information, especially with personally identifiable information. Building on its established role in securing cloud providers FedRAMP is well positioned to guide these protocols, ensuring agencies work only with trusted vendors and strengthening overall data protection.
Recommendation 2. Catalyze Federal Data Sharing through Privacy Enhancing Technologies (link to full memo >>>)
To maintain America’s leadership in AI and digital innovation, the administration must ensure that government agencies can securely leverage data while protecting privacy and maintaining public trust. The federal government can lead by example through the adoption of Privacy Enhancing Technologies (PETs)—tools that enable data analysis while minimizing exposure of sensitive information. Agencies should be encouraged to adopt PETs with support from a Responsible Data Sharing Corps, while NIST develops a decision-making framework to guide their use. OMB should require agencies to apply this framework in data-sharing initiatives and report on PET adoption, with a PET Use Case Inventory and annual reports enhancing transparency. A federal fellowship program could also bring in experts from academia and industry to drive PET innovation. These measures would strengthen privacy, security, and public trust while positioning the U.S. as a global leader in responsible data use.
Recommendation 3. Establish Data-Sharing Standards to Support AI Development in Healthcare (link to full memo >>>)
The U.S. healthcare system generates vast amounts of data daily, yet fragmentation, privacy concerns, and lack of interoperability severely limit its use in AI development, hindering medical innovation. To address this, the AI Action Plan should direct NIST to lead an interagency coalition in developing standardized protocols for health data anonymization, secure sharing, and third-party access. By establishing clear technical and governance standards—similar to NIST’s Cryptographic and Biometric Standards Programs—this initiative would enable responsible research while ensuring compliance with privacy and security requirements. These standards would unlock AI-driven advancements in diagnostics, treatment planning, and health system efficiency. Other nations, including the U.K., Australia, and Finland, are already implementing centralized data-sharing frameworks; without federal leadership, the U.S. risks falling behind. By taking decisive action, the administration can position the U.S. as a global leader in medical AI, accelerating innovation while maintaining strong privacy protections.
Security, Safety, and Trustworthiness
AI holds immense promise for job growth, national security, and innovation, but accidents or misuse risk undermining public trust and slowing adoption—threatening the U.S.’s leadership in this critical field. The following proposals use limited, targeted government action alongside private-sector collaboration to strengthen America’s AI capabilities while upholding public confidence and protecting our national interests.
Recommendation 1. Establish an Early Warning System for AI-Powered Threats to National Security and Public Safety (link to full memo >>>)
Emerging AI capabilities could also pose severe threats to public safety and national security. AI companies are already evaluating their most advanced models to identify dual-use capabilities, such as the capacity to conduct offensive cyber operations, enable the development of biological or chemical weapons, and autonomously replicate and spread. These capabilities can arise unpredictably and undetected during development and after deployment. To prepare for these emerging risks, the federal government should establish a coordinated “early-warning system” for novel dual-use AI capabilities to gain awareness of emerging risks before models are deployed. A government agency could serve as a central information clearinghouse—an approach adapted from the original congressional proposal linked above. Advanced AI model developers could confidentially report newly discovered or assessed dual-use capabilities, and the White House could direct relevant government agencies to form specialized working groups that engage with private sector and other non-governmental partners to rapidly mitigate risks and leverage defensive applications. This initiative would ensure that the federal government and its stakeholders have maximum lead time to prepare for emerging AI-powered threats, positioning the U.S. as a leader in safe and responsible AI innovation.
Recommendation 2. Create a Voluntary AI Incident Reporting Hub to Monitor Security Incidents from AI (link to full memo >>>)
The federal government should establish a voluntary national Artificial Intelligence Incident Reporting Hub to better track, analyze, and address incidents from increasingly complex and capable AI systems that are deployed in the real world. Such an initiative could be modeled after successful incident reporting and info-sharing systems operated by the National Cybersecurity FFRDC, the Federal Aviation Administration, and the Food and Drug Administration. By providing comprehensive yet confidential data collection under the umbrella of an agency (e.g. NIST) this initiative would bolster public trust, facilitate the sharing of critical risk information, and enable prompt government action on emerging threats, from cybersecurity vulnerabilities to potential misuse of AI in sensitive areas like chemical, biological, radiological, or nuclear contexts. This proposal builds on bipartisan legislation introduced in the last Congress, as well as the memo linked above, which was originally targeted at Congressional action.
Recommendation 3. Promote AI Trustworthiness by Providing a Safe Harbor for AI Researchers (link to full memo >>>)
Independent AI research plays a key role in ensuring safe and reliable AI systems. In 2024, over 350 researchers signed an open letter calling for “a safe harbor for independent AI evaluation”, noting that generative AI companies offer no legal protections for independent safety researchers. This situation is unlike established voluntary protections from companies for traditional software, and Department of Justice (DOJ) guidance not to prosecute good faith security research. The proposal linked above was targeted at Congressional action, however the executive branch could adapt these ideas in several ways, by, for example: 1) instructing the Office of Management and Budget (OMB) to issue guidance to all federal agencies requiring that contracting documents for generative AI systems include safe-harbor provisions for good-faith external research, consistent with longstanding federal policies that promote responsible vulnerability disclosure. 2) Coordinating with DOJ and relevant agencies to clarify that good-faith AI security and safety testing—such as red-teaming and adversarial evaluation—does not violate the Computer Fraud and Abuse Act (CFAA) or other laws when conducted according to established guidelines.
Recommendation 4. Build a National Digital Content Authentication Technologies Research Ecosystem (link to full memo >>>)
AI generated synthetic content (such as fake videos, images, and audio) is increasingly used by malicious actors to defraud elderly Americans, spread child sexual abuse material, and impersonate political figures. To counter these threats, the United States must invest in developing technical solutions for reliable synthetic content detection. Through the National Institute of Standards and Technology (NIST), the Trump Administration can: 1) establish dedicated university-led national research centers, 2) develop a national synthetic content database, and 3) run and coordinate prize competitions to strengthen technical countermeasures.These initiatives will help build a robust research ecosystem to keep pace with the rapidly evolving synthetic content threat landscape, maintaining America’s role as a global leader in responsible and secure AI.
Recommendation 5. Strengthen National Security by Evaluating AI-Driven Biological Threats (link to full memo >>>)
Over the past two years, the rapid advance of AI in biology and large language models has highlighted an urgent need for a targeted U.S. Government program to assess and mitigate biosecurity risks. While AI-enabled tools hold immense promise for drug discovery, vaccine research, and other beneficial applications, their dual-use potential (e.g., identifying viral mutations that enhance vaccine evasion) makes them a national security priority. Building on the Department of Homeland Security’s (DHS) previous work on AI and CBRN threats, the Department of Energy (DOE), DHS, and other relevant agencies, should now jointly launch a “Bio Capability Evaluations” program, backed by sustained funding, to develop specialized benchmarks and standards for evaluating dangerous biological capabilities in AI-based research tools. By forming public-private partnerships, creating a DOE “sandbox” for ongoing testing, and integrating results into intelligence assessments, such a program would enable more nuanced, evidence-based regulations and help the United States stay ahead of potential adversaries seeking to exploit AI’s biological capabilities.
Policy Proposals to Strengthen Existing World-Class U.S. Government AI Institutions and Programs that are Key to the Trump Administration’s AI Agenda
A robust institutional framework is essential for ensuring that the government fulfills its role in AI research, industry coordination, and ecosystem development. The previous Trump administration laid the groundwork for American AI leadership, and the institutions established since then can be leveraged to further assert U.S. dominance in this critical technological space.
Recommendation 1. Support the NIST AI Safety Institute as a Key Pillar of American AI Excellence
The NIST AI Safety Institute (AISI) has assembled a world-leading team to ensure that the U.S. leads in safe, reliable, and trustworthy AI development. As AI integrates into critical sectors like national security, healthcare, and finance, strong safety standards are essential. AISI develops rigorous benchmarks, tests model security, and collaborates with industry to set standards, mitigating risks from unreliable AI. Strengthening AISI protects U.S. consumers, businesses, and national security while boosting global trust in the U.S. AI ecosystem—enhancing international adoption of American AI models. AISI has broad support, with bipartisan legislation to codify the AISI advanced in Congress and backing from organizations across industry and academia. The AI Action Plan should prioritize AISI as a pillar of AI policy.
Recommendation 2. Expand the National Artificial Intelligence Research Resource from Pilot to Full Program
For decades, academic researchers have driven AI breakthroughs, laying the foundation for the technologies that now shape global competition. However, as AI development becomes increasingly concentrated within large technology companies, the U.S. risks losing the ecosystem that made these advances possible. The National AI Research Resource (NAIRR) Pilot is a critical initiative to keep American AI innovation competitive and accessible. By providing researchers and educators across the country access to cutting-edge AI tools, datasets, and computing power, NAIRR ensures that innovation is not confined to a handful of dominant firms but widely distributed. To keep America at the forefront of AI, the Trump Administration should expand NAIRR into a full-fledged program. Allowing the program to lapse would erode America’s leadership in AI research, forcing top talent to seek resources elsewhere. To secure its future, the White House should support bipartisan legislation to fully authorize NAIRR and include it in the President’s Budget Request, ensuring sustained investment in this vital initiative.
Recommendation 3. Enhance Transparency, Accountability, and Industry Engagement by Preserving the AI Use Case Inventory (link to letter of support >>>)
The AI Use Case Inventory, established under President Trump’s Executive Order 13960 and later codified in section 7225 of the FY23 National Defense Authorization Act, plays a crucial role in fostering public trust and innovation in government AI use. Recent OMB guidance (M-24-10) has expanded its scope, refining AI classifications and standardizing AI definitions. The inventory enhances public trust and accountability by ensuring transparency in AI deployments, tracks AI successes and risks to improve government services, and supports AI vendors by providing visibility into public-sector AI needs, thereby driving industry innovation. As the federal government considers revisions to M-24-10 and its plan for AI adoption within federal agencies, OMB should uphold the 2024 guidance on federal agency AI Use Case Inventories and ensure agencies have the necessary resources to complete it effectively.
Recommendation 4. Propel U.S. Scientific and Security AI Leadership by Supporting AI and Computing at DOE
The Department of Energy (DOE) hosts leading research and innovation centers, particularly under the Undersecretary for Science and Innovation. The Office of Critical and Emerging Technologies (CET), for example, plays a key role in coordinating AI initiatives, including the proposed Frontiers in Artificial Intelligence for Science, Security, and Technology (FASST) program. To fully harness AI’s potential, DOE should establish a dedicated AI and Computing Laboratory under the Undersecretary, ensuring a strategic, mission-driven approach to AI development. This initiative would accelerate scientific discovery, strengthen national security, and tackle energy challenges by leveraging DOE’s advanced computational infrastructure and expertise. To ensure success, it should be supported by a multi-year funding commitment and flexible operational authorities, modeled after ARPA-E, to streamline hiring, procurement, and industry-academic partnerships.
Conclusion
These recommendations offer a roadmap for securing America’s leadership in artificial intelligence while upholding the fundamental values of innovation, competitiveness, and trustworthiness. By investing in cutting-edge research, equipping government and educators with the tools to navigate the AI era, and ensuring safety, the new administration can position America as a global standard-bearer for trustworthy and effective AI development.
Increasing Responsible Data Sharing Capacity throughout Government
Deriving insights from data is essential for effective governance. However, collecting and sharing data—if not managed properly—can pose privacy risks for individuals. Current scientific understanding shows that so-called “anonymization” methods that have been widely used in the past are inadequate for protecting privacy in the era of big data and artificial intelligence. The evolving field of Privacy-Enhancing Technologies (PETs), including differential privacy and secure multiparty computation, offers a way forward for sharing data safely and responsibly.
The administration should prioritize the use of PETs by integrating them into data-sharing processes and strengthening the executive branch’s capacity to deploy PET solutions.
Challenge and Opportunity
A key function of modern government is the collection and dissemination of data. This role of government is enshrined in Article 1, Section 2 of the U.S. Constitution in the form of the decennial census—and has only increased with recent initiatives to modernize the federal statistical system and expand evidence-based policymaking. The number of datasets itself has also grown; there are now over 300,000 datasets on data.gov, covering everything from border crossings to healthcare. The release of these datasets not only accomplishes important transparency goals, but also represents an important step toward advancing American society fairer, as data are a key ingredient in identifying policies that benefit the public.
Unfortunately, the collection and dissemination of data comes with significant privacy risks. Even with access to aggregated information, motivated attackers can extract information specific to individual data subjects and cause concrete harm. A famous illustration of this risk occurred in 1997 when Latanya Sweeney was able to identify the medical record of then-Governor of Massachusetts, William Weld, from a public, anonymized dataset. Since then, the power of data re-identification techniques—and incentives for third parties to learn sensitive information about individuals—have only increased, compounding this risk. As a democratic, civil-rights respecting nation, it is irresponsible for our government agencies to continue to collect and disseminate datasets without careful consideration of the privacy implications of data sharing.
While there may appear to be an irreconcilable tension between facilitating data-driven insight and protecting the privacy of individual’s data, an emerging scientific consensus shows that Privacy-Enhancing Technologies (PETs) offer a path forward. PETs are a collection of techniques that enable data to be used while tightly controlling the risk incurred by individual data subjects. One particular PET, differential privacy (DP), was recently used by the U.S. Census Bureau within their disclosure avoidance system for the 2020 decennial census in order to meet their dual mandates of data release and confidentiality. Other PETs, including variations of secure multiparty computation, have been used experimentally by other agencies, including to link long-term income data to college records and understand mental health outcomes for individuals who have earned doctorates. The National Institute of Standards and Technology (NIST) has produced frameworks and reports on data and information privacy, including PETs topics such as DP (see Q&A section). However, these reports still lack a comprehensive and actionable framework on how organizations should consider, use and deploy PETs in organizations.
As artificial intelligence becomes more prevalent inside and outside government and relies on increasingly large datasets, the need for responsible data sharing is growing more urgent. The federal government is uniquely positioned to foster responsible innovation and set a strong example by promoting the use of PETs. The use of DP in the 2020 decennial census was an extraordinary example of the government’s capacity to lead global innovation in responsible data sharing practices. While the promise of continuing this trend is immense, expanding the use of PETs within government poses twin challenges: (1) sharing data within government comes with unique challenges—both technical and legal—that are only starting to be fully understood and (2) expertise on using PETs within government is limited. In this proposal, we outline a concrete plan to overcome these challenges and unlock the potential of PETs within government.
Plan of Action
Using PETs when sharing data should be a key priority for the executive branch. The new administration should encourage agencies to consider the use of PETs when sharing data and build a United States DOGE Service (USDS) “Responsible Data Sharing Corps” of professionals who can provide in-house guidance around responsible data sharing.
We believe that enabling data sharing with PETs requires (1) gradual, iterative refinement of norms and (2) increased capacity in government. With these in mind, we propose the following recommendations for the executive branch.
Strategy Component 1. Build consideration of PETs into the process of data sharing
Recommendation 1. NIST should produce a decision-making framework for organizations to rely on when evaluating the use of PETs.
NIST should provide a step-by-step decision-making framework for determining the appropriate use of PETs within organizations, including whether PETs should be used, and if so, which PET and how it should be deployed. Specifically, this guidance should be at the same level of granularity as NIST Risk Management Framework for Cybersecurity. NIST should consult with a range of stakeholders from the broad data sharing ecosystem to create this framework. This includes data curators (i.e., organizations that collect and share data, within and outside the government); data users (i.e., organizations that consume, use and rely on shared data, including government agencies, special interest groups and researchers); data subjects; experts across fields such as information studies, computer science, and statistics; and decision makers within public and private organizations who have prior experience using PETs for data sharing. The report may build on NIST’s existing related publications and other guides for policymakers considering the use of specific PETs, and should provide actionable guidance on factors to consider when using PETs. The output of this process should be not only a decision, but also a report documenting the execution of decision-making framework (which will be instrumental for Recommendation 3).
Recommendation 2. The Office of Management and Budget (OMB) should mandate government agencies interested in data sharing to use the NIST’s decision-making framework developed in Recommendation 1 to determine the appropriateness of PETs to protect their data pipelines.
The risks to data subjects associated with data releases can be significantly mitigated with the use of PETs, such as differential privacy. Along with considering other mechanisms of disclosure control (e.g., tiered access, limiting data availability), agencies should investigate the feasibility and tradeoffs around using PETs to protect data subjects while sharing data for policymaking and public use. To that end, OMB should require government agencies to use the decision-making framework produced by NIST (in Recommendation 1) for each instance of data sharing. We emphasize that this decision-making process may lead to a decision not to use PETs, as appropriate. Agencies should compile the produced reports such that they can be accessed by OMB as part of Recommendation 3.
Recommendation 3. OMB should produce a PET Use Case Inventory and annual reports that provide insights on the use of PETs in government data-sharing contexts.
To promote transparency and shared learning, agencies should share the reports produced as part of their PET deployments and associated decision-making processes with OMB. Using these reports, OMB should (1) publish a federal government PET Use Case Inventory (similar to the recently established Federal AI Use Case Inventory) and (2) synthesize these findings into an annual report. These findings should provide high-level insights into the decisions that are being made across agencies regarding responsible data sharing, and highlight the barriers to adoption of PETs within various government data pipelines. These reports can then be used to update the decision-making frameworks we propose that NIST should produce (Recommendation 1) and inspire further technical innovation in academia and the private sector.
Strategy Component 2. Build capacity around responsible data sharing expertise
Increasing in-depth decision-making around responsible data sharing—including the use of PETs—will require specialized expertise. While there are some government agencies with teams well-trained in these topics (e.g., the Census Bureau and its team of DP experts), expertise across government is still lacking. Hence, we propose a capacity-building initiative that increases the number of experts in responsible data sharing across government.
Recommendation 4. Announce the creation of a “Responsible Data Sharing Corps.”
We propose that the USDS create a “Responsible Data Sharing Corps” (RDSC). This team will be composed of experts in responsible data sharing practices and PETs. RDSC experts can be deployed into other government agencies as needed to support decision-making about data sharing. They may also be available for as-needed consultations with agencies to answer questions or provide guidance around PETs or other relevant areas of expertise.
Recommendation 5. Build opportunities for continuing education and training for RDSC members.
Given the evolving nature of responsible data practices, including the rapid development of PETs and other privacy and security best practices, members of the RDSC should have 20% effort reserved for continuing education and training. This may involve taking online courses or attending workshops and conferences that describe state-of-the-art PETs and other relevant technologies and methodologies.
Recommendation 6. Launch a fellowship program to maintain the RDSC’s cutting-edge expertise in deploying PETS.
Finally, to ensure that the RDSC stays at the cutting edge of relevant technologies, we propose an RDSC fellowship program similar to or part of the Presidential Innovation Fellows. Fellows may be selected from academia or industry, but should have expertise in PETs and propose a novel use of PETs in a government data-sharing context. During their one-year terms, fellows will perform their proposed work and bring new knowledge to the RDSC.
Conclusion
Data sharing has become a key priority for the government in recent years, but privacy concerns make it critical to modernize technology for responsible data use to leverage data for policymaking and transparency. PETs such as differential privacy, secure multiparty computation, and others offer a promising way forward. However, deploying PETs at a broad scale requires changing norms and increasing capacity in government. The executive branch should lead these efforts by encouraging agencies to consider PETs when making data-sharing decisions and building a “Responsible Data Sharing Corps” who can provide expertise and support for agencies in this effort. By encouraging the deployment of PETs, the government can increase fairness, utility and transparency of data while protecting itself—and its data subjects—from privacy harms.
This action-ready policy memo is part of Day One 2025 — our effort to bring forward bold policy ideas, grounded in science and evidence, that can tackle the country’s biggest challenges and bring us closer to the prosperous, equitable and safe future that we all hope for whoever takes office in 2025 and beyond.
PLEASE NOTE (February 2025): Since publication several government websites have been taken offline. We apologize for any broken links to once accessible public data.
Data sharing requires a careful balance of multiple factors, with privacy and utility being particularly important.
- Data products released without appropriate and modern privacy protection measures could facilitate abuse, as attackers can weaponize information contained in these data products against individuals, e.g., blackmail, stalking, or publicly harassing those individuals.
- On the other hand, the lack of accessible data can also cause harm due to reduced utility: various actors, such as state and local government entities, may have limited access to accurate or granular data, resulting in the inefficient allocation of resources to small or marginalized communities.
Privacy-Enhancing Technologies is a broad umbrella category that includes many different technical tools. Leading examples of these tools include differential privacy, secure multiparty computation, trusted execution environments, and federated learning. Each one of these technologies is designed to address different privacy threats. For additional information, we suggest the UN Guide on Privacy-Enhancing Technologies for Official Statistics and the ICO’s resources on Privacy-Enhancing Technologies.
NIST has multiple publications related to data privacy, such as the Risk Management Framework for Cybersecurity and the Privacy Framework. The report De-Identifying Government Datasets: Techniques and Governance focuses on responsible data sharing by government organizations, while the Guidelines for Evaluating Differential Privacy Guarantees provides a framework to assess the privacy protection level provided by differential privacy for any organization.
Differential privacy is a framework for controlling the amount of information leaked about individuals during a statistical analysis. Typically, random noise is injected into the results of the analysis to hide individual people’s specific information while maintaining overall statistical patterns in the data. For additional information, we suggest Differential Privacy: A Primer for a Non-technical Audience.
Secure multiparty computation is a technique that allows several actors to jointly aggregate information while protecting each actor’s data from disclosure. In other words, it allows parties to jointly perform computations on their data while ensuring that each party learns only the result of the computation. For additional information, we suggest Secure Multiparty Computation FAQ for Non-Experts.
There are multiple examples of PET deployments at both the federal and local levels both domestically and internationally. We list several examples below, and refer interested readers to the in-depth reports by Advisory Committee on Data for Evidence Building (report 1 and report 2):
- The Census Bureau used differential privacy in their disclosure avoidance system to release results from the 2020 decennial census data. Using differential privacy allowed the bureau to provide formal disclosure avoidance guarantees as well as precise information about the impact of this system on the accuracy of the data.
- The Boston Women’s Workforce Council (BWWC) measures wage disparities among employers in the greater Boston area using secure multiparty computation (MPC).
- The Israeli Ministry of Health publicly released its National Life Birth Registry using differential privacy.
- Privacy-preserving record linkage, a variant of secure multiparty computation, has been used experimentally by both the U.S. Department of Education and the National Center for Health Statistics. Additionally, it has been used at the county level in Allegheny County, PA.
Additional examples can also be found in the UN’s case-study repository of PET deployments.
Data-sharing projects are not new to the government, and pockets of relevant expertise—particularly in statistics, software engineering, subject matter areas, and law—already exist. Deploying PET solutions requires technical computer science expertise for building and integrating PETs into larger systems, as well as sociotechnical expertise in communicating the use of PETs to relevant parties and facilitating decision-making around critical choices.
Federation of American Scientists Welcomes Dr. Yong-Bee Lim as Associate Director of the Global Risk Team
Washington, D.C. – March 7, 2025 – The Federation of American Scientists (FAS) is pleased to welcome Dr. Yong-Bee Lim as the new Associate Director of Global Risk. In this role, Dr. Lim will help develop, organize, and implement FAS’s growing contribution in the area of catastrophic risk prevention, including on core areas of nuclear weapons, AI and national security, space and other emerging technologies.
“The role of informed, credible and engaging organizations in support of sound public policy is more important than ever” said Jon Wolfsthal, FAS Director of Global Risk. “Yong-Bee embodies what it means to be an effective policy entrepreneur and to make meaningful contributions to US and global security. We are really excited that he is now part of the FAS team.”
Dr. Lim is a recognized expert in biosecurity, emerging technologies, and converging risks through his former roles as Deputy Director of both the the Converging Risks Lab and the Janne E. Nolan Center at the Council on Strategic Risks, his research and leadership roles in academia, and through his work at key agencies (DoD, HHS/ASPR, and DoE) in the United States. He completed his Ph.D. in Biodefense from George Mason University’s Biodefense program, where he conducted critical work on understanding the safety, security, and cultural dimensions of the U.S.-based Do-It-Yourself Biology (DIYBio) community. His recent accolades include being in the inaugural fellowship class of the Editorial Fellows program at the Bulletin of the Atomic Scientists and his selection and involvement in the Emerging Leaders in Biosecurity Initiative hosted by the Johns Hopkins Center for Health Security.
“As emerging capabilities change the very contours of safety, security, and innovation, FAS has positioned itself to both highlight the global opportunities we must seize and address the global risks we must mitigate,” Lim said. “Founded in 1945, FAS continues to display thought leadership and impact because it has not forgotten its core mission: to ensure that scientific and technical expertise continue to have a seat at the policymaking table. I am honored to be part of an organization with a legacy and mission like FAS.”
ABOUT FAS
The Federation of American Scientists (FAS) works to advance progress on a broad suite of issues where science, technology, and innovation policy can deliver transformative impact, and seeks to ensure that scientific and technical expertise have a seat at the policymaking table. Established in 1945 by scientists in response to the atomic bomb, FAS continues to bring scientific rigor and analysis to address contemporary challenges. More information about FAS work at fas.org and Global Risk, here.
The Federation of American Scientists Calls on OMB to Maintain the Agency AI Use Case Inventories at Their Current Level of Detail
The federal government’s approach to deploying AI systems is a defining force in shaping industry standards, academic research, and public perception of these technologies. Public sentiment toward AI remains mixed, with many Americans expressing a lack of trust in AI systems. To fully harness the benefits of AI, the public must have confidence that these systems are deployed responsibly and enhance their lives and livelihoods.
The first Trump Administration’s AI policies clearly recognized the opportunity to promote AI adoption through transparency and public trust. President Trump’s Executive Order 13859 explicitly stated that agencies must design, develop, acquire, and use “AI in a manner that fosters public trust and confidence while protecting privacy, civil rights, civil liberties, and American values.” This commitment laid the foundation for increasing government accountability in AI use.
A major step in this direction was the AI Use Case Inventory, established under President Trump’s Executive Order 13960 and later codified in the 2023 Advancing American AI Act. The agency inventories have since become a crucial tool in fostering public trust and innovation in government AI use. Recent OMB guidance (M-24-10) has expanded its scope, standardizing AI definitions, and collecting information on potential adverse impacts. The detailed inventory enhances accountability by ensuring transparency in AI deployments, tracks AI successes and risks to improve government services, and supports AI vendors by providing visibility into public-sector AI needs, thereby driving industry innovation.
The end of 2024 marked a major leap in government transparency regarding AI use. Agency reporting on AI systems saw dramatic improvements, with federal AI inventories capturing more than 1,700 AI use cases —a 200% increase in reported use cases from the previous year. The Department of Homeland Security (DHS) alone reported 158 active AI use cases. Of these, 29 were identified as high-risk, with detailed documentation on how 24 of those use cases are mitigating potential risks. This level of disclosure is essential for maintaining public trust and ensuring responsible AI deployment.
OMB is set to release revisions to its AI guidance (M-24-10) in mid-March, presenting an opportunity to ensure that transparency remains a top priority.
To support continued transparency and accountability in government AI use, the Federation of American Scientists has written a letter urging OMB to maintain its detailed guidance on AI inventories. We believe that sustained transparency is crucial to ensuring responsible AI governance, fostering public trust, and enabling industry innovation.
A Federal Center of Excellence to Expand State and Local Government Capacity for AI Procurement and Use
The administration should create a federal center of excellence for state and local artificial intelligence (AI) procurement and use—a hub for expertise and resources on public sector AI procurement and use at the state, local, tribal, and territorial (SLTT) government levels. The center could be created by expanding the General Services Administration’s (GSA) existing Artificial Intelligence Center of Excellence (AI CoE). As new waves of AI technologies enter the market, shifting both practice and policy, such a center of excellence would help bridge the gap between existing federal resources on responsible AI and the specific, grounded challenges that individual agencies face. In the decades ahead, new AI technologies will touch an expanding breadth of government services—including public health, child welfare, and housing—vital to the wellbeing of the American people. An AI CoE federal center would equip public sector agencies with sustainable expertise and set a consistent standard for practicing responsible AI procurement and use. This resource ensures that AI truly enhances services, protects the public interest, and builds public trust in AI-integrated state and local government services.
Challenge and Opportunity
State, local, tribal, and territorial (SLTT) governments provide services that are critical to the welfare of our society. Among these: providing housing, child support, healthcare, credit lending, and teaching. SLTT governments are increasingly interested in using AI to assist with providing these services. However, they face immense challenges in responsibly procuring and using new AI technologies. While grappling with limited technical expertise and budget constraints, SLTT government agencies considering or deploying AI must navigate data privacy concerns, anticipate and mitigate biased model outputs, ensure model outputs are interpretable to workers, and comply with sector-specific regulatory requirements, among other responsibilities.
The emergence of foundation models (large AI systems adaptable to many different tasks) for public sector use exacerbates these existing challenges. Technology companies are now rapidly developing new generative AI services tailored towards public sector organizations. For example, earlier this year, Microsoft announced that Azure OpenAI Service would be newly added to Azure Government—a set of AI services that target government customers. These types of services are not specifically created for public sector applications and use contexts, but instead are meant to serve as a foundation for developing specific applications.
For SLTT government agencies, these generative AI services blur the line between procurement and development: Beyond procuring specific AI services, we anticipate that agencies will increasingly be tasked with the responsible use of general AI services to develop specific AI applications. Moreover, recent AI regulations suggest that responsibility and liability for the use and impacts of procured AI technologies will be shared by the public sector agency that deploys them, rather than just resting with the vendor supplying them.
SLTT agencies must be well-equipped with responsible procurement practices and accountability mechanisms pivotal to moving forward given the shifts across products, practice, and policy. Federal agencies have started to provide guidelines for responsible AI procurement (e.g., Executive Order 13960, OMB-M-21-06, NIST RMF). But research shows that SLTT governments need additional support to apply these resources.: Whereas existing federal resources provide high-level, general guidance, SLTT government agencies must navigate a host of challenges that are context-specific (e.g., specific to regional laws, agency practices, etc.). SLTT government agency leaders have voiced a need for individualized support in accounting for these context-specific considerations when navigating procurement decisions.
Today, private companies are promising state and local government agencies that using their AI services can transform the public sector. They describe diverse potential applications, from supporting complex decision-making to automating administrative tasks. However, there is minimal evidence that these new AI technologies can improve the quality and efficiency of public services. There is evidence, on the other hand, that AI in public services can have unintended consequences, and when these technologies go wrong, they often worsen the problems they are aimed at solving. For example, by increasing disparities in decision-making when attempting to reduce them.
Challenges to responsible technology procurement follow a historical trend: Government technology has frequently been critiqued for failures in the past decades. Because public services such as healthcare, social work, and credit lending have such high stakes, failures in these areas can have far-reaching consequences. They also entail significant financial costs, with millions of dollars wasted on technologies that ultimately get abandoned. Even when subpar solutions remain in use, agency staff may be forced to work with them for extended periods despite their poor performance.
The new administration is presented with a critical opportunity to redirect these trends. Training each relevant individual within SLTT government agencies, or hiring new experts within each agency, is not cost- or resource-effective. Without appropriate training and support from the federal government, AI adoption is likely to be concentrated in well-resourced SLTT agencies, leaving others with fewer resources (and potentially more low income communities) behind. This could lead to disparate AI adoption and practices among SLTT agencies, further exacerbating existing inequalities. The administration urgently needs a plan that supports SLTT agencies in learning how to handle responsible AI procurement and use–to develop sustainable knowledge about how to navigate these processes over time—without requiring that each relevant individual in the public sector is trained. This plan also needs to ensure that, over time, the public sector workforce is transformed in their ability to navigate complicated AI procurement processes and relationships—without requiring constant retraining of new waves of workforces.
In the context of federal and SLTT governments, a federal center of excellence for state and local AI procurement would accomplish these goals through a “hub and spoke” model. This center of excellence would serve as the “hub” that houses a small number of selected experts from academia, non-profit organizations, and government. These experts would then train “spokes”—existing state and local public sector agency workers—in navigating responsible procurement practices. To support public sector agencies in learning from each others’ practices and challenges, this federal center of excellence could additionally create communication channels for information- and resource-sharing across the state and local agencies.
Procured AI technologies in government will serve as the backbone of local public services for decades to come. Upskilling government agencies to make smart decisions about which AI technologies to procure (and which are best avoided) would not only protect the public from harmful AI systems but would also save the government money by decreasing the likelihood of adopting expensive AI technologies that end up getting dropped.
Plan of Action
A federal center of excellence for state and local AI procurement would ensure that procured AI technologies are responsibly selected and used to serve as a strong and reliable backbone for public sector services. This federal center of excellence can support both intra-agency and inter-agency capacity-building and learning about AI procurement and use—that is, mechanisms to support expertise development within a given public sector agency and between multiple public sector agencies. This federal center of excellence would not be deliberative (i.e., SLTT governments would receive guidance and support but would not have to seek approval on their practices). Rather, the goal would be to upskill SLTT agencies so they are better equipped to navigate their own AI procurement and use endeavors.
To upskill SLTT agencies through inter-agency capacity-building, the federal center of excellence would house experts in relevant domain areas (e.g., responsible AI, public interest technology, and related topics). Fellows would work with cohorts of public sector agencies to provide training and consultation services. These fellows, who would come from government, academia, and civil society, would build on their existing expertise and experiences with responsible AI procurement, integrating new considerations proposed by federal standards for responsible AI (e.g., Executive Order 13960, OMB-M-21-06, NIST RMF). The fellows would serve as advisors to help operationalize these guidelines into practical steps and strategies, helping to set a consistent bar for responsible AI procurement and use practices along the way.
Cohorts of SLTT government agency workers, including existing agency leaders, data officers, and procurement experts, would work together with an assigned advisor to receive consultation and training support on specific tasks that their agency is currently facing. For example, for agencies or programs with low AI maturity or familiarity (e.g., departments that are beginning to explore the adoption of new AI tools), the center of excellence can help navigate the procurement decision-making process, help them understand their agency-specific technology needs, draft procurement contracts, select amongst proposals, and negotiate plans for maintenance. For agencies and programs with high AI maturity or familiarity, the advisor can train the programs about unexpected AI behaviors and mitigation strategies, when this arises. These communication pathways would allow federal agencies to better understand the challenges state and local governments face in AI procurement and maintenance, which can help seed ideas for improving existing resources and create new resources for AI procurement support.
To scaffold intra-agency capacity-building, the center of excellence can build the foundations for cross-agency knowledge-sharing. In particular, it would include a communication platform and an online hub of procurement resources, both shared amongst agencies. The communication platform would allow state and local government agency leaders who are navigating AI procurement to share challenges, learned lessons, and tacit knowledge to support each other. The online hub of resources can be collected by the center of excellence and SLTT government agencies. Through the online hub, agencies can upload and learn about new responsible AI resources and toolkits (e.g., such as those created by government and the research community), as well as examples of procurement contracts that agencies themselves used.
To implement this vision, the new administration should expand the U.S. General Services Administration’s (GSA) existing Artificial Intelligence Center of Excellence (AI CoE), which provides resources and infrastructural support for AI adoption across the federal government. We propose expanding this existing AI CoE to include the components of our proposed center of excellence for state and local AI procurement and use. This would direct support towards SLTT government agencies—which are currently unaccounted for in the existing AI CoE—specifically via our proposed capacity-building model.
Over the next 12 months, the goals of expanding the AI CoE would be three-fold:
1. Develop the core components of our proposed center of excellence within the AI CoE.
- Recruit a core set of fellows with expertise in responsible AI, public interest technology, and related topics from government, academia, and civil society for a 1-2 year placement;
- Develop a centralized onboarding and training program for the fellows to set standards for responsible AI procurement and use guidelines and goals;
- Create a research strategy to streamline documentation of SLTT agencies’ on-the-ground practices and challenges for procuring new AI technologies, which could help prepare future fellows.
2. Launch collaborations for the first sample of SLTT government agencies. Focus on building a path for successful collaborations:
- Identify a small set of state and local government agencies who desire federal support in navigating AI procurement and use (e.g., deciding which AI use cases to adopt, how to effectively evaluate AI deployments through time, what organizational policies to create to help govern AI use);
- Ensure there is a clear communication pathway between the agency and their assigned fellow;
- Have each fellow and agency pair create a customized plan of action to ensure the agency is upskilled in their ability to independently navigate AI procurement and use with time.
3. Build a path for our proposed center of excellence to grow and gain experience. If the first few collaborations show strong reviews, design a scaling strategy that will:
- Incorporate the center of excellence’s core budget into future budget planning;
- Identify additional fellows for the program;
- Roll out the program to additional state and local government agencies.
Conclusion
Expanding the existing AI CoE to include our proposed federal center of excellence for AI procurement and use can help ensure that SLTT governments are equipped to make informed, responsible decisions about integrating AI technologies into public services. This body would provide necessary guidance and training, helping to bridge the gap between high-level federal resources and the context-specific needs of SLTT agencies. By fostering both intra-agency and inter-agency capacity-building for responsible AI procurement and use, this approach builds sustainable expertise, promotes equitable AI adoption, and protects public interest. This ensures that AI enhances—rather than harms—the efficiency and quality of public services. As new waves of AI technologies continue to enter the public sector, touching a breadth of services critical to the welfare of the American people, this center of excellence will help maintain high standards for responsible public sector AI for decades to come.
This action-ready policy memo is part of Day One 2025 — our effort to bring forward bold policy ideas, grounded in science and evidence, that can tackle the country’s biggest challenges and bring us closer to the prosperous, equitable and safe future that we all hope for whoever takes office in 2025 and beyond.
PLEASE NOTE (February 2025): Since publication several government websites have been taken offline. We apologize for any broken links to once accessible public data.
Federal agencies have published numerous resources to support responsible AI procurement, including the Executive Order 13960, OMB-M-21-06, NIST RMF. Some of these resources provide guidance on responsible AI development in organizations broadly, across the public, private, and non-profit sectors. For example, the NIST RMF provides organizations with guidelines to identify, assess, and manage risks in AI systems to promote the deployment of more trustworthy and fair AI systems. Others focus on public sector AI applications. For instance, the OMB Memorandum published by the Office of Management and Budget describes strategies for federal agencies to follow responsible AI procurement and use practices.
Research describes how these forms of resources often require additional skills and knowledge that make it challenging for agencies to effectively use on their own. A federal center of excellence for state and local AI procurement could help agencies learn to use these resources. Adapting these guidelines to specific SLTT agency contexts necessitates a careful task of interpretation which may, in turn, require specialized expertise or resources. The creation of this federal center of excellence to guide responsible SLTT procurement on-the-ground can help bridge this critical gap. Fellows in the center of excellence and SLTT procurement agencies can build on this existing pool of guidance to build a strong theoretical foundation to guide their practices.
The hub and spoke model has been used across a range of applications to support efficient management of resources and services. For instance, in healthcare, providers have used the hub and spoke model to organize their network of services; specialized, intensive services would be located in “hub” healthcare establishments whereas secondary services would be provided in “spoke” establishments, allowing for more efficient and accessible healthcare services. Similar organizational networks have been followed in transportation, retail, and cybersecurity. Microsoft follows a hub and spoke model to govern responsible AI practices and disseminate relevant resources. Microsoft has a single centralized “hub” within the company that houses responsible AI experts—those with expertise on the implementation of the company’s responsible AI goals. These responsible AI experts then train “spokes”—workers residing in product and sales teams across the company, who learn about best practices and support their team in implementing them.
During the training, experts would form a stronger foundation for (1) on-the-ground challenges and practices that public sector agencies grapple with when developing, procuring, and using AI technologies and (2) existing AI procurement and use guidelines provided by federal agencies. The content of the training would be taken from syntheses of prior research on public sector AI procurement and use challenges, as well as existing federal resources available to guide responsible AI development. For example, prior research has explored public sector challenges to supporting algorithmic fairness and accountability and responsible AI design and adoption decisions, amongst other topics.
The experts who would serve as fellows for the federal center of excellence would be individuals with expertise and experience studying the impacts of AI technologies and designing interventions to support more responsible AI development, procurement, and use. Given the interdisciplinary nature of the expertise required for the role, individuals should have an applied, socio-technical background on responsible AI practices, ideally (but not necessarily) for the public sector. The individual would be expected to have the skills needed to share emerging responsible AI practices, strategies, and tacit knowledge with public sector employees developing or procuring AI technologies. This covers a broad range of potential backgrounds.
For example, a professor in academia who studies how to develop public sector AI systems that are more fair and aligned with community needs may be a good fit. A socio-technical researcher in civil society with direct experience studying or developing new tools to support more responsible AI development, who has intuition over which tools and practices may be more or less effective, may also be a good candidate. A data officer in a state government agency who has direct experience procuring and governing AI technologies in their department, with an ability to readily anticipate AI-related challenges other agencies may face, may also be a good fit. The cohort of fellows should include a balanced mix of individuals coming from government, academia, and civil society.
Strengthening Information Integrity with Provenance for AI-Generated Text Using ‘Fuzzy Provenance’ Solutions
Synthetic text generated by artificial intelligence (AI) can pose significant threats to information integrity. When users accept deceptive AI-generated content—such as large-scale false social media posts by malign foreign actors—as factual, national security is put at risk. One way to help mitigate this danger is by giving users a clear understanding of the provenance of the information they encounter online.
Here, provenance refers to any verifiable indication of whether text was generated by a human or by AI, for example by using a watermark. However, given the limitations of watermarking AI-generated text, this memo also introduces the concept of fuzzy provenance, which involves identifying exact text matches that appear elsewhere on the internet. As these matches will not always be available, the descriptor “fuzzy” is used. While this information will not always establish authenticity with certainty, it offers users additional clues about the origins of a piece of text.
To ensure platforms can effectively provide this information to users, the National Institute of Standards and Technology (NIST)’s AI Safety Institute should develop guidance on how to display to users both provenance and fuzzy provenance—where available—within no more than one click. To expand the utility of fuzzy provenance, NIST could also issue guidance on how generative AI companies could allow the records of their free AI models to be crawled and indexed by search engines, thereby making potential matches to AI-generated text easier to discover. Tradeoffs surrounding this approach are explored further in the FAQ section.
By creating a reliable, user-friendly framework for surfacing these details, NIST would empower readers to better discern the trustworthiness of the text they encounter, thereby helping to counteract the risks posed by deceptive AI-generated content.
Challenge and Opportunity
Synthetic Text and Information Integrity
In the past two years, generative AI models have become widely accessible, allowing users to produce customized text simply by providing prompts. As a result, there has been a rapid proliferation of “synthetic” text—AI-generated content—across the internet. As NIST’s Generative Artificial Intelligence Profile notes, this means that there is a “[l]owered barrier of entry to generated text that may not distinguish fact from opinion or fiction or acknowledge uncertainties, or could be leveraged for large scale dis- and mis-information campaigns.”
Information integrity risks stemming from synthetic text—particularly when generated for non-creative purposes—can pose a serious threat to national security. For example, in July 2024 the Justice Department disrupted Russian generative-AI-enabled disinformation bot farms. These Russian bots produced synthetic text, including in the form of social media posts by fake personas, meant to promote messages aligned with the interests of the Russian government.
Provenance Methods For Reducing Information Integrity Risks
NIST has an opportunity to provide community guidance to reduce the information integrity risks posed by all types of synthetic content. The main solution currently being considered by NIST for reducing the risks of synthetic content in general is provenance, which refers to whether a piece of content was generated by AI or a human. As described by NIST, provenance is often ascertained by creating a non-fungible watermark, or a cryptographic signature for a piece of content. The watermark is permanently associated with the piece of content. Where available, provenance information is helpful because knowing the origin of text can help a user know whether to rely on the facts it contains. For example, an AI-generated news report may currently be less trustworthy than a human news report because the former is more prone to fabrications.
However, there are currently no methods widely accepted as effective for determining the provenance of synthetic text. As NIST’s report, Reducing Risks Posed by Synthetic Content, details, “[t]he effectiveness of synthetic text detection is subject to ongoing debate” (Sec. 3.2.2.4). Even if a piece of text is originally AI-generated with a watermark (e.g., by generating words with a unique statistical pattern), people can easily copy a piece of text by paraphrasing (especially via AI), without transferring the original watermark. Text watermarks are also vulnerable to adversarial attacks, with malicious actors able to mimic the watermark signature and make text appear watermarked when it is not.
Plan of Action
To capture the benefits of provenance, while mitigating some of its weaknesses, NIST should issue guidance on how platforms can make available to users both provenance and “fuzzy provenance” of text. Fuzzy provenance is coined here to refer to exact text matches on the internet, which can sometimes reflect provenance but not necessarily (thus “fuzzy”). Optionally, NIST could also consider issuing guidance on how generative AI companies can make their free models’ records available to be crawled and indexed by search engines, so that fuzzy provenance information would show text matches with generative AI model records. There are tradeoffs to this recommendation, which is why it is optional; see FAQs for further discussion. Making both provenance and fuzzy provenance information available (in no more than one click) will give users more information to help them evaluate how trustworthy a piece of text is and reduce information integrity risks.
Combined Provenance and Fuzzy Provenance Approach
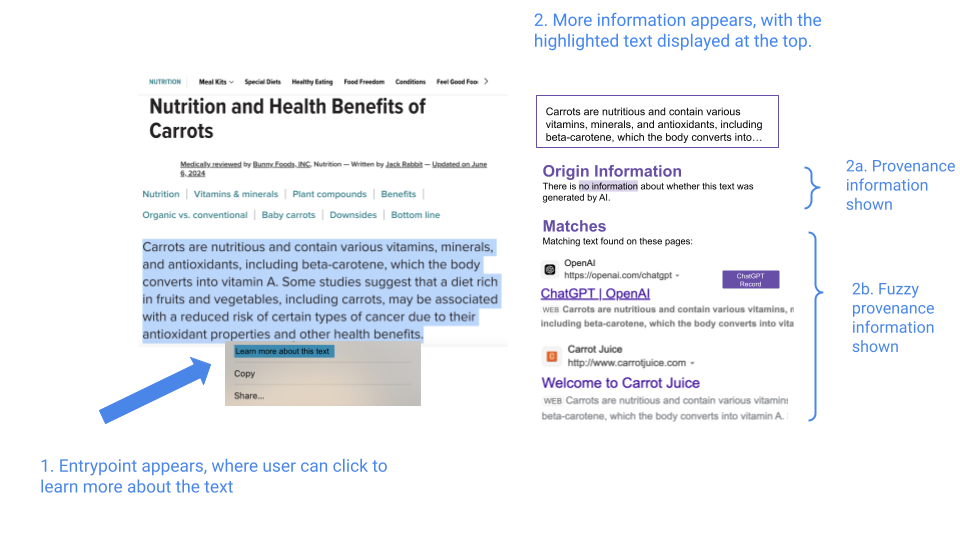
Figure 1. Mock implementation of combined provenance and fuzzy provenance
The above image captures what an implementation of the combined provenance and fuzzy provenance guidance might include. When a user highlights a piece of text that is sufficiently long, they can click “learn more about this text” to find more information.
There are ways to communicate provenance and fuzzy provenance so that it is both useful and easy-to-understand. In this concept showing the provenance of text, for example:
- Provenance information is shown under the heading “Origin Information”. This includes whether there is conclusive metadata (like watermarks) showing the text was generated by AI, according to C2PA standards, where available.
- Fuzzy provenance information is shown under the heading “Matches,” and includes other websites that have an exact text match to the highlighted text, similar to the results that come up when using a search engine. Furthermore, depending on NIST guidance, generative AI companies could have their free models’ records available to be crawled and indexed by search engines. This would enable these records to appear in the results if they contain an exact text match. These records would be clearly labeled (e.g., “ChatGPT Record”) and ranked at the top.
- See the following video for what this might look like for a user: user journeys.
Benefits of the Combined Approach
Showing both provenance and fuzzy provenance information provides users with critical context to evaluate the trustworthiness of a piece of text. Between provenance and fuzzy provenance, users would have access to information about many pieces of high-impact text, especially claims that could be particularly harmful for individuals, groups, or society at large. Making all this information immediately available also reduces friction for users so that they can get this information right where they encounter text.
Provenance information can be helpful to provide to users when it is available. For instance, knowing that a tech support company’s website description was AI-generated may encourage users to check other sources (like reviews) to see if the company is a real entity (and AI was used just to generate the description) or a fake entity entirely, before giving a deposit to hire the company (see user journey 1 in this video for an example).
Where clear provenance information is not available, fuzzy provenance can help fill the gap by providing valuable context to users in several ways:
- First, if there is no exact text match on the internet, it may indicate that the content is original—whether AI-generated or human-created—which can be especially relevant for assessing the trustworthiness of certain documents (see user journey 2 in this video for an example).
- Second, when presented with a misleading claim like “ginger is 10000x more effective than chemotherapy”, seeing that the text matches are from fact-check sites and unreliable sources such as other social media posts can encourage users to further investigate that claim (see user journey 3 in this video for an example).
- Third, absent any text matches from reliable sources, knowing that a claim (e.g., about carrots and cancer) matched an AI model’s record may cause a user to be skeptical, as they may realize that the text has the potential to be false content generated by AI (see user journey 4 in this video for an example).
Fuzzy provenance is also effective because it shows context and gives users autonomy to decide how to interpret that context. Academic studies have found that users tend to be more receptive when presented with further information they can use for their own critical thinking compared to being shown a conclusion directly (like a label), which can even backfire or be misinterpreted. This is why users may trust contextual methods like crowdsourced information more than provenance labels.
Finally, fuzzy provenance methods are generally feasible at scale, since they can be easily implemented with existing search engine capabilities (via an exact text match search). Furthermore, since fuzzy provenance only relies on exact text matching with other sources on the internet, it works without needing coordination among text-producers or compliance from bad actors.
Conclusion
To reduce the information integrity risks posed by synthetic text in a scalable and effective way, the National Institute for Standards and Technology (NIST) should develop community guidance on how platforms hosting text-based digital content can make accessible (in no more than one click) the provenance and “fuzzy provenance” of the piece of text, when available. NIST should also consider issuing guidance on how AI companies could make their free generative AI records available to be crawled by search engines, to amplify the effectiveness of “fuzzy provenance”.
This action-ready policy memo is part of Day One 2025 — our effort to bring forward bold policy ideas, grounded in science and evidence, that can tackle the country’s biggest challenges and bring us closer to the prosperous, equitable and safe future that we all hope for whoever takes office in 2025 and beyond.
PLEASE NOTE (February 2025): Since publication several government websites have been taken offline. We apologize for any broken links to once accessible public data.
Making free generative AI records available to be crawled by search engines includes tradeoffs, which is why it is an optional recommendation to consider. Below are some questions regarding implementation guidance, and trade offs including privacy and proprietary information.
Guidance could instruct AI model companies how to make their free generative AI conversation records available to be crawled and indexed by search engines. Similar to ChatGPT logs or Perplexity threads, a unique URL would be created for each conversation, capturing the date it occurred. The key difference is that all free model conversation records would be made available, but only with the AI outputs of the conversation, after removing personally identifiable information (PII) (see “Privacy considerations” section below). Because users can already choose to share conversations with each other (meaning the conversation logs are retained), and conversation logs for major model providers do not currently appear to have an expiration date, this requirement shouldn’t impose an additional storage burden for AI model companies.
Guidance could instruct search engines how to crawl and index these model logs so that queries with exact text matches to the AI outputs would surface the appropriate model logs. This would not be very different from search engines crawling/indexing other types of new URLs and should be well-within existing search engine capabilities. In terms of storage, since only free model logs would be crawled and indexed, and most free models rate-limit the number of user messages allowed, storage should also not be a concern. For instance, even with 200 million weekly active users for ChatGPT, the number of conversations in a year would only be on the order of billions, which is well-within the current scale that existing search engines have to operate to enable users to “search the web”.
- Output filtering on the AI outputs should be done to remove any personal identifiable information (PII) present in the model’s responses. However, it might still be possible to extrapolate who the original user was just by looking at the AI outputs taken together and inferring some of the user prompts. This is a privacy concern that should be further investigated. Some possible mitigations include additionally removing any location references of a certain granularity (i.e. removing mentions of neighborhoods, but retaining mentions of states) and presenting AI responses in the conversation in a randomized order.
- Removals should be made possible by a user-initiated process demonstrating privacy concerns, similar to existing search engine removal protocols.
- User consent would also be an important consideration here. NIST could propose that free model users must “opt-in”, or that free model record crawling/indexing be “opt-out” by default for users, though this may greatly compromise the reliability of fuzzy provenance.
- Training on AI-generated text: AI companies are concerned about accidentally picking up on too much AI-generated text on the web and training on that instead of higher human-generated text, thus degrading the quality of their own generative models. However, because they would have identifiable domain prefixes (ie chatgpt.com, perplexity.ai), it would be easy to exclude these AI-generated conversation logs if desired during training. Indeed, provenance and fuzzy provenance may help AI companies avoid unintentionally training on AI-generated text.
- Sharing model outputs: On the flipside, AI companies might be concerned that making so many AI-generated model outputs available for competitors to access may result in helping competitors improve their own models. This is a fair concern, though partially mitigated by a) specific user inputs are not available, and only the AI outputs; and b) only free model outputs would be logged, rather than any premium models, thus providing some proprietary protection. However, it is still possible that competitors may be able to enhance their own responses by training on the structure of AI outputs from other models at scale.
Blank Checks for Black Boxes: Bring AI Governance to Competitive Grants
The misuse of AI in federally-funded projects can risk public safety and waste taxpayer dollars.
The Trump administration has a pivotal opportunity to spot wasteful spending, promote public trust in AI, and safeguard Americans from unchecked AI decisions. To tackle AI risks in grant spending, grant-making agencies should adopt trustworthy AI practices in their grant competitions and start enforcing them against reckless grantees.
Federal AI spending could soon skyrocket. One ambitious legislative plan from a Senate AI Working Group calls for doubling non-defense AI spending to $32 billion a year by 2026. That funding would grow AI across R&D, cybersecurity, testing infrastructure, and small business support.
Yet as federal AI investment accelerates, safeguards against snake oil lag behind. Grants can be wasted on AI that doesn’t work. Grants can pay for untested AI with unknown risks. Grants can blur the lines of who is accountable for fixing AI’s mistakes. And grants offer little recourse to those affected by an AI system’s flawed decisions. Such failures risk exacerbating public distrust of AI, discouraging possible beneficial uses.
Oversight for federal grant spending is lacking, with:
- No AI-specific policies vetting discretionary grants
- Varying AI expertise on grant judging panels
- Unclear AI quality standards set by grantmaking agencies
- Little-to-no pre- or post-award safeguards that identify and monitor high-risk AI deployments.
Watchdogs, meanwhile, play a losing game, chasing after errant programs one-by-one only after harm has been done. Luckily, momentum is building for reform. Policymakers recognize that investing in untrustworthy AI erodes public trust and stifles genuine innovation. Steps policymakers could take include setting clear AI quality standards, training grant judges, monitoring grantee’s AI usage, and evaluating outcomes to ensure projects achieve their potential. By establishing oversight practices, agencies can foster high-potential projects for economic competitiveness, while protecting the public from harm.
Challenge and Opportunity
Poor AI Oversight Jeopardizes Innovation and Civil Rights
The U.S. government advances public goals in areas like healthcare, research, and social programs by providing various types of federal assistance. This funding can go to state and local governments or directly to organizations, nonprofits, and individuals. When federal agencies award grants, they typically do so expecting less routine involvement than they would with other funding mechanisms, for example cooperative agreements. Not all federal grants look the same—agencies administer mandatory grants, where the authorizing statute determines who receives funding, and competitive grants (or “discretionary grants”), where the agency selects award winners. In competitive grants, agencies have more flexibility to set program-specific conditions and award criteria, which opens opportunities for policymakers to structure how best to direct dollars to innovative projects and mitigate emerging risks.
These competitive grants fall short on AI oversight. Programmatic policy is set in cross-cutting laws, agency-wide policies, and grant-specific rules; a lack of AI oversight mars all three. To date, no government-wide AI regulation extends to AI grantmaking. Even when President Biden’s 2023 AI Executive Order directed agencies to implement responsible AI practices, the order’s implementing policies exempted grant spending (see footnote 25) entirely from the new safeguards. In this vacuum, the 26 grantmaking agencies are on their own to set agency-wide policies. Few have. Agencies can also set AI rules just for specific funding opportunities. They do not. In fact, in a review of a large set of agency discretionary grant programs, only a handful of funding notices announced a standard for AI quality in a proposed program. (See: One Bad NOFO?) The net result? A policy and implementation gap for the use of AI in grant-funded programs.
Funding mistakes damage agency credibility, stifle innovation, and undermines the support for people and communities financial assistance aims to provide. Recent controversies highlight how today’s lax measures—particularly in setting clear rules for federal financial assistance, monitoring how they are used, and responding to public feedback—have led to inefficient and rights-trampling results. In just the last few years, some of the problems we have seen include:
- The Department of Housing and Urban Development set few rules on a grant to protect public housing residents, letting officials off the hook when they bought facial recognition cameras to surveil and evict residents.
- Senators called for a pause on predictive policing grants, finding the Department of Justice failed to check that their grantees’ use of AI complied with civil rights laws—the same laws on which the grant awards were conditioned.
- The National Institute of Justice issued a recidivism forecasting challenge which researchers argued “incentivized exploiting the metrics used to judge entrants, leading to the development of trivial solutions that could not realistically work in practice.”
Any grant can attract controversy, and these grants are no exception. But the cases above spotlight transparency, monitoring, and participation deficits—the same kinds of AI oversight problems weakening trust in government that policymakers aim to fix in other contexts.
Smart spending depends on careful planning. Without it, programs may struggle to drive innovation or end up funding AI that infringes peoples’ rights. OMB, as well as agency Inspectors General, and grant managers will need guidance to evaluate what money is going towards AI and how to implement effective oversight. Government will face tradeoffs and challenges promoting AI innovation in federal grants, particularly due to:
1) The AI Screening Problem. When reviewing applications, agencies might fail to screen out candidates that exaggerate their AI capabilities—or fail to report bunk AI use altogether. Grantmaking requires calculated risks on ideas that might fail. But grant judges who are not experts in AI can make bad bets. Applicants will pitch AI solutions directly to these non-experts, and grant winners, regardless of their original proposal, will likely purchase and deploy AI, creating additional oversight challenges.
2) The grant-procurement divide. When planning a grant, agencies might set overly burdensome restrictions that dissuade qualified applicants from applying or otherwise take up too much time, getting in the way of grant goals. Grants are meant to be hands-off; fostering breakthroughs while preventing negligence will be a challenging needle to thread.
3) Limited agency capacity. Agencies may be unequipped to monitor grant recipients’ use of AI. After awarding funding, agencies can miss when vetted AI breaks down on launch. While agencies audit grantees, those audits typically focus on fraud and financial missteps. In some cases, agencies may not be measuring grantee performance well at all (slides 12-13). Yet regular monitoring, similar to the oversight used in procurement, will be necessary to catch emergent problems that affect AI outcomes. Enforcement, too, could be cause for concern; agencies clawback funds for procedural issues, but “almost never withhold federal funds when grantees are out of compliance with the substantive requirements of their grant statutes.” Even as the funding agency steps away, an inaccurate AI system can persist, embedding risks over a longer period of time.
Plan of Action
Recommendation 1. OMB and agencies should bake-in pre-award scrutiny through uniform requirements and clearer guidelines
- Agencies should revise funding notices to require applicants disclose plans to use AI, with a greater level of disclosure required for funding in a foreseeable high-risk context. Agencies should take care not to overburden applicants with disclosures on routine AI uses, particularly as the tools grow in popularity. States may be a laboratory to watch for policy innovation; Illinois, for example, has proposed AI disclosure policies and penalties for its state grants.
- Agencies should make AI-related grant policies clearer to prospective applicants. Such a change would be consistent with OMB policy and the latest Uniform Guidance, the set of rules OMB sets for agencies to manage their grants. For example, in grant notices, any AI-related review criteria should be plainly stated, rather than inferred from a project’s description. Any AI restrictions should be spelled out too, not merely incorporated by reference. More generally, agencies should consider simplifying grant notices, publishing yearly AI grant priorities, hosting information sessions, and/or extending public comment periods to significant AI-related discretionary spending.
- Agencies could consider generally-applicable metrics with which to evaluate applicants’ suggested AI uses. For example, agencies may require applicants to demonstrate they have searched for less discriminatory algorithms in the development of an automated system.
- OMB could formally codify pre-award AI risk assessments in the Uniform Guidance, the set of rules OMB sets for agencies to manage their grants. OMB updates its guidance periodically (with the most recent updates in 2024) and can also issue smaller revisions.
- Agencies could also provide resources targeted at less established AI developers, who might otherwise struggle to meet auditing and compliance standards.
Recommendation 2. OMB and grant marketplaces should coordinate information sharing between agencies
To support review of AI-related grants, OMB and grantmaking agency staff should pool knowledge on AI’s tricky legal, policy, and technical matters.
- OMB, through its Council on Federal Financial Assistance, should coordinate information-sharing between grantmaking agencies on AI risks.
- The White House Office of Science and Technology Policy, the National Institute of Standards and Technology, and the Administrative Conference of the United States (ACUS) should support agencies by devising what information agencies should collect on their grantee’s use of AI so as to limit administrative burden on grantees.
- OMB can share expertise on monitoring and managing grant risks, best practices and guides, and trade offs; other relevant interagency councils can share grant evaluation criteria and past performance templates across agencies.
- Online grants marketplaces, such as the Grants Quality Service Management Office Marketplace operated by the Department of Health and Human Services, should improve grantmakers’ decisions by sharing AI-specific information like an applicants’ AI quality, audit and enforcement history, where applicable.
Recommendation 3. Agencies should embrace targeted hiring and talent exchanges for grant review boards
Agencies should have experts in a given AI topic judging grant competitions. To do so requires overcoming talent acquisition challenges. To that end:
- Agency Chief AI Officers should assess grant office needs as part of their OMB-required assessments on AI talent. Those officers should also include grant staff in any AI trainings, and, when prudent, agency-wide risk assessment meetings
- Agencies should staff review boards with technical experts through talent exchanges and targeted hiring.
- OMB should coordinate drop-in technical experts who can sit in as consultants across agencies.
- OMB should support the training of federal grants staff on matters that touch AI– particularly as surveyed grant managers see training as an area of need.
Recommendation 4. Agencies should step up post-award monitoring and enforcement
You can’t improve what you don’t measure—especially when it comes to AI. Quantifying, documenting, and enforcing against careless AI uses can be a new task for grantmaking agencies. Incident reporting will improve the chances that existing cross-cutting regulations, including civil rights laws, can reel back AI gone awry.
- Congress can delegate investigative authority to agencies with AI audit expertise. Such an effort might mirror the cross-agency approach taken by the Department of Justice’s Procurement Collusion Strike Force, which investigates antitrust crimes in procurement and grantmaking.
- Congress can require agencies to cut off funds when grantees show repeated or egregious violations of grant terms pertaining to AI use. Agencies, where authorized, should voluntarily enforce against repeat bad players through spending clawbacks, cutoffs or ban lists.
- Agencies should consider introducing dispute resolution procedures that give redress to enforced-upon grantees.
Recommendation 5. Agencies should encourage and fund efforts to investigate and measure AI harms
- Agencies should invest in establishing measurement and standards within their topic areas on which to evaluate prospective applicants. For example, the National Institute of Justice recently opened funding to research evaluating the use of AI in the criminal legal system.
- Agencies should follow through on longstanding calls to encourage public whistleblowing on grantee missteps, particularly around AI.
- Agencies should solicit feedback from the public through RFIs on grants.gov on how to innovate in AI in their specific research or topic area.
Conclusion
Little limits how grant winners can spend federal dollars on AI. With the government poised to massively expand its spending on AI, that should change.
The federal failure to oversee AI use in grants erodes public trust, civil rights, effective service delivery and the promise of government-backed innovation. Congressional efforts to remedy these problems–starting probes, drafting letters–are important oversight measures, but only come after the damage is done.
Both the Trump and Biden administrations have recognized that AI is exceptional and needs exceptional scrutiny. Many of the lessons learned from scrutinizing federal agency AI procurement apply to grant competitions. Today’s confluence of public will, interest, and urgency is a rare opportunity to widen the aperture of AI governance to include grantmaking.
This action-ready policy memo is part of Day One 2025 — our effort to bring forward bold policy ideas, grounded in science and evidence, that can tackle the country’s biggest challenges and bring us closer to the prosperous, equitable and safe future that we all hope for whoever takes office in 2025 and beyond.
PLEASE NOTE (February 2025): Since publication several government websites have been taken offline. We apologize for any broken links to once accessible public data.
Enabling statutes for agencies often are the authority for grant competitions. For grant competitions, the statutory language leaves it to agencies to place further specific policies on the competition. Additionally, laws, like the DATA Act and Federal Grant and Cooperative Agreement Act, offer definitions and guidance to agencies in the use of federal funds.
Agencies already conduct a great deal of pre-award planning to align grantmaking with Executive Orders. For example, in one survey of grantmakers, a little over half of respondents updated their pre-award processes, such as applications and organization information, to comply with an Executive Order. Grantmakers aligning grant planning with the Trump administration’s future Executive Orders will likely follow similar steps.
A wide range of states, local governments, companies, and individuals receive grant competition funds. Spending records, available on USASpending.gov, give some insight into where grant funding goes, though these records too, can be incomplete.
Fighting Fakes and Liars’ Dividends: We Need To Build a National Digital Content Authentication Technologies Research Ecosystem
The U.S. faces mounting challenges posed by increasingly sophisticated synthetic content. Also known as digital media ( images, audio, video, and text), increasingly, these are produced or manipulated by generative artificial intelligence (AI). Already, there has been a proliferation in the abuse of generative AI technology to weaponize synthetic content for harmful purposes, such as financial fraud, political deepfakes, and the non-consensual creation of intimate materials featuring adults or children. As people become less able to distinguish between what is real and what is fake, it has become easier than ever to be misled by synthetic content, whether by accident or with malicious intent. This makes advancing alternative countermeasures, such as technical solutions, more vital than ever before. To address the growing risks arising from synthetic content misuse, the National Institute of Standards and Technology (NIST) should take the following steps to create and cultivate a robust digital content authentication technologies research ecosystem: 1) establish dedicated university-led national research centers, 2) develop a national synthetic content database, and 3) run and coordinate prize competitions to strengthen technical countermeasures. In turn, these initiatives will require 4) dedicated and sustained Congressional funding of these initiatives. This will enable technical countermeasures to be able to keep closer pace with the rapidly evolving synthetic content threat landscape, maintaining the U.S.’s role as a global leader in responsible, safe, and secure AI.
Challenge and Opportunity
While it is clear that generative AI offers tremendous benefits, such as for scientific research, healthcare, and economic innovation, the technology also poses an accelerating threat to U.S. national interests. Generative AI’s ability to produce highly realistic synthetic content has increasingly enabled its harmful abuse and undermined public trust in digital information. Threat actors have already begun to weaponize synthetic content across a widening scope of damaging activities to growing effect. Project losses from AI-enabled fraud are anticipated to reach up to $40 billion by 2027, while experts estimate that millions of adults and children have already fallen victim to being targets of AI-generated or manipulated nonconsensual intimate media or child sexual abuse materials – a figure that is anticipated to grow rapidly in the future. While the widely feared concern of manipulative synthetic content compromising the integrity of the 2024 U.S. election did not ultimately materialize, malicious AI-generated content was nonetheless found to have shaped election discourse and bolstered damaging narratives. Equally as concerning is the accumulative effect this increasingly widespread abuse is having on the broader erosion of public trust in the authenticity of all digital information. This degradation of trust has not only led to an alarming trend of authentic content being increasingly dismissed as ‘AI-generated’, but has also empowered those seeking to discredit the truth, or what is known as the “liar’s dividend”.
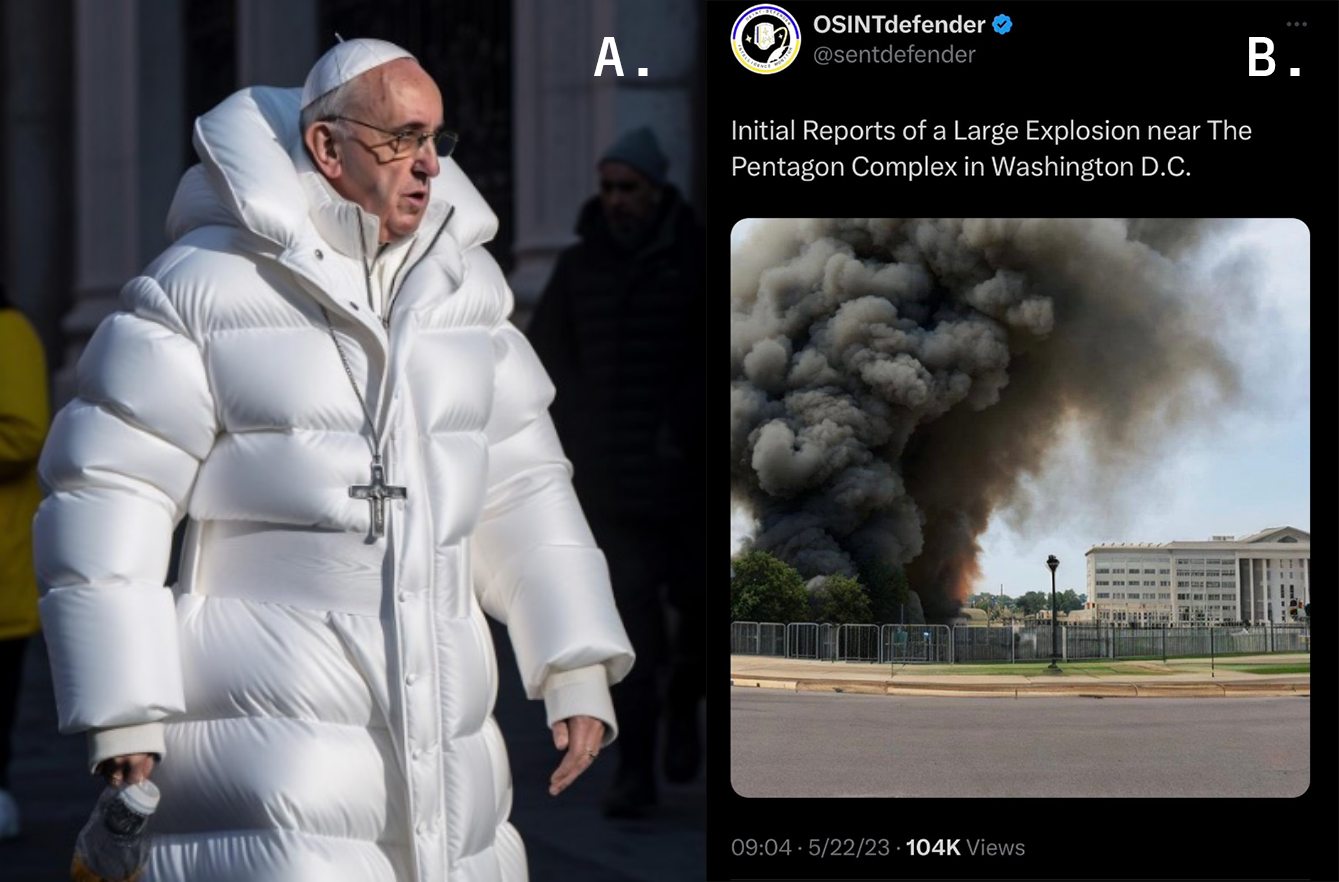
A. In March 2023, a humorous synthetic image of Pope Francis, first posted on Reddit by creator Pablo Xavier, wearing a Balenciaga coat quickly went viral across social media.
B. In May 2023, this synthetic image was duplicitously published on X as an authentic photograph of an explosion near the Pentagon. Before being debunked by authorities, the image’s widespread circulation online caused significant confusion and even led to a temporary dip in the U.S. stock market.
Research has demonstrated that current generative AI technology is able to produce synthetic content sufficiently realistic enough that people are now unable to reliably distinguish between AI-generated and authentic media. It is no longer feasible to continue, as we currently do, to rely predominantly on human perception capabilities to protect against the threat arising from increasingly widespread synthetic content misuse. This new reality only increases the urgency of deploying robust alternative countermeasures to protect the integrity of the information ecosystem. The suite of digital content authentication technologies (DCAT), or techniques, tools, and methods that seek to make the legitimacy of digital media transparent to the observer, offers a promising avenue for addressing this challenge. These technologies encompass a range of solutions, from identification techniques such as machine detection and digital forensics to classification and labeling methods like watermarking or cryptographic signatures. DCAT also encompasses technical approaches that aim to record and preserve the origin of digital media, including content provenance, blockchain, and hashing.
Evolution of Synthetic Media
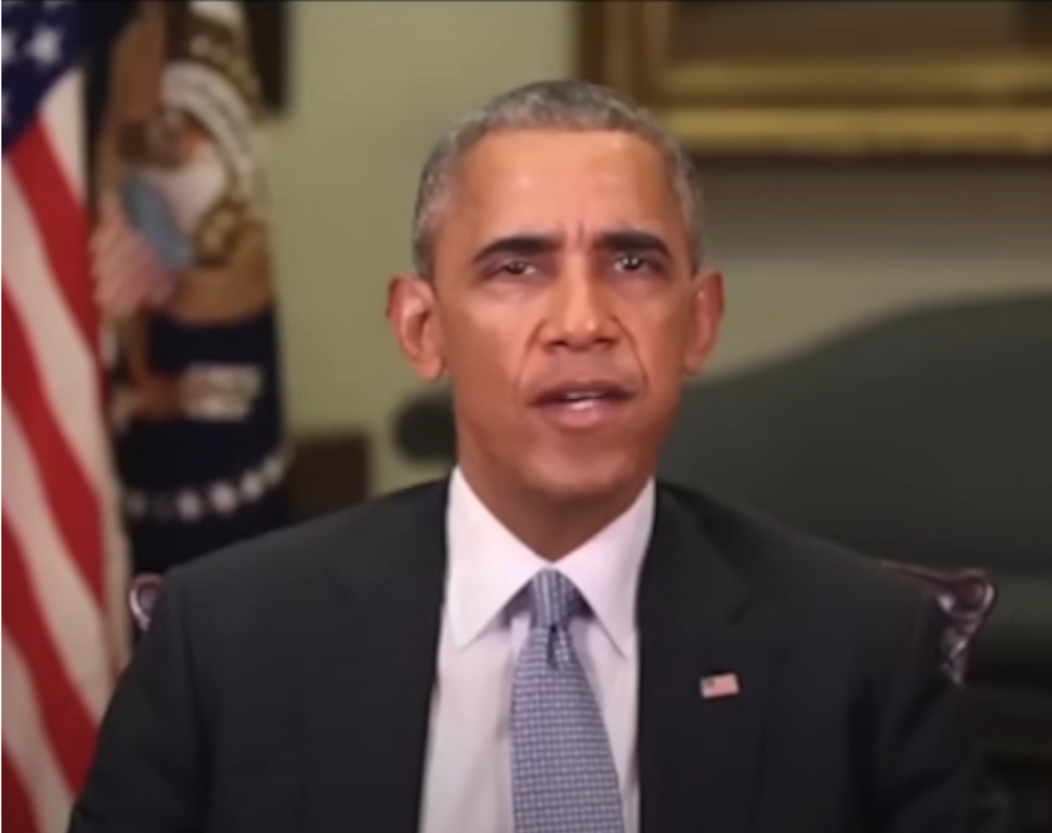
Published in 2018, this now infamous PSA sought to illustrate the dangers of synthetic content. It shows an AI-manipulated video of President Obama, using narration from a comedy sketch by comedian Jordan Peele.
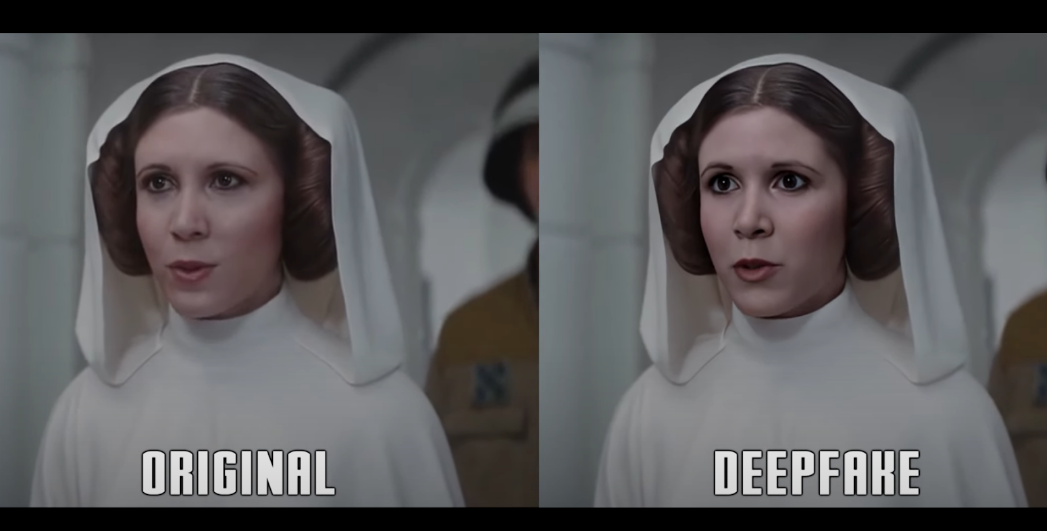
In 2020, a hobbyist creator employed an open-source generative AI model to ‘enhance’ the Hollywood CGI version of Princess Leia in the film Rouge One.
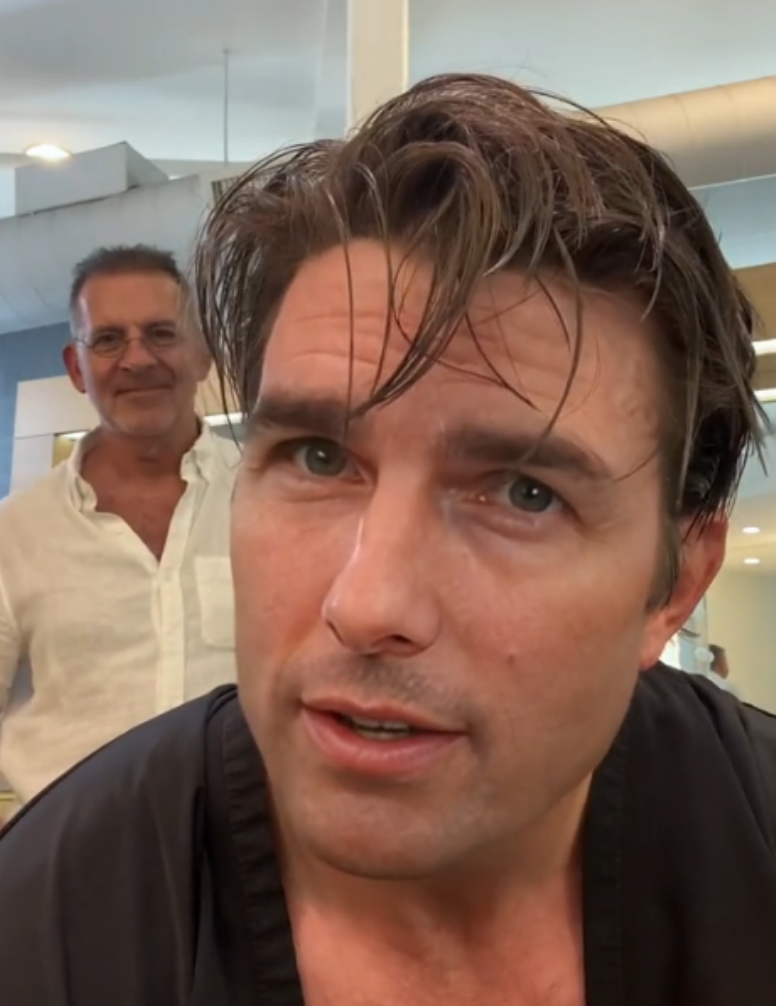
The hugely popular Tiktok account @deeptomcruise posts parody videos featuring a Tom Cruise imitator face-swapped with the real Tom Cruise’s real face, including this 2022 video, racking up millions of views.
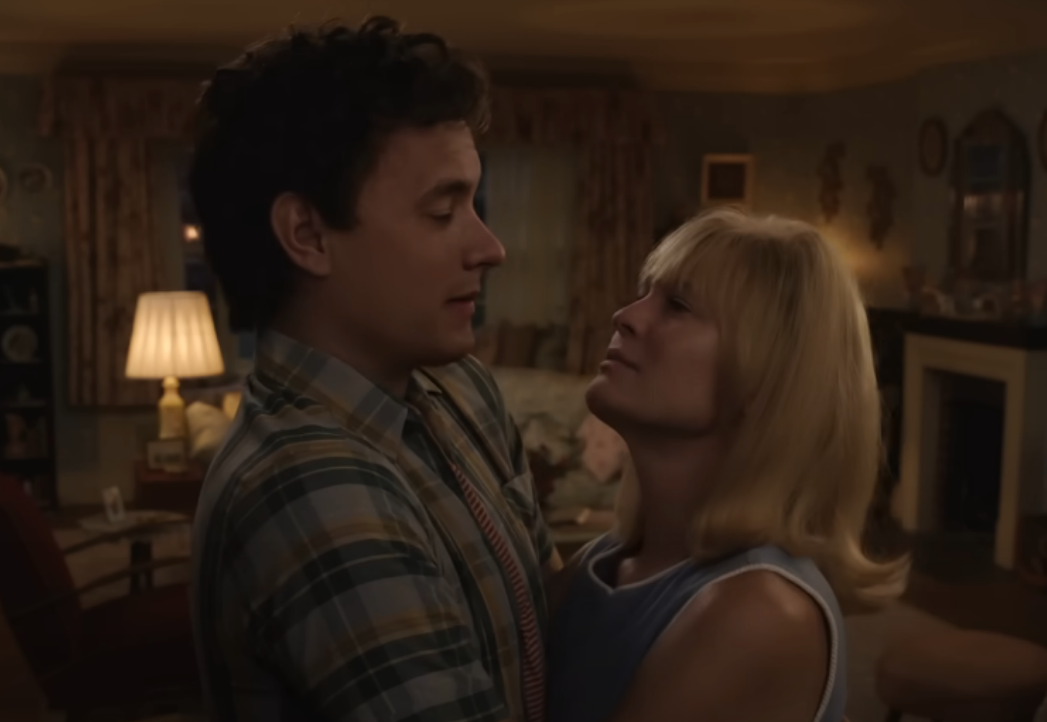
The 2024 film Here relied extensively on generative AI technology to de-age and face-swap actors in real-time as they were being filmed.
Robust DCAT capabilities will be indispensable for defending against the harms posed by synthetic content misuse, as well as bolstering public trust in both information systems and AI development. These technical countermeasures will be critical for alleviating the growing burden on citizens, online platforms, and law enforcement to manually authenticate digital content. Moreover, DCAT will be vital for enforcing emerging legislation, including AI labeling requirements and prohibitions on illegal synthetic content. The importance of developing these capabilities is underscored by the ten bills (see Fig 1) currently under Congressional consideration that, if passed, would require the employment of DCAT-relevant tools, techniques, and methods.
However, significant challenges remain. DCAT capabilities need to be improved, with many currently possessing weaknesses or limitations such brittleness or security gaps. Moreover, implementing these countermeasures must be carefully managed to avoid unintended consequences in the information ecosystem, like deploying confusing or ineffective labeling to denote the presence of real or fake digital media. As a result, substantial investment is needed in DCAT R&D to develop these technical countermeasures into an effective and reliable defense against synthetic content threats.
The U.S. government has demonstrated its commitment to advancing DCAT to reduce synthetic content risks through recent executive actions and agency initiatives. The 2023 Executive Order on AI (EO 14110) mandated the development of content authentication and tracking tools. Charged by the EO 14110 to address these challenges, NIST has taken several steps towards advancing DCAT capabilities. For example, NIST’s recently established AI Safety Institute (AISI) takes the lead in championing this work in partnership with NIST’s AI Innovation Lab (NAIIL). Key developments include: the dedication of one of the U.S. Artificial Intelligence Safety Institute Consortium’s (AISIC) working groups to identifying and advancing DCAT R&D; the publication of NIST AI 100-4, which “examines the existing standards, tools, methods, and practices, as well as the potential development of further science-backed standards and techniques” regarding current and prospective DCAT capabilities; and the $11 million dedicated to international research on addressing dangers arising from synthetic content announced at the first convening of the International Network of AI Safety Institutes. Additionally, NIST’s Information Technology Laboratory (ITL) has launched the GenAI Challenge Program to evaluate and advance DCAT capabilities. Meanwhile, two pending bills in Congress, the Artificial Intelligence Research, Innovation, and Accountability Act (S. 3312) and the Future of Artificial Intelligence Innovation Act (S. 4178), include provisions for DCAT R&D.
Although these critical first steps have been taken, an ambitious and sustained federal effort is necessary to facilitate the advancement of technical countermeasures such as DCAT. This is necessary to more successfully combat the risks posed by synthetic content—both in the immediate and long-term future. To gain and maintain a competitive edge in the ongoing race between deception and detection, it is vital to establish a robust national research ecosystem that fosters agile, comprehensive, and sustained DCAT R&D.
Plan of Action
NIST should engage in three initiatives: 1) establishing dedicated university-based DCAT research centers, 2) curating and maintaining a shared national database of synthetic content for training and evaluation, as well as 3) running and overseeing regular federal prize competitions to drive innovation in critical DCAT challenges. The programs, which should be spearheaded by AISI and NAIIL, are critical for enabling the creation of a robust and resilient U.S. DCAT research ecosystem. In addition, the 118th Congress should 4) allocate dedicated funding to supporting these enterprises.
These recommendations are not only designed to accelerate DCAT capabilities in the immediate future, but also to build a strong foundation for long-term DCAT R&D efforts. As generative AI capabilities expand, authentication technologies must too keep pace, meaning that developing and deploying effective technical countermeasures will require ongoing, iterative work. Success demands extensive collaboration across technology and research sectors to expand problem coverage, maximize resources, avoid duplication, and accelerate the development of effective solutions. This coordinated approach is essential given the diverse range of technologies and methodologies that must be considered when addressing synthetic content risks.
Recommendation 1. Establish DCAT Research Institutes
NIST should establish a network of dedicated university-based research to scale up and foster long-term, fundamental R&D on DCAT. While headquartered at leading universities, these centers would collaborate with academic, civil society, industry, and government partners, serving as nationwide focal points for DCAT research and bringing together a network of cross-sector expertise. Complementing NIST’s existing initiatives like the GenAI Challenge, the centers’ research priorities would be guided by AISI and NAIIL, with expert input from the AISIC, the International Network of AISI, and other key stakeholders.
A distributed research network offers several strategic advantages. It leverages elite expertise from industry and academia, and having permanent institutions dedicated to DCAT R&D enables the sustained, iterative development of authentication technologies to better keep pace with advancing generative AI capabilities. Meanwhile, central coordination by AISI and NAIIL would also ensure comprehensive coverage of research priorities while minimizing redundant efforts. Such a structure provides the foundation for a robust, long-term research ecosystem essential for developing effective countermeasures against synthetic content threats.
There are multiple pathways via which dedicated DCAT research centers could be stood up. One approach is direct NIST funding and oversight, following the model of Carnegie Mellon University’s AI Cooperative Research Center. Alternatively, centers could be established through the National AI Research Institutes Program, similar to the University of Maryland’s Institute for Trustworthy AI in Law & Society, leveraging NSF’s existing partnership with NIST.
The DCAT research agenda could be structured in two ways. Informed by NIST’s report NIST AI 100-4, a vertical approach could be taken to centers’ research agendas, assigning specific technologies to each center (e.g. digital watermarking, metadata recording, provenance data tracking, or synthetic content detection). Centers would focus on all aspects of a specific technical capability, including: improving the robustness and security of existing countermeasures; developing new techniques to address current limitations; conducting real-world testing and evaluation, especially in a cross-platform environment; and studying interactions with other technical safeguards and non-technical countermeasures like regulations or educational initiatives. Conversely, a horizontal approach might seek to divide research agendas across areas such as: the advancement of multiple established DACT techniques, tools, and methods; innovation of novel techniques, tools, and methods; testing and evaluation of combined technical approaches in real-world settings; examining the interaction of multiple technical countermeasures with human factors such as label perception and non-technical countermeasures. While either framework provides a strong foundation for advancing DCAT capabilities, given institutional expertise and practical considerations, a hybrid model combining both approaches is likely the most feasible option.
Recommendation 2. Build and Maintain a National Synthetic Content Database
NIST should also build and maintain a national database of synthetic content database to advance and accelerate DCAT R&D, similar to existing federal initiatives such as NIST’s National Software Reference Library and NSF’s AI Research Resource pilot. Current DCAT R&D is severely constrained by limited access to diverse, verified, and up-to-date training and testing data. Many researchers, especially in academia, where a significant portion of DCAT research takes place, lack the resources to build and maintain their own datasets. This results in less accurate and more narrowly applicable authentication tools that struggle to keep pace with rapidly advancing AI capabilities.
A centralized database of synthetic and authentic content would accelerate DCAT R&D in several critical ways. First, it would significantly alleviate the effort on research teams to generate or collect synthetic data for training and evaluation, encouraging less well-resourced groups to conduct research as well as allowing researchers to focus more on other aspects of R&D. This includes providing much-needed resources for the NIST-facilitated university-based research centers and prize competitions proposed here. Moreover, a shared database would be able to provide more comprehensive coverage of the increasingly varied synthetic content being created today, permitting the development of more effective and robust authentication capabilities. The database would be useful for establishing standardized evaluation metrics for DCAT capabilities – one of NIST’s critical aims for addressing the risks posed by AI technology.
A national database would need to be comprehensive, encompassing samples of both early and state-of-the-art synthetic content. It should have controlled laboratory-generated along with verified “in the wild” or real world synthetic content datasets, including both benign and potentially harmful examples. Further critical to the database’s utility is its diversity, ensuring synthetic content spans multiple individual and combined modalities (text, image, audio, video) and features varied human populations as well as a variety of non-human subject matter. To maintain the database’s relevance as generative AI capabilities continue to evolve, routinely incorporating novel synthetic content that accurately reflects synthetic content improvements will also be required.
Initially, the database could be built on NIST’s GenAI Challenge project work, which includes “evolving benchmark dataset creation”, but as it scales up, it should operate as a standalone program with dedicated resources. The database could be grown and maintained through dataset contributions by AISIC members, industry partners, and academic institutions who have either generated synthetic content datasets themselves or, as generative AI technology providers, with the ability to create the large-scale and diverse datasets required. NIST would also direct targeted dataset acquisition to address specific gaps and evaluation needs.
Recommendation 3. Run Public Prize Competitions on DCAT Challenges
Third, NIST should set up and run a coordinated prize competition program, while also serving as federal oversight leads for prize competitions run by other agencies. Building on existing models such as the DARPA SemaFor’s AI FORCE and the FTC’s Voice Cloning challenge, the competitions would address expert-identified priorities as informed by the AISIC, International Network of AISI, and proposed DCAT national research centers. Competitions represent a proven approach to spurring innovation for complex technical challenges, enabling the rapid identification of solutions through diverse engagement. In particular, monetary prize competitions are especially successful at ensuring engagement. For example, the 2019 Kaggle Deepfake Detection competition, which had a prize of $1 million, fielded twice as many participants as the 2024 competition, which gave no cash prize.
By providing structured challenges and meaningful incentives, public competitions can accelerate the development of critical DCAT capabilities while building a more robust and diverse research community. Such competitions encourage novel technical approaches, rapid testing of new methods, facilitate the inclusion of new or non-traditional participants, and foster collaborations. The more rapid-cycle and narrow scope of the competitions would also complement the longer-term and broader research being conducted by the national DCAT research centers. Centralized federal oversight would also prevent the implementation gaps which have occurred in past approved federal prize competitions. For instance, the 2020 National Defense Authorization Act (NDAA) authorized a $5 million machine detection/deepfakes prize competition (Sec. 5724), and the 2024 NDAA authorized a ”Generative AI Detection and Watermark Competition” (Sec. 1543). However, neither prize competition has been carried out, and Watermark Competition has now been delayed to 2025. Centralized oversight would also ensure that prize competitions are run consistently to address specific technical challenges raised by expert stakeholders, encouraging more rapid development of relevant technical countermeasures.
Some examples of possible prize competitions might include: machine detection and digital forensic methods to detect partial or fully AI-generated content across single or multimodal content; assessing the robustness, interoperability, and security of watermarking and other labeling methods across modalities; testing innovations in tamper-evident or -proofing content provenance tools and other data origin techniques. Regular assessment and refinement of competition categories will ensure continued relevance as synthetic content capabilities evolve.
Recommendation 4. Congressional Funding of DCAT Research and Activities
Finally, the 118th Congress should allocate funding for these three NIST initiatives in order to more effectively establish the foundations of a strong DCAT national research infrastructure. Despite widespread acknowledgement of the vital role of technical countermeasures in addressing synthetic content risks, the DCAT research field remains severely underfunded. Although recent initiatives, such as the $11 million allocated to the International Network of AI Safety Institutes, are a welcome step in the right direction, substantially more investment is needed. Thus far, the overall financing of DCAT R&D has been only a drop in the bucket when compared to the many billions of dollars being dedicated by industry alone to improve generative AI technology.
This stark disparity between investment in generative AI versus DCAT capabilities presents an immediate opportunity for Congressional action. To address the widening capability gap, and to support pending legislation which will be reliant on technical countermeasures such as DCAT, the 118th Congress should establish multi-year appropriations with matching fund requirements. This will encourage private sector investment and permit flexible funding mechanisms to address emerging challenges. This funding should be accompanied by regular reporting requirements to track progress and impact.
One specific action that Congress could take to jumpstart DCAT R&D investment would be to reauthorize and appropriate the budget that was earmarked for the unexecuted machine detection competition it approved in 2020. Despite the 2020 NDAA authorizing $5 million for it, no SAC-D funding was allocated, and the competition never took place. Another action would be for Congress to explicitly allocate prize money for the watermarking competition authorized by the 2024 NDAA, which currently does not have any monetary prize attached to it, to encourage higher levels of participation in the competition when it takes place this year.
Conclusion
The risks posed by synthetic content present an undeniable danger to U.S. national interests and security. Advancing DCAT capabilities is vital for protecting U.S. citizens against both the direct and more diffuse harms resulting from the proliferating misuse of synthetic content. A robust national DCAT research ecosystem is required to accomplish this. Critically, this is not a challenge that can be addressed through one-time solutions or limited investment—it will require continuous work and dedicated resources to ensure technical countermeasures keep pace alongside increasingly sophisticated synthetic content threats. By implementing these recommendations with sustained federal support and investment, the U.S. will be able to more successfully address current and anticipated synthetic content risks, further reinforcing its role as a global leader in responsible AI use.
This action-ready policy memo is part of Day One 2025 — our effort to bring forward bold policy ideas, grounded in science and evidence, that can tackle the country’s biggest challenges and bring us closer to the prosperous, equitable and safe future that we all hope for whoever takes office in 2025 and beyond.
PLEASE NOTE (February 2025): Since publication several government websites have been taken offline. We apologize for any broken links to once accessible public data.
An Agenda for Ensuring Child Safety in the AI Era
The next administration should continue to make responsible policy on Artificial intelligence (AI) and children, especially in K-12, a top priority and create an AI and Kids Initiative led by the administration. AI is transforming how children learn and live, and policymakers, industry, and educators owe it to the next generation to set in place a responsible policy that embraces this new technology while at the same time ensuring all children’s well-being, privacy, and safety is respected. The federal government should develop clear prohibitions, enforce them, and serve as a national clearinghouse for AI K-12 educational policy. It should also support comprehensive digital literacy related to AI.
Specifically, we think these policy elements need to be front of mind for decision-makers: build a coordinated framework for AI Safety; champion legislation to support youth privacy and online safety in AI; and ensure every child can benefit from the promise of AI.
In terms of building a coordinated framework for AI Safety, the next administration should: ensure parity with existing child data protections; develop safety guidance for developers, including specific prohibitions to limit harmful designs, and inappropriate uses; and direct the National Institute of Standards and Technology (NIST) to serve as the lead organizer for federal efforts on AI safety for children. When championing legislation to support youth privacy and online safety in AI, the next administration should support the passage of online safety laws that address harmful design features that can lead to medically recognized mental health disorders and patterns of use indicating addiction-like behavior, and modernize federal children’s privacy laws including updating The Family Educational Rights and Privacy Act (FERPA) and passing youth privacy laws to explicitly address AI data use issues, including prohibiting developing commercial models from students’ educational information, with strong enforcement mechanisms. And, in order to ensure every child can benefit from the promise of AI, the next administration should support comprehensive digital literacy efforts and prevent deepening the digital divide.
Importantly, policy and frameworks need to have teeth and need to take the burden off of individual states, school districts, or actors to assess AI tools for children. Enforcement should be tailored to specific laws, but should include as appropriate private rights of action, well-funded federal enforcers, and state and local enforcement. Companies should feel incentivized to act. The framework cannot be voluntary, enabling companies to pick and choose whether or not to follow recommendations.. We’ve seen what happens when we do not put in place guardrails for tech, such as increased risk of child addiction, depression and self-harm–and it should not happen again. We cannot say that this is merely a nascent technology and that we can delay the development of protections. We already know AI will critically impact our lives. We’ve watched tech critically impact lives and AI-enabled tech is both faster and potentially more extreme.
Challenge and Opportunity
AI is already embedded in children’s lives and education. According to Common Sense Media research, seven in ten teens have used generative AI, and the most common use is for help with homework. The research also found most parents are in the dark about their child’s generative AI use–only a third of parents whose children reported using generative AI were aware of such use. Beyond generative AI, machine learning systems are embedded in just about every application kids use at school and at home. Further, most teens and parents say schools have either no AI policy or have not communicated one.
Educational uses of AI are recognized to pose higher risk, according to the EU Artificial Intelligence Act and other international frameworks. The EU recognized that risk management requires special consideration when an AI system is likely to be accessed by children. The U.S. has developed a risk management framework, but the U.S. has not yet articulated risk levels or developed a specific educational or youth profile using NIST’s Risk Management Framework. There is still a deep need to ensure that AI systems likely to be accessed by children, including in schools, to be assessed in terms of risk management and impact on youth.
It is well established that children and teenagers are vulnerable to manipulation by technology. Youth report struggling to set boundaries from technology, and according to a U.S. Surgeon General report, almost a third of teens say they are on social media almost constantly. Almost half of youth say social media has reduced their attention span, and takes time away from other activities they care about. They are unequipped to assess sophisticated and targeted advertising, as most children cannot distinguish ads from content until they are at least eight years old, and most children do not realize ads can be customized. Additionally, social media design features lead, in addition to addiction, to teens suffering other mental or physical harm: from unattainable beauty filters to friend comparison to recommendation systems that promote harmful content, such as the algorithmic promotion of viral “challenges” that can lead to death. AI technology is particularly concerning given its novelness, and the speed and autonomy at which the technology can operate, and the frequent opacity even to developers of AI systems about how inputs and outputs may be used or exposed.
Particularly problematic uses of AI in products used in education and/or by children so far include products that use emotion detection, biometric data, facial recognition (built from scraping online images that include children), companion AI, automated education decisions, and social scoring. This list will continue to grow as AI is further adopted.
There are numerous useful frameworks and toolkits from expert organizations like EdSafe, and TeachAI, and from government organizations like NIST, the National Telecommunications and Information Administration (NTIA), and Department of Education (ED). However, we need the next administration to (1) encourage Congress to pass clear rules regarding AI products used with children, (2) have NIST develop risk management frameworks specifically addressing use of AI in education and by children more broadly, and serve as a clearinghouse function so individual actors and states do not bear that responsibility, and (3) ensure frameworks are required and prohibitions are enforced. This is also reflected in the lack of updated federal privacy and safety laws that protect children and teens.
Plan of Action
The federal government should take note of the innovative policy ideas bubbling up at the state level. For example, there is legislation and proposals in Colorado, California, Texas, and detailed guidance in over 20 states, including Ohio, Alabama, and Oregon.
Policymakers should take a multi-pronged approach to address AI for children and learning, recognizing they are higher risk and therefore additional layers of protection should apply:
Recommendation 1. Build a coordinated framework an AI Safety and Kids Initiative at NIST
As the federal government further details risk associated with uses of AI, common uses of AI by kids should be designated or managed as high risk. This is a foundational step to support the creation of guardrails or ensure protections for children as they use AI systems. The administration should clearly categorize education and use by children with in a risk level framework. For example, the EU is also considering risk in AI with the EU AI Act, which has different risk levels. If the risk framework includes education and AI systems that are likely to be accessed by children it provides a strong signal to policymakers at the state and federal level that these are uses that require protections (audits, transparency, or enforcement) to prevent or address potential harm.
NIST, in partnership with others, should develop risk management profiles for platform developers building AI products for use in Education and for products likely to be accessed by children. Emphasis should be on safety and efficacy before technology products come to market, with audits throughout development. NIST should:
- Develop a committee with ED,, FTC, and CPSC, to periodically update of risk management framework (RMF) profiles, including benchmarking standards related to safety.
- Refine risk levels and RMFs relevant to education, working in in partnership with NTIA and ED, through an open call to stakeholders.
Work in partnership with NTIA, FTC, CPSC, and HHS to refine risk levels and risk management profiles for AI systems likely to be accessed by children.
The administration should task NIST’s Safety Institute to provide clarity on how safety should be considered for the use of AI in education and for AI systems likely to be accessed by children. This is accomplished through:
- Developer guidance: Promulgate safety guidance for developers of AI systems likely to be accessed by children or used in education
- Procurement guidance: In collaboration with the Dept of Education to provide guidance on safety, efficacy, and privacy to support educational procurement of AI systems
- Information clearinghouse: To support state bodies and other entities developing guidance on use of AI systems by serving as a clearinghouse for information on the state of AI systems, developments in efficacy and safety, and to highlight through periodic reporting the concerns of and needs of users.
Recommendation 2. Ensure every child benefits from the promise of AI innovations
The administration should support comprehensive digital literacy and prevent a deepening of the digital divide.
- Highlighting Meaningful Use: Provide periodically updated guidance on best uses available for schools, teachers, students, and caregivers to support their use of AI technology for education.
- Support Professional Development: Dept of Ed and NSF can collaborate on Professional Development guidelines, and flag new areas for teacher training and administer funding to support educator professional development.
- Comprehensive Digital Literacy: NTIA, Dept of Ed should collaborate to administer funds for digital literacy efforts that support both students and caregivers. Digital literacy guidance should support both use and dynamically addresses concerns around current risks or safety issues as they arise.
- Clearinghouse for AI Developments: In addition to funding this work experts in government at NIST, NTIA, FTC, FCC, and Dept of Ed can work collaboratively to periodically alert and inform consumers and digital literacy organizations about developments with AI systems. Federal government can serve as a resource to alert stakeholders downstream on both positive and negative developments, for example the FCC Consumer Advisory Committee was tasked with developing recommendation with a consumer education outreach plan regarding AI generated robocalls.
Recommendation 3. Encourage Congress to pass clear enforceable rules re privacy and safety for AI products used by children
Champion Congressional updates to privacy laws like COPPA and FERPA to address use (especially for training) and sharing of personal information (PI) by AI tools. These laws can work in tandem, see for example recent proposed COPPA updates that would address use of technology in educational settings by children.
- Consumer Protections: In the consumer space, consider requirements generally prohibiting use of children’s PI for training AI models, unless deidentified or aggregated and with consent (see CA AB 2877).
- Education Protections: In education settings, it may be unclear when information about students shared with AI systems is subject to FERPA. Dept of Ed has acknowledged that educational uses of AI models may not be aligned with FERPA or state student privacy laws. FERPA should be updated to explicitly cover personal information collected by and shared with LLMs: covered education records must include this data; sharing of directory information for all purposes including AI should be limited; the statute should address when ed tech vendors operate as “school officials” and generally prohibit training AI models on student personal information.
Push for Congress to pass AI specific legislation addressing the development and deployment of AI systems for use by children
- Address High Risk Uses: Support legislation to prohibit the use of AI systems in high-risk educational contexts, or when likely to be accessed by children, unless committee-identified benchmarks are met. Use of AI in educational contexts and when accessed by children should be default deemed high risk unless it can be demonstrated otherwise. Specific examples of high risks uses in education include AI for threat detection and disciplinary uses, exam proctoring, automated grading and admissions, and generative and companion AI use by minor students.
- Require Third-Party Audits: Support legislation to require third-party audits at the application, model, and governance level, considering functionality, performance, robustness, security and privacy, safety, educational efficacy (as appropriate), accessibility, risks, and mitigation strategies.
- Require Transparency: Support legislation to require transparency reporting by AI developers.
Support Congressional passage of online safety laws that address harmful design features in technology–specifically addressing design features that can lead to medically recognized mental health disorders like anxiety, depression, eating disorders, substance use, and suicide, and patterns of use indicating addiction-like behavior, as in Title I of the Senate-passed Kids Online Safety and Privacy Act.
Moving Forward
One ultimate recommendation is that, critically, standards and requirements need teeth. Frameworks should require that companies comply with legal requirements or face effective enforcement (such as by a well-funded expert regulator, or private lawsuits), with tools such as fines and injunctions. We have seen with past technological developments that voluntary frameworks and suggestions will not adequately protect children. Social media for example has failed to voluntarily protect children and poses risks to their mental health and well being. From exacerbating body image issues to amplifying peer pressure and social comparison, from encouraging compulsive device use to reducing attention spans, from connecting youth to extremism, illegal products, and deadly challenges, the financial incentives do not appear to exist for technology companies to appropriately safeguard children on their own. The next Administration can support enforcement by funding government positions who will be enforcing such laws.
Antitrust in the AI Era: Strengthening Enforcement Against Emerging Anticompetitive Behavior
The advent of artificial intelligence (AI) has revolutionized business practices, enabling companies to process vast amounts of data and automate complex tasks in ways previously unimaginable. However, while AI has gained much praise for its capabilities, it has also raised various antitrust concerns. Among the most pressing is the potential for AI to be used in an anticompetitive manner. This includes algorithms that facilitate price-fixing, predatory pricing, and discriminatory pricing (harming the consumer market), as well as those which enable the manipulation of wages and worker mobility (harming the labor market). More troubling perhaps is the fact that the overwhelming majority of the AI landscape is controlled by just a few market players. These tech giants—some of the world’s most powerful corporations—have established a near-monopoly over the development and deployment of AI. Their dominance over necessary infrastructure and resources makes it increasingly challenging for smaller firms to compete.
While the antitrust enforcement agencies—the FTC and DOJ—have recently begun to investigate these issues, they are likely only scratching the surface. The covert and complex nature of AI makes it difficult to detect when it is being used in an anticompetitive manner. To ensure that business practices remain competitive in the era of AI, the enforcement agencies must be adequately equipped with the appropriate strategies and resources. The best way to achieve this is to (1) require the disclosure of AI technologies during the merger-review process and (2) reinforce the enforcement agencies’ technical strategy in assessing and mitigating anticompetitive AI practices.
Challenge & Opportunity
Since the late 1970s, antitrust enforcement has been in decline, in part due to a more relaxed antitrust approach put forth by the Chicago school of economics. Both the budgets and the number of full-time employees at the enforcement agencies have steadily decreased, while the volume of permitted mergers and acquisitions has risen (see Figure 1). This resource gap has limited the ability of the agencies to effectively oversee and regulate anticompetitive practices.
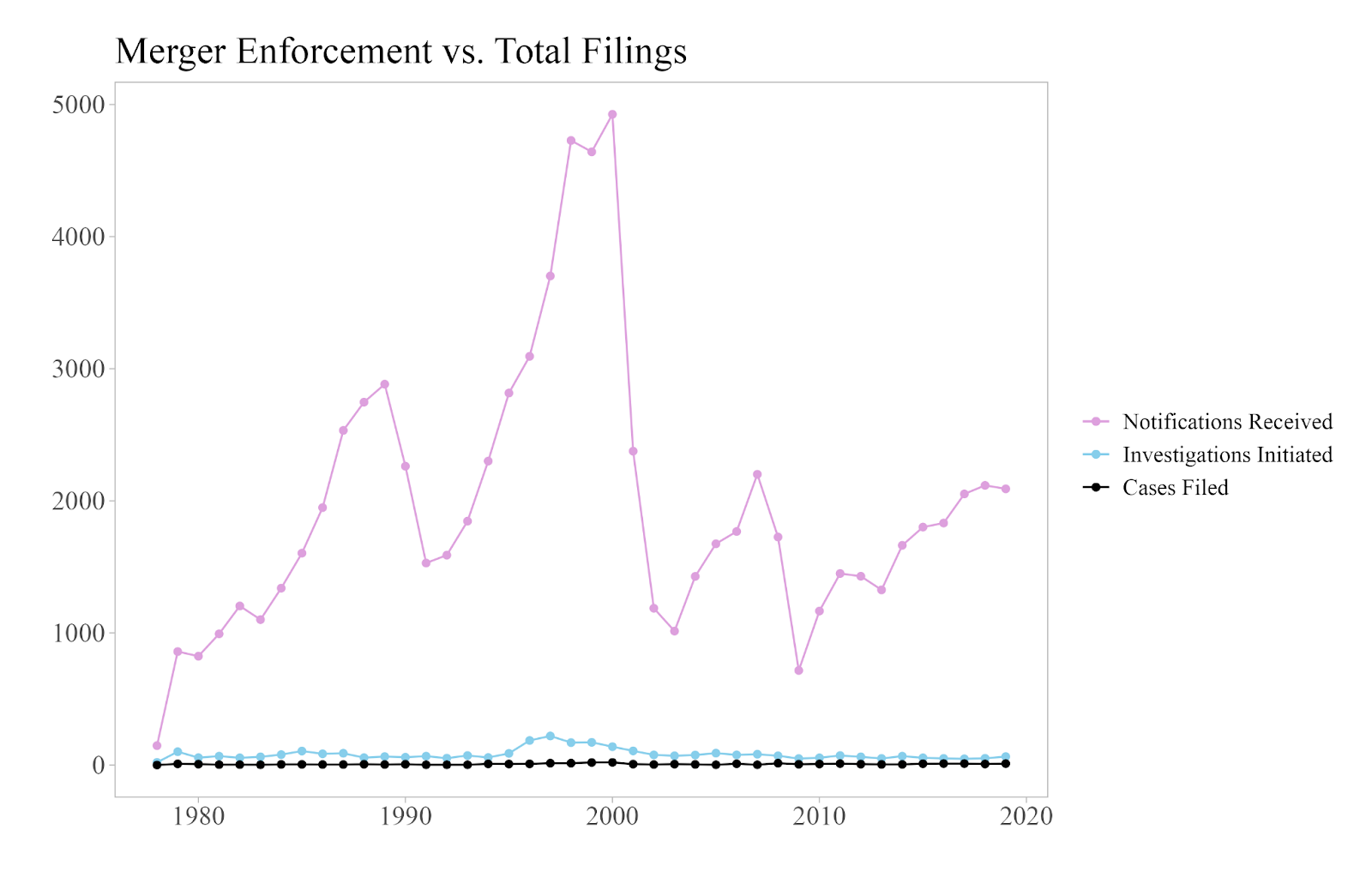
Changing attitudes surrounding big business, as well as recent shifts in leadership at the enforcement agencies—most notably President Biden’s appointment of Lina Khan to FTC Chair—have signaled a more aggressive approach to antitrust law. But even with this renewed focus, the agencies are still not operating at their full potential.
This landscape provides a significant opportunity to make some much-needed changes. Two areas for improvement stand out. First, agencies can make use of the merger review process to aid in the detection of anticompetitive AI practices. In particular, the agencies should be on the look-out for algorithms that facilitate price-fixing, where competitors use AI to monitor and adjust prices automatically, covertly allowing for tacit collusion; predatory pricing algorithms, which enable firms to undercut competitors only to later raise prices once dominance is achieved; and dynamic pricing algorithms, which allow firms to discriminate against different consumer groups, resulting in price disparities that may distort market competition. On the labor side, agencies should screen for wage-fixing algorithms and other data-driven hiring practices that may suppress wages and limit job mobility. Requiring companies to disclose the use of such AI technologies during merger assessments would allow regulators to examine and identify problematic practices early on. This is especially useful for flagging companies with a history of anticompetitive behavior or those involved in large transactions, where the use of AI could have the strongest anticompetitive effects.
Second, agencies can use AI to combat AI. Research has demonstrated that AI can be more effective in detecting anticompetitive behavior than other traditional methods. Leveraging such technology could transform enforcement capabilities by allowing agencies to cover more ground despite limited resources. While increasing funding for these agencies would be requisite, AI nonetheless provides a cost-effective solution, enhancing efficiency in detecting anticompetitive practices, without requiring massive budget increases.
The success of these recommendations hinges on the enforcement agencies employing technologists who have a deep understanding of AI. Their knowledge on algorithm functionality, the latest insights in AI, and the interplay between big data and anticompetitive behavior is instrumental. A detailed discussion of the need for AI expertise is covered in the following section.
Plan Of Action
Recommendation 1. Require Disclosure of AI Technologies During Merger-Review.
Currently, there is no formal requirement in the merger review process that mandates the reporting of AI technologies. This lack of transparency allows companies to withhold critical information that may help agencies determine potential anticompetitive effects. To effectively safeguard competition, it is essential that the FTC and DOJ have full visibility of businesses’ technologies, particularly those that may impact market dynamics. While the agencies can request information on certain technologies further in the review process, typically during the second request phase, a formalized reporting requirement would provide a more proactive approach. Such an approach would be beneficial for several reasons. First, it would enable the agencies to identify anticompetitive technologies they might have otherwise overlooked. Second, an early assessment would allow the agencies to detect and mitigate risk upfront, rather than having to address it post-merger or further along in the merger review process, when remedies may be more difficult to enforce. This is particularly applicable with regard to deep integrations that often occur between digital products post-merger. For instance, the merger of Instagram and Facebook complicated the FTC’s subsequent efforts to challenge Meta. As Dmitry Borodaenko, a former Facebook engineer, explained:
“Instagram is no longer viable outside of Facebook’s infrastructure. Over the course of six years, they integrated deeply… Undoing this would not be a simple task—it would take years, not just the click of a button.”
Lastly, given the rapidly evolving nature of AI, this requirement would help the agencies identify trends and better determine which technologies are harmful to competition, under what circumstances, and in which industries. Insights gained from one sector could inform investigations in other sectors, where similar technologies are being deployed. For example, the DOJ recently filed suit against RealPage, a property management software company, for allegedly using price-fixing algorithms to coordinate rent increases among competing landlords. The case is the first of its kind, as there had not been any previous lawsuit addressing price-fixing in the rental market. With this insight, however, if the agencies detect similar algorithms during the merger review process, they would be better equipped to intervene and prevent such practices.
There are several ways the government could implement this recommendation. To start, The FTC and DOJ should issue interpretive guidelines specifying that anticompetitive effects stemming from AI technologies are within the purview of the Hart-Scott-Rodino (HSR) Act, and that accordingly, such technologies should be disclosed in the pre-merger notification process. In particular, the agencies should instruct companies to report detailed descriptions of all AI technologies in use, how they might change post-merger, and their potential impact on competition metrics (e.g., price, market share). This would serve as a key step in signaling to companies that AI considerations are integral during merger review. Building on this, Congress could pass legislation mandating AI disclosures, thereby formalizing the requirement. Ultimately, in a future round of HSR revisions, the agencies could incorporate this mandate as a binding rule within the pre-merger framework. To avoid unnecessary burden on businesses, reporting should only be required when AI plays a significant role in the company’s operations or is expected to post-merger. What constitutes a ‘significant role’ should be left to the discretion of the agencies but could include AI systems central to core functions such as pricing, customer targeting, wage-setting, or automation of critical processes.
Recommendation 2. Reinforce the FTC and DOJ’s Technical Strategy in Assessing and Mitigating Anticompetitive AI Practices.
Strengthening the agencies’ ability to address AI requires two actions: integrating computational antitrust strategies and increasing technical expertise. A wave of recent research has highlighted AI as a powerful tool in helping detect anticompetitive behavior. For instance, scholars at the Stanford Computational Antitrust Project have demonstrated that methods such as machine learning, natural language processing, and network analysis can assist with tasks, ranging from uncovering collusion between firms to distinguishing digital markets. While the DOJ has already partnered with the Project, the FTC could benefit by pursuing a similar collaboration. More broadly, the agencies should deepen their technical expertise by expanding workshops and training with AI academic leaders. Doing so would not only provide them with access to the most sophisticated techniques in the field, but would also help bridge the gap between academic research and real-world implementation. Examples may include the use of machine learning algorithms to identify price-fixing and wage-setting; sentiment analysis, topic modeling, and other natural language processing tools to detect intention to collude in firm communications; or reverse-engineering algorithms to predict outcomes of AI-driven market manipulation.
Leveraging such computational strategies would enable regulators to analyze complex market data more effectively, enhancing the efficiency and precision of antitrust investigations. Given AI’s immense power, only a small—but highly skilled—team is needed to make significant progress. For instance, the UK’s Competition and Markets Authority (CMA) recently stood up a Data, Technology and Analytics unit, whereby they implement machine learning strategies to investigate various antitrust matters. For the U.S. agencies to facilitate this, the DOJ and FTC should hire more ML/AI experts, data scientists, and technologists, who could serve several key functions. First, they could conduct research on the most effective methods for detecting collusion and anticompetitive behavior in both digital and non-digital markets. Second, based on such research, they could guide the implementation of selected AI solutions in investigations and policy development. Third, they could perform assessments of AI technologies, evaluating the potential risks and benefits of AI applications in specific markets and companies. These assessments would be particularly useful during merger review, as previously discussed in Recommendation 1. Finally, they could help establish guidelines for transparency and accountability, ensuring the responsible and ethical use of AI both within the agencies and across the markets they regulate.
To formalize this recommendation, the President should submit a budget proposal to Congress requesting increased funding for the FTC and DOJ to (1) hire technology/AI experts and (2) provide necessary training for other selected employees on AI algorithms and datasets. The FTC may separately consider using its 6(b) subpoena powers to conduct a comprehensive study of the AI industry or of the use of AI practices more generally (e.g., to set prices or wages). Finally, the agencies should strive to foster collaboration between each other (e.g., establishing a Joint DOJ-FTC Computational Task Force), as well as with those in academia and the private sector, to ensure that enforcement strategies remain at the cutting edge of AI advancements.
Conclusion
The nation is in the midst of an AI revolution, and with it comes new avenues for anticompetitive behavior. As it stands, the antitrust enforcement agencies lack the necessary tools to adequately address this growing threat.
However, this environment also presents a pivotal opportunity for modernization. By requiring the disclosure of AI technologies during the merger review process, and by reinforcing the technical strategy at the FTC and DOJ, the antitrust agencies can strengthen their ability to detect and prevent anticompetitive practices. Leveraging the expertise of technologists in enforcement efforts can enhance the agencies’ capacity to monitor levels of competition in markets, as well as allow them to identify patterns between certain technologies and violations of antitrust.
Given the rapid pace of AI advancement, a proactive effort triumphs over a reactive one. Detecting antitrust violations early allows agencies to save both time and resources. To protect consumers, workers, and the economy more broadly, it is imperative that the FTC and DOJ adapt their enforcement strategies to meet the complexities of the AI era.
This action-ready policy memo is part of Day One 2025 — our effort to bring forward bold policy ideas, grounded in science and evidence, that can tackle the country’s biggest challenges and bring us closer to the prosperous, equitable and safe future that we all hope for whoever takes office in 2025 and beyond.
PLEASE NOTE (February 2025): Since publication several government websites have been taken offline. We apologize for any broken links to once accessible public data.
A National Guidance Platform for AI Acquisition
Streamlining the procurement process for more equitable, safe, and innovative government use of AI
The federal government’s approach to procuring AI systems serves two critical purposes: it not only shapes industry and academic standards but also determines how effectively AI can enhance public services. By leveraging its substantial purchasing power responsibly, the government can encourage high-quality, inclusive AI solutions that address diverse citizen needs while setting a strong precedent for innovation and accountability. Guidance issued in October 2024 by the White House’s Office of Management and Budget (OMB) gives recommendations on how agencies should use AI systems, focusing on public trust and data transparency. However, it is unclear how these guidelines align with general procurement regulations like the Federal Acquisition Regulation (FAR).
To reduce bureaucratic hurdles and encourage safe government innovation, the General Services Administration (GSA) should develop a digital platform that guides federal agencies through an “acquisition journey” for AI procurement. This recommendation is for streamlining guidance for procuring AI systems and should not be confused with the use of AI to simplify the procurement process. The platform should be intuitive and easy to navigate by clearly outlining the necessary information, requirements, and resources at each process stage, helping users understand what they need at any point in the procurement lifecycle. Such a platform would help agencies safely procure and implement AI technologies while staying informed on the latest guidelines and adhering to existing federal procurement rules. GSA should take inspiration from Brazil’s well-regarded Public Procurement Platform for Innovation (CPIN). CPIN helps public servants navigate the procurement process by offering best practices, risk assessments, and contract guidance, ensuring transparency and fairness at each stage of the procurement process.
Challenges and Opportunities
The federal government’s approach to AI systems is a crucial societal benchmark, shaping standards that ripple through industries, academia, and public discourse. Along with shaping the market, the government also faces a delicate balancing act when it comes to its own use of AI: it must harness AI’s potential to dramatically enhance efficiency and effectiveness in public service delivery while simultaneously adhering to the highest AI safety and equity standards. As such, the government’s handling of AI technologies carries immense responsibility and opportunity.
The U.S. federal government procures AI for numerous different tasks—from analyzing weather hazards and expediting benefits claims to processing veteran feedback. Positive impacts could potentially include faster and more accurate public services, cost savings, better resource allocation, improved decision-making based on data insights, and enhanced safety and security for citizens. However, risks can include privacy breaches, algorithmic bias leading to unfair treatment of certain groups, over-reliance on AI for critical decisions, lack of transparency in AI-driven processes, and cybersecurity vulnerabilities. These issues could erode public trust, inhibit the adoption of beneficial AI, and exacerbate existing social inequalities.
The federal government has recently published several guidelines on the acquisition and use of AI systems within the federal government, specifically how to identify and mitigate systems that may impact public trust in these systems. For example:
- OMB Memo M-24-10 (2024): Guides federal agencies on the use of artificial intelligence. It emphasizes responsible AI development and deployment, focusing on key principles such as safety, security, fairness, and transparency. The memo outlines requirements for AI governance, risk management, and public transparency in federal AI applications.
- OMB Memo M-24-18 (2024): Provides Guidance on AI acquisitions, such as transparency, continued guidance for incident reporting on rights and safety impacting AI, data management, and specific advice for AI-based biometrics.
- Agency Memos (2024): Per M-24-10, many U.S. agencies have published their internal strategies for AI use.
- AI Use Case Inventory (2024): Requires agencies to perform an annual inventory of AI systems with information on Procurement Instrument Identifiers and potential for rights or safety impacts.
- Executive Order on the Safe, Secure, and Trustworthy Development and Use of Artificial Intelligence (2023) This requires agencies to adopt trustworthy and responsible AI practices. It mandates using AI safety standards, including rigorous testing, auditing, and privacy protections across federal systems.
- Executive Order 13960 (2020) promotes the use of trustworthy artificial intelligence in government and outlines the responsibilities of agencies to ensure their AI use is ethical, transparent, and accountable. It includes the need for agencies to consider risks, fairness, and bias in AI systems.
This guidance, coupled with the already extensive set of general procurement regulations such as the Federal Acquisition Regulation (FAR ), can be overwhelming for public servants. In conversations with the author of this memo, stakeholders, including agency personnel and vendors, frequently noted that they needed clarification about when impact and risk assessments should occur in the FAR process.
How can government agencies adequately follow their mandate to provide safe and trustworthy AI for public services while reducing the bureaucratic burden that can result in an aversion to government innovation? A compelling example comes from Brazil. The Public Procurement Platform for Innovation (CPIN), managed by the Brazilian Ministry of Development, Industry, Commerce, and Services (MDIC), is an open resource designed to share knowledge and best practices on public procurement for innovation. In 2023, the platform was recognized by the Federal Court of Auditors (TCU—the agency that oversees federal procurement) as an essential new asset in facilitating public service. The CPIN helps public servants navigate the procurement process by diagnosing needs and selecting suitable contracting methods through questionnaires. Then, it orients agencies through a procurement journey, identifying what procurement process should be used, what kinds of dialogue the agency should have with potential vendors and other stakeholders, guidance for risk assessments, and contract language. The platform is meant to guide public servants through each stage of the procurement process, ensuring they know their obligations for transparency, fairness, and risk mitigation at any given time. CPIN is open to the public and is meant to be a resource, not new requirements that supplant existing mandates by Brazilian authorities.
Here in the U.S., the Office of Federal Procurement (OFFP) within the Office of Management and Budget (OMB) in partnership with the General Services Administration (GSA) and the Council of Chief AI Officers (CAIO), should develop a similar centralized resource to help federal agencies procure AI technologies safely and effectively. This platform would ensure agencies have up-to-date guidelines on AI acquisition integrated with existing procurement frameworks.
This approach is beneficial because:
- Public-facing access reduces information gaps between government entities, vendors, and stakeholders, fostering transparency and leveling the playing field for mid- and small-sized vendors.
- Streamlined processes alleviate complexity, making it easier for agencies to procure AI technologies.
- Clear guidance for agencies throughout each step of the procurement process ensures that they complete essential tasks such as impact evaluations and risk assessments within the appropriate time frame.
GSA has created similar tools before. For example, the Generative AI Acquisition Resource Guide assists federal buyers in procuring and implementing generative AI technologies by describing key considerations, best practices, and potential challenges associated with acquiring generative AI solutions. However, this digital platform would go one step further and align best practices, recommendations, and other AI considerations within the processes outlined in the FAR and other procurement methods.
Plan of Action
Recommendation 1. Establish a Working Group led by the OMB OFPP, with participation from GSA, OSTP, and the CAIO Council, tasked with systematically mapping all processes and policies influencing public sector AI procurement.
This includes direct AI-related guidance and tangential policies such as IT, data management, and cybersecurity regulations. The primary objective is identifying and addressing existing AI procurement guidance gaps, ensuring that the forthcoming platform can provide clear, actionable information to federal agencies. To achieve this, the working group should:
Conduct a thorough review of current mandates (see the FAQ for a non-exhaustive list of current mandates), executive orders, OMB guidance, and federal guidelines that pertain to AI procurement. This includes mapping out the requirements and obligations agencies must meet during acquisition. Evaluate if these mandates come with explicit deadlines or milestones that need to be integrated into the procurement timeline (e.g., AI risk assessments, ethics reviews, security checks)
Conduct a gap analysis to identify areas where existing AI procurement guidance needs to be clarified, completed, or updated. Prioritize gaps that can be addressed by clarifying existing rules or providing additional resources like best practices rather than creating new mandates to avoid unnecessary regulatory burdens. For example, guidance on handling personally identifiable information within commercially available information, guidance on data ownership between government and vendors, and the level of detail required for risk assessments.
Categorize federal guidance into two main buckets: general federal procurement guidance (e.g., Federal Acquisition Regulation [FAR]) and agency-specific guidelines (e.g., individual AI policies from agencies such as DoD’s AI Memos or NASA’s Other Transaction Authorities [OTAs]). Ensure that agency-specific rules are clearly distinguished on the platform, allowing agencies to understand when general AI acquisition rules apply and when specialized guidance takes precedence. Since the FAR may take years to update to reflect agency best practices, this could help give visibility to potential gaps.
Recommendation 2. The OMB OFPP-GSA-CAIO Council Working Group should convene a series of structured engagements with government and external stakeholders to co-create non-binding, practical guidance addressing gaps in AI procurement to be included in the platform.
These stakeholders should include government agency departments (e.g., project leads, procurement officers, IT departments) and external partners (vendors, academics, civil society organizations). The working group’s recommendations should focus on providing agencies with the tools, content, and resources they need to navigate AI procurement efficiently. Key focus areas would include risk management, ethical considerations, and compliance with cybersecurity policies throughout the procurement process. The guidance should also highlight areas where more frequent updates will be required, particularly in response to rapid developments in AI technologies and federal policies.
Topics that these stakeholder convenings could cover include:
Procurement Process
- Acquisition Pathways: What acquisition methods (e.g., FAR, Other Transaction Authorities [OTA], and joint acquisition programs) can be leveraged for procuring AI? Identify the most appropriate mechanisms for different AI use cases. For example, agencies looking to develop an advanced AI system with the help of external researchers may want to consider OTA if that is available to them.
- Integrating New Guidance: How can recent AI-related guidance from OMB memos (like M-24-10 and M-24-18) be incorporated into existing procurement frameworks, especially within the FAR?
- Stakeholder Responsibilities: Clearly define the roles and obligations of each party in the AI procurement process, from agency departments (such as project teams, procurement offices, and IT) to vendors and contractors. Determine who manages AI-related risks, evaluates AI systems, and ensures compliance with relevant policies.
- NIST AI Risk Management Framework (RMF): Explore how the NIST AI RMF can be integrated into the acquisition process and ensure agencies are equipped to assess AI risks effectively within procurement.
Transparency
- Public Disclosure: Define what information must be shared with the public at various stages of the AI acquisition process. Ensure there is a balance between transparency and protecting sensitive information.
- Data Sharing and Protection: Identify resources to help agencies understand their obligations regarding data sharing and protection under OMB Memo M-24-18 or forthcoming memos from the new administration to ensure compliance with any data security and privacy requirements.
- Risk Communication: Establish when and how to communicate to relevant stakeholders (e.g., the public and civil society) that a potential AI acquisition could impact public trust in AI technologies. Outline the types of transparency that should accompany AI systems that carry such risks.
Resources:
- External Best Practices: Gather and share civil society toolkits, industry best practices, and academic evaluations that can help agencies ensure the trustworthy use of AI. This would provide agencies with access to external expertise to complement federal guidelines and standards. The stakeholder convening should deliberate on whether these best practices will just be linked to the platform or if they need some kind of endorsement from government agencies.
Recommendation 3. The OPPF, in collaboration with GSA and the United States Digital Service (USDS) should then develop an intuitive, easy-to-navigate digital platform that guides federal agencies through an “acquisition journey” for AI procurement.
While the focus of this memo is on the broader procurement of AI systems, this digital platform could also benefit from the incorporation of AI, for example, by using a chatbot that is able to refer government users to the specific regulations governing their use cases. At each process stage, the platform should clearly outline the necessary information collected during the previous phases of this project to help users understand exactly what is needed at any given point in the procurement lifecycle.
The platform should serve as a central repository that unites all relevant AI procurement requirements, guidance from federal regulations (e.g., FAR, OMB memos), and insights from stakeholder convenings (e.g., vendors, academics, civil society). Each procurement stage should feature the most up-to-date guidance, ensuring a comprehensive and organized resource for federal employees.
The system should be designed for ease of navigation, potentially modeled after Brazil’s CPIN, which is organized like a city subway map. Users can begin with a simple questionnaire recommending a specific “subway line” or procurement process. Each “stop” along the line would represent a key stage in the procurement journey, offering relevant guidance, requirements, and best practices for that phase.
OPPF and GSA must regularly update the platform to reflect the latest federal AI and procurement policies and evolving best practices from government, civil society, and industry sources. Regular updates ensure that agencies use the most current information, especially as AI technologies and policies evolve rapidly.
The Federal Acquisition Institute within OFPP should create robust training programs to familiarize public servants with the new platform and how to use it effectively. These programs should explain how the platform supports AI acquisition and links to broader agency AI strategies.
- Roll out the platform gradually through agency-specific capacity-building sessions, demonstrating its utility for different departments. These sessions should show how the resource can help public servants meet their AI procurement needs and align with their agency’s strategic AI goals.
- Develop specialized training modules for different government stakeholders. For example, project teams might focus on aligning AI systems with mission objectives, procurement specialists on contract compliance, and IT departments on technical evaluations and cybersecurity.
- To ensure broad understanding and transparency, host public briefings for external stakeholders such as vendors, civil society organizations, and researchers. These sessions would clarify AI procurement requirements, fostering trust and collaboration between the public and private sectors.
Conclusion
The proposed centralized platform would represent a significant step forward in streamlining and standardizing the acquisition of AI technologies across federal agencies. By consolidating guidance, resources, and best practices into a user-friendly digital interface, this initiative would address gaps in the current AI acquisition landscape without increasing bureaucracy. This initiative supports individual agencies in their AI adoption efforts. It promotes a cohesive, government-wide approach to responsible AI implementation, ultimately benefiting both public servants and the citizens they serve.
This action-ready policy memo is part of Day One 2025 — our effort to bring forward bold policy ideas, grounded in science and evidence, that can tackle the country’s biggest challenges and bring us closer to the prosperous, equitable and safe future that we all hope for whoever takes office in 2025 and beyond.
PLEASE NOTE (February 2025): Since publication several government websites have been taken offline. We apologize for any broken links to once accessible public data.
There are so many considerations based on a particular agency’s many needs. A non-exhaustive list of legislation, executive orders, standards and other guidance relating to innovation procurement and agency use of AI can be found here. One approach to top-level simplification and communication is to create something similar to Brazil’s city subway map, discussed above.
The original Brazilian CPIN is designed for general innovation procurement and is agnostic to specific technologies or services. However, this memo focuses on artificial intelligence (AI) in light of recent guidance from the Office of Management and Budget (OMB) and the growing interest in AI from both the Biden Administration and the incoming Trump Administration. Establishing a platform specifically for AI system procurement could serve as a pilot for developing a broader innovation procurement platform.
The platform seeks to ensure responsible public sector AI by mitigating information asymmetries between government agencies and vendors, specifically by:
- Incorporating the latest OMB guidelines on AI system usage, focusing on human rights, safety, and data transparency. These guidelines are seamlessly integrated into each step of the procurement process.
- Throughout the “acquisition journey,” the platform should include clarifying checkpoints where agencies can demonstrate how their procurement plans align with established safety, equity, and ethical standards.
- Prompting agencies to consider how procured AI systems will address context-specific risks by integrating agency-specific guidance (e.g., the Department of Labor’s AI Principles) into the existing AI procurement frameworks.