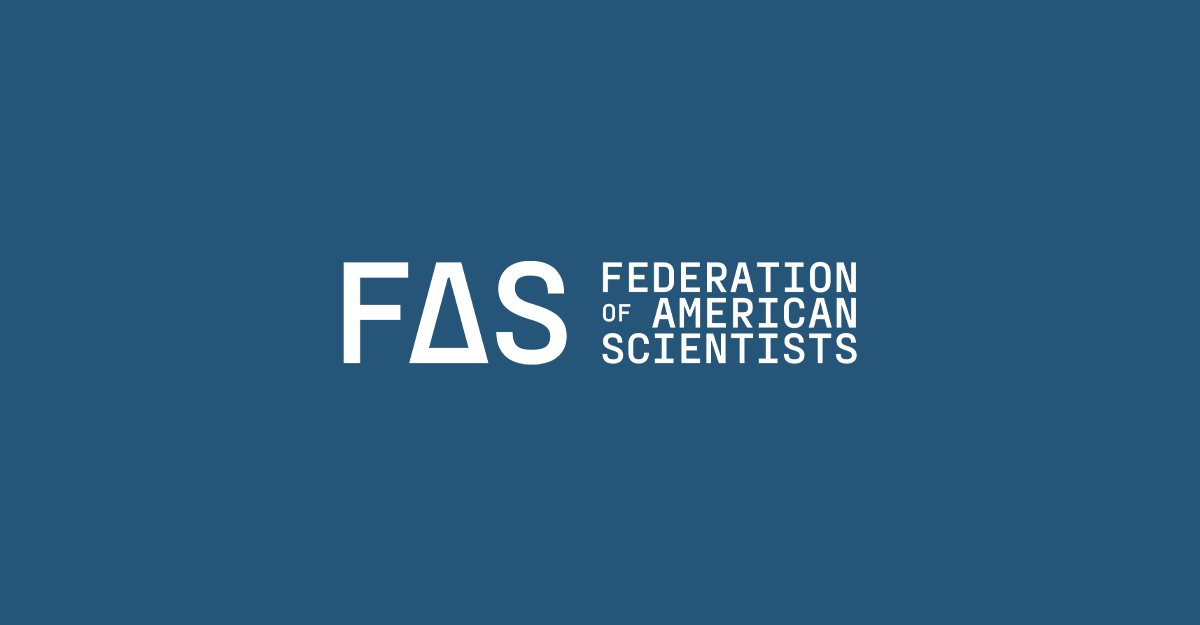
Public Comment on the U.S. Artificial Intelligence Safety Institute’s Draft Document: NIST AI 800-1, Managing Misuse Risk for Dual-Use Foundation Models
Public comments serve the executive branch by informing more effective, efficient program design and regulation. As part of our commitment to evidence-based, science-backed policy, FAS staff leverage public comment opportunities to embed science, technology, and innovation into policy decision-making.
The Federation of American Scientists (FAS) is a non-partisan organization dedicated to using science and technology to benefit humanity through equitable and impactful policy. With a strong track record in AI governance, FAS has actively contributed to the development of AI standards and frameworks, including providing feedback on NIST AI 600-1, the Generative AI Profile. Our work spans advocating for federal AI testbeds, recommending policy measures for frontier AI developers, and evaluating industry adoption of the NIST AI Risk Management Framework. We are members of the U.S. AI Safety Institute Research Consortium, and we responded to NIST’s request for information earlier this year concerning its responsibilities under sections 4.1, 4.5, and 11 of the AI Executive Order.
We commend NIST’s U.S. Artificial Intelligence Safety Institute for developing the draft guidance on “Managing Misuse Risk for Dual-Use Foundation Models.” This document represents a significant step toward establishing robust practices for mitigating catastrophic risks associated with advanced AI systems. The guidance’s emphasis on comprehensive risk assessment, transparent decision-making, and proactive safeguards aligns with FAS’s vision for responsible AI development.
In our response, we highlight several strengths of the guidance, including its focus on anticipatory risk assessment and the importance of clear documentation. We also identify areas for improvement, such as the need for harmonized language and more detailed guidance on model development safeguards. Our key suggestions include recommending a more holistic socio-technical approach to risk evaluation, strengthening language around halting development for unmanageable risks, and expanding the range of considered safeguards. We believe these adjustments will further strengthen NIST’s crucial role in shaping responsible AI development practices.
Background and Context
The rapid advancement of AI foundation models has spurred novel industry-led risk mitigation strategies. Leading AI companies have voluntarily adopted frameworks like Responsible Scaling Policies and Preparedness Frameworks, outlining risk thresholds and mitigation strategies for increasingly capable AI systems. (Our response to NIST’s February RFI was largely an exploration of these policies, their benefits and drawbacks, and how they could be strengthened.)
Managing misuse risks in foundation models is of paramount importance given their broad applicability and potential for dual use. As these models become more powerful, they may inadvertently enable malicious actors to cause significant harm, including facilitating the development of weapons, enabling sophisticated cyber attacks, or generating harmful content. The challenge lies not only in identifying current risks but also in anticipating future threats that may emerge as AI capabilities expand.
NIST’s new guidance on “Managing Misuse Risk for Dual-Use Foundation Models” builds upon these industry initiatives, providing a more standardized and comprehensive approach to risk management. By focusing on objectives such as anticipating potential misuse, establishing clear risk thresholds, and implementing robust evaluation procedures, the guidance creates a framework that can be applied across the AI development ecosystem. This approach is crucial for ensuring that as AI technology advances, appropriate safeguards are in place to protect against potential misuse while still fostering innovation.
Strengths of the guidance
1. Comprehensive Documentation and Transparency
The guidance’s emphasis on thorough documentation and transparency represents a significant advancement in AI risk management. For every practice under every objective, the guidance indicates appropriate documentation; this approach is more thorough in advancing transparency than any comparable guidance to date. The creation of a paper trail for decision-making and risk evaluation is crucial for both internal governance and potential external audits.
The push for transparency extends to collaboration with external stakeholders. For instance, practice 6.4 recommends providing “safe harbors for third-party safety research,” including publishing “a clear vulnerability disclosure policy for model safety issues.” This openness to external scrutiny and feedback is essential for building trust and fostering collaborative problem-solving in AI safety. (FAS has published a legislative proposal calling for enshrining “safe harbor” protections for AI researchers into law.)
2. Lifecycle Approach to Risk Management
The guidance excels in its holistic approach to risk management, covering the entire lifecycle of foundation models from pre-development assessment through to post-deployment monitoring. This comprehensive approach is evident in the structure of the document itself, which follows a logical progression from anticipating risks (Objective 1) through to responding to misuse after deployment (Objective 6).
The guidance demonstrates a proactive stance by recommending risk assessment before model development. Practice 1.3 suggests to “Estimate the model’s capabilities of concern before it is developed…”, which helps anticipate and mitigate potential harms before they materialize. The framework for red team evaluations (Practice 4.2) is particularly robust, recommending independent external experts and suggesting ways to compensate for gaps between red teams and real threat actors. The guidance also emphasizes the importance of ongoing risk assessment. Practice 3.2 recommends to “Periodically revisit estimates of misuse risk stemming from model theft…” This acknowledgment of the dynamic nature of AI risks encourages continuous vigilance.
3. Strong Stance on Model Security and Risk Tolerance
The guidance takes a firm stance on model security and risk tolerance, particularly in Objective 3. It unequivocally states that models relying on confidentiality for misuse risk management should only be developed when theft risk is sufficiently mitigated. This emphasizes the critical importance of security in AI development, including considerations for insider threats (Practice 3.1).
The guidance also demonstrates a realistic approach to the challenges posed by different deployment strategies. In Practice 5.1, it notes, “For example, allowing fine-tuning via API can significantly limit options to prevent jailbreaking and sharing the model’s weights can significantly limit options to monitor for misuse (Practice 6.1) and respond to instances of misuse (Practice 6.2).” This candid discussion of the limitations of safety interventions for open weight foundation models is crucial for fostering realistic risk assessments.
Additionally, the guidance promotes a conservative approach to risk management. Practice 5.3 recommends to “Consider leaving a margin of safety between the estimated level of risk at the point of deployment and the organization’s risk tolerance.” It further suggests considering “a larger margin of safety to manage risks that are more severe or less certain.” This approach provides an extra layer of protection against unforeseen risks or rapid capability advancements, which is crucial given the uncertainties inherent in AI development.
These elements collectively demonstrate NIST’s commitment to promoting realistic and robust risk management practices that prioritize safety and security in AI development and deployment. However, while the NIST guidance demonstrates several important strengths, there are areas where it could be further improved to enhance its effectiveness in managing misuse risks for dual-use foundation models.
Areas for improvement
1. Need for a More Comprehensive Socio-technical Approach to Measuring Misuse Risk
Objective 4 of the guidance demonstrates a commendable effort to incorporate elements of a socio-technical approach in measuring misuse risk. The guidance recognizes the importance of considering both technical and social factors, emphasizes the use of red teams to assess potential misuse scenarios, and acknowledges the need to consider different levels of access and various threat actors. Furthermore, it highlights the importance of avoiding harm during the measurement process, which is crucial in a socio-technical framework.
However, the guidance falls short in fully embracing a comprehensive socio-technical perspective. While it touches on the importance of external experts, it does not sufficiently emphasize the value of diverse perspectives, particularly from individuals with lived experiences relevant to specific risk scenarios. The guidance also lacks a structured approach to exploring the full range of potential misuse scenarios across different contexts and risk areas. Finally, the guidance does not mention measuring absolute versus marginal risks (ie., how much total misuse risk a model poses in a specific context versus how much marginal risk it poses compared to existing tools). These gaps limit the effectiveness of the proposed risk measurement approach in capturing the full complexity of AI system interactions with human users and broader societal contexts.
Specific recommendations for improving socio-technical approach
The NIST guidance in Practice 1.3 suggests estimating model capabilities by comparison to existing models, but provides little direction on how to conduct these comparisons effectively. To improve this, NIST could incorporate the concept of “available affordances.” This concept emphasizes that an AI system’s risk profile depends not just on its absolute capabilities, but also on the environmental resources and opportunities for affecting the world that are available to it.
Additionally, Kapoor et al. (2024) emphasize the importance of assessing the marginal risk of open foundation models compared to existing technologies or closed models. This approach aligns with a comprehensive socio-technical perspective by considering not just the absolute capabilities of AI systems, but also how they interact with existing technological and social contexts. For instance, when evaluating cybersecurity risks, they suggest considering both the potential for open models to automate vulnerability detection and the existing landscape of cybersecurity tools and practices. This marginal risk framework helps to contextualize the impact of open foundation models within broader socio-technical systems, providing a more nuanced understanding of their potential benefits and risks.
NIST could recommend that organizations assess both the absolute capabilities of their AI systems and the affordances available to them in potential deployment contexts. This approach would provide a more comprehensive view of potential risks than simply comparing models in isolation. For instance, the guidance could suggest evaluating how a system’s capabilities might change when given access to different interfaces, actuators, or information sources.
Similarly, Weidinger et al. (2023) argue that while quantitative benchmarks are important, they are insufficient for comprehensive safety evaluation. They suggest complementing quantitative measures with qualitative assessments, particularly at the human interaction and systemic impact layers. NIST could enhance its guidance by providing more specific recommendations for integrating qualitative evaluation methods alongside quantitative benchmarks.
NIST should acknowledge potential implementation challenges with a comprehensive socio-technical approach. Organizations may struggle to create benchmarks that accurately reflect real-world misuse scenarios, particularly given the rapid evolution of AI capabilities and threat landscapes. Maintaining up-to-date benchmarks in a fast-paced field presents another ongoing challenge. Additionally, organizations may face difficulties in translating quantitative assessments into actionable risk management strategies, especially when dealing with novel or complex risks. NIST could enhance the guidance by providing strategies for navigating these challenges, such as suggesting collaborative industry efforts for benchmark development or offering frameworks for scalable testing approaches.
OpenAI‘s approach of using human participants to evaluate AI capabilities provides both a useful model for more comprehensive evaluation and an example of quantification challenges. While their evaluation attempted to quantify biological risk increase from AI access, they found that, as they put it, “Translating quantitative results into a meaningfully calibrated threshold for risk turns out to be difficult.” This underscores the need for more research on how to set meaningful thresholds and interpret quantitative results in the context of AI safety.
2. Inconsistencies in Risk Management Language
There are instances where the guidance uses varying levels of strength in its recommendations, particularly regarding when to halt or adjust development. For example, Practice 2.2 recommends to “Plan to adjust deployment or development strategies if misuse risks rise to unacceptable levels,” while Practice 3.2 uses stronger language, suggesting to “Adjust or halt further development until the risk of model theft is adequately managed.” This variation in language could lead to confusion and potentially weaker implementation of risk management strategies.
Furthermore, while the guidance emphasizes the importance of managing risks before deployment, it does not provide clear criteria for what constitutes “adequately managed” risk, particularly in the context of development rather than deployment. More consistent and specific language around these critical decision points would strengthen the guidance’s effectiveness in promoting responsible AI development.
Specific recommendations for strengthening language on halting development for unmanageable risks
To address the inconsistencies noted above, we suggest the following changes:
1. Standardize the language across the document to consistently use strong phrasing such as “Adjust or halt further development” when discussing responses to unacceptable levels of risk.
The current guidance uses varying levels of strength in its recommendations regarding development adjustments. For instance, Recommendation 4 of Practice 2.2 uses the phrase “Plan to adjust deployment or development strategies,” while Recommendation 3 of Practice 3.2 more strongly suggests to “Adjust or halt further development.” Consistent language would emphasize the critical nature of these decisions and reduce potential confusion or weak implementation of risk management strategies. This could be accomplished by changing the language of Practice 2.2, Recommendation 4 to “Plan to adjust or halt further development or deployment if misuse risks rise to unacceptable levels before adequate security and safeguards are available to manage risk.”
The need for stronger language regarding halting development is reflected both in NIST’s other work and in commitments that many frontier AI developers have publicly agreed to. For instance, the NIST AI Risk Management Framework, section 1.2.3 (Risk Prioritization), suggests: “In some cases where an AI system presents the highest risk – where negative impacts are imminent, severe harms are actually occurring, or catastrophic risks are present – development and deployment should cease in a safe manner until risks can be sufficiently mitigated.” Further, the AI Seoul Summit frontier AI safety commitments explicitly state that organizations should “set out explicit processes they intend to follow if their model or system poses risks that meet or exceed the pre-defined thresholds.” Importantly, these commitments go on to specify that “In the extreme, organisations commit not to develop or deploy a model or system at all, if mitigations cannot be applied to keep risks below the thresholds.”
2. Add to the list of transparency documentation for Practice 2.2 the following: “A decision-making framework for determining when risks have become truly unmanageable, considering factors like the severity of potential harm, the likelihood of the risk materializing, and the feasibility of mitigation strategies.”
While the current guidance emphasizes the importance of managing risks before deployment (e.g., in Practice 5.3), it does not provide clear criteria for what constitutes “adequately managed” risk, particularly in the context of development rather than deployment. A decision-making framework would provide clearer guidance on when to take the serious step of halting development. This addition would help prevent situations where development continues despite unacceptable risks due to a lack of clear stopping criteria. This recommendation aligns with the approach suggested by Alaga and Schuett (2023) in their paper on coordinated pausing, where they emphasize the need for clear thresholds and decision criteria to determine when AI development should be halted due to unacceptable risks.
3. Gaps in Model Development Safeguards
The guidance’s treatment of safeguards, particularly those related to model development, lacks sufficient detail to be practically useful. This is most evident in Appendix B, which lists example safeguards. While this appendix is a valuable addition, the safeguards related to model training (“Improve the model’s training”) are notably lacking in detail compared to the safeguards around model security and detecting misuse.
While the guidance covers many aspects of risk management comprehensively, especially model security, it does not provide enough specific recommendations for technical approaches to building safer models during the development phase. This gap could limit the practical utility of the guidance for AI developers seeking to implement safety measures from the earliest stages of model creation.
Specific recommendations for additional safeguards for model development
For some safeguards, we recommend that the misuse risk guidance explicitly reference relevant sections of NIST 600-1, the Generative Artificial Intelligence Profile. Specifically, the GAI profile offers more comprehensive guidance on data-related and monitoring safeguards. For instance, the profile emphasizes documenting training data curation policies (MP-4.1-004) and establishing policies for data collection, retention, and quality (MP-4.1-005), which are crucial for managing misuse risk from the earliest stages of development. Additionally, the profile suggests implementing real-time monitoring processes for analyzing generated content performance and trustworthiness characteristics (MG-3.2-006), which could significantly enhance ongoing risk management during development. These references to the GAI Profile on model development safeguards could take the form of an additional item in Appendix B, or be incorporated into the relevant sections earlier in the guidance.
Beyond pointing to the model development safeguards included in the GAI Profile, we also recommend expanding Appendix B to include further safeguards for the model development phase. Both the GAI Profile and the current misuse risk guidance lack specific recommendations for two key model development safeguards: iterative safety testing throughout development and staged development/release processes. Below are two proposed additions to Appendix B:
The proposed safeguard “Implement iterative safety testing throughout development” addresses the current guidance’s limited detail on model training and development safeguards. This approach aligns with Barrett, et al.’s AI Risk-Management Standards Profile for General-Purpose AI Systems and Foundation Models (the “GPAIS Profile”)’s emphasis on proactive and ongoing risk assessment. Specifically, the Profile recommends identifying “GPAIS impacts…and risks (including potential uses, misuses, and abuses), starting from an early AI lifecycle stage and repeatedly through new lifecycle phases or as new information becomes available” (Barrett et al., 2023, p. 19). The GPAIS Profile further suggests that for larger models, developers should “analyze, customize, reanalyze, customize differently, etc., then deploy and monitor” (Barrett et al., 2023, p. 19), where “analyze” encompasses probing, stress testing, and red teaming. This iterative safety testing would integrate safety considerations throughout development, aligning with the guidance’s emphasis on proactive risk management and anticipating potential misuse risk.
Similarly, the proposed safeguard “Establish a staged development and release process” addresses a significant gap in the current guidance. While Practice 5.1 discusses pre-deployment risk assessment, it lacks a structured approach to incrementally increasing model capabilities or access. Solaiman et al. (2023) propose a “gradient of release” framework for generative AI, a phased approach to model deployment that allows for iterative risk assessment and mitigation. This aligns with the guidance’s emphasis on ongoing risk management and could enhance the ‘margin of safety’ concept in Practice 5.3. Implementing such a staged process would introduce multiple risk assessment checkpoints throughout development and deployment, potentially improving safety outcomes.
Conclusion
NIST’s guidance on “Managing Misuse Risk for Dual-Use Foundation Models” represents a significant step forward in establishing robust practices for mitigating catastrophic risks associated with advanced AI systems. The document’s emphasis on comprehensive risk assessment, transparent decision-making, and proactive safeguards demonstrates a commendable commitment to responsible AI development. However, to more robustly contribute to risk mitigation, the guidance must evolve to address key challenges, including a stronger approach to measuring misuse risk, consistent language on halting development, and more detailed model development safeguards.
As the science of AI risk assessment advances, this guidance should be recursively updated to address emerging risks and incorporate new best practices. While voluntary guidance is crucial, it is important to recognize that it cannot replace the need for robust policy and regulation. A combination of industry best practices, government oversight, and international cooperation will be necessary to ensure the responsible development of high-risk AI systems.
We appreciate the opportunity to provide input on this important document. FAS stands ready to continue assisting NIST in refining and implementing this guidance, as well as in developing further resources for responsible AI development. We believe that close collaboration between government agencies, industry leaders, and civil society organizations is key to realizing the benefits of AI while effectively mitigating its most serious risks.
The U.S. government should establish a public-private National Exposome Project (NEP) to generate benchmark human exposure levels for the ~80,000 chemicals to which Americans are regularly exposed.
The federal government is responsible for ensuring the safety and privacy of the processing of personally identifiable information within commercially available information used for the development and deployment of artificial intelligence systems
The United States is in the midst of a once in a generation effort to rebuild its transportation and mobility systems. Meeting this moment will require bold investments in new and emerging transportation technologies.
Employee ownership is a powerful solution that preserves local business ownership, protects supply chains, creates quality jobs, and grows the household balance sheets of American workers and their families.