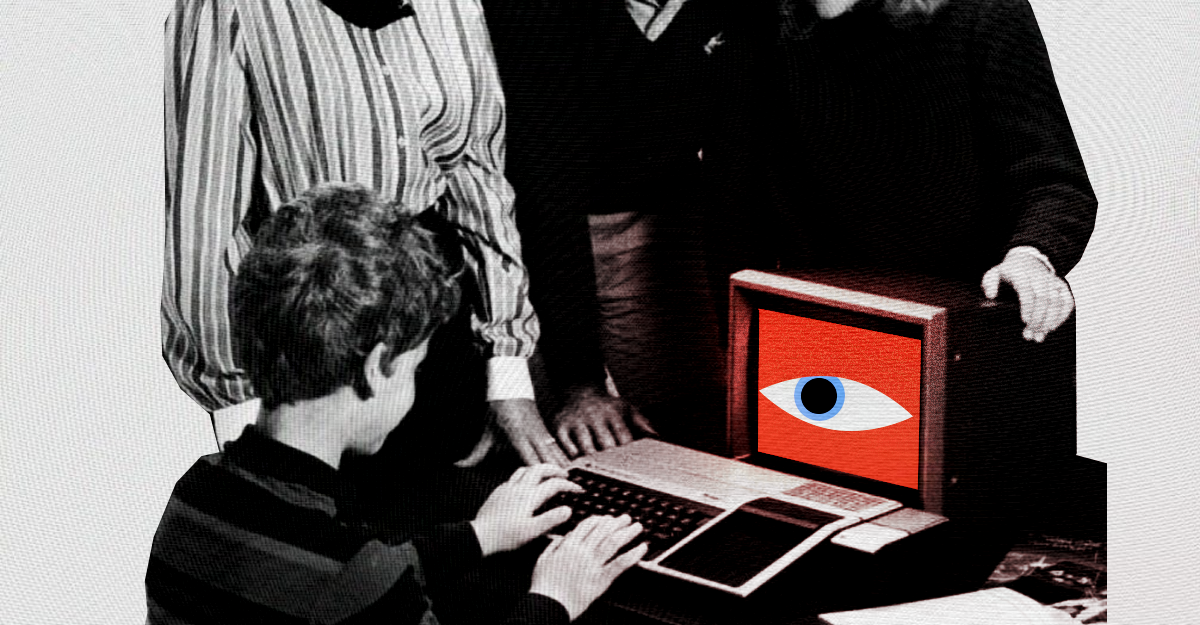
Scaling AI Safely: Can Preparedness Frameworks Pull Their Weight?
A new class of risk mitigation policies has recently come into vogue for frontier AI developers. Known alternately as Responsible Scaling Policies or Preparedness Frameworks, these policies outline commitments to risk mitigations that developers of the most advanced AI models will implement as their models display increasingly risky capabilities. While the idea for these policies is less than a year old, already two of the most advanced AI developers, Anthropic and OpenAI, have published initial versions of these policies. The U.K. AI Safety Institute asked frontier AI developers about their “Responsible Capability Scaling” policies ahead of the November 2023 UK AI Safety Summit. It seems that these policies are here to stay.
The National Institute of Standards & Technology (NIST) recently sought public input on its assignments regarding generative AI risk management, AI evaluation, and red-teaming. The Federation of American Scientists was happy to provide input; this is the full text of our response. NIST’s request for information (RFI) highlighted several potential risks and impacts of potentially dual-use foundation models, including: “Negative effects of system interaction and tool use…chemical, biological, radiological, and nuclear (CBRN) risks…[e]nhancing or otherwise affecting malign cyber actors’ capabilities…[and i]mpacts to individuals and society.” This RFI presented a good opportunity for us to discuss the benefits and drawbacks of these new risk mitigation policies.
This report will provide some background on this class of risk mitigation policies (we use the term Preparedness Framework, for reasons to be described below). We outline suggested criteria for robust Preparedness Frameworks (PFs) and evaluate two key documents, Anthropic’s Responsible Scaling Policy and OpenAI’s Preparedness Framework, against these criteria. We claim that these policies are net-positive and should be encouraged. At the same time, we identify shortcomings of current PFs, chiefly that they are underspecified, insufficiently conservative, and address structural risks poorly. Improvement in the state of the art of risk evaluation for frontier AI models is a prerequisite for a meaningfully binding PF. Most importantly, PFs, as unilateral commitments by private actors, cannot replace public policy.
Motivation for Preparedness Frameworks
As AI labs develop potentially dual-use foundation models (as defined by Executive Order No. 14110, the “AI EO”) with capability, compute, and efficiency improvements, novel risks may emerge, some of them potentially catastrophic. Today’s foundation models can already cause harm and pose some risks, especially as they are more broadly used. Advanced large language models at times display unpredictable behaviors.
To this point, these harms have not risen to the level of posing catastrophic risks, defined here broadly as “devastating consequences for vast numbers of people.” The capabilities of models at the current state of the art simply do not imply levels of catastrophic risk above current non-AI related margins.1 However, as these models continue to scale in training compute, some speculate they may develop novel capabilities that could potentially be misused. The specific capabilities that will emerge from further scaling remain difficult to predict with confidence or certainty. Some analysis indicates that as training compute for AI models has doubled approximately every six months since 2015, performance on capability benchmarks has also steadily improved. While it’s possible that bigger models could lead to better performance, it wouldn’t be surprising if smaller models emerge with better capabilities, as despite years of research by machine learning theorists, our knowledge of just how the number of model parameters relates to model capabilities remains uncertain.
Nonetheless, as capabilities increase, risks may also increase, and new risks may appear. Executive Order 14110 (the Executive Order on Artificial Intelligence, or the “AI EO”) detailed some novel risks of potentially dual-use foundation models, including potential risks associated with chemical, biological, radiological, or nuclear (CBRN) risks and advanced cybersecurity risks. Other risks are more speculative, such as risks of model autonomy, loss of control of AI systems, or negative impacts on users including risks of persuasion.2 Without robust risk mitigations, it is plausible that increasingly powerful AI systems will eventually pose greater societal risks.
Other technologies that pose catastrophic risks, such as nuclear technologies, are heavily regulated in order to prevent those risks from resulting in serious harms. There is a growing movement to regulate development of potentially dual-use biotechnologies, particularly gain-of-function research on the most pathogenic microbes. Given the rapid pace of progress at the AI frontier, comprehensive government regulation has yet to catch up; private companies that develop these models are starting to take it upon themselves to prevent or mitigate the risks of advanced AI development.
Prevention of such novel and consequential risks requires developers to implement policies that address potential risks iteratively. That is where preparedness frameworks come in. A preparedness framework is used to assess risk levels across key categories and outline associated risk mitigations. As the introduction to OpenAI’s PF states, “The processes laid out in each version of the Preparedness Framework will help us rapidly improve our understanding of the science and empirical texture of catastrophic risk, and establish the processes needed to protect against unsafe development.” Without such processes and commitments, the tendency to prioritize speed over safety concerns might prevail. While the exact consequences of failing to mitigate these risks are uncertain, they could potentially be significant.
Preparedness frameworks are limited in scope to catastrophic risks. These policies aim to prevent the worst conceivable outcomes of the development of future advanced AI systems; they are not intended to cover risks from existing systems. We acknowledge that this is an important limitation of preparedness frameworks. Developers can and should address both today’s risks and future risks at the same time; preparedness frameworks attempt to address the latter, while other “trustworthy AI” policies attempt to address a broader swathe of risks. For instance, OpenAI’s “Preparedness” team sits alongside its “Safety Systems” team, which “focuses on mitigating misuse of current models and products like ChatGPT.”
A note about terminology: The term “Responsible Scaling Policy” (RSP) is the term that took hold first, but it presupposes scaling of compute and capabilities by default. “Preparedness Framework” (PF) is a term coined by OpenAI, and it communicates the idea that the company needs to be prepared as its models approach the level of artificial general intelligence. Of the two options, “Preparedness Framework” communicates the essential idea more clearly: developers of potentially dual-use foundation models must be prepared for and mitigate potential catastrophic risks from development of these models.
The Industry Landscape
In September of 2023, ARC Evals (now METR, “Model Evaluation & Threat Research”) published a blog post titled “Responsible Scaling Policies (RSPs).” This post outlined the motivation and basic structure of an RSP, and revealed that ARC Evals had helped Anthropic write its RSP (version 1.0) which had been released publicly a few days prior. (ARC Evals had also run pre-deployment evaluations on Anthropic’s Claude model and OpenAI’s GPT-4.) And in December 2023, OpenAI published its Preparedness Framework in beta; while using new terminology, this document is structurally similar to ARC Evals’ outline of the structure of an RSP. Both OpenAI and Anthropic have indicated that they plan to update their PFs with new information as the frontier of AI development advances.
Not every AI company should develop or maintain a preparedness framework. Since these policies relate to catastrophic risk from models with advanced capabilities, only those developers whose models could plausibly attain those capabilities should use PFs. Because these advanced capabilities are associated with high levels of training compute, a good interim threshold for who should develop a PF could be the same as the AI EO threshold for potentially dual-use foundation models; that is, developers of models trained on over 10^26 FLOPS (or October 2023-equivalent level of compute adjusted for compute efficiency gains).3 Currently, only a handful of developers have models that even approach this threshold. This threshold should be subject to change, like that of the AI EO, as developers continue to push the frontier (e.g. by developing more efficient algorithms or realizing other compute efficiency gains).
While several other companies published “Responsible Capability Scaling” documents ahead of the UK AI Safety Summit, including DeepMind, Meta, Microsoft, Amazon, and Inflection AI, the rest of this report focuses primarily on OpenAI’s PF and Anthropic’s RSP.
Weaknesses of Preparedness Frameworks
Preparedness frameworks are not panaceas for AI-associated risks. Even with improvements in specificity, transparency, and strengthened risk mitigations, there are important weaknesses to the use of PFs. Here we outline a couple weaknesses of PFs and possible answers to them.
1. Spirit vs. text: PFs are voluntary commitments whose success depends on developers’ faithfulness to their principles.
Current risk thresholds and mitigations are defined loosely. In Anthropic’s RSP, for instance, the jump from the current risk level posed by Claude 2 (its state of the art model) to the next risk level is defined in part by the following: “Access to the model would substantially increase the risk of catastrophic misuse, either by proliferating capabilities, lowering costs, or enabling new methods of attack….” A “substantial increase” is not well-defined. This ambiguity leaves room for interpretation; since implementing risk mitigations can be costly, developers could have an incentive to take advantage of such ambiguity if they do not follow the spirit of the policy.
This concern about the gap between following the spirit of the PF and following the text might be somewhat eased with more specificity about risk thresholds and associated mitigations, and especially with more transparency and public accountability to these commitments.
To their credit, OpenAI’s PF and Anthropic’s RSP show a serious approach to the risks of developing increasingly advanced AI systems. OpenAI’s PF includes a commitment to fine-tune its models to better elicit capabilities along particular risk categories, then evaluate “against these enhanced models to ensure we are testing against the ‘worst case’ scenario we know of.” They also commit to triggering risk mitigations “when any of the tracked risk categories increase in severity, rather than only when they all increase together.” And Anthropic “commit[s] to pause the scaling and/or delay the deployment of new models whenever our scaling ability outstrips our ability to comply with the safety procedures for the corresponding ASL [AI Safety Level].” These commitments are costly signals that these developers are serious about their PFs.
2. Private commitment vs. public policy: PFs are unilateral commitments that individual developers take on; we might prefer more universal policy (or regulatory) approaches.
Private companies developing AI systems may not fully account for broader societal risks. Consider an analogy to climate change—no single company’s emissions are solely responsible for risks like sea level rise or extreme weather. The risk comes from the aggregate emissions of all companies. Similarly, AI developers may not consider how their systems interact with others across society, potentially creating structural risks. Like climate change, the societal risks from AI will likely come from the cumulative impact of many different systems. Unilateral commitments are poor tools to address such risks.
Furthermore, PFs might reduce the urgency for government intervention. By appearing safety-conscious, developers could diminish the perceived need for regulatory measures. Policymakers might over-rely on self-regulation by AI developers, potentially compromising public interest for private gains.
Policy can and should step into the gap left by PFs. Policy is more aligned to the public good, and as such is less subject to competing incentives. And policy can be enforced, unlike voluntary commitments. In general, preparedness frameworks and similar policies help hold private actors accountable to their public commitments; this effect is stronger with more specificity in defining risk thresholds, better evaluation methods, and more transparency in reporting. However, these policies cannot and should not replace government action to reduce catastrophic risks (especially structural risks) of frontier AI systems.
Suggested Criteria for Robust Preparedness Frameworks
These criteria are adapted from the ARC Evals post, Anthropic’s RSP, and OpenAI’s PF. Broadly, they are aspirational; no existing preparedness framework meets all or most of these criteria.
For each criterion, we explain the key considerations for developers adopting PFs. We analyze OpenAI’s PF and Anthropic’s RSP to illustrate the strengths and shortcomings of their approaches. Again, these policies are net-positive and should be encouraged. They demonstrate costly unilateral commitments to measuring and addressing catastrophic risk from their models; they meaningfully improve on the status quo. However, these initial PFs are underspecified and insufficiently conservative. Improvement in the state of the art of risk evaluation and mitigation, and subsequent updates, would make them more robust.
1. Preparedness frameworks should cover the breadth of potential catastrophic risks of developing frontier AI models.
These risks may include:
- CBRN risks. Advanced AI models might enable or aid the creation of chemical, biological, radiological, and/or nuclear threats. OpenAI’s PF includes CBRN risks as their own category; Anthropic’s RSP includes CBRN risks within risks from misuse.
- Model autonomy. Anthropic’s RSP defines this as: “risk that a model is capable of accumulating resources (e.g. through fraud), navigating computer systems, devising and executing coherent strategies, and surviving in the real world while avoiding being shut down.” OpenAI’s PF defines this as: “[enabling] actors to run scaled misuse that can adapt to environmental changes and evade attempts to mitigate or shut down operations. Autonomy is also a prerequisite for self-exfiltration, self-improvement, and resource acquisition.” OpenAI’s definition includes risk from misuse of a model in model autonomy; Anthropic’s focuses on risks from the model itself.
- Potential for misuse, including cybersecurity and critical infrastructure. OpenAI’s PF defines cybersecurity risk (in their own category) as “risks related to use of the model for cyber-exploitation to disrupt confidentiality, integrity, and/or availability of computer systems.” Anthropic’s RSP mentions cyber risks in the context of risks from misuse.
- Adverse impact on human users. OpenAI’s PF includes a tracked risk category for persuasion: “Persuasion is focused on risks related to convincing people to change their beliefs (or act on) both static and interactive model-generated content.” Anthropic’s RSP does not mention persuasion per se.
- Unknown future risks. As developers create and evaluate more highly capable models, new risk vectors might become clear. PFs should acknowledge that unknown future risks are possible with any jump in capabilities. OpenAI’s PF includes a commitment to tracking “currently unknown categories of catastrophic risk as they emerge.”
Preparedness frameworks should apply to catastrophic risks in particular because they govern the scaling of capabilities of the most advanced AI models, and because catastrophic risks are of the highest consequence to such development. PFs are one tool among many that developers of the most advanced AI models should use to prevent harm. Developers of advanced AI models tend to also have other “trustworthy AI” policies, which seek to prevent and address already-existing risks such as harmful outputs, disinformation, and synthetic sexual content. Despite PFs’ focus on potentially catastrophic risks, faithfully applying PFs may help developers catch many other kinds of risks as well, since they involve extensive evaluation for misuse potential and adverse human impacts.
2. Preparedness frameworks should define the developer’s acceptable risk level (“risk appetite”) in terms of likelihood and severity of risk, in accordance with the NIST AI Risk Management Framework, section Map 1.5.
Neither OpenAI nor Anthropic has publicly declared their risk appetite. This is a nascent field of research, as these risks are novel and perhaps less predictable than eg. nuclear accident risk.5 NIST and other standard-setting bodies will be crucial in developing AI risk metrology. For now, PFs should state developers’ risk appetites as clearly as possible, and update them regularly with research advances.6
AI developers’ risk appetites might be different than a regulatory risk appetite. Developers should elucidate their risk appetite in quantitative terms so their PFs can be evaluated accordingly. As in the case of nuclear technology, regulators may eventually impose risk thresholds on frontier AI developers. At this point, however, there is no standard, scientifically-grounded approach to measuring the potential for catastrophic AI risk; this has to start with the developers of the most capable AI models.
3. Preparedness frameworks should clearly define capability levels and risk thresholds. Risk thresholds should be quantified robustly enough to hold developers accountable to their commitments.
OpenAI and Anthropic both outline qualitative risk thresholds corresponding with different categories of risk. For instance, in OpenAI’s PF, the High risk threshold in the CBRN category reads: “Model enables an expert to develop a novel threat vector OR model provides meaningfully improved assistance that enables anyone with basic training in a relevant field (e.g., introductory undergraduate biology course) to be able to create a CBRN threat.” And Anthropic’s RSP defines the ASL-3 [AI Safety Level] threshold as: “Low-level autonomous capabilities, or access to the model would substantially increase the risk of catastrophic misuse, either by proliferating capabilities, lowering costs, or enabling new methods of attack, as compared to a non-LLM baseline of risk.”
These qualitative thresholds are under-specified; reasonable people are likely to differ on what “meaningfully improved assistance” looks like, or a “substantial increase [in] the risk of catastrophic misuse.” In PFs, these thresholds should be quantified to the extent possible.
To be sure, the AI development research community currently lacks a good empirical understanding of the likelihood or quantification of frontier AI-related risks. Again, this is a novel science that needs to be developed with input from both the private and public sectors. Since this science is still developing, it is natural to want to avoid too much quantification. A conceivable failure mode is that developers “check the boxes,” which may become obsolete quickly, in lieu of using their judgment to determine when capabilities are dangerous enough to warrant higher risk mitigations. Again, as research improves, we should expect to see improvements in PFs’ specification of risk thresholds.
4. Preparedness frameworks should include detailed evaluation procedures for AI models, ensuring comprehensive risk assessment within a developer’s tolerance.
Anthropic and OpenAI both have room for improvement on detailing their evaluation procedures. Anthropic’s RSP includes evaluation procedures for model autonomy and misuse risks. Its evaluation procedures for model autonomy are impressively detailed, including clearly defined tasks on which it will evaluate its models. Its evaluation procedures for misuse risk are much less well-defined, though it does include the following note: “We stress that this will be hard and require iteration. There are fundamental uncertainties and disagreements about every layer…It will take time, consultation with experts, and continual updating.” And OpenAI’s PF includes a “Model Scorecard,” a mock evaluation of an advanced AI model. This model scorecard includes the hypothetical results of various evaluations in all four of their tracked risk categories; it does not appear to be a comprehensive list of evaluation procedures.
Again, the science of AI model evaluation is young. The AI EO directs NIST to develop red-teaming guidance for developers of potentially dual-use foundation models. NIST, along with private actors such as METR and other AI evaluators, will play a crucial role in creating and testing red-teaming practices and model evaluations that elicit all relevant capabilities.
5. For different risk thresholds, preparedness frameworks should identify and commit to pre-specified risk mitigations.
Classes of risk mitigations may include:
- Restricting development and/or deployment of models at different risk thresholds
- Enhanced cybersecurity measures, to prevent exfiltration of model weights
- Internal compartmentalization and tiered access
- Interacting with the model only in restricted environments
- Deleting model weights8
Both OpenAI’s PF and Anthropic’s RSP commit to a number of pre-specified risk mitigations for different thresholds. For example, for what Anthropic calls “ASL-2” models (including its most advanced model, Claude 2), they commit to measures including publishing model cards, providing a vulnerability reporting mechanism, enforcing an acceptable use policy, and more. Models at higher risk thresholds (what Anthropic calls “ASL-3” and above) have different, more stringent risk mitigations, including “limit[ing] access to training techniques and model hyperparameters…” and “implement[ing] measures designed to harden our security…”
Risk mitigations can and should differ in approaches to development versus deployment. There are different levels of risk associated with possessing models internally and allowing external actors to interact with them. Both OpenAI’s PF and Anthropic’s RSP include different risk mitigation approaches for development and deployment. For example, OpenAI’s PF restricts deployment of models such that “Only models with a post-mitigation score of “medium” or below can be deployed,” whereas it restricts development of models such that “Only models with a post-mitigation score of “high” or below can be developed further.”
Mitigations should be defined as specifically as possible, with the understanding that as the state of the art changes, this too is an area that will require periodic updates. Developers should include some room for judgment here.
6. Preparedness frameworks’ pre-specified risk mitigations must effectively address potentially catastrophic risks.
Having confidence that the risk mitigations do in fact address potential catastrophic risks is perhaps the most important and difficult aspect of a PF to evaluate. Catastrophic risk from AI is a novel and speculative field; evaluating AI capabilities is a science in its infancy; and there are no empirical studies of the effectiveness of risk mitigations preventing such risks. Given this uncertainty, frontier AI developers should err on the side of caution.
Both OpenAI and Anthropic should be more conservative in their risk mitigations. Consider OpenAI’s commitment to restricting development: “[I]f we reach (or are forecasted to reach) ‘critical’ pre-mitigation risk along any risk category, we commit to ensuring there are sufficient mitigations in place…for the overall post-mitigation risk to be back at most to ‘high’ level.” To understand this commitment, we have to look at their threshold definitions. Under the Model Autonomy category, the “critical” threshold in part includes: “model can self-exfiltrate under current prevailing security.” Setting aside that this threshold is still quite vague and difficult to evaluate (and setting aside the novelty of this capability), a model that approaches or exceeds this threshold by definition can self-exfiltrate, rendering all other risk mitigations ineffective. A more robust approach to restricting development would not permit training or possessing a model that comes close to exceeding this threshold.
As for Anthropic, consider their threshold for “ASL-3,” which reads in part: “Access to the model would substantially increase the risk of catastrophic misuse…” The risk mitigations for ASL-3 models include the following: “Harden security such that non-state attackers are unlikely to be able to steal model weights and advanced threat actors (e.g. states) cannot steal them without significant expense.” While an admirable approach to development of potentially dual-use foundation models, assuming state actors seek out tools whose misuse involves catastrophic risk, a more conservative mitigation would entail hardening security such that it is unlikely that any actor, state or non-state, could steal the model weights of such a model.9
7. Preparedness frameworks should combine credible risk mitigation commitments with governance structures that ensure these commitments are fulfilled.
Preparedness Frameworks should detail governance structures that incentivize actually undertaking pre-committed risk mitigations when thresholds are met. Other incentives, including profit and shareholder value, sometimes conflict with risk management.
Anthropic’s RSP includes a number of procedural commitments meant to enhance the credibility of its risk mitigation commitments. For example, Anthropic commits to proactively planning to pause scaling of its models,10 publicly sharing evaluation results, and appointing a “Responsible Scaling Officer.” However, Anthropic’s RSP also includes the following clause: “[I]n a situation of extreme emergency, such as when a clearly bad actor (such as a rogue state) is scaling in so reckless a manner that it is likely to lead to lead to imminent global catastrophe if not stopped…we could envisage a substantial loosening of these restrictions as an emergency response…” This clause potentially undermines the credibility of Anthropic’s other commitments in the RSP, if at any time it can point to another actor who in its view is scaling recklessly.
OpenAI’s PF also outlines commendable governance measures, including procedural commitments, meant to enhance its risk mitigation credibility. It summarizes its operation structure: “(1) [T]here is a dedicated team “on the ground” focused on preparedness research and monitoring (Preparedness team), (2) there is an advisory group (Safety Advisory Group) that has a sufficient diversity of perspectives and technical expertise to provide nuanced input and recommendations, and (3) there is a final decision-maker (OpenAI Leadership, with the option for the OpenAI Board of Directors to overrule).”
8. Preparedness frameworks should include a mechanism for regular updates to the framework itself, in light of ongoing research and advances in AI.
Both OpenAI’s PF and Anthropic’s RSP acknowledge the importance of regular updates. This is reflected in both of these documents’ names: Anthropic labels its RSP as “Version 1.0,” while OpenAI’s PF is labeled as “(Beta).”
Anthropic’s RSP includes an “Update Process” that reads in part: “We expect most updates to this process to be incremental…as we learn more about model safety features or unexpected capabilities…” This language directly commits Anthropic to changing its RSP as the state of the art changes. OpenAI references updates throughout its PF, notably committing to updating its evaluation methods and rubrics (“The Scorecard will be regularly updated by the Preparedness team to help ensure it reflects the latest research and findings”).
9. For models with risk above the lowest level, most evaluation results and methods should be public, including any performed mitigations.
Publishing model evaluations and mitigations is an important tool for holding developers accountable to their PF commitments. Sensitivity about the level of transparency is key. For example, full information about evaluation methodology and risk mitigations could be exploited by malicious actors. Anthropic’s RSP takes a balanced approach in committing to “[p]ublicly share evaluation results after model deployment where possible, in some cases in the initial model card, in other cases with a delay if it serves a broad safety interest.” OpenAI’s PF does not commit to publishing its Model Scorecards, but OpenAI has since published related research on whether its models aid the creation of biological threats.
Conclusion
Preparedness frameworks represent a promising approach for AI developers to voluntarily commit to robust risk management practices. However, current versions have weaknesses—particularly their lack of specificity in risk thresholds, insufficiently conservative risk mitigation approaches, and inadequacy in addressing structural risks. Frontier AI developers without PFs should consider adopting them, and OpenAI and Anthropic should update their policies to strengthen risk mitigations and include more specificity.
Strengthening preparedness frameworks will require advancing AI safety science to enable precise risk quantification and develop new mitigations. NIST, academics, and companies plan to collaborate to measure and model frontier AI risks. Policymakers have a crucial opportunity to adapt regulatory approaches from other high-risk technologies like nuclear power to balance AI innovation and catastrophic risk prevention. Furthermore, standards bodies could develop more robust AI evaluations best practices, including guidance for third-party auditors.
Overall the AI community must view safety as an intrinsic priority, not just private actors creating preparedness frameworks. All stakeholders, including private companies, academics, policymakers and civil society organizations have roles to play in steering AI development toward societally beneficial outcomes. Preparedness frameworks are one tool, but not sufficient absent more comprehensive, multi-stakeholder efforts to scale AI safely and for the public good.
Many thanks to Madeleine Chang, Di Cooke, Thomas Woodside, and Felipe Calero Forero for providing helpful feedback.
Despite their importance, programs focused on AI trustworthiness form only a small fragment of total funding allocated for AI R&D by the National Science Foundation.
As Congress moves forward with the appropriations process, both the House and Senate have proposed various provisions related to artificial intelligence (AI) and machine learning (ML) across different spending bills.
The Federation of American Scientists (FAS) announced today its participation in the new AI Safety Institute Consortium (AISIC) launched by the National Institute of Standards and Technology (NIST)
The landscape of biosecurity risks related to AI is complex and rapidly changing, and understanding the range of issues requires diverse perspectives and expertise. Here are five promising ideas that match the diversity of challenges that AI poses in the life sciences.