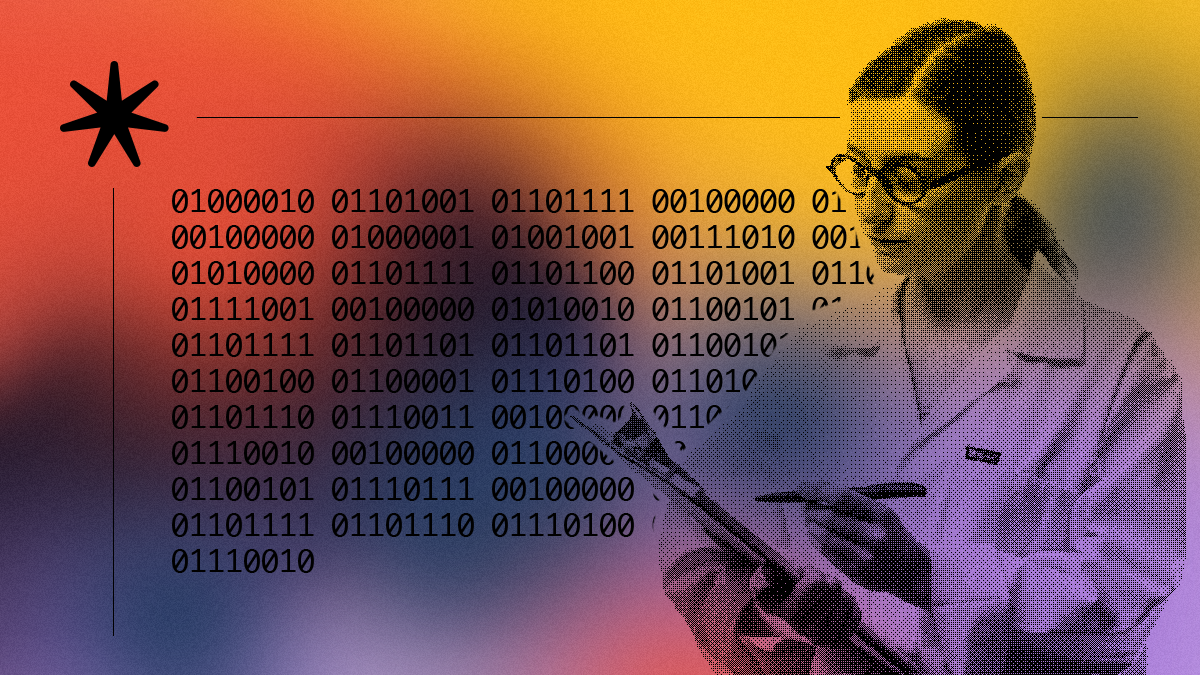
Bio x AI: Policy Recommendations for a New Frontier
Artificial intelligence (AI) is likely to yield tremendous advances in our basic understanding of biological systems, as well as significant benefits for health, agriculture, and the broader bioeconomy. However, AI tools, if misused or developed irresponsibly, can also pose risks to biosecurity. The landscape of biosecurity risks related to AI is complex and rapidly changing, and understanding the range of issues requires diverse perspectives and expertise. To better understand and address these challenges, FAS initiated the Bio x AI Policy Development Sprint to solicit creative recommendations from subject matter experts in the life sciences, biosecurity, and governance of emerging technologies. Through a competitive selection process, FAS identified six promising ideas and, over the course of seven weeks, worked closely with the authors to develop them into the recommendations included here. These recommendations cover a diverse range of topics to match the diversity of challenges that AI poses in the life sciences. We believe that these will help inform policy development on these topics, including the work of the National Security Commission on Emerging Biotechnologies.
AI tool developers and others have put significant effort into establishing frameworks to evaluate and reduce risks, including biological risks, that might arise from “foundation” models (i.e., large models designed to be used for many different purposes). These include voluntary commitments from major industry stakeholders, and several efforts to develop methods for evaluations of these models. The Biden Administration’s recent Executive Order on the Safe, Secure, and Trustworthy Development and Use of Artificial Intelligence (Bioeconomy EO) furthers this work and establishes a framework for evaluating and reducing risks related to AI.
However, the U.S. government will need creative solutions to establish oversight for biodesign tools (i.e., more specialized AI models that are trained on biological data and provide insight into biological systems). Although there are differing perspectives among experts, including those who participated in this Policy Sprint, about the magnitude of risks that these tools pose, they undoubtedly are an important part of the landscape of biosecurity risks that may arise from AI. Three of the submissions to this Policy Sprint address the need for oversight of these tools. Oliver Crook, a postdoctoral researcher at the University of Oxford and a machine learning expert, calls on the U.S. government to ensure responsible development of biodesign tools by instituting a framework for checklist-based, institutional oversight for these tools while Richard Moulange, AI-Biosecurity Fellow at the Centre for Long-Term Resilience, and Sophie Rose, Senior Biosecurity Policy Advisor at the Centre for Long-Term Resilience, expand on the Executive Order on AI with recommendations for establishing standards for evaluating their risks. In his submission, Samuel Curtis, an AI Governance Associate at The Future Society, takes a more open-science approach, with a recommendation to expand infrastructure for cloud-based computational resources internationally to promote critical advances in biodesign tools while establishing norms for responsible development.
Two of the submissions to this Policy Sprint work to improve biosecurity at the interface where digital designs might become biological reality. Shrestha Rath, a scientist and biosecurity researcher, focuses on biosecurity screening of synthetic DNA, which the Executive Order on AI highlights as a key safeguard, and contains recommendations for how to improve screening methods to better prepare for designs produced using AI. Tessa Alexanian, a biosecurity and bioweapons expert, calls for the U.S. government to issue guidance on biosecurity practices for automated laboratories, sometimes called “cloud labs,” that can generate organisms and other biological agents.
This Policy Sprint highlights the diversity of perspectives and expertise that will be needed to fully explore the intersections of AI with the life sciences, and the wide range of approaches that will be required to address their biosecurity risks. Each of these recommendations represents an opportunity for the U.S. government to reduce risks related to AI, solidify the U.S. as a global leader in AI governance, and ensure a safer and more secure future.
Recommendations
- Develop a Screening Framework Guidance for AI-Enabled Automated Labs by Tessa Alexanian
- An Evidence-Based Approach to Identifying and Mitigating Biological Risks From AI-Enabled Biological Tools by Richard Moulange & Sophie Rose
- A Path to Self-governance of AI-Enabled Biology by Oliver Crook
- A Global Compute Cloud to Advance Safe Science and Innovation by Samuel Curtis
- Establish Collaboration Between Developers of Gene Synthesis Screening Tools and AI Tools Trained on Biological Data by Shrestha Rath
- Responsible and Secure AI in Production Agriculture by Jennifer Clarke
Develop a Screening Framework Guidance for AI-Enabled Automated Labs
Tessa Alexanian
Protecting against the risk that AI is used to engineer dangerous biological materials is a key priority in the Executive Order on Safe, Secure, and Trustworthy Artificial Intelligence (AI EO). AI-engineered biological materials only become dangerous after digital designs are converted into physical biological agents, and biosecurity organizations have recommended safeguarding this digital-to-physical transition. In Section 4.4(b), the AI EO targets this transition by calling for standards and incentives that ensure appropriate screening of synthetic nucleic acids. This should be complemented by screening at another digital-to-physical interface: AI-enabled automated labs, such as cloud labs and self-driving labs.1
Laboratory biorisk management does not need to be reinvented for AI-enabled labs; existing US biosafety practices and Dual Use Research of Concern (DURC) oversight can be adapted and applied, as can emerging best practices for AI safety. However, the U.S. government should develop guidance that addresses two unique aspects of AI-enabled automated labs:
- Remote access to laboratory equipment may allow equipment to be misused by actors who would find it difficult to purchase or program it themselves.
- Unsupervised engineering of biological materials could produce dangerous agents without appropriate safeguards (e.g., if a viral vector regains transmissibility during autonomous experiments).
In short, guidance should ensure that providers of labs are aware of who is using the lab (customer screening), and what it is being used for (experiment screening).
It’s unclear precisely if and when automated labs will become broadly accessible to biologists, though a 2021 WHO horizon scan described them as posing dual-use concerns within five years. These are dual-use concerns, and policymakers must also consider the benefits that remotely accessible, high throughput, AI-driven labs offer for scientific discovery and biomedical innovation. The Australia Group discussed potential policy responses to cloud labs in 2019, including customer screening, experiment screening, and cybersecurity, though no guidance has been released. This is the right moment to develop screening guidance because automated labs are not yet widely used, but are attracting increasing investment and attention.
Recommendations
The evolution of U.S. policy on nucleic acid synthesis screening shows how the government can proactively identify best practices, issue voluntary guidance, allow stakeholders to test the guidance, and eventually require that federally-funded researchers procure from providers that follow a framework derived from the guidance.
Recommendation 1. Convene stakeholders to identify screening best practices
Major cloud lab companies already implement some screening and monitoring, and developers of self-driving labs recognize risks associated with them, but security practices are not standardized. The government should bring together industry and academic stakeholders to assess which capabilities of AI-enabled automated labs pose the most risk and share best practices for appropriate management of these risks.
As a starting point, aspects of the Administration for Strategic Preparedness and Response’s (ASPR) Screening Framework Guidance for synthetic nucleic acids can be adapted for AI-enabled automated labs. Labs that offer remote access could follow a similar process for customer screening, including verifying identity for all customers and verifying legitimacy for work that poses elevated dual-use concerns. If an AI system operating an autonomous or self-driving lab places a synthesis order for a sequence of concern, this could trigger a layer of human-in-the-loop approval.
Best practices will require cross-domain collaboration between experts in machine learning, laboratory automation, autonomous science, biosafety and biosecurity. Consortia such as the International Gene Synthesis Consortium and Global Biofoundy Alliance already have U.S. cloud labs among their members and may be a useful starting point for stakeholder identification.
Recommendation 2. Develop guidance based on these best practices
The Director of the Office of Science and Technology Policy (OSTP) should lead an interagency policy development process to create screening guidance for AI-enabled automated labs. The guidance will build upon stakeholder consultations conducted under Recommendation 1, as well as the recent ASPR-led update to the Screening Framework Guidance, ongoing OSTP-led consultations on DURC oversight, and OSTP-led development of a nucleic acid synthesis screening framework under Section 4.4(b) of the AI EO.
The guidance should describe processes for customer screening and experiment screening. It should address biosafety and biosecurity risks associated with unsupervised engineering of biological materials, including recommended practices for:
- Dual-use review for automated protocols. Automated protocols typically undergo human review because operators of automated labs don’t want to run experiments that fail. Guidance should outline when protocols should undergo additional review for dual-use; the categories of experiments in the DURC policy provide a starting point.
- Identifying biological agents in automated labs. When agents are received from customers, their DNA should be sequenced to ensure they have been labeled correctly. Agents engineered through unsupervised experiments should also be screened after some number of closed-loop experimental cycles.
Recommendation 3. Invest in predictive biology for risk mitigation
The Department of Homeland Security (DHS) and Department of Defense (DOD), building off the evaluation they will conduct under 4.4(a)(i) of the AI EO, should fund programs to develop predictive models to improve biorisk management in AI-enabled automated labs.
It is presently difficult to predict the behavior of biological systems, and there is little focus specifically on predictive biology for risk mitigation. AI could perform real-time risk evaluations and anomaly detection in self-driving labs; for example, autonomous science researchers have highlighted the need to develop models that can recognize novel compounds with potentially harmful properties. The government can actively contribute to innovation in this area; the IARPA Fun GCAT program, which developed methods to assess whether DNA sequences pose a threat, is an example of relevant government-funded AI capability development.
An evidence-based approach to identifying and mitigating biological risks from AI-enabled biological tools
Richard Moulange & Sophie Rose
Both AI-enabled biological tools and large language models (LLMs) have advanced rapidly in a short time. While these tools have immense potential to drive innovation, they could also threaten the United States’ national security.
AI-enabled biological tools refer to AI tools trained on biological data using machine learning techniques, such as deep neural networks. They can already design novel proteins, viral vectors and other biological agents, and may in the future be able to fully automate parts of the biomedical research and development process.
Sophisticated state and non-state actors could potentially use AI-enabled tools to more easily develop biological weapons (BW) or design them to evade existing countermeasures . As accessibility and ease of use of these tools improves, a broader pool of actors is enabled.
This threat was recognized by the recent Executive Order on Safe AI, which calls for evaluation of all AI models (not just LLMs) for capabilities enabling chemical, biological, radiological and nuclear (CBRN) threats, and recommendations for how to mitigate identified risks.
Developing novel AI-enabled biological tool -evaluation systems within 270 days, as directed by the Executive Order §4.1(b), will be incredibly challenging, because:
- There appears to have been little progress on developing benchmarks or evaluations for AI-enabled biological tools in academia or industry, and government capacity (in the U.S. and the UK) has so far focused on model evaluations for LLMs, not AI-enabled biological tools.
- Capabilities are entirely dual-use: for example, tools that can predict which viral mutations improve vaccine targeting can very likely identify mutations that increase vaccine evasion.
To achieve this, it will be important to identify and prioritize those AI-enabled biological tools that pose the most urgent risks, and balance these against the potential benefits. However, government agencies and tool developers currently seem to struggle to:
- Specify which AI–bio capabilities are the most concerning;
- Determine the scope of AI–enabled tools that pose significant biosecurity risks; and
- Anticipate how these risks might evolve as more tools are developed and integrated
Some frontier AI labs have assessed the biological risks associated with LLMs , but there is no public evidence of AI-enabled biological tool evaluation or red-teaming, nor are there currently standards for developing—or requirements to implement—them. The White House Executive Order will build upon industry evaluation efforts for frontier models, addressing the risk posed by LLMs, but analogous efforts are needed for AI-enabled biological tools.
Given the lack of research on AI-enabled biological tool evaluation, the U.S. Government must urgently stand up a specific program to address this gap and meet the Executive Order directives. Without evaluation capabilities, the United States will be unable to scope regulations around the deployment of these tools, and will be vulnerable to strategic surprise. Doing so now is essential to capitalize on the momentum generated by the Executive Order, and comprehensively address the relevant directives within 270 days.
Recommendations
The U.S. Government should urgently acquire the ability to evaluate biological capabilities of AI-enabled biological tools via a specific joint program at the Departments of Energy (DOE) and Homeland Security (DHS), in collaboration with other relevant agencies.
Strengthening the U.S. Government’s ability to evaluate models prior to their deployment is analogous to responsible drug or medical device development: we must ensure novel products do not cause significant harm, before making them available for widespread public use.
The objective(s) of this program would be:
- Develop state-of-the-art evaluations for dangerous biological capabilities
- Establish Department of Energy (DOE) sandbox for testing evaluations on a variety of AI-enabled biological tools
- Produce standards for performance, structure and securitisation of capability evaluations
- Use evaluations of the maturity and capabilities of AI-enabled biological tools to inform U.S. Intelligence Community assessments of potential adversaries’ current bio-weapon capabilities
Implementation
- Standing up and sustaining DOE and DHS’s ‘Bio Capability Evaluations’ program will require an initial investment of $2 million USD and $2 million/year until 2030 to sustain. Funding should draw on existing National Intelligence Program appropriations.
- Supporting DOE to establish a sandbox for conducting ongoing evaluations of AI-enabled biological tools will require investment of $10 million annually. This could be appropriated to DOE under the National Defense Authorization Act (Title II: Research, Development, Test and Evaluation), which establishes funding for AI defense programs.
Lead agencies and organizations
- U.S. Department of Energy (DOE) can draw on expertise from National Labs, which often evaluate—and develop risk mitigation measures for—technologies with CBRN implications.
- U.S. Department of Homeland Security (DHS) can inform threat assessments and inform biological risk mitigation strategy and policy.
- National Institute for Standards and Technology (NIST) can develop the standards for the performance, structure and securitization of dangerous capability evaluations.
- U.S. Department of Health and Human Services (HHS) can leverage their AI Community of Practice (CoP) as an avenue for communicating with BT developers and researchers. The National Institutes of Health (NIH) funds relevant research and will therefore need to be involved in evaluations.
They should coordinate with other relevant agencies, including but not limited to the Department of Defense, and the National Counterproliferation and Biosecurity Center.
The benefits of implementing this program include:
Leveraging public-private expertise. Public-private partnerships (involving both academia and industry) will produce comprehensive evaluations that incorporate technical nuances and national security considerations. This allows the U.S. Government to retain access to diverse expertise whilst safeguarding the sensitive nature of dangerous capability evaluations contents and output—which is harder to guarantee with third-party evaluators.
Enabling evidence-based regulatory decision-making. Evaluating AI tools allows the U.S. Government to identify the models and capabilities that pose the greatest biosecurity risks, enabling effective and appropriately-scoped regulations. Avoiding blanket regulations results in a better balance of the considerations of innovation and economic growth with those of risk mitigation and security.
Broad scope of evaluation application. AI-enabled biological tools vary widely in their application and current state of maturity. Subsequently, what constitutes a concerning, or dangerous, capability may vary widely across tools, necessitating the development of tailored evaluations.
A path to self-governance of AI-enabled biology
Oliver Crook
Artificial intelligence (AI) and machine learning (ML) are being increasingly employed for the design of proteins with specific functions. By adopting these tools, researchers have been able to achieve high success rates designing and generating proteins with certain properties. This will accelerate the design of new medical therapies such as antibodies, vaccines and biotechnologies such as nanopores. However, AI-enabled biology could also be used for malicious – rather than benevolent – purposes. Despite this potential for misuse, there is little to no oversight over what tools can be developed, the data they can be trained on, and how the developed tool can be deployed. While more robust guardrails are needed, any proposed regulation must also be balanced, so that it encourages responsible innovation.
AI-enabled biology is still a specialized methodology that requires significant technical expertise, access to powerful computational resources, and sufficient quantities of data. As the performance of these models increases, their potential for generating significantly harmful agents grows as well. With AI-enabled biology becoming more accessible, the value of guardrails early on in the development of this technology is paramount before widespread technology proliferation makes it challenging – or impossible – to govern. Furthermore, smart policies implemented now can allow us to better monitor the pace of development, and guide reasonable and measured policy in the future.
Here, we propose that fostering self-governance and self-reporting is a scalable approach to this policy challenge. During the research, development and deployment (RDD) phases, practitioners report on a pre-decided checklist and make an ethics declaration. While advancing knowledge is an academic imperative, funders, editors, and institutions need to be fully aware of the risks of some research and have opportunities to adjust the RDD plan, as needed, to ensure that AI models are developed responsibly. Whilst similar policies have already been introduced by some machine learning venues (1, 2, 3), the proposal here seeks to strengthen, formalize and broaden the scope of those proposals. Ultimately, the checklist and ethics declarations seek confirmation from multiple parties during each of the RDD phases that the research is of fundamental public good. We recommend that the National Institutes of Health (NIH) leads on this policy challenge and builds upon decades of experience on related issues.
The recent executive order framework for safe AI provides an opportunity to build upon initial recommendations on reporting but with greater specificity on AI-enabled biology. The proposal fits squarely into the desire under section 4.4 for the executive order to reduce the misuse of AI to assist in the development and design of biological weapons.
Recommendations
We propose the following recommendations:
Recommendation 1. With leadership from the NIH Office of Science Policy, life sciences funding agencies should coordinate development of a checklist in consultation with AI-enabled biology model developers, non-government funders, publishers, and nonprofit organizations that evaluates risks and benefits of the model.
The checklist should take the form of a list of pre-specified questions and guided free-form text. The questions should gather basic information about the models employed: their size, their compute usage and the data they were trained on. This will allow them to be characterized in comparison with existing models. The intended use of the model should be stated along with any dual-use behavior of the model that has already been identified. The document should also reveal whether any strategies have been employed to mitigate the harmful capabilities that the model might demonstrate.
At each stage of the RDD, the predefined checklist for that stage is completed and submitted to the institution.
Recommendation 2. Each institute employing AI-enabled biology across RDD should elect a small internally-led, cross-disciplinary committee to examine and evaluate, at each phase, the submitted checklists. To reduce workload, only models that fall under the executive order specifications or dual use research of concern (DURC) should be considered. The committee makes recommendations based on the value of the work. The committee then posts their proceedings of meetings publicly (as for Institutional Biosafety Committees), except for publicly sensitive intellectual property. If the benefit of the work cannot be evaluated or the outcomes are largely unpredictable the committee should work with the model developer to adjust the RDD plan, as needed. The checklist and institutional signature are then made available to NIH and funding agencies and, upon completion of the project, such as at publication, the checklists are made publicly available.
By following these recommendations, high-risk research will be caught at an institutional level and internal recommendations can facilitate timely mitigation of harms. Public release of committee deliberations and ethics checklists will enable third parties to scrutinize model development and raise concerns. This approach ensures a hierarchy of oversight that allows individuals, institutes, funders and governments to identify and address risks before AI models are developed rather than after the work has been completed.
We recommend that $5 million dollars be provided to the NIH Office of Science Policy to implement this policy. This money would cover hiring a ‘Director of Ethics of AI-enabled Biology’ to oversee this research and several full time researchers/administrators ($1.5 million). These employees should conduct outreach to the institutes to ensure that the policy is understood, to answer any questions, and to facilitate community efforts to develop and update the checklist ($1 million). Additional grants should be made available to allow researchers and non-profit organizations to audit the checklists and committees, evaluate the checklists, and research the socio-technological implications of the checklists ($1.5 million). The rapid pace of development of AI means that the checklists will need to be reevaluated on a yearly basis, with $1 million of funding available to evaluate the impact of these grants. Funding should grow inline with the pace of technological development. Specific subareas of AI-enabled biology may need specific checklists depending on their risk profile.
This recommendation is scalable; once the checklists have been made, the majority of the work is placed in the hands of practitioners rather than government. In addition, these checklists provide valuable information to inform future governance agendas. For example, limiting computational resources to curtail dangerous applications (compute governance) cannot proceed without detailed understanding of how much compute is required to achieve certain goals. Furthermore, it places responsibility on practitioners requiring them to engage with the risk that could arise from their work, with institutes having the ability to make recommendations on how to reduce the risks from models. This approach draws on similar frameworks that support self-governance, such as oversight by Institutional Biosafety Committees (IBCs). This self-governance proposal is well complemented by alternative policies around open access of AI-enabled biology tools, as well as policies strengthening DNA synthesis screening protocols to catch misuse at different places along a broadly-defined value chain.
A Global Compute Cloud to Advance Safe Science and Innovation
Samuel Curtis
Advancements in deep learning have ushered in significant progress in the predictive accuracy and design capabilities of biological design tools (BDTs), opening new frontiers in science and medicine through the design of novel functional molecules. However, these same technologies may be misused to create dangerous biological materials. Mitigating the risks of misuse of BDTs is complicated by the need to maintain openness and accessibility among globally-distributed research and development communities. One approach toward balancing both risks of misuse and the accessibility requirements of development communities would be to establish a federally-funded and globally-accessible compute cloud through which developers could provide secure access to their BDTs.
The term “biological design tools” (or “BDTs”) is a neologism referring to “systems trained on biological data that can help design new proteins or other biological agents.” Computational biological design is, in essence, a data-driven optimization problem. Consequently, over the past decade, breakthroughs in deep learning have propelled progress in computational biology. Today, many of the most advanced BDTs incorporate deep learning techniques and are used and developed by networks of academic researchers distributed across the globe. For example, the Rosetta Software Suite, one of the most popular BDT software packages, is used and developed by Rosetta Commons—an academic consortium of over 100 principal investigators spanning five continents.
Contributions of BDTs to science and medicine are difficult to overstate. There are already several AI-designed molecules in early-stage clinical trials. BDTs are now used to identify new drug targets, design new therapeutics, and construct faster and less expensive drug synthesis techniques. There are already several AI-designed molecules in early-stage clinical trials.
Unfortunately, these same BDTs can be used for harm. They may be used to create pathogens that are more transmissible or virulent than known agents, target specific sub-populations, or evade existing DNA synthesis screening mechanisms. Moreover, developments in other classes of AI systems portend reduced barriers to BDT misuse. One group at RAND Corporation found that language models could provide guidance that could assist in planning and executing a biological attack, and another group from MIT demonstrated how language models could be used to elicit instructions for synthesizing a potentially pandemic pathogen. Similarly, language models could accelerate the acquisition or interpretation of information required to misuse BDTs. Technologies on the horizon, such as multimodal “action transformers,” could help individuals navigate BDT software, further lowering barriers to misuse.
Research points to several measures BDT developers could employ to reduce risks of misuse, such as securing machine learning model weights (the numerical values representing the learned patterns and information that the model has acquired during training), implementing structured access controls, and adopting Know Your Customer (KYC) processes. However, precaution would have to be taken to not unduly limit access to these tools, which could, in aggregate, impede scientific and medical advancement. For any given tool, access limitations risk diminishing its competitiveness (its available features and performance relative to other tools). These tradeoffs extend to their developers’ interests, whereby stifling the development of tools may jeopardize research, funding, and even career stability. The difficulties of striking a balance in managing risk are compounded by the decentralized, globally-distributed nature of BDT development communities. To suit their needs, risk-mitigation measures should involve minimal, if any, geographic or political restrictions placed on access while simultaneously expanding the ability to monitor for and respond to indicators of risk or patterns of misuse.
One approach that would balance the simultaneous needs for accessibility and security would be for the federal government to establish a global compute cloud for academic research, bearing the costs of running servers and maintaining the security of the cloud infrastructure in the shared interests of advancing public safety and medicine. A compute cloud would enable developers to provide access to their tools through computing infrastructure managed—and held to specific security standards—by U.S. public servants. Such infrastructure could even expand access for researchers, including underserved communities, through fast-tracked grants in the form of computational resources.
However, if computing infrastructure is not designed to reflect the needs of the development community—namely, its global research community—it is unlikely to be adopted in practice. Thus, to fully realize the potential of a compute cloud among BDT development communities, access to the infrastructure should extend beyond U.S. borders. At the same time, the efforts should ensure the cloud has requisite monitoring capabilities to identify risk indicators or patterns of misuse and impose access restrictions flexibly. By balancing oversight with accessibility, a thoughtfully-designed compute cloud could enable transparency and collaboration while mitigating the risks of these emerging technologies.
Recommendations
The U.S. government should establish a federally-funded, globally-accessible compute cloud through which developers could securely provide access to BDTs. In fact, the Biden Administration’s October 2023 “Executive Order on the Safe, Secure, and Trustworthy Development and Use of Artificial Intelligence” (the “AI EO”) lays groundwork by establishing a pilot program of a National AI Research Resource (NAIRR)—a shared research infrastructure providing AI researchers and students with expanded access to computational resources, high-quality data, educational tools, and user support. Moving forward, to increase the pilot program’s potential for adoption by BDT developers and users, relevant federal departments and agencies should take concerted action in the timelines circumscribed by the AI EO to address the practical requirements of BDT development communities: the simultaneous need to expand access outside U.S. borders while bolstering the capacity to monitor for misuse.
It is important to note that a federally-funded compute cloud has been years in the making. The National AI Initiative Act of 2020 directed the National Science Foundation (NSF), in consultation with the Office of Science and Technology Policy (OSTP), to establish a task force to create a roadmap for the NAIRR. In January 2023, the NAIRR Task Force released its final report, “Strengthening and Democratizing the U.S. Artificial Intelligence Innovation Ecosystem,” which presented a detailed implementation plan for establishing the NAIRR. The Biden Administration’s AI EO then directed the Director of NSF, in coordination with the heads of agencies deemed appropriate by the Director, to launch a pilot program “consistent with past recommendations of the NAIRR Task Force.”
However, the Task Force’s past recommendations are likely to fall short of the needs of BDT development communities (not to mention other AI development communities). In its report, the Task Force described NAIRR’s primary user groups as “U.S.-based AI researchers and students at U.S. academic institutions, non-profit organizations, Federal agencies or FFRDCs, or startups and small businesses awarded [Small Business Innovation Research] or [Small Business Technology Transfer] funding,” and its resource allocation process is oriented toward this user base. Separately, Stanford University’s Institute for Human-centered AI (HAI) and the National Security Commission on Artificial Intelligence (NSCAI) have proposed institutions, building upon or complementing NAIRR, that would support international research consortiums (a Multilateral AI Research Institute and an International Digital Democracy Initiative, respectively), but the NAIRR Task Force’s report—upon which the AI EO’s pilot program is based—does not substantively address this user base.
In launching the NAIRR pilot program under Sec. 5.2(a)(i), the NSF should put the access and security needs of international research consortiums front and center, conferring with heads of departments and agencies with relevant scope and expertise, such as the Department of State, US Agency for International Development (USAID), Department of Education, the National Institutes of Health, and the Department of Energy. The NAIRR Operating Entity (as defined in the Task Force’s report) should investigate how funding, resource allocation, and cybersecurity could be adapted to accommodate researchers outside of U.S. borders. In implementing the NAIRR pilot program, the NSF should incorporate BDTs in their development of guidelines, standards, and best practices for AI safety and security, per Sec. 4.1, which could serve as standards with which NAIRR users should be required to comply. Furthermore, the NSF Regional Innovation Engine launched through Sec. 5(a)(ii) should consider focusing on international research collaborations, such as those in the realm of biological design.
Besides the NSF, which is charged with piloting NAIRR, relevant departments and agencies should take concerted action in implementing the AI EO to address issues of accessibility and security that are intertwined with international research collaborations. This includes but is not limited to:
- In accordance with Sec. 5.2(a)(i), the departments and agencies listed above should be tasked with investigating the access and security needs of international research collaborations and include these in the reports they are required to submit to the NSF. This should be done in concert with the development of guidelines, standards, and best practices for AI safety and security required by Sec. 4.1.
- In fulfilling the requirements of Sec. 5.2(c-d), the Under Secretary of Commerce for Intellectual Property, the Director of the United States Patent and Trademark Office, and the Secretary of Homeland Security should, in the reports and guidance on matters related to intellectual property that they are required to develop, clarify ambiguities and preemptively address challenges that might arise in the cross-border data use agreements.
- Under the terms of Sec. 5.2(h), the President’s Council of Advisors on Science and Technology should, in its development of “a report on the potential role of AI […] in research aimed at tackling major societal and global challenges,” focus on the nature of decentralized, international collaboration on AI systems used for biological design.
- Pursuant to Sec. 11(a-d), the Secretary of State, the Assistant to the President for National Security Affairs, the Assistant to the President for Economic Policy, and the Director of OSTP should focus on AI used for biological design as a use case for expanding engagements with international allies and partners, and establish a robust international framework for managing the risks and harnessing the benefits of AI. Furthermore, the Secretary of Commerce should make this use case a key feature of its plan for global engagement in promoting and developing AI standards.
The AI EO provides a window of opportunity for the U.S. to take steps toward mitigating the risks posed by BDT misuse. In doing so, it will be necessary for regulatory agencies to proactively seek to understand and attend to the needs of BDT development communities, which will increase the likelihood that government-supported solutions, such as the NAIRR pilot program—and potentially future fully-fledged iterations enacted via Congress—are adopted by these communities. By making progress toward reducing BDT misuse risk while promoting safe, secure access to cutting-edge tools, the U.S. could affirm its role as a vanguard of responsible innovation in 21st-century science and medicine.
Establish collaboration between developers of gene synthesis screening tools and AI tools trained on biological data
Shrestha Rath
Biological Design Tools (BDTs) are a subset of AI models trained on genetic and/or protein data developed for use in life sciences. These tools have recently seen major performance gains, enabling breakthroughs like accurate protein structure predictions by AlphaFold2, and alleviating a longstanding challenge in life sciences.
While promising for legitimate research, BDTs risk misuse without oversight. Because universal screening of gene synthesis is currently lacking, potential threat agents could be digitally designed with assistance from BDTs and then physically made using gene synthesis. BDTs pose particular challenges because:
- The growing number of gene synthesis orders evade current screening capabilities. Industry experts in gene synthesis companies report that a small but concerning portion of orders for synthetic nucleic acid sequences show little or no homology with known sequences in widely-used genetic databases and so are not captured by current screening techniques. Advances in BDTs are likely going to make such a scenario more common, thus exacerbating the risk of misuse of synthetic DNA. The combined use of BDTs and gene synthesis has the potential to aid the “design” and “build” steps for malicious misuse. Strengthening screening capabilities to keep up with advances in BDTs is an attractive early intervention point to prevent this misuse.
- Potential for substantial breakthroughs in BDTs. While BDTs for applications beyond protein design face significant challenges, and most are not yet mature, companies are likely to invest in generating data to improve these tools because they see significant economic value in doing so. Moreover, some AI experts speculate that if we used the same amount of computational resources as LLMs when training protein language models, we could significantly improve their performance. Thus there is significant uncertainty about rapid advances in BDTs and how they may affect the potential for misuse.
BDT development is currently concentrated among a few actors, which makes policy implementation tractable, but risks will decentralize over time away from a handful of well-resourced academic labs and private AI companies. The U.S. government should take advantage of this unique window of opportunity to implement policy guardrails while the next generation of advanced BDTs are in development.
In short, it is important that developers of BDTs work together with developers and users of gene synthesis screening tools. This will promote shared understanding of the risks around potential misuse of synthetic nucleic acids, which may be exacerbated by advances in AI.
By bringing together key stakeholders to share information and align on safety standards the U.S. government can steer these technologies to maximize benefits and minimize widespread harms. Section 4.4 (b) of the Executive Order on the Safe, Secure, and Trustworthy Development and Use of Artificial Intelligence (henceforth referred to as the “Executive Order”) also emphasizes mitigating risks from the “misuse of synthetic nucleic acids, which could be substantially increased by AI’s capabilities”.
Gene synthesis companies and/or organizations involved in developing gene screening mechanisms are henceforth referred to as “DNA Screener”. Academic and for-profit stakeholders developing BDTs are henceforth referred to as “BDT Developer”. Gene synthesis companies providing synthetic DNA as a service irrespective of their screening capabilities are referred to as “DNA Provider”.
Recommendations
By bringing together key stakeholders (BDT developers, DNA screeners, and security experts) to share information and align on safety standards, the U.S. government can steer these technologies to maximize benefits and minimize widespread harms. Implementing these recommendations requires allocating financial resources and coordinating interagency work.
There are near-term and long-term opportunities to improve coordination between DNA Screeners, DNA Providers (that use in-house screening mechanisms) and BDT Developers, such as to avoid future potential backlash, over-regulation and legal liability. As part of the implementation of Section 4.4 of the Executive Order, the Department of Energy and the National Institute for Standards and Technology should:
Recommendation 1. Convene BDT Developers, DNA Screeners along with ethics, security and legal experts to a) Share information on AI model capabilities and their implications for DNA sequence screening; and b) Facilitate discussion on shared safeguards and security standards. Technical security standards may include adversarial training to make the AI models robust against purposeful misuse, BDTs refusing to follow user requests when the requested action may be harmful (refusals and blacklisting), and maintaining user logs.
Recommendation 2. Create an advisory group to investigate metrics that measure performance of protein BDTs for DNA Screeners, in line with Section 4.4 (a)(ii)(A) of the Executive Order. Metrics that capture BDT performance and thus risk posed by advanced BDTs would give DNA Screeners helpful context while screening orders. For example, some current methods for benchmarking AI-enabled protein design methods focus on sequence recovery, where the backbones of natural proteins with known amino-acid sequences are passed as the input and the accuracy of the method is measured by identity between the predicted sequence and the true sequence.
Recommendation 3. Support and fund development of AI-enabled DNA screening mechanisms that will keep pace with BDTs. U.S. national laboratories should support these types of efforts because commercial incentives for such tools are lacking. IARPA’s Fun GCAT is an exemplary case in this regard.
Recommendation 4. Conduct structured red teaming for current DNA screening methods to ensure they account for functional variants of Sequence Of Concern (SOCs) that may be developed with the help of BDTs and other AI tools. Such red teaming exercises should include expert stakeholders involved in development of screening mechanisms and national security community.
Recommendation 5. Establish both policy frameworks and technical safeguards for identification of certifiable origins. Designs produced by BDTs could require a cryptographically signed certificate detailing the inputs used in the design process of their synthetic nucleic acid order, ultimately providing useful contextual information aids DNA Screeners to check for harmful intent captured in the requests made to the model.
Recommendation 6. Fund third-party evaluations of BDTs to determine how their use might affect DNA sequence screening and provide this information to those performing screening. Having these evaluations would be helpful for new, small and growing DNA Providers and alleviate the burden on established DNA Providers as screening capabilities become more sophisticated. A similar system exists in the automobile industry where insurance providers conduct their own car safety and crash tests to inform premium-related decisions.
Proliferation of open-source tools accelerates innovation and democratization of AI, and is a growing concern in the context of biological misuse. The recommendations here strengthen biosecurity screening at a key point in realizing these risks. This framework could be implemented alongside other approaches to reduce the risks that arise from BDTs. These include: introducing Know Your Customer (KYC) frameworks that monitor buyers/users of AI tools; requiring BDT developers to undergo training on assessing and mitigating dual-use risks of their work; and encouraging voluntary guidelines to reduce misuse risks, for instance, by employing model evaluations prior to release, refraining from publishing preprints or releasing model weights until such evaluations are complete. This multi-pronged approach can help ensure that AI tools are developed responsibly and that biosecurity risks are managed.
Responsible and Secure AI in Production Agriculture
Jennifer Clarke
Agriculture, food, and related industries represent over 5% of domestic GDP. The health of these industries has a direct impact on domestic food security, which equates to a direct impact on national security. In other words, food security is biosecurity is national security. As the world population continues to increase and climate change brings challenges to agricultural production, we need an efficiency and productivity revolution in agriculture. This means using less land and natural resources to produce more food and feed. For decision-makers in agriculture, the lack of human resources and narrow economic margins are driving interest in automation and properly utilizing AI to help increase productivity while decreasing waste amid increasing costs.
Congress should provide funding to support the establishment of a new office within the USDA to coordinate, enable, and oversee the use of AI in production agriculture and agricultural research.
The agriculture, food, and related industries are turning to AI technologies to enable automation and drive the adoption of precision agriculture technologies. The use of AI in agriculture often depends on proprietary approaches that have not been validated by an independent, open process. In addition, it is unclear whether AI tools aimed at the agricultural sector will address critical needs as identified by the producer community. This leads to the potential for detrimental recommendations and loss of trust across producer communities. These will impede adoption of precision agriculture technologies, which is necessary to domestic and sustainable food security.
The industry is promoting AI technologies to help yield healthier crops, control pests, monitor soil and growing conditions, organize data for farmers, help with workload, and improve a wide range of agriculture-related tasks in the entire food supply chain.
However, the use of networked technologies approaches in agriculture poses risks. AI use could add to this problem if not implemented carefully. For example, the use of biased or irrelevant data in AI development can result in poor performance, which erodes producer trust in both extension services and expert systems, hindering adoption. As adoption increases, it is likely that farmers will use a small number of available platforms; this creates centralized points of failure where a limited attack can cause disproportionate harm. The 2021 cyberattack on JBS, the world’s largest meat processor, and a 2021 ransomware attack on NEW Cooperative, which provides feed grains for 11 million farm animals in the United States, demonstrate the potential risks from agricultural cybersystems. Without established cybersecurity standards for AI systems, those systems with broad adoption across agricultural sectors will represent targets of opportunity.
As evidenced by the recent Executive Order on the Safe Secure and Trustworthy Development and Use of Artificial Intelligence and AI Safety Summit held at Bletchley Park, there is considerable interest and attention being given to AI governance and policy by both national and international regulatory bodies. There is a recognition that the risks of AI require more attention and investments in both technical and policy research.
This recognition dovetails with an increase in emphasis on the use of automation and AI in agriculture to enable adoption of new agricultural practices. Increased adoption in the short term is required to reduce greenhouse gas emissions and ensure sustainability of domestic food production. Unfortunately, trust in commercial and governmental entities among agricultural producers is low and has been eroded by corporate data policies. Fortunately, this erosion can be reversed by prompt action on regulation and policy that respects the role of the producer in food and national security. Now is the time to promote the adoption of best practices and responsible development to establish security as a habit among agricultural stakeholders.
Recommendations
To ensure that the future of domestic agriculture and food production leverages the benefits of AI while mitigating the risks of AI, the U.S. government should invest in institutional cooperation; AI research and education; and development and enforcement of best practices.
Recommendation: An Office should be established within USDA focused on AI in Production Agriculture, and Congress should appropriate $5 million over the next 5 years for a total of $25 million for this office. Cooperation among multiple institutions (public, private, nonprofit) will be needed to provide oversight on the behavior of AI in production agriculture including the impact of non-human algorithms and data sharing agreements (“the algorithmic economy”). This level of funding will encourage both federal and non-federal partners to engage with the Office and support its mission. This Office should establish and take direction from an Advisory body led by USDA with inclusive representation across stakeholder organizations including industry (e.g., AgGateway, Microsoft, John Deere), nonprofit organizations (e.g., AgDataTransparent, American Farmland Trust, Farm Bureaus, Ag Data Coalition, Council for Agricultural Science and Technology (CAST), ASABE, ISO), government (e.g., NIST, OSTP), and academia (e.g., APLU, Ag Extension). This advisory body will operate under the Federal Advisory Committee Act (FACA) to identify challenges and recommend solutions, e.g., develop regulations or other oversight specific to agricultural use of AI, including data use agreements and third-party validation, that reduces the uncertainty about risk scenarios and the effect of countermeasures. The office and its advisory body can solicit broad input on regulation, necessary legislation, incentives and reforms, and enforcement measures through Requests for Information and Dear Colleague letters. It should promote best practices as described below, i.e., incentivize responsible use and adoption, through equitable data governance, access, and private-public partnerships. An example of an incentive is providing rebates to producers who purchase equipment that utilizes validated AI technology.
To support development of best practices for the use of AI in production agriculture, in partnership with NIH, NSF, and DOD/DOE, the proposed Office should coordinate funding for research and education on the sociotechnical context of AI in agriculture across foundational disciplines including computer science, mathematics, statistics, psychology, and sociology. This new discipline of applied AI (built on theoretical advances in AI since the 1950s) should provide a foundation for developing best practices for responsible AI development starting with general, accepted standards (e.g., NIST’s framework). For example, best practices may include transparency through the open source community and independent validation processes for models and software. AI model training requires an immense amount of data and AI models for agriculture will require many types of data sets specific to production systems (e.g., weather, soil, management practices, etc.). There is an urgent need for standards around data access and use that balance advances and adoption of precision agriculture with privacy and cybersecurity concerns.
In support of the work of the proposed Office, Congress should appropriate funding at $20M/year to USDA to support the development of programs at land-grant universities that provide multidisciplinary training in AI and production agriculture. The national agricultural production cyberinfrastructure (CI) has become critical to food security and carbon capture in the 21st century. A robust talent pipeline is necessary to support, develop, and implement this CI in preparation for the growth in automation and AI. There is also a critical need for individuals trained in both AI and production agriculture who can lead user-centered design and digital services on behalf of producers. Training must include foundation knowledge of statistics, computer science, engineering, and agricultural sciences coupled with experiential learning that provide trainees with opportunities to translate their knowledge to address current CI challenges. These opportunities may arise from interagency cooperation at the federal, state, and local levels, in partnership with grower cooperatives, farm bureaus, and land-grant universities, to ensure that training meets pressing and future needs in agricultural systems.
By advocating for the integration of technology-focused green jobs within federal initiatives, there is an opportunity to broaden the talent pool and harness the potential of emerging technologies to tackle pressing environmental issues.
“We really wanted a range of perspectives – specifically from voices that have been traditionally left out of the conversation”
Understanding the implications of climate change in agriculture and forestry is crucial for our nation to forge ahead with effective strategies and outcomes.
In the quest for sustainable energy and materials, biomass emerges as a key player, bridging the gap between the energy sector and the burgeoning U.S. and regional bioeconomies.