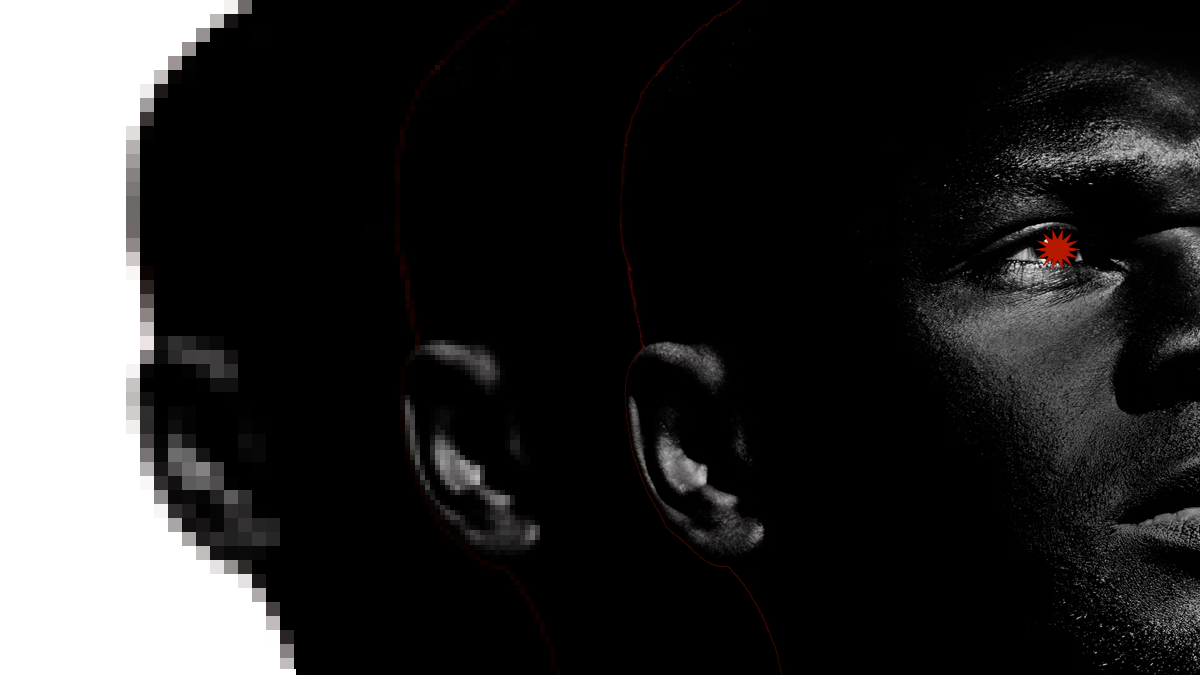
How Do OpenAI’s Efforts To Make GPT-4 “Safer” Stack Up Against The NIST AI Risk Management Framework?
In March, OpenAI released GPT-4, another milestone in a wave of recent AI progress. This is OpenAI’s most advanced model yet, and it’s already being deployed broadly to millions of users and businesses, with the potential for drastic effects across a range of industries.
But before releasing a new, powerful system like GPT-4 to millions of users, a crucial question is: “How can we know that this system is safe, trustworthy, and reliable enough to be released?” Currently, this is a question that leading AI labs are free to answer on their own–for the most part. But increasingly, the issue has garnered greater attention as many have become worried that the current pre-deployment risk assessment and mitigation methods like those done by OpenAI are insufficient to prevent potential risks, including the spread of misinformation at scale, the entrenchment of societal inequities, misuse by bad actors, and catastrophic accidents.
This concern is central to a recent open letter, signed by several leading machine learning (ML) researchers and industry leaders, which calls for a 6-month pause on the training of AI systems “more powerful” than GPT-4 to allow more time for, among other things, the development of strong standards which would “ensure that systems adhering to them are safe beyond a reasonable doubt” before deployment. There’s a lot of disagreement over this letter, from experts who contest the letter’s basic narrative, to others who think that the pause is “a terrible idea” because it would unnecessarily halt beneficial innovation (not to mention that it would be impossible to implement). But almost all of the participants in this conversation tend to agree, pause or no, that the question of how to assess and manage risks of an AI system before actually deploying it is an important one.
A natural place to look for guidance here is the National Institute of Standards and Technology (NIST), which released its AI Risk Management Framework (AI RMF) and an associated playbook in January. NIST is leading the government’s work to set technical standards and consensus guidelines for managing risks from AI systems, and some cite its standard-setting work as a potential basis for future regulatory efforts.
In this piece we walk through both what OpenAI actually did to test and improve GPT-4’s safety before deciding to release it, limitations of this approach, and how it compares to current best practices recommended by the National Institute of Standards and Technology (NIST). We conclude with some recommendations for Congress, NIST, industry labs like OpenAI, and funders.
What did OpenAI do before deploying GPT-4?
OpenAI claims to have taken several steps to make their system “safer and more aligned”. What are those steps? OpenAI describes these in the GPT-4 “system card,” a document which outlines how OpenAI managed and mitigated risks from GPT-4 before deploying it. Here’s a simplified version of what that process looked like:
- They brought in over 50 “red-teamers,” outside experts across a range of domains to test the model, poking and prodding at it to find ways that it could fail or cause harm. (Could it “hallucinate” in ways that would contribute to massive amounts of cheaply produced misinformation? Would it produce biased/discriminatory outputs? Could it help bad actors produce harmful pathogens? Could it make plans to gain power of its own?)
- Where red-teamers found ways that the model went off the rails, they could train out many instances of undesired outputs via Reinforcement Learning on Human Feedback (RLHF), a process in which human raters give feedback on the kinds of outputs provided by the model (both through human-generated examples of how a model should respond given some type of input, and with “thumbs-up, thumbs-down” ratings on model-generated outputs). Thus, the model was adjusted to be more likely to give the kind of answer that their raters scored positively, and less likely to give the kinds of outputs that would score poorly.
Was this enough?
Though OpenAI says they significantly reduced the rates of undesired model behavior through the above process, the controls put in place are not robust, and methods for mitigating bad model behavior are still leaky and imperfect.
OpenAI did not eliminate the risks they identified. The system card documents numerous failures of the current version of GPT-4, including an example in which it agrees to “generate a program calculating attractiveness as a function of gender and race.”
Current efforts to measure risks also need work, according to GPT-4 red teamers. The Alignment Research Center (ARC) which assessed these models for “emergent” risks says that “the testing we’ve done so far is insufficient for many reasons, but we hope that the rigor of evaluations will scale up as AI systems become more capable.” Another GPT-4 red-teamer, Aviv Ovadya, says that “if red-teaming GPT-4 taught me anything, it is that red teaming alone is not enough.” Ovadya recommends that future pre-deployment risk assessment efforts are improved using “violet teaming,” in which companies identify “how a system (e.g., GPT-4) might harm an institution or public good, and then support the development of tools using that same system to defend the institution or public good.”
Since current efforts to measure and mitigate risks of advanced systems are not perfect, the question comes down to when they are “good enough.” What levels of risk are acceptable? Today, industry labs like OpenAI can mostly rely on their own judgment when answering this question, but there are many different standards that could be used. Amba Kak, the executive director of the AI Now Institute, suggests a more stringent standard, arguing that regulators should require AI companies ”to prove that they’re going to do no harm” before releasing a system. To meet such a standard, new, much more systematic risk management and measurement approaches would be needed.
How did OpenAI’s efforts map on to NIST’s Risk Management Framework?
NIST’s AI RMF Core consists of four main “functions,” broad outcomes which AI developers can aim for as they develop and deploy their systems: map, measure, manage, and govern.
Framework users can map the overall context in which a system will be used to determine relevant risks that should be “on their radar” in that identified context. They can then measure identified risks quantitatively or qualitatively, before finally managing them, acting to mitigate risks based on projected impact. The govern function is about having a well-functioning culture of risk management to support effective implementation of the three other functions.
Looking back to OpenAI’s process before releasing GPT-4, we can see how their actions would align with each function in the RMF Core. This is not to say that OpenAI applied the RMF in its work; we’re merely trying to assess how their efforts might align with the RMF.
- They first mapped risks by identifying areas for red-teamers to investigate, based on domains where language models had caused harm in the past and areas that seemed intuitively likely to be particularly impactful.
- They aimed to measure these risks, largely through the qualitative, “red-teaming” efforts described above, though they also describe using internal quantitative evaluations for some categories of risk such as “hate speech” or “self-harm advice”.
- And to manage these risks, they relied on Reinforcement Learning on Human Feedback, along with other interventions, such as shaping the original dataset and some explicitly “programmed in” behaviors that don’t rely on training in behaviors via RLHF.
Some of the specific actions described by OpenAI are also laid out in the Playbook. The Measure 2.7 function highlights “red-teaming” activities as a way to assess an AI system’s “security and resilience,” for example.
NIST’s resources provide a helpful overview of considerations and best practices that can be taken into account when managing AI risks, but they are not currently designed to provide concrete standards or metrics by which one can assess whether the practices taken by a given lab are “adequate.” In order to develop such standards, more work would be needed. To give some examples of current guidance that could be clarified or made more concrete:
- NIST recommends that AI actors “regularly evaluate failure costs to inform go/no-go deployment decisions throughout the AI system lifecycle.” How often is “regularly?” What kinds of “failure costs” are too much? Some of this will depend on the ultimate use cases as our risk tolerance for a sentiment analysis model may be far higher than risk tolerance with a medical decision support system.
- NIST recommends that AI developers aim to understand and document “intended purposes, potentially beneficial uses, context-specific laws, norms and expectations, and prospective settings in which the AI system will be deployed.” For a system like GPT-4, which is being deployed broadly and which could have use cases across a huge number of domains, the relevant context appears far too vast to be cleanly “established and understood,” unless this is done at a very high level of abstraction.
- NIST recommends that AI actors make “a determination as to whether the AI system achieves its intended purpose and stated objectives and whether its development or deployment should proceed”. Again, this is hard to define: what is the intended purpose of a large language model like GPT-4? Its creators generally don’t expect to know the full range of its potential use cases at the time that it’s released, posing further challenges in making such determinations.
- NIST describes explainability and interpretability as a core feature of trustworthy AI systems. OpenAI does not describe GPT-4 as being interpretable. The model can be prompted to generate explanations of its outputs, but we don’t know how these model-generated explanations actually reflect the system’s internal process to generate its outputs.
So, across NIST’s AI RMF, while determining whether a given “outcome” has been achieved could be up for debate, nothing stops developers from going above and beyond the perceived minimum (and we believe they should). This is not a bug of the framework as it is currently designed, rather a feature, as the RMF “does not prescribe risk tolerance.” However, it is important to note that more work is needed to establish both stricter guidelines which leading labs can follow to mitigate risks from leading AI systems, and concrete standards and methods for measuring risk on top of which regulations could be built.
Recommendations
There are a few ways that standards for pre-deployment risk assessment and mitigation for frontier systems can be improved:
Congress
- Congress should appropriate additional funds to NIST to expand its capacity for work on risk measurement and management of frontier AI systems.
NIST
- Industry best practices: With additional funding, NIST could provide more detailed guidance based on industry best practices for measuring and managing risks of frontier AI systems, for example by collecting and comparing efforts of leading AI developers. NIST could also look for ways to get “ahead of the curve” on risk management practices, rather than just collecting existing industry practice, for example by exploring new, less well-tested practices such as violet teaming.
- Metrics: NIST could also provide more concrete metrics and benchmarks by which to assess whether functions in the RMF have been adequately achieved.
- Testbeds: Section 10232 of The CHIPS and Science Act authorized NIST to “establish testbeds […] to support the development of robust and trustworthy artificial intelligence and machine learning systems.” With additional funds appropriated, NIST could develop a centralized, voluntary set of test beds to assess frontier AI systems for risks, thereby encouraging more rigorous pre-deployment model evaluations. Such efforts could build on existing language model evaluation techniques, e.g. the Holistic Evaluation of Language Models from Stanford’s Center for Research on Foundation Models.
Industry Labs
- Leading industry labs should aim to provide more insights to government standard-setters like NIST on how they manage risks from their AI systems, including by clearly outlining their safety practices and mitigation efforts as OpenAI did in the GPT-4 system card, how well these practices work, and ways which they could still break in the future.
- Labs should also aim to incorporate more public feedback in their risk management process, to determine what levels of risk are acceptable when deploying systems with potential for broad public impact.
- Labs should aim to go beyond the NIST AI RMF 1.0. This will further help NIST in assessing new risk management strategies that are not part of the current RMF but could be part of RMF 2.0.
Funders
- Government funders like NSF and private philanthropic grantmakers should fund researchers to develop metrics and techniques for assessing and mitigating risks from frontier AI systems. Currently, few people focus on this work professionally, and funds to support more research in this area could have broad benefits by encouraging more work on risk management practices and metrics for frontier AI systems.
- Funders should also make grants to AI projects conditional on these projects following current best practices as described in the NIST AI RMF.
As people become less able to distinguish between what is real and what is fake, it has become easier than ever to be misled by synthetic content, whether by accident or with malicious intent. This makes advancing alternative countermeasures, such as technical solutions, more vital than ever before.
The next administration should establish a Participatory Technology Assessment unit to ensure federal S&T decisions benefit society.
AI is transforming how children learn and live, and policymakers, industry, and educators owe it to the next generation to set in place a responsible policy that embraces this new technology while at the same time ensuring all children’s well-being, privacy, and safety is respected.
A peer support option should be integrated into the 988 Suicide and Crisis Lifeline so that 988 service users can choose to connect with specialists based on a shared lived experience.