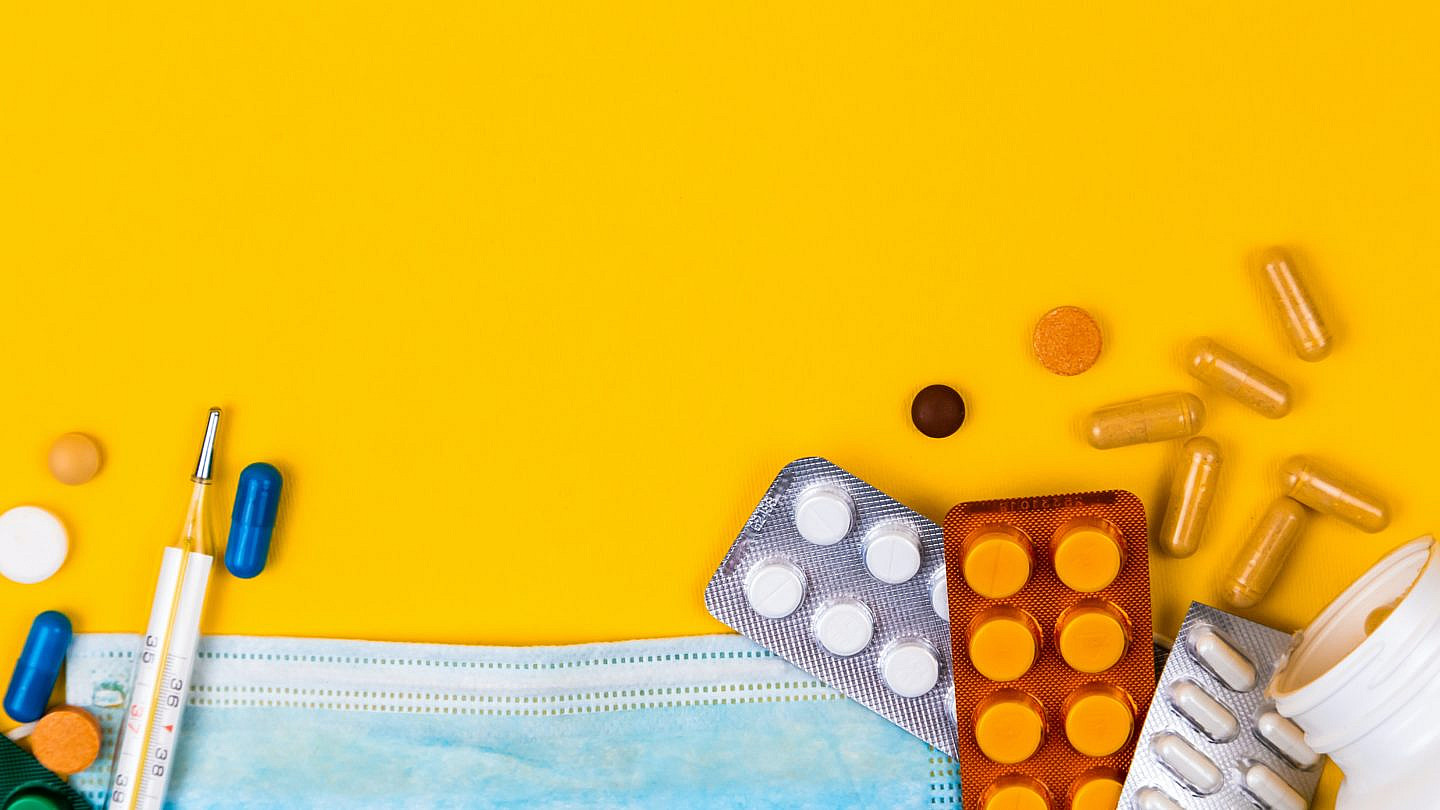
Promoting Fairness in Medical Innovation
There is a crisis within healthcare technology research and development, wherein certain groups due to their age, gender, or race and ethnicity are under-researched in preclinical studies, under-represented in clinical trials, misunderstood by clinical practitioners, and harmed by biased medical technology. These issues in turn contribute to costly disparities in healthcare outcomes, leading to losses of $93 billion a year in excess medical-care costs, $42 billion a year in lost productivity, and $175 billion a year due to premature deaths. With the rise of artificial intelligence (AI) in healthcare, there’s a risk of encoding and recreating existing biases at scale.
The next Administration and Congress must act to address bias in medical technology at the development, testing and regulation, and market-deployment and evaluation phases. This will require coordinated effort across multiple agencies. In the development phase, science funding agencies should enforce mandatory subgroup analysis for diverse populations, expand funding for under-resourced research areas, and deploy targeted market-shaping mechanisms to incentivize fair technology. In the testing and regulation phase, the FDA should raise the threshold for evaluation of medical technologies and algorithms and expand data-auditing processes. In the market-deployment and evaluation phases, infrastructure should be developed to perform impact assessments of deployed technologies and government procurement should incentivize technologies that improve health outcomes.
Challenge and Opportunity
Bias is regrettably endemic in medical innovation. Drugs are incorrectly dosed to people assigned female at birth due to historical exclusion of women from clinical trials. Medical algorithms make healthcare decisions based on biased health data, clinically disputed race-based corrections, and/or model choices that exacerbate healthcare disparities. Much medical equipment is not accessible, thus violating the Americans with Disabilities Act. And drugs, devices, and algorithms are not designed with the lifespan in mind, impacting both children and the elderly. Biased studies, technology, and equipment inevitably produce disparate outcomes in U.S. healthcare.
The problem of bias in medical innovation manifests in multiple ways: cutting across technological sectors in clinical trials, pervading the commercialization pipeline, and impeding equitable access to critical healthcare advances.
Bias in medical innovation starts with clinical research and trials
The 1993 National Institutes of Health (NIH) Revitalization Act required federally funded clinical studies to (i) include women and racial minorities as participants, and (ii) break down results by sex and race or ethnicity. As of 2019, the NIH also requires inclusion of participants across the lifespan, including children and older adults. Yet a 2019 study found that only 13.4% of NIH-funded trials performed the mandatory subgroup analysis, and challenges in meeting diversity targets continue into 2024 . Moreover, the increasing share of industry-funded studies are not subject to Revitalization Act mandates for subgroup analysis. These studies frequently fail to report differences in outcomes by patient population as a result. New requirements for Diversity Action Plans (DAPs), mandated under the 2023 Food and Drug Omnibus Reform Act, will ensure drug and device sponsors think about enrollment of diverse populations in clinical trials. Yet, the FDA can still approve drugs and devices that are not in compliance with their proposed DAPs, raising questions around weak enforcement.
The resulting disparities in clinical-trial representation are stark: African Americans represent 12% of the U.S. population but only 5% of clinical-trial participants, Hispanics make up 16% of the population but only 1% of clinical trial participants, and sex distribution in some trials is 67% male. Finally, many medical technologies approved prior to 1993 have not been reassessed for potential bias. One outcome of such inequitable representation is evident in drug dosing protocols: sex-aware prescribing guidelines exist for only a third of all drugs.
Bias in medical innovation is further perpetuated by weak regulation
Algorithms
Regulation of medical algorithms varies based on end application, as defined in the 21st Century Cures Act. Only algorithms that (i) acquire and analyze medical data and (ii) could have adverse outcomes are subject to FDA regulation. Thus, clinical decision-support software (CDS) is not regulated even though these technologies make important clinical decisions in 90% of U.S. hospitals. The FDA has taken steps to try and clarify what CDS must be considered a medical device, although these actions have been heavily criticized by industry. Finally, the lack of regulatory frameworks for generative AI tools is leading to proliferation without oversight.
Even when a medical algorithm is regulated, regulation may occur through relatively permissive de novo pathways and 510(k) pathways. A de novo pathway is used for novel devices determined to be low to moderate risk, and thus subject to a lower burden of proof with respect to safety and equity. A 510(k) pathway can be used to approve a medical device exhibiting “substantial equivalence” to a previously approved device, i.e., it has the same intended use and/or same technological features. Different technical features can be approved so long as there are no questions raised around safety and effectiveness.
Medical algorithms approved through de novo pathways can be used as predicates for approval of devices through 510(k) pathways. Moreover, a device approved through a 510(k) pathway can remain on the market even if its predicate device was recalled. Widespread use of 510(k) approval pathways has generated a “collapsing building” phenomenon, wherein many technologies currently in use are based on failed predecessors. Indeed, 97% of devices recalled between 2008 to 2017 were approved via 510(k) clearance.
While DAP implementation will likely improve these numbers, for the 692 AI-ML enabled medical devices, only 3.6% reported race or ethnicity, 18.4% reported age, and only .9% include any socioeconomic information. Further, less than half did detailed analysis of algorithmic performance and only 9% included information on post-market studies, raising the risk of algorithmic bias following approvals and broad commercialization.
Even more alarming is evidence showing that machine learning can further entrench medical inequities. Because machine learning medical algorithms are powered by data from past medical decision-making, which is rife with human error, these algorithms can perpetuate racial, gender, and economic bias. Even algorithms demonstrated to be ‘unbiased’ at the time of approval can evolve in biased ways over time, with little to no oversight from the FDA. As technological innovation progresses, especially generative AI tools, an intentional focus on this problem will be required.
Medical devices
Currently, the Medical Device User Fee Act requires the FDA to consider the least burdensome appropriate means for manufacturers to demonstrate the effectiveness of a medical device or to demonstrate a device’s substantial equivalence. This requirement was reinforced by the 21st Century Cures Act, which also designated a category for “breakthrough devices” subject to far less-stringent data requirements. Such legislation shifts the burden of clinical data collection to physicians and researchers, who might discover bias years after FDA approval. This legislation also makes it difficult to require assessments on the differential impacts of technology.
Like medical algorithms, many medical devices are approved through 510(k) exemptions or de novo pathways. The FDA has taken steps since 2018 to increase requirements for 510(k) approval and ensure that Class III (high-risk) medical devices are subject to rigorous pre-market approval, but problems posed by equivalence and limited diversity requirements remain.
Finally, while DAPs will be required for many devices seeking FDA approval, the recommended number of patients in device testing is shockingly low. For example, currently, only 10 people are required in a study of any new pulse oximeter’s efficacy and only 2 of those people need to be “darkly pigmented”. This requirement (i) does not have the statistical power necessary to detect differences between demographic groups, and (i) does not represent the composition of the U.S. population. The standard is currently under revision after immense external pressure. FDA-wide, there are no recommended guidelines for addressing human differences in device design, such as pigmentation, body size, age, and pre-existing conditions.
Pharmaceuticals
The 1993 Revitalization Act strictly governs clinical trials for pharmaceuticals and does not make recommendations for adequate sex or genetic diversity in preclinical research. The results are that a disproportionately high number of male animals are used in research and that only 5% of cell lines used for pharmaceutical research are of African descent. Programs like All of Us, an effort to build diverse health databases through data collection, are promising steps towards improving equity and representation in pharmaceutical research and development (R&D). But stronger enforcement is needed to ensure that preclinical data (which informs function in clinical trials) reflects the diversity of our nation.
Bias in medical innovation are not tracked post-regulatory approval
FDA-regulated medical technologies appear trustworthy to clinicians, where the approval signals safety and effectiveness. So, when errors or biases occur (if they are even noticed), the practitioner may blame the patient for their lifestyle rather than the technology used for assessment. This in turn leads to worse clinical outcomes as a result of the care received.
Bias in pulse oximetry is the perfect case study of a well-trusted technology leading to significant patient harm. During the COVID-19 pandemic, many clinicians and patients were using oximeter technology for the first time and were not trained to spot factors, like melanin in the skin, that cause inaccurate measurements and impact patient care. Issues were largely not attributed to the device. This then leads to underreporting of adverse events to the FDA — which is already a problem due to the voluntary nature of adverse-event reporting.
Even when problems are ultimately identified, the federal government is slow to respond. The pulse oximeter’s limitations in monitoring oxygenation levels across diverse skin tones was identified as early as the 1990s. 34 years later, despite repeated follow-up studies indicating biases, no manufacturer has incorporated skin-tone-adjusted calibration algorithms into pulse oximeters. It required the large Sjoding study, and the media coverage it garnered around delayed care and unnecessary deaths, for the FDA to issue a safety communication and begin reviewing the regulation.
Other areas of HHS are stepping up to address issues of bias in deployed technologies. A new ruling by the HHS Office of Civil Rights (OCR) on Section 1557 of the Affordable Care Act requires covered providers and institutions (i.e. any receiving federal funding) to identify their use of patient care decision support tools that directly measure race, color, national origin, sex, age, or disability, and to make reasonable efforts to mitigate the risk of discrimination from their use of these tools. Implementation of this rule will depend on OCR’s enforcement, and yet it provides another route to address bias in algorithmic tools.
Differential access to medical innovation is a form of bias
Americans face wildly different levels of access to new medical innovations. As many new innovations have high cost points, these drugs, devices, and algorithms exist outside the price range of many patients, smaller healthcare institutions and federally funded healthcare service providers, including the Veterans Health Administration, federally qualified health centers and the Indian Health Service. Emerging care-delivery strategies might not be covered by Medicare and Medicaid, meaning that patients insured by CMS cannot access the most cutting-edge treatments. Finally, the shift to digital health, spurred by COVID-19, has compromised access to healthcare in rural communities without reliable broadband access.
Finally, the Advanced Research Projects Agency for Health (ARPA-H) has a commitment to have all programs and projects consider equity in their design. To fulfill ARPA-H’s commitment, there is a need for action to ensure that medical technologies are developed fairly, tested with rigor, deployed safely, and made affordable and accessible to everyone.
Plan of Action
The next Administration should launch “Healthcare Innovation for All Americans” (HIAA), a whole of government initiative to improve health outcomes by ensuring Americans have access to bias-free medical technologies. Through a comprehensive approach that addresses bias in all medical technology sectors, at all stages of the commercialization pipeline, and in all geographies, the initiative will strive to ensure the medical-innovation ecosystem works for all. HIAA should be a joint mandate of Health and Human Services (HHS) and the Office of Science Technology and Policy (OSTP) to work with federal agencies on priorities of equity, non-discrimination per Section 1557 of the Affordable Care Act and increasing access to medical innovation, and initiative leadership should sit at both HHS and OSTP.
This initiative will require involvement of multiple federal agencies, as summarized in the table below. Additional detail is provided in the subsequent sections describing how the federal government can mitigate bias in the development phase; testing, regulation, and approval phases; and market deployment and evaluation phases.
Three guiding principles should underlie the initiative:
- Equity and non-discrimination should drive action. Actions should seek to improve the health of those who have been historically excluded from medical research and development. We should design standards that repair past exclusion and prevent future exclusion.
- Coordination and cooperation are necessary. The executive and legislative branches must collaborate to address the full scope of the problem of bias in medical technology, from federal processes to new regulations. Legislative leadership should task the Government Accountability Office (GAO) to engage in ongoing assessment of progress towards the goal of achieving bias-free and fair medical innovation.
- Transparent, evidence-based decision making is paramount. There is abundant peer-reviewed literature that examines bias in drugs, devices, and algorithms used in healthcare settings — this literature should form the basis of a non-discrimination approach to medical innovation. Gaps in evidence should be focused on through deployed research funding. Moreover, as algorithms become ubiquitous in medicine, every effort should be made to ensure that these algorithms are trained on representative data of those experiencing a given healthcare condition.
Addressing bias at the development phase
The following actions should be taken to address bias in medical technology at the innovation phase:
- Enforce parity in government-funded research. For clinical research, NIH should examine the widespread lack of adherence to regulations requiring that government funded clinical trials report sex, racial or ethnicity, and age breakdown of trial participants. Funding should be reevaluated for non-compliant trials. For preclinical research, NIH should require gender parity in animal models and representation of diverse cell lines used in federally funded studies.
- Deploy funding to address research gaps. Where data sources for historically marginalized people are lacking, such as for women’s cardiovascular health, NIH should deploy strategic, targeted funding programs to fill these knowledge gaps. This could build on efforts like the Initiative on Women’s Health Research. Increased funding should include resources for underrepresented groups to participate in research and clinical trials through building capacity in community organizations. Results should be added to a publicly available database so they can be accessed by designers of new technologies. Funding programs should also be created to fill gaps in technology, such as in diagnostics and treatments for high-prevalence and high-burden uterine diseases like endometriosis (found in 10% of reproductive-aged people with uteruses).
- Invest in research into healthcare algorithms and databases. Given the explosion of algorithms in healthcare decision-making, NIH and NSF should launch a new research program focused on the study, evaluation, and application of algorithms in healthcare delivery, and on how artificial intelligence and machine learning (AI/ML) can exacerbate healthcare inequities. The initial request for proposals should focus on design strategies for medical algorithms that mitigate bias from data or model choices.
- Task ARPA-H with developing metrics for equitable medical technology development. ARPA-H should prioritize developing a set of procedures and metrics for equitable development of medical technology. Once developed, these processes should be rapidly deployed across ARPA-H, as well as published for potential adoption by additional federal agencies, industry, and other stakeholders. ARPA-H could also collaborate with NIST on standards setting with NIST and ASTP on relevant standards setting. For instance, NIST has developed an AI Risk Management Framework and the ONC engages in setting standards that achieve equity by design. CMS could use resultant standards for Medicare and Medicaid reimbursements.
- Leverage procurement as a demand-signal for medical technologies that work for diverse populations. As the nation’s largest healthcare system, the Veterans Health Administration (VHA) can generate demand-signals for bias-free medical technologies through its procurement processes and market-shaping mechanisms. For example, the VA could put out a call for a pulse oximeter that works equally well across the entire range of human skin pigmentation and offer contracts for the winning technology.
Addressing bias at the testing, regulation, and approval phases
The following actions should be taken to address bias in medical innovation at the testing, regulation, and approval phases:
- Raise the threshold for FDA evaluation of devices and algorithms. Equivalency necessary to receive 510(k) clearance should be narrowed. For algorithms, this would involve consideration of whether the datasets for machine learning tactics used by the new device and its predicate are similar. For devices (including those that use algorithms), this would require tightening the definition of “same intended use” (currently defined as a technology having the same functionality as one previously approved by the FDA) as well as eliminating the approval of new devices with “different technological characteristics” (the application of one technology to a new area of treatment in which that technology is untested).
- Evaluate FDA’s guidance on specific technology groups for equity. Requirements for the safety of a given drug, medical device, or algorithm should have the statistical power necessary to detect differences between demographic groups and represent all end-users of the technology..
- Establish a data bank for auditing medical algorithms. The newly established Office of Digital Transformation within the FDA should create a “data bank” of healthcare images and datasets representative of the U.S. population, which could be done in partnership with the All of Us program. Medical technology developers could use the data bank to assess the performance of medical algorithms across patient populations. Regulators could use the data bank to ground claims made by those submitting a technology for FDA approval.
- Allow data submitted to the FDA to be examined by the broader scientific community. Currently, data submitted to the FDA as part of its regulatory-approval process is kept as a trade secret and not released pre-authorization to researchers. Releasing the data via an FDA-invited “peer review” step in the regulation of high-risk technologies, like automated decision-making algorithms, Class III medical devices, and drugs, will ensure that additional, external rigor is applied to the technologies that could cause the most harm due to potential biases.
- Establish an enforceable AI Bill of Rights. The federal government and Congress should create protections for necessary uses of artificial intelligence (AI) identified by OSTP. Federally funded healthcare centers, like facilities part of the Veterans Health Administration, could refuse to buy software or technology products that violate this “AI Bill of Rights” through changes to federal acquisition regulation (FAR).
Addressing bias at the market deployment and evaluation phases
- Strengthen reporting mechanisms at the FDA. Healthcare providers, who are often closest to the deployment of medical technologies, should be made mandatory reporters to the FDA of all witnessed adverse events related to bias in medical technology. In addition, the FDA should require the inclusion of unique device identifiers (UDIs) in adverse-response reporting. Using this data, Congress should create a national and publicly accessible registry that uses UDIs to track post-market medical outcomes and safety.
- Require impact assessments of deployed technologies. Congress must establish systems of accountability for medical technologies, like algorithms, that can evolve over time. Such work could be done by passing the Algorithmic Accountability Act which would require companies that create “high-risk automated decision systems” to conduct impact assessments reviewed by the FTC as frequently as necessary.
- Assess disparities in patient outcomes to direct technical auditing. AHRQ should be given the funding needed to fully investigate patient-outcome disparities that could be caused by biases in medical technology, such as its investigation into the impacts of healthcare algorithms on racial and ethnic disparities. The results of this research should be used to identify technologies that the FDA should audit post-market for efficacy or the FTC should investigate. CMS and its accrediting agencies can monitor these technologies and assess whether they should receive Medicare and Medicaid funding.
- Review reimbursement guidelines that are dependent on medical technologies with known bias. CMS should review its national coverage determinations for technologies, like pulse oximetry, that are known to perform differently across populations. For example, pulse oximeters can be used to determine home oxygen therapy provision, thus potentially excluding darkly-pigmented populations from receiving this benefit.
- Train physicians to identify bias in medical technologies and identify new areas of specialization. ED should work with medical schools to develop curricula training physicians to identify potential sources of bias in medical technologies and ensuring that physicians understand how to report adverse events to the FDA. In addition, ED should consider working with the American Medical Association to create new medical specialties that work at the intersection of technology and care delivery.
- Ensure that technologies developed by ARPA-H have an enforceable access plan. ARPA-H will produce cutting-edge technologies that must be made accessible to all Americans. ARPA-H should collaborate with the Center for Medicare and Medicaid Innovation to develop strategies for equitable delivery of these new technologies. A cost-effective deployment strategy must be identified to service federally-funded healthcare institutions like Veterans Health Administration hospitals and clinical, federally qualified health centers, and Indian Health Service.
- Create a fund to support digital health technology infrastructure in rural hospitals. To capitalize on the $65 billion expansion of broadband access allocated in the Bipartisan Infrastructure Bill, HRSA should deploy strategic funding to federally qualified health centers and rural health clinics to support digital health strategies — such as telehealth and mobile health monitoring — and patient education for technology adoption.
A comprehensive road map is needed
The GAO should conduct a comprehensive investigation of “black box” medical technologies utilizing algorithms that are not transparent to end users, medical providers, and patients. The investigation should inform a national strategic plan for equity and non-discrimination in medical innovation that relies heavily on algorithmic decision-making. The plan should include identification of noteworthy medical technologies leading to differential healthcare outcomes, creation of enforceable regulatory standards, development of new sources of research funding to address knowledge gaps, development of enforcement mechanisms for bias reporting, and ongoing assessment of equity goals.
Timeline for action
Realizing HIAA will require mobilization of federal funding, introduction of regulation and legislation, and coordination of stakeholders from federal agencies, industry, healthcare providers, and researchers around a common goal of mitigating bias in medical technology. Such an initiative will be a multi-year undertaking and require funding to enact R&D expenditures, expand data capacity, assess enforcement impacts, create educational materials, and deploy personnel to staff all the above.
Near-term steps that can be taken to launch HIAA include issuing a public request for information, gathering stakeholders, engaging the public and relevant communities in conversation, and preparing a report outlining the roadmap to accomplishing the policies outlined in this memo.
Conclusion
Medical innovation is central to the delivery of high-quality healthcare in the United States. Ensuring equitable healthcare for all Americans requires ensuring that medical innovation is equitable across all sectors, phases, and geographies. Through a bold and comprehensive initiative, the next Administration can ensure that our nation continues leading the world in medical innovation while crafting a future where healthcare delivery works for all.
This action-ready policy memo is part of Day One 2025 — our effort to bring forward bold policy ideas, grounded in science and evidence, that can tackle the country’s biggest challenges and bring us closer to the prosperous, equitable and safe future that we all hope for whoever takes office in 2025 and beyond.
PLEASE NOTE (February 2025): Since publication several government websites have been taken offline. We apologize for any broken links to once accessible public data.
HIAA will be successful when medical policies, projects, and technologies yield equitable health care access, treatment, and outcomes. For instance, success would yield the following outcomes:
- Representation in preclinical and clinical research equivalent to the incidence of a studied condition in the general population.
- Research on a disease condition funded equally per affected patient.
- Existence of data for all populations facing a given disease condition.
- Medical algorithms that have equal efficacy across subgroup populations.
- Technologies that work equally well in testing as they do when deployed to the market.
- Healthcare technologies made available and affordable to all care facilities.
Regulation alone cannot close the disparity gap. There are notable gaps in preclinical and clinical research data for women, people of color, and other historically underrepresented groups that need to be filled. There are also historical biases encoded in AI/ML decision making algorithms that need to be studied and rectified. In addition, the FDA’s role is to serve as a safety check on new technologies — the agency has limited oversight over technologies once they are out on the market due to the voluntary nature of adverse reporting mechanisms. This means that agencies like the FTC and CMS need to be mobilized to audit high-risk technologies once they reach the market. Eliminating bias in medical technology is only possible through coordination and cooperation of federal agencies with each other as well as with partners in the medical device industry, the pharmaceutical industry, academic research, and medical care delivery.
A significant focus of the medical device and pharmaceutical industries is reducing the time to market for new medical devices and drugs. Imposing additional requirements for subgroup analysis and equitable use as part of the approval process could work against this objective. On the other hand, ensuring equitable use during the development and approval stages of commercialization will ultimately be less costly than dealing with a future recall or a loss of Medicare or Medicaid eligibility if discriminatory outcomes are discovered.
Healthcare disparities exist in every state in America and are costing billions a year in economic growth. Some of the most vulnerable people live in rural areas, where they are less likely to receive high-quality care because costs of new medical technologies are too high for the federally qualified health centers that serve one in five rural residents as well as rural hospitals. Furthermore, during continued use, a biased device creates adverse healthcare outcomes that cost taxpayers money. A technology functioning poorly due to bias can be expensive to replace. It is economically imperative to ensure technology works as expected, as it leads to more effective healthcare and thus healthier people.
The transition to a clean energy future and diversified sources of energy requires a fundamental shift in how we produce and consume energy across all sectors of the U.S. economy.
Advancing the U.S. leadership in emerging biotechnology is a strategic imperative, one that will shape regional development within the U.S., economic competitiveness abroad, and our national security for decades to come.
Inconsistent metrics and opaque reporting make future AI power‑demand estimates extremely uncertain, leaving grid planners in the dark and climate targets on the line
As AI becomes more capable and integrated throughout the United States economy, its growing demand for energy, water, land, and raw materials is driving significant economic and environmental costs, from increased air pollution to higher costs for ratepayers.