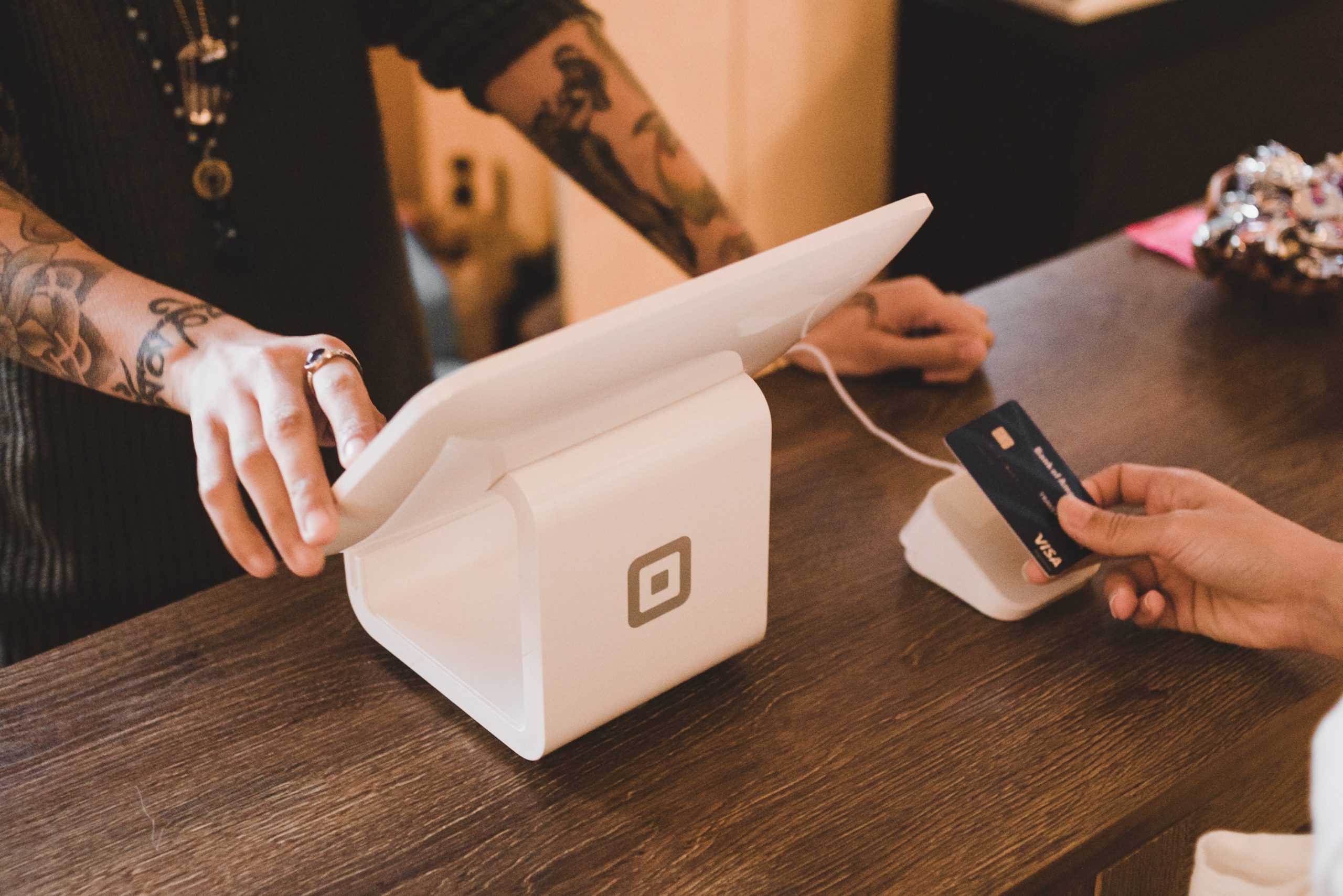
Strengthening the Integrity of Government Payments Using Artificial Intelligence
Summary
Tens of billions of taxpayer dollars are lost every year due to improper payments to the federal government. These improper payments arise from agency and claimant errors as well as outright fraud. Data analytics can help identify errors and fraud, but often only identify improper payments after they have already been issued.
Artificial intelligence (AI) in general—and machine learning (ML) in particular (AI/ML)—could substantially improve the accuracy of federal payment systems. The next administration should launch an initiative to integrate AI/ML into federal agencies’ payment processes. As part of this initiative, the federal government should work extensively with non-federal entities—including commercial firms, nonprofits, and academic institutions—to address major enablers and barriers pertaining to applications of AI/ML in federal payment systems. These include the incidence of false positives and negatives, perceived and actual fairness and bias issues, privacy and security concerns, and the use of ML for predicting the likelihood of future errors and fraud.
To sustain America’s leadership in AI innovation, accelerate adoption across the economy, and guarantee that AI systems remain secure and trustworthy, we offer a set of policy recommendations.
Current scientific understanding shows that so-called “anonymization” methods that have been widely used in the past are inadequate for protecting privacy in the era of big data and artificial intelligence.
To fully harness the benefits of AI, the public must have confidence that these systems are deployed responsibly and enhance their lives and livelihoods.
The first Trump Administration’s E.O. 13859 commitment laid the foundation for increasing government accountability in AI use; this should continue