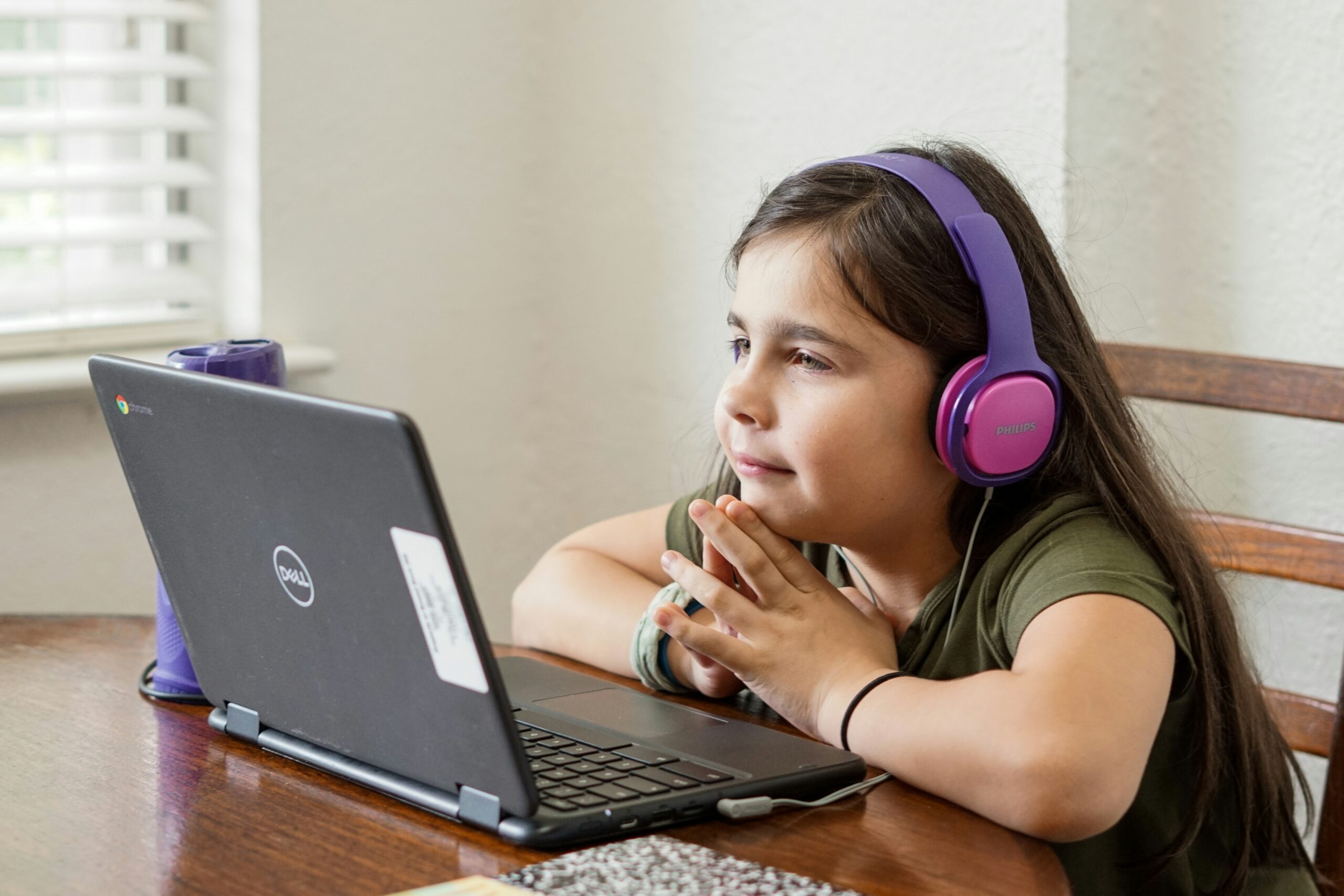
Modernizing AI Fairness Analysis in Education Contexts
The 2022 release of ChatGPT and subsequent foundation models sparked a generative AI (GenAI) explosion in American society, driving rapid adoption of AI-powered tools in schools, colleges, and universities nationwide. Education technology was one of the first applications used to develop and test ChatGPT in a real-world context. A recent national survey indicated that nearly 50% of teachers, students, and parents use GenAI Chatbots in school, and over 66% of parents and teachers believe that GenAI Chatbots can help students learn more and faster. While this innovation is exciting and holds tremendous promise to personalize education, educators, families, and researchers are concerned that AI-powered solutions may not be equally useful, accurate, and effective for all students, in particular students from minoritized populations. It is possible that as this technology further develops that bias will be addressed; however, to ensure that students are not harmed as these tools become more widespread it is critical for the Department of Education to provide guidance for education decision-makers to evaluate AI solutions during procurement, to support EdTech developers to detect and mitigate bias in their applications, and to develop new fairness methods to ensure that these solutions serve the students with the most to gain from our educational systems. Creating this guidance will require leadership from the Department of Education to declare this issue as a priority and to resource an independent organization with the expertise needed to deliver these services.
Challenge and Opportunity
Known Bias and Potential Harm
There are many examples of the use of AI-based systems introducing more bias into an already-biased system. One example with widely varying results for different student groups is the use of GenAI tools to detect AI-generated text as a form of plagiarism. Liang et. al found that several GPT-based plagiarism checkers frequently identified the writing of students for whom English is not their first language as AI-generated, even though their work was written before ChatGPT was available. The same errors did not occur with text generated by native English speakers. However, in a publication by Jiang (2024), no bias against non-native English speakers was encountered in the detection of plagiarism between human-authored essays and ChatGPT-generated essays written in response to analytical writing prompts from the GRE, which is an example of how thoughtful AI tool design and representative sampling in the training set can achieve fairer outcomes and mitigate bias.
Beyond bias, researchers have raised additional concerns about the overall efficacy of these tools for all students; however, more understanding around different results for subpopulations and potential instances of bias(es) is a critical aspect of deciding whether or not these tools should be used by teachers in classrooms. For AI-based tools to be usable in high-stakes educational contexts such as testing, detecting and mitigating bias is critical, particularly when the consequences of being incorrect are so high, such as for students from minoritized populations who may not have the resources to recover from an error (e.g., failing a course, being prevented from graduating school).
Another example of algorithmic bias before the widespread emergence of GenAI which illustrates potential harms is found in the Wisconsin Dropout Early Warning System. This AI-based tool was designed to flag students who may be at risk of dropping out of school; however, an analysis of the outcomes of these predictions found that the system disproportionately flagged African American and Hispanic students as being likely to drop out of school when most of these students were not at risk of dropping out). When teachers learn that one of their students is at risk, this may change how they approach that student, which can cause further negative treatment and consequences for that student, creating a self-fulfilling prophecy and not providing that student with the education opportunities and confidence that they deserve. These examples are only two of many consequences of using systems that have underlying bias and demonstrate the criticality of conducting fairness analysis before these systems are used with actual students.
Existing Guidance on Fair AI & Standards for Education Technology Applications
Guidance for Education Technology Applications
Given the harms that algorithmic bias can cause in educational settings, there is an opportunity to provide national guidelines and best practices that help educators avoid these harms. The Department of Education is already responsible for protecting student privacy and provides guidelines via the Every Student Succeeds Act (ESSA) Evidence Levels to evaluate the quality of EdTech solution evidence. The Office of Educational Technology, through support of a private non-profit organization (Digital Promise) has developed guidance documents for teachers and administrators, and another for education technology developers (U.S. Department of Education, 2023, 2024). In particular, “Designing for Education with Artificial Intelligence” includes guidance for EdTech developers including an entire section called “Advancing Equity and Protecting Civil Rights” that describes algorithmic bias and suggests that, “Developers should proactively and continuously test AI products or services in education to mitigate the risk of algorithmic discrimination.” (p 28). While this is a good overall guideline, the document critically is not sufficient to help developers conduct these tests.
Similarly, the National Institute of Standards and Technology has released a publication on identifying and managing bias in AI . While this publication highlights some areas of the development process and several fairness metrics, it does not provide specific guidelines to use these fairness metrics, nor is it exhaustive. Finally demonstrating the interest of industry partners, the EDSAFE AI Alliance, a philanthropically-funded alliance representing a diverse group of companies in educational technology, has also created guidance in the form of the 2024 SAFE (Safety, Accountability, Fairness, and Efficacy) Framework. Within the Fairness section of the framework, the authors highlight the importance of using fair training data, monitoring for bias, and ensuring accessibility of any AI-based tool. But again, this framework does not provide specific actions that education administrators, teachers, or EdTech developers can take to ensure these tools are fair and are not biased against specific populations. The risk to these populations and existing efforts demonstrate the need for further work to develop new approaches that can be used in the field.
Fairness in Education Measurement
As AI is becoming increasingly used in education, the field of educational measurement has begun creating a set of analytic approaches for finding examples of algorithmic bias, many of which are based on existing approaches to uncovering bias in educational testing. One common tool is called Differential Item Functioning (DIF), which checks that test questions are fair for all students regardless of their background. For example, it ensures that native English speakers and students learning English have an equal chance to succeed on a question if they have the same level of knowledge . When differences are found, this indicates that a student’s performance on that question is not based on their knowledge of the content.
While DIF checks have been used for several decades as a best practice in standardized testing, a comparable process in the use of AI for assessment purposes does not yet exist. There also is little historical precedent indicating that for-profit educational companies will self-govern and self-regulate without a larger set of guidelines and expectations from a governing body, such as the federal government.
We are at a critical juncture as school districts begin adopting AI tools with minimal guidance or guardrails, and all signs point to an increase of AI in education. The US Department of Education has an opportunity to take a proactive approach to ensuring AI fairness through strategic programs of support for school leadership, developers in educational technology, and experts in the field. It is important for the larger federal government to support all educational stakeholders under a common vision for AI fairness while the field is still at the relative beginning of being adopted for educational use.
Plan of Action
To address this situation, the Department of Education’s Office of the Chief Data Officer should lead development of a national resource that provides direct technical assistance to school leadership, supports software developers and vendors of AI tools in creating quality tech, and invests resources to create solutions that can be used by both school leaders and application developers. This office is already responsible for data management and asset policies, and provides resources on grants and artificial intelligence for the field. The implementation of these resources would likely be carried out via grants to external actors with sufficient technical expertise, given the rapid pace of innovation in the private and academic research sectors. Leading the effort from this office ensures that these advances are answering the most important questions and can integrate them into policy standards and requirements for education solutions. Congress should allocate additional funding to the Department of Education to support the development of a technical assistance program for school districts, establish new grants for fairness evaluation tools that span the full development lifecycle, and pursue an R&D agenda for AI fairness in education. While it is hard to provide an exact estimate, similar existing programs currently cost the Department of Education between $4 and $30 million a year.
Action 1. The Department of Education Should Provide Independent Support for School Leadership Through a Fair AI Technical Assistance Center (FAIR-AI-TAC)
School administrators are hearing about the promise and concerns of AI solutions in the popular press, from parents, and from students. They are also being bombarded by education technology providers with new applications of AI within existing tools and through new solutions.
These busy school leaders do not have time to learn the details of AI and bias analysis, nor do they have the technical background required to conduct deep technical evaluations of fairness within AI applications. Leaders are forced to either reject these innovations or implement them and expose their students to significant potential risk with the promise of improved learning. This is not an acceptable status quo.
To address these issues, the Department of Education should create an AI Technical Assistance Center (the Center) that is tasked with providing direct guidance to state and local education leaders who want to incorporate AI tools fairly and effectively. The Center should be staffed by a team of professionals with expertise in data science, data safety, ethics, education, and AI system evaluation. Additionally, the Center should operate independently of AI tool vendors to maintain objectivity.
There is precedent for this type of technical support. The U.S. Department of Education’s Privacy Technical Assistance Center (PTAC) provides guidance related to data privacy and security procedures and processes to meet FERPA guidelines; they operate a help desk via phone or email, develop training materials for broad use, and provide targeted training and technical assistance for leaders. A similar kind of center could be stood up to support leaders in education who need support evaluating proposed policy or procurement decisions.
This Center should provide a structured consulting service offering a variety of levels of expertise based on the individual stakeholder’s needs and the variety of levels of potential impact of the system/tool being evaluated on learners; this should include everything from basic levels of AI literacy to active support in choosing technological solutions for educational purposes. The Center should partner with external organizations to develop a certification system for high-quality AI educational tools that have passed a series of fairness checks. Creating a fairness certification (operationalized by third party evaluators) would make it much easier for school leaders to recognize and adopt fair AI solutions that meet student needs.
Action 2. The Department of Education Should Provide Expert Services, Data, and Grants for EdTech Developers
There are many educational technology developers with AI-powered innovations. Even when well-intentioned, some of these tools do not achieve their desired impacts or may be unintentionally unsafe due to a lack of processes and tests for fairness and safety.
Educational Technology developers generally operate under significant constraints when incorporating AI models into their tools and applications. Student data is often highly detailed and deeply personal, potentially containing financial, disability, and educational status information that is currently protected by FERPA, which makes it unavailable for use in AI model training or testing.
Developers need safe, legal, and quality datasets that they can use for testing for bias, as well as appropriate bias evaluation tools. There are several promising examples of these types of applications and new approaches to data security, such as the recently awarded NSF SafeInsights project, which allows analysis without disclosing the underlying data. In addition, philanthropically-funded organizations such as the Allen Institute for AI have released LLM evaluation tools that could be adapted and provided to Education Technology developers for testing. A vetted set of evaluation tools, along with more detailed technical resources and instructions for how to use them would encourage developers to incorporate bias evaluations early and often. Currently, there are very few market incentives or existing requirements that push developers to invest the necessary time or resources into this type of fairness analysis. Thus, the government has a key role to play here.
The Department of Education should also fund a new grant program that tasks grantees with developing a robust and independently validated third-party evaluation system that checks for fairness violations and biases throughout the model development process from pre-processing of data, to the actual AI use, to testing after AI results are created. This approach would support developers in ensuring that the tools they are publishing meet an agreed-upon minimum threshold for safe and fair use and could provide additional justification for the adoption of AI tools by school administrators.
Action 3. The Department of Education Should Develop Better Fairness R&D Tools with Researchers
There is still no consensus on best practices for how to ensure that AI tools are fair. As AI capabilities evolve, the field needs an ongoing vetted set of analyses and approaches that will ensure that any tools being used in an educational context are safe and fair for use with no unintended consequences.
The Department of Education should lead the creation of a a working group or task force comprised of subject matter experts from education, educational technology, educational measurement, and the larger AI field to identify the state of the art in existing fairness approaches for education technology and assessment applications, with a focus on modernized conceptions of identity. This proposed task force would be an inter-organizational group that would include representatives from several different federal government offices, such as the Office of Educational Technology and the Chief Data Office as well as prominent experts from industry and academia. An initial convening could be conducted alongside leading national conferences that already attract thousands of attendees conducting cutting-edge education research (such as the American Education Research Association and National Council for Measurement in Education).
The working group’s mandate should include creating a set of recommendations for federal funding to advance research on evaluating AI educational tools for fairness and efficacy. This research agenda would likely span multiple agencies including NIST, the Institute of Education Sciences of the U.S. Department of Education, and the National Science Foundation. There are existing models for funding early stage research and development with applied approaches, including the IES “Accelerate, Transform, Scale” programs that integrate learning sciences theory with efforts to scale theories through applied education technology program and Generative AI research centers that have the existing infrastructure and mandates to conduct this type of applied research.
Additionally, the working group should recommend the selection of a specialized group of researchers who would contribute ongoing research into new empirically-based approaches to AI fairness that would continue to be used by the larger field. This innovative work might look like developing new datasets that deliberately look for instances of bias and stereotypes, such as the CrowS-Pairs dataset. It may build on current cutting edge research into the specific contributions of variables and elements of LLM models that directly contribute to biased AI scores, such as the work being done by the AI company Anthropic. It may compare different foundation LLMs and demonstrate specific areas of bias within their output. It may also look like a collaborative effort between organizations, such as the development of the RSM-Tool, which looks for biased scoring. Finally, it may be an improved auditing tool for any portion of the model development pipeline. In general, the field does not yet have a set of universally agreed upon actionable tools and approaches that can be used across contexts and applications; this research team would help create these for the field.
Finally, the working group should recommend policies and standards that would incentivize vendors and developers working on AI education tools to adopt fairness evaluations and share their results.
Conclusion
As AI-based tools continue being used for educational purposes, there is an urgent need to develop new approaches to evaluating these solutions to fairness that include modern conceptions of student belonging and identity. This effort should be led by the Department of Education, through the Office of the Chief Data Officer, given the technical nature of the services and the relationship with sensitive data sources. While the Chief Data Officer should provide direction and leadership for the project, partnering with external organizations through federal grant processes would provide necessary capacity boosts to fulfill the mandate described in this memo.As we move into an age of widespread AI adoption, AI tools for education will be increasingly used in classrooms and in homes. Thus, it is imperative that robust fairness approaches are deployed before a new tool is used in order to protect our students, and also to protect the developers and administrators from potential litigation, loss of reputation, and other negative outcomes.
This action-ready policy memo is part of Day One 2025 — our effort to bring forward bold policy ideas, grounded in science and evidence, that can tackle the country’s biggest challenges and bring us closer to the prosperous, equitable and safe future that we all hope for whoever takes office in 2025 and beyond.
PLEASE NOTE (February 2025): Since publication several government websites have been taken offline. We apologize for any broken links to once accessible public data.
When AI is used to grade student work, fairness is evaluated by comparing the scores assigned by AI to those assigned by human graders across different demographic groups. This is often done using statistical metrics, such as the standardized mean difference (SMD), to detect any additional bias introduced by the AI. A common benchmark for SMD is 0.15, which suggests the presence of potential machine bias compared to human scores. However, there is a need for more guidance on how to address cases where SMD values exceed this threshold.
In addition to SMD, other metrics like exact agreement, exact + adjacent agreement, correlation, and Quadratic Weighted Kappa are often used to assess the consistency and alignment between human and AI-generated scores. While these methods provide valuable insights, further research is needed to ensure these metrics are robust, resistant to manipulation, and appropriately tailored to specific use cases, data types, and varying levels of importance.
Existing approaches to demographic post hoc analysis of fairness assume that there are two discrete populations that can be compared, for example students from African-American families vs. those not from African-American families, students from an English language learner family background vs. those that are not, and other known family characteristics. However in practice, people do not experience these discrete identities. Since at least the 1980s, contemporary sociological theories have emphasized that a person’s identity is contextual, hybrid, and fluid/changing. One current approach to identity that integrates concerns of equity that has been applied to AI is “intersectional identity” theory . This approach has begun to develop promising new methods that bring contemporary approaches to identity into evaluating fairness of AI using automated methods. Measuring all interactions between variables results in too small a sample; these interactions can be prioritized using theory or design principles or more advanced statistical techniques (e.g., dimensional data reduction techniques).
FAS is invested in seeing more students gain science and technology skills and enter STEM careers, both for students and for our country’s competitive advantage.
By investing in the mechanisms that connect learning ecosystems, policymakers can build “neighborhoods” of learning that prepare students for citizenship, work, and life.
Early-career and out-of-state teachers tend to be most heavily concentrated in Alaska’s rural schools, where they face a steep curve in adjusting to a new way of life while learning the ropes of teaching.
The next administration should establish a national, federally-funded initiative to develop a robust and diverse pipeline of STEM talent.