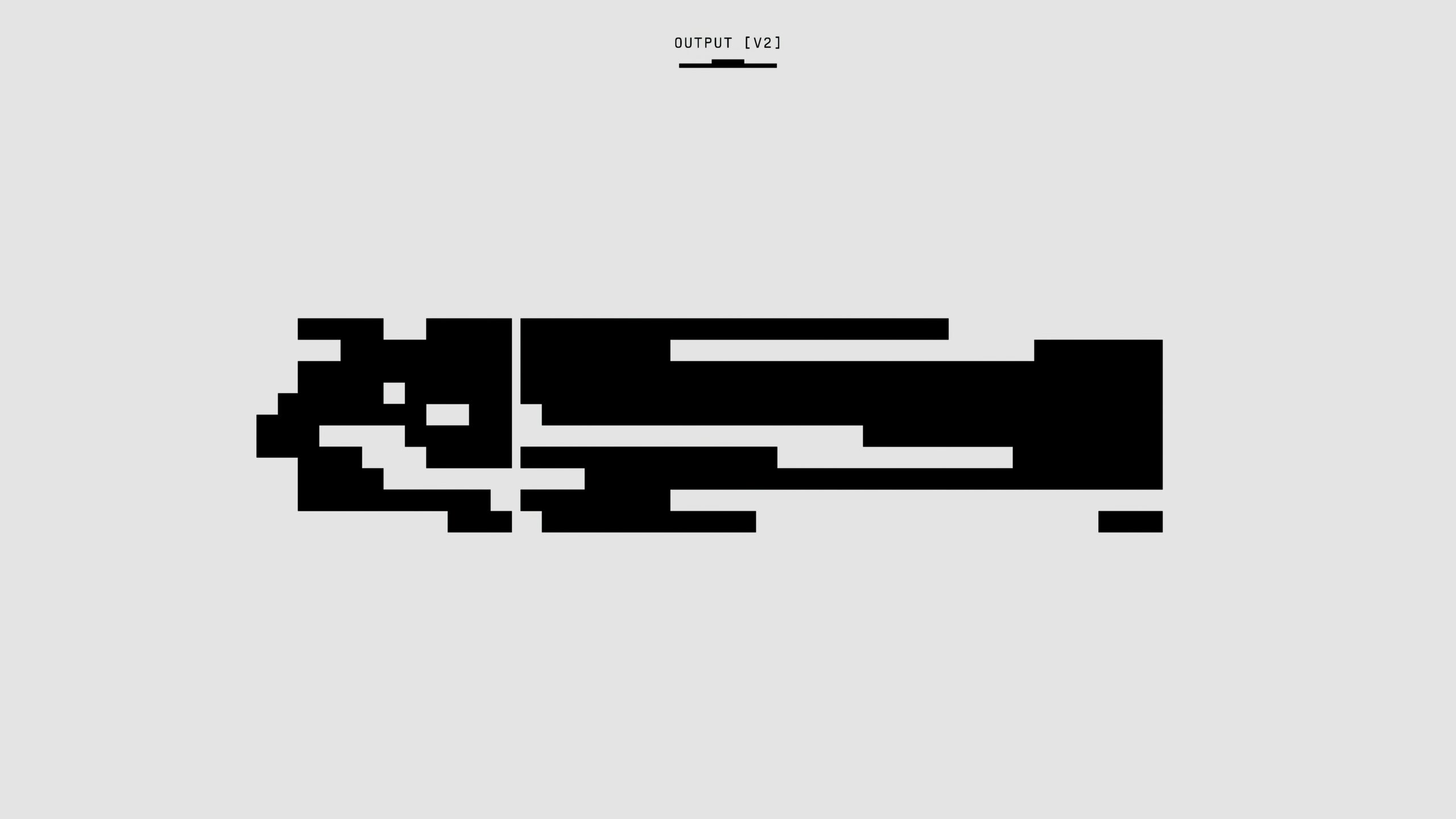
Improving Health Equity Through AI
Clinical decision support (CDS) artificial intelligence (AI) refers to systems and tools that utilize AI to assist healthcare professionals in making more informed clinical decisions. These systems can alert clinicians to potential drug interactions, suggest preventive measures, and recommend diagnostic tests based on patient data. Inequities in CDS AI pose a significant challenge to healthcare systems and individuals, potentially exacerbating health disparities and perpetuating an already inequitable healthcare system. However, efforts to establish equitable AI in healthcare are gaining momentum, with support from various governmental agencies and organizations. These efforts include substantial investments, regulatory initiatives, and proposed revisions to existing laws to ensure fairness, transparency, and inclusivity in AI development and deployment.
Policymakers have a critical opportunity to enact change through legislation, implementing standards in AI governance, auditing, and regulation. We need regulatory frameworks, investment in AI accessibility, incentives for data collection and collaboration, and regulations for auditing and governance of AI systems used in CDS systems/tools. By addressing these challenges and implementing proactive measures, policymakers can harness AI’s potential to enhance healthcare delivery and reduce disparities, ultimately promoting equitable access to quality care for everyone.
Challenge and Opportunity
AI has the potential to revolutionize healthcare, but its misuse and unequal access can lead to unintended dire consequences. For instance, algorithms may inadvertently favor certain demographic groups, allocating resources disproportionately and deepening disparities. Efforts to establish equitable AI in healthcare have seen significant momentum and support from various governmental agencies and organizations, specifically regarding medical devices. The White House recently announced substantial investments, including $140 million for the National Science Foundation (NSF) to establish institutes dedicated to assessing existing generative AI (GenAI) systems. While not specific to healthcare, President Biden’s blueprint for an “AI Bill of Rights” outlines principles to guide AI design, use, and deployment, aiming to protect individuals from its potential harms. The Food and Drug Administration (FDA) has also taken steps by releasing a beta version of its regulatory framework for medical device AI used in healthcare. The Department of Health and Human Services (DHHS) has proposed revisions to Section 1557 of the Patient Protection and Affordable Care Act, which would explicitly prohibit discrimination in the use of clinical algorithms to support decision-making in covered entities.
How Inequities in CDS AI Hurt Healthcare Delivery
Exacerbate and Perpetuate Health Disparities
The inequitable use of AI has the potential to exacerbate health disparities. Studies have revealed how population health management algorithms, which proxy healthcare needs with costs, allocate more care to white patients than to Black patients, even when health needs are accounted for. This disparity arises because the proxy target, correlated with access to and use of healthcare services, tends to identify frequent users of healthcare services, who are disproportionately less likely to be Black patients due to existing inequities in healthcare access. Inequitable AI perpetuates data bias when trained on skewed or incomplete datasets, inheriting and reinforcing the biases through algorithmic decisions, thereby deepening existing disparities and hindering efforts to achieve fairness and equity in healthcare delivery.
Increased Costs
Algorithms trained on biased datasets may exacerbate disparities by misdiagnosing or overlooking conditions prevalent in marginalized communities, leading to unnecessary tests, treatments, and hospitalizations and driving up costs. Health disparities, estimated to contribute $320 billion in excess healthcare spending, are compounded by the uneven adoption of AI in healthcare. The unequal access to AI-driven services widens gaps in healthcare spending, with affluent communities and resource-rich health systems often pioneering AI technologies, leaving underserved areas behind. Consequently, delayed diagnoses and suboptimal treatments escalate healthcare spending due to preventable complications and advanced disease stages.
Decreased Trust
The unequal distribution of AI-driven healthcare services breeds skepticism within marginalized communities. For instance, in one study, an algorithm demonstrated statistical fairness in predicting healthcare costs for Black and white patients, but disparities emerged in service allocation, with more white patients receiving referrals despite similar sickness levels. This disparity undermines trust in AI-driven decision-making processes, ultimately adding to mistrust in healthcare systems and providers.
How Bias Infiltrates CDS AI
Lack of Data Diversity and Inclusion
The datasets used to train AI models often mirror societal and healthcare inequities, propagating biases present in the data. For instance, if a model is trained on data from a healthcare system where certain demographic groups receive inferior care, it will internalize and perpetuate those biases. Compounding the issue, limited access to healthcare data leads AI researchers to rely on a handful of public databases, contributing to dataset homogeneity and lacking diversity. Additionally, while many clinical factors have evidence-based definitions and data collection standards, attributes that often account for variance in healthcare outcomes are less defined and more sparsely collected. As such, efforts to define and collect these attributes and promote diversity in training datasets are crucial to ensure the effectiveness and fairness of AI-driven healthcare interventions.
Lack of Transparency and Accountability
While AI systems are designed to streamline processes and enhance decision-making across healthcare, they also run the risk of inadvertently inheriting discrimination from their human creators and the environments from which they draw data. Many AI decision support technologies also struggle with a lack of transparency, making it challenging to fully comprehend and appropriately use their insights in a complex, clinical setting. By gaining clear visibility into how AI systems reach conclusions and establishing accountability measures for their decisions, the potential for harm can be mitigated and fairness promoted in their application. Transparency allows for the identification and remedy of any inherited biases, while accountability incentivizes careful consideration of how these systems may negatively or disproportionately impact certain groups. Both are necessary to build public trust that AI is developed and used responsibly.
Algorithmic Biases
The potential for algorithmic bias to permeate healthcare AI is significant and multifaceted. Algorithms and heuristics used in AI models can inadvertently encode biases that further disadvantage marginalized groups. For instance, an algorithm that assigns greater importance to variables like income or education levels may systematically disadvantage individuals from socioeconomically disadvantaged backgrounds.
Data scientists can adjust algorithms to reduce AI bias by tuning hyperparameters that optimize decision thresholds. These thresholds for flagging high-risk patients may need adjustment for specific groups to balance accuracy. Regular monitoring ensures thresholds address emerging biases over time. In addition, fairness-aware algorithms can apply statistical parity, where protected attributes like race or gender do not predict outcomes.
Unequal Access
Unequal access to AI technology exacerbates existing disparities and subjects the entire healthcare system to heightened bias. Even if an AI model itself is developed without inherent bias, the unequal distribution of access to its insights and recommendations can perpetuate inequities. When only healthcare organizations that can afford advanced AI for CDS leverage these tools, their patients enjoy the advantages of improved care that remain inaccessible to disadvantaged groups. Federal policy initiatives must prioritize equitable access to AI by implementing targeted investments, incentives, and partnerships for underserved populations. By ensuring that all healthcare entities, regardless of financial resources, have access to AI technologies, policymakers can help mitigate biases and promote fairness in healthcare delivery.
Misuse
The potential for bias in healthcare through the misuse of AI extends beyond the composition of training datasets to encompass the broader context of AI application and utilization. Ensuring the generalizability of AI predictions across diverse healthcare settings is as imperative as equity in the development of algorithms. It necessitates a comprehensive understanding of how AI applications will be deployed and whether the predictions derived from training data will effectively translate to various healthcare contexts. Failure to consider these factors may lead to improper use or abuse of AI insights.
Opportunity
Urgent policy action is essential to address bias, promote diversity, increase transparency, and enforce accountability in CDS AI systems. By implementing responsible oversight and governance, policymakers can harness the potential of AI to enhance healthcare delivery and reduce costs, while also ensuring fairness and inclusion. Regulations mandating the auditing of AI systems for bias and requiring explainability, auditing, and validation processes can hold organizations accountable for the ethical development and deployment of healthcare technologies. Furthermore, policymakers can establish guidelines and allocate funding to maximize the benefits of AI technology while safeguarding vulnerable groups. With lives at stake, eliminating bias and ensuring equitable access must be a top priority, and policymakers must seize this opportunity to enact meaningful change. The time for action is now.
Plan of Action
The federal government should establish and implement standards in AI governance and auditing for algorithms directly influencing diagnosis, treatment, and access to care of patients. These efforts should address and measure issues such as bias, transparency, accountability, and fairness. They should be flexible enough to accommodate advancements in AI technology while ensuring that ethical considerations remain paramount.
Regulate Auditing and Governance of AI
The federal government should implement a detailed auditing framework for AI in healthcare, beginning with stringent pre-deployment evaluations that require rigorous testing and validation against established industry benchmarks. These evaluations should thoroughly examine data privacy protocols to ensure patient information is securely handled and protected. Algorithmic transparency must be prioritized, requiring developers to provide clear documentation of AI decision-making processes to facilitate understanding and accountability. Bias mitigation strategies should be scrutinized to ensure AI systems do not perpetuate or exacerbate existing healthcare disparities. Performance reliability should be continuously monitored through real-time data analysis and periodic reviews, ensuring AI systems maintain accuracy and effectiveness over time. Regular audits should be mandated to verify ongoing compliance, with a focus on adapting to evolving standards and incorporating feedback from healthcare professionals and patients. AI algorithms evolve due to shifts in the underlying data, model degradation, and changes to application protocols. Therefore, routine auditing should occur at a minimum of annually.
With nearly 40% of Americans receiving benefits under a Medicare or Medicaid program, and the tremendous growth and focus on value-based care, the Centers for Medicare & Medicaid Services (CMS) is positioned to provide the catalyst to measure and govern equitable AI. Since many health systems and payers leverage models across multiple other populations, this could positively affect the majority of patient care. Both the companies making critical decisions and those developing the technology should be obliged to assess the impact of decision processes and submit select impact-assessment documentation to CMS.
For healthcare facilities participating in CMS programs, this mandate should be included as a Condition of Participation. Through this same auditing process, the federal government can capture insight into the performance and responsibility of AI systems. These insights should be made available to healthcare organizations throughout the country to increase transparency and quality between AI partners and decision-makers. This will help the Department of Health and Human Services (HHS) meet the “Promote Trustworthy AI Use and Development” pillar of its AI strategy (Figure 1).
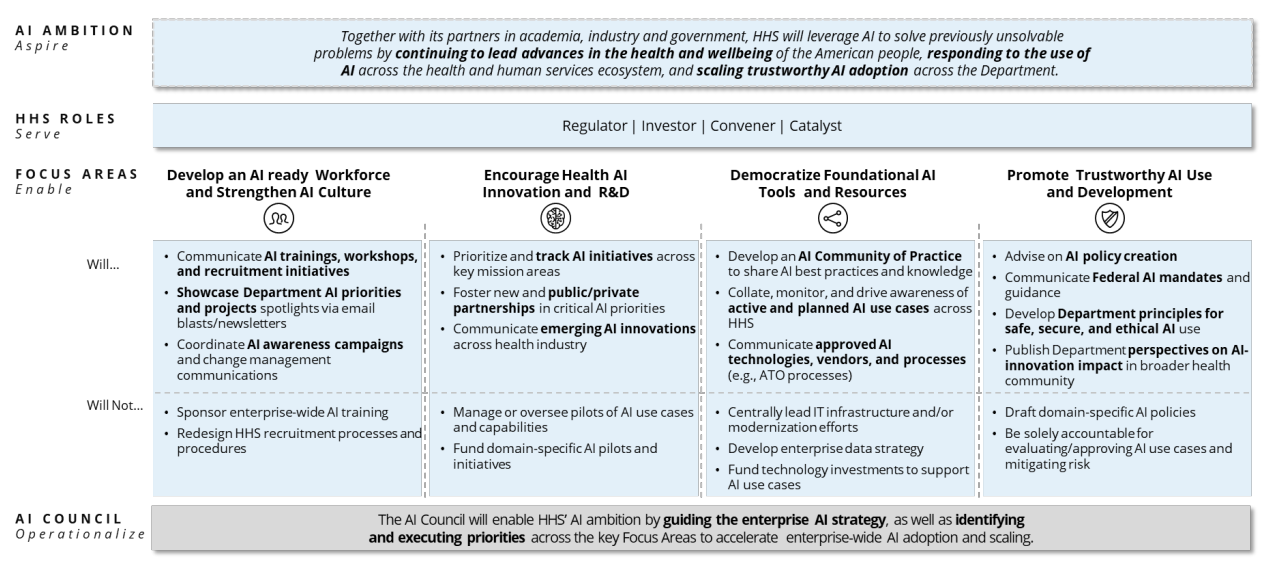
Congress must enforce these systems of accountability for advanced algorithms. Such work could be done by amending and passing the 2023 Algorithmic Accountability Act. This proposal mandates that companies evaluate the effects of automating critical decision-making processes, including those already automated. However, it fails to make these results visible to the organizations that leveraging these tools. An extension should be added to make results available to governing bodies and member organizations, such as the American Hospital Association (AHA).
Invest in AI Accessibility and Improvement
AI that integrates the social and clinical risk factors that influence preventive care could be beneficial in managing health outcomes and resource allocation, specifically for facilities providing care to mostly rural areas and patients. While organizations serving large proportions of marginalized patients may have access to nascent AI tools, it is very likely they are inadequate given they weren’t trained with data adequately representing this population. Therefore, the federal government should allocate funding to support AI access for healthcare organizations serving higher percentages of vulnerable populations. Initial support should stem from subsidies to AI service providers that support safety net and rural health providers.
The Health Resources and Services Administration should deploy strategic innovation funding to federally qualified health centers and rural health providers to contribute to and consume equitable AI. This could include funding for academic institutions, research organizations, and private-sector partnerships focused on developing AI algorithms that are fair, transparent, and unbiased specific for these populations.
Large language models (LLM) and GenAI solutions are being rapidly adopted in CDS tooling, providing clinicians with an instant second opinion in diagnostic and treatment scenarios. While these tools are powerful, they are not infallible and pose a risk without the ability to evolve. Therefore, research regarding AI self-correction should be a focus of future policy. Self-correction is the ability for an LLM or GenAI to identify and rectify errors without external or human intervention. Mastering the ability for these complex engines to recognize possible life-threatening errors would be crucial in their adoption and application. Healthcare agencies, such as the Agency for Healthcare Research and Quality (AHRQ) and the Office of the National Coordinator for Health Information Technology, should fund and oversee research for AI self-correction specifically leveraging clinical and administrative claims data. This should be an extension of either of the following efforts:
- 45 CFR Parts 170, 171 as it “promotes the responsible development and use of artificial intelligence through transparency and improves patient care through policies…which are central to the Department of Health and Human Services’ efforts to enhance and protect the health and well-being of all Americans”
- AHRQ’s funding opportunity from May 2024 (NOT-HS-24-014), Examining the Impact of AI on Healthcare Safety (R18)
Much like the Breakthrough Device Program, AI that can prove it decreases health disparities and/or increases accessibility can be fast-tracked through the audit process and highlighted as “best-in-class.”
Incentivize Data Collection and Collaboration
The newly released “Driving U.S. Innovation in Artificial Intelligence” roadmap considers healthcare a high-impact area for AI and makes specific recommendations for future “legislation that supports further deployment of AI in health care and implements appropriate guardrails and safety measures to protect patients,… and promoting the usage of accurate and representative data.” While auditing and enabling accessibility in healthcare AI, the government must ensure that the path to build equity into AI solutions does not remain an obstacle. This entails improved data collection and data sharing to ensure that AI algorithms are trained on diverse and representative datasets. As the roadmap declares, there must be “support the NIH in the development and improvement of AI technologies…with an emphasis on making health care and biomedical data available for machine learning and data science research while carefully addressing the privacy issues raised by the use of AI in this area.”
These data exist across the healthcare ecosystem, and therefore decentralized collaboration can enable a more diverse corpus of data to be available to train AI. This may involve incentivizing healthcare organizations to share anonymized patient data for research purposes while ensuring patient privacy and data security. This incentive could come in the form of increased reimbursement from CMS for particular services or conditions that involve collaborating parties.
To ensure that diverse perspectives are considered during the design and implementation of AI systems, any regulation handed down from the federal government should not only encourage but evaluate the diversity and inclusivity in AI development teams. This can help mitigate biases and ensure that AI algorithms are more representative of the diverse patient populations they serve. This should be evaluated by accrediting parties such as The Joint Commission (a CMS-approved accrediting organization) and their Healthcare Equity Certification.
Conclusion
Achieving health equity through AI in CDS requires concerted efforts from policymakers, healthcare organizations, researchers, and technology developers. AI’s immense potential to transform healthcare delivery and improving outcomes can only be realized if accompanied by measures to address biases, ensure transparency, and promote inclusivity. As we navigate the evolving landscape of healthcare technology, we must remain vigilant in our commitment to fairness and equity so that AI can serve as a tool for empowerment rather than perpetuating disparities. Through collective action and awareness, we can build a healthcare system that truly leaves no one behind.
This idea is part of our AI Legislation Policy Sprint. To see all of the policy ideas spanning innovation, education, healthcare, and trust, safety, and privacy, head to our sprint landing page.
By providing essential funding mechanisms, the Bioeconomy Finance Program will reduce the risks inherent in biotechnology innovation, encouraging more private sector investment.
While the U.S. has made significant advancements and remained a global leader in biotechnology over the past decade, the next four years will be critical in determining whether it can sustain that leadership.
It’s paramount to balance both innovation capabilities and risk as we work towards ensuring that the U.S. bioeconomy is a priority area for both the Nation and for National Security.
The Federation of American Scientists supports the National Security Commission on Emerging Biotechnology’s Final Report and the Recommendations contained within it.