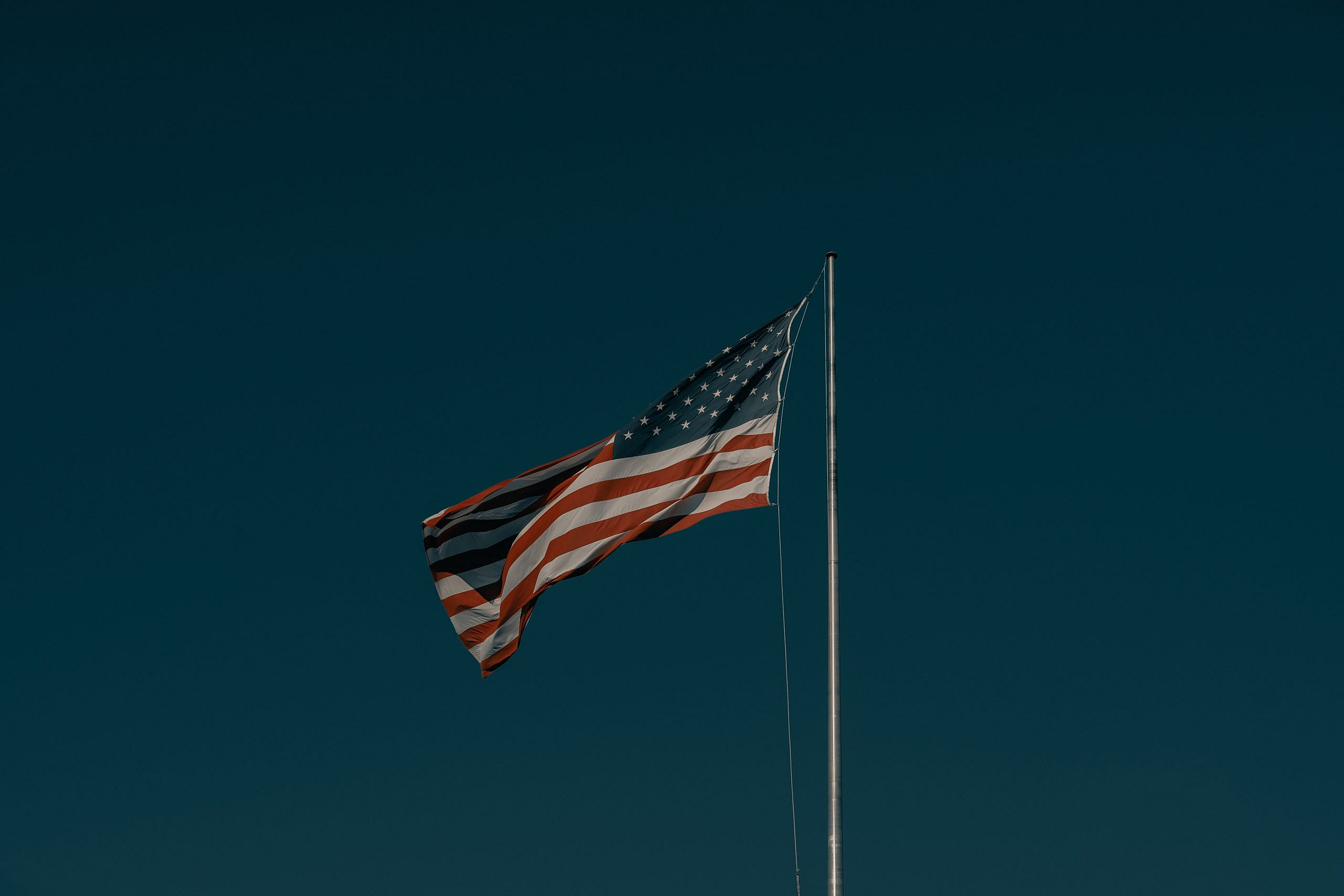
Modernizing Enforcement of the Civil Rights Act to Mitigate Algorithmic Harm in Determining Federal Benefits
The Department of Justice should modernize the enforcement of Title VI of the Civil Rights Act to guide effective corrective action for algorithmic systems that produce discriminatory outcomes with regard to federal benefits. To do so, the Department of Justice should clarify the definition of “algorithmic discrimination” in the context of federal benefits, establish systems to identify which federally funded public benefits offices use machine-learning algorithms, and secure the necessary human resources to properly address algorithmic discrimination. This crucial action would leverage a demonstrable, growing interest in regulating algorithms that has bloomed over the past year via policy actions in both the White House and Congress but has yet to concretely establish an appropriate enforcement mechanism for acting on instances of demonstrated algorithmic harm.
Challenge and Opportunity
Algorithmic systems are inescapable in modern life. They have become core elements of everyday activities, like surfing the web, driving to work, and applying for a job. It is virtually impossible to go through life without encountering an algorithmic system multiple times per day.
As machine-learning technologies have become more pervasive, they have also become gatekeepers for crucial resources, like accessing credit, receiving healthcare, securing housing, and getting a mortgage. Both local and federal governments have embraced algorithmic decision-making to determine which constituents are able to access key services, often with little transparency, if any, for those who are subject to such decision-making.
When it comes to federal benefits, imperfections in these systems scale significantly. For example, the deployment of flawed algorithmic tools led to the wrongful termination of Medicaid for 19% of beneficiaries in Arkansas, the wrongful termination of Social Security income for thousands in New York, wrongful termination of $78 million worth of Medicaid and Supplemental Nutrition Assistance Program benefits in Indiana, and erroneous unemployment fraud charges for 40,000 people in Michigan. These errors are particularly harmful to low-income Americans for whom access to credit, housing, job opportunities, and healthcare are especially important.
Over the past year, momentum for regulating algorithmic systems has grown, resulting in several key policy actions. In February 2022, Senators Ron Wyden and Cory Booker and Representative Yvette Clarke introduced the Algorithmic Accountability Act. Endorsed by AI experts, this bill would have required deployers of algorithmic systems to conduct and publicly share impact assessments of their systems. In October 2022, the White House released its Blueprint for an AI Bill of Rights. Although not legally enforceable, this robust rights-based framework for algorithmic systems was developed with a broad coalition of support through an intensive, yearlong public consultation process with community members, private sector representatives, tech workers, and policymakers. Also in October 2022, the AI Training Act was passed into law. The legislation requires the development of a training curriculum covering core concepts in artificial intelligence for federal employees in a limited range of roles, primarily those involved in procurement. Finally, January 2023 saw the introduction of the NIST AI Risk Management Framework to guide how organizations and individuals design, develop, deploy, or use artificial intelligence to manage risk and promote responsible use.
Collectively, these actions demonstrate clear interest in preventing harm caused by algorithmic systems, but none of them provide clear enforcement mechanisms for federal agencies to pursue corrective action in the wake of demonstrated algorithmic harm.
However, Title VI of the Civil Rights Act offers a viable and legally enforceable mechanism to aid anti-discrimination efforts in the algorithmic age. At its core, Title VI bans the use of federal funding to support programs (including state and local governments, educational institutions, and private companies) that discriminate on the basis of race, color, or national origin. Modernizing the enforcement of Title VI, specifically in the context of federal benefits, offers a clear opportunity for developing and refining a modern enforcement approach to civil rights law that can respond appropriately and effectively to algorithmic discrimination.
Plan of Action
Fundamentally, this plan of action seeks to:
- Clarify how “algorithmic bias” is defined, specifically in the context of federal benefits.
- Identify where and when public benefits systems use machine-learning algorithms.
- Equip federal agencies with authority and skill sets to address algorithmic discrimination.
Clarify the Framework for Algorithmic Bias in Federal Benefits
Recommendation 1. Fund the Department of Justice (DOJ) to develop a new working group focused specifically on civil rights concerns around artificial intelligence.
The DOJ has already requested funding for and justified the existence of this unit in its FY2023 Performance Budget. In that budget, the DOJ requested $4.45 million to support 24 staff.
Clear precedents for this type of cross-sectional working group already exist within the Department of Justice (e.g., the Indian Working Group and LGBTQI+ Working Group). Both of these groups contain members of the 11 sections of the Civil Rights Division to ensure a comprehensive strategy for protecting the civil rights of Indigenous peoples and the LGBTQ+ community, respectively. The pervasiveness of algorithmic systems in modern life suggests a similarly broad scope is appropriate for this issue.
Recommendation 2. Direct the working group to develop a framework that defines algorithmic discrimination and appropriate corrective action specifically in the context of public benefits.
A clear framework or rubric for assessing when algorithmic discrimination has occurred is a prerequisite for appropriate corrective action. Despite having a specific technical definition, the term “algorithmic bias” can vary widely in its interpretation depending on the specific context in which an automated decision is being made. Even if algorithmic bias does exist, researchers and legal scholars have made the case that biased algorithms may be preferable to biased human decision-makers on the basis of consistency and the relative ease of behavior change. Consequently, the DOJ should develop a context-specific framework for determining when algorithmic bias leads to harmful discriminatory outcomes in federal benefits systems, starting with major federal systems like Social Security and Medicare/Medicaid.
As an example, the Brookings Institution has produced a helpful report that illustrates what it means to define algorithmic bias in a specific context. Cross-walking this blueprint with existing Title VI procedures can yield guidelines for how the Department of Justice can notify relevant offices of algorithmic discrimination and steer corrective action.
Identify Federal Benefits Systems that Use Algorithmic Tools
Recommendation 3. Establish a federal register or database for offices that administer federally funded public benefits to document when they use machine-learning algorithms.
This system should specifically detail the developer of the algorithmic system and the office using said system. If possible, descriptions of relevant training data should be included as well, especially if these data are federal property. Consider working with the Office of Federal Contract Compliance Programs to secure this information from current and future government contractors within the federal benefits domain.
In terms of cost, previous budget requests for databases of this type have ranged from $2 million to $5 million.
Recommendation 4. Provide public access to the federal register.
Making the federal register public would provide baseline transparency regarding the federal funding of algorithmic systems. This would facilitate external investigative efforts to identify possible instances of algorithmic discrimination in public benefits, which would complement internal efforts by directing limited federal staff bandwidth towards cases that have already been identified. The public-facing portion of this registry should be structured to respecting appropriate privacy and trade secrecy restrictions
Recommendation 5. Link the public-facing register to a public-facing form for submitting claims of algorithmic discrimination in the context of federal benefits.
This step would help channel public feedback regarding claims of algorithmic discrimination with a sufficiently high threshold to minimize frivolous claims. A well-designed system will ask for evidence and data to justify any claim of algorithmic discrimination, allowing federal employees to prioritize which claims to pursue.
Equip Agencies with Necessary Resources for Addressing Algorithmic Discrimination
Recommendation 6. Authorize funding for technical hires in enforcement arms of federal regulatory agencies, including but not limited to the Department of Justice.
Effective enforcement of anti-discrimination statutes today requires technical fluency in machine-learning techniques. In addition to the DOJ’s Civil Rights Division (see Recommendation 1), consider directing funds to hire or train technical experts within the enforcement arms of other federal agencies with explicit anti-discrimination enforcement authority, including the Federal Trade Commission, Federal Communications Commission, and Department of Education.
Recommendation 7. Pass the Stopping Unlawful Negative Machine Impacts through National Evaluation Act.
This act was introduced with bipartisan support in the Senate at the very end of the 2021–2022 legislative session by Senator Rob Portman. The short bill seeks to clarify that civil rights legislation applies to artificial intelligence systems and decisions made by these systems will be liable to claims of discrimination under said legislation, including the Civil Rights Act, the Americans with Disabilities Act, and the Age Discrimination Act of 1975, among others. Passing the bill is a simple but effective way to indicate to federal regulatory agencies (and those they regulate) that artificial intelligence systems must comply with civil rights law and affirms the federal government’s authority to ensure they do so.
Conclusion
On his first day in office, President Biden signed an executive order to address the entrenched denial of equal opportunities for underserved communities in the United States. Ensuring that federal benefits are not systematically denied via algorithmic discrimination to low-income Americans and Americans of color is crucial to successfully meeting the goals of that order and the rising chorus of voices who want meaningful regulation for algorithmic systems. The authority for such regulation in the context of federal benefits already exists. To ensure that authority can be effectively enforced in the modern age, the federal government needs to clearly define algorithmic discrimination in the context of federal benefits, identify where federal funding is supporting algorithmic determination of federal benefits, and recruit the necessary talent to verify instances of algorithmic discrimination.
An algorithm is a structured set of steps for doing something. In the context of this memo, an algorithm usually means computer code that is written to do something in a structured, repeatable way, such as determining if someone is eligible for Medicare, identifying someone’s face using a facial recognition tool, or matching someone’s demographic profile to a certain kind of advertisement.
Machine-learning techniques are a specific set of algorithms that train a computer to do different tasks by taking in a massive amount of data and looking for patterns. Artificial intelligence generally refers to technical systems that have been trained to perform tasks with minimal human oversight. Machine learning and artificial intelligence are similar and often used as interchangeable terms.
We can identify algorithmic bias by comparing the expected outputs of an algorithm to the actual outputs for an algorithm. For example, if we find that an algorithm uses race as a decisive factor in determining whether someone is eligible for federal benefits that should be race-neutral, that would be an example of algorithmic bias. In practice, these assessments often take the form of statistical tests that are run over multiple outputs of the same algorithmic system.
Although many algorithms are biased, not all biases are equally harmful. This is due to the highly contextual nature in which an algorithm is used. For example, a false positive in a criminal-sentencing algorithm arguably causes more harm than a false positive in a federal benefits determination. Algorithmic bias is not inherently a bad thing and, in some cases, can actually advance equity and inclusion efforts depending on the specific contexts (consider a hiring algorithm for higher-level management that weights non-male gender or non-white race more heavily for selection).
While the U.S. has made significant advancements and remained a global leader in biotechnology over the past decade, the next four years will be critical in determining whether it can sustain that leadership.
It’s paramount to balance both innovation capabilities and risk as we work towards ensuring that the U.S. bioeconomy is a priority area for both the Nation and for National Security.
The Federation of American Scientists supports the National Security Commission on Emerging Biotechnology’s Final Report and the Recommendations contained within it.
The U.S. should create a new non-governmental Innovation Accelerator modeled after the successful In-Q-Tel program to invest in small and mid-cap companies creating technologies that address critical needs of the United States.