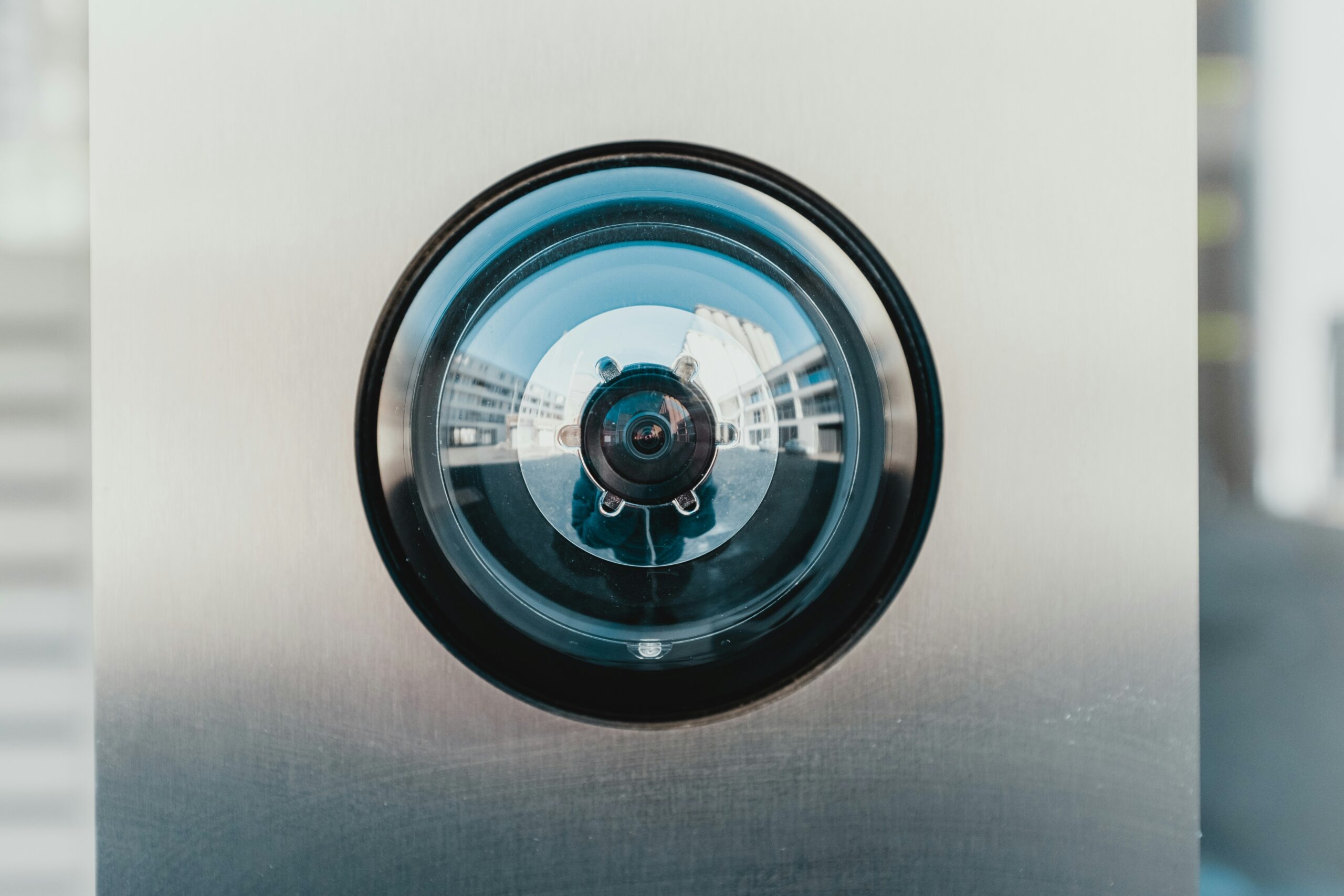
Increasing Responsible Data Sharing Capacity throughout Government
Deriving insights from data is essential for effective governance. However, collecting and sharing data—if not managed properly—can pose privacy risks for individuals. Current scientific understanding shows that so-called “anonymization” methods that have been widely used in the past are inadequate for protecting privacy in the era of big data and artificial intelligence. The evolving field of Privacy-Enhancing Technologies (PETs), including differential privacy and secure multiparty computation, offers a way forward for sharing data safely and responsibly.
The administration should prioritize the use of PETs by integrating them into data-sharing processes and strengthening the executive branch’s capacity to deploy PET solutions.
Challenge and Opportunity
A key function of modern government is the collection and dissemination of data. This role of government is enshrined in Article 1, Section 2 of the U.S. Constitution in the form of the decennial census—and has only increased with recent initiatives to modernize the federal statistical system and expand evidence-based policymaking. The number of datasets itself has also grown; there are now over 300,000 datasets on data.gov, covering everything from border crossings to healthcare. The release of these datasets not only accomplishes important transparency goals, but also represents an important step toward advancing American society fairer, as data are a key ingredient in identifying policies that benefit the public.
Unfortunately, the collection and dissemination of data comes with significant privacy risks. Even with access to aggregated information, motivated attackers can extract information specific to individual data subjects and cause concrete harm. A famous illustration of this risk occurred in 1997 when Latanya Sweeney was able to identify the medical record of then-Governor of Massachusetts, William Weld, from a public, anonymized dataset. Since then, the power of data re-identification techniques—and incentives for third parties to learn sensitive information about individuals—have only increased, compounding this risk. As a democratic, civil-rights respecting nation, it is irresponsible for our government agencies to continue to collect and disseminate datasets without careful consideration of the privacy implications of data sharing.
While there may appear to be an irreconcilable tension between facilitating data-driven insight and protecting the privacy of individual’s data, an emerging scientific consensus shows that Privacy-Enhancing Technologies (PETs) offer a path forward. PETs are a collection of techniques that enable data to be used while tightly controlling the risk incurred by individual data subjects. One particular PET, differential privacy (DP), was recently used by the U.S. Census Bureau within their disclosure avoidance system for the 2020 decennial census in order to meet their dual mandates of data release and confidentiality. Other PETs, including variations of secure multiparty computation, have been used experimentally by other agencies, including to link long-term income data to college records and understand mental health outcomes for individuals who have earned doctorates. The National Institute of Standards and Technology (NIST) has produced frameworks and reports on data and information privacy, including PETs topics such as DP (see Q&A section). However, these reports still lack a comprehensive and actionable framework on how organizations should consider, use and deploy PETs in organizations.
As artificial intelligence becomes more prevalent inside and outside government and relies on increasingly large datasets, the need for responsible data sharing is growing more urgent. The federal government is uniquely positioned to foster responsible innovation and set a strong example by promoting the use of PETs. The use of DP in the 2020 decennial census was an extraordinary example of the government’s capacity to lead global innovation in responsible data sharing practices. While the promise of continuing this trend is immense, expanding the use of PETs within government poses twin challenges: (1) sharing data within government comes with unique challenges—both technical and legal—that are only starting to be fully understood and (2) expertise on using PETs within government is limited. In this proposal, we outline a concrete plan to overcome these challenges and unlock the potential of PETs within government.
Plan of Action
Using PETs when sharing data should be a key priority for the executive branch. The new administration should encourage agencies to consider the use of PETs when sharing data and build a United States DOGE Service (USDS) “Responsible Data Sharing Corps” of professionals who can provide in-house guidance around responsible data sharing.
We believe that enabling data sharing with PETs requires (1) gradual, iterative refinement of norms and (2) increased capacity in government. With these in mind, we propose the following recommendations for the executive branch.
Strategy Component 1. Build consideration of PETs into the process of data sharing
Recommendation 1. NIST should produce a decision-making framework for organizations to rely on when evaluating the use of PETs.
NIST should provide a step-by-step decision-making framework for determining the appropriate use of PETs within organizations, including whether PETs should be used, and if so, which PET and how it should be deployed. Specifically, this guidance should be at the same level of granularity as NIST Risk Management Framework for Cybersecurity. NIST should consult with a range of stakeholders from the broad data sharing ecosystem to create this framework. This includes data curators (i.e., organizations that collect and share data, within and outside the government); data users (i.e., organizations that consume, use and rely on shared data, including government agencies, special interest groups and researchers); data subjects; experts across fields such as information studies, computer science, and statistics; and decision makers within public and private organizations who have prior experience using PETs for data sharing. The report may build on NIST’s existing related publications and other guides for policymakers considering the use of specific PETs, and should provide actionable guidance on factors to consider when using PETs. The output of this process should be not only a decision, but also a report documenting the execution of decision-making framework (which will be instrumental for Recommendation 3).
Recommendation 2. The Office of Management and Budget (OMB) should mandate government agencies interested in data sharing to use the NIST’s decision-making framework developed in Recommendation 1 to determine the appropriateness of PETs to protect their data pipelines.
The risks to data subjects associated with data releases can be significantly mitigated with the use of PETs, such as differential privacy. Along with considering other mechanisms of disclosure control (e.g., tiered access, limiting data availability), agencies should investigate the feasibility and tradeoffs around using PETs to protect data subjects while sharing data for policymaking and public use. To that end, OMB should require government agencies to use the decision-making framework produced by NIST (in Recommendation 1) for each instance of data sharing. We emphasize that this decision-making process may lead to a decision not to use PETs, as appropriate. Agencies should compile the produced reports such that they can be accessed by OMB as part of Recommendation 3.
Recommendation 3. OMB should produce a PET Use Case Inventory and annual reports that provide insights on the use of PETs in government data-sharing contexts.
To promote transparency and shared learning, agencies should share the reports produced as part of their PET deployments and associated decision-making processes with OMB. Using these reports, OMB should (1) publish a federal government PET Use Case Inventory (similar to the recently established Federal AI Use Case Inventory) and (2) synthesize these findings into an annual report. These findings should provide high-level insights into the decisions that are being made across agencies regarding responsible data sharing, and highlight the barriers to adoption of PETs within various government data pipelines. These reports can then be used to update the decision-making frameworks we propose that NIST should produce (Recommendation 1) and inspire further technical innovation in academia and the private sector.
Strategy Component 2. Build capacity around responsible data sharing expertise
Increasing in-depth decision-making around responsible data sharing—including the use of PETs—will require specialized expertise. While there are some government agencies with teams well-trained in these topics (e.g., the Census Bureau and its team of DP experts), expertise across government is still lacking. Hence, we propose a capacity-building initiative that increases the number of experts in responsible data sharing across government.
Recommendation 4. Announce the creation of a “Responsible Data Sharing Corps.”
We propose that the USDS create a “Responsible Data Sharing Corps” (RDSC). This team will be composed of experts in responsible data sharing practices and PETs. RDSC experts can be deployed into other government agencies as needed to support decision-making about data sharing. They may also be available for as-needed consultations with agencies to answer questions or provide guidance around PETs or other relevant areas of expertise.
Recommendation 5. Build opportunities for continuing education and training for RDSC members.
Given the evolving nature of responsible data practices, including the rapid development of PETs and other privacy and security best practices, members of the RDSC should have 20% effort reserved for continuing education and training. This may involve taking online courses or attending workshops and conferences that describe state-of-the-art PETs and other relevant technologies and methodologies.
Recommendation 6. Launch a fellowship program to maintain the RDSC’s cutting-edge expertise in deploying PETS.
Finally, to ensure that the RDSC stays at the cutting edge of relevant technologies, we propose an RDSC fellowship program similar to or part of the Presidential Innovation Fellows. Fellows may be selected from academia or industry, but should have expertise in PETs and propose a novel use of PETs in a government data-sharing context. During their one-year terms, fellows will perform their proposed work and bring new knowledge to the RDSC.
Conclusion
Data sharing has become a key priority for the government in recent years, but privacy concerns make it critical to modernize technology for responsible data use to leverage data for policymaking and transparency. PETs such as differential privacy, secure multiparty computation, and others offer a promising way forward. However, deploying PETs at a broad scale requires changing norms and increasing capacity in government. The executive branch should lead these efforts by encouraging agencies to consider PETs when making data-sharing decisions and building a “Responsible Data Sharing Corps” who can provide expertise and support for agencies in this effort. By encouraging the deployment of PETs, the government can increase fairness, utility and transparency of data while protecting itself—and its data subjects—from privacy harms.
This action-ready policy memo is part of Day One 2025 — our effort to bring forward bold policy ideas, grounded in science and evidence, that can tackle the country’s biggest challenges and bring us closer to the prosperous, equitable and safe future that we all hope for whoever takes office in 2025 and beyond.
PLEASE NOTE (February 2025): Since publication several government websites have been taken offline. We apologize for any broken links to once accessible public data.
Data sharing requires a careful balance of multiple factors, with privacy and utility being particularly important.
- Data products released without appropriate and modern privacy protection measures could facilitate abuse, as attackers can weaponize information contained in these data products against individuals, e.g., blackmail, stalking, or publicly harassing those individuals.
- On the other hand, the lack of accessible data can also cause harm due to reduced utility: various actors, such as state and local government entities, may have limited access to accurate or granular data, resulting in the inefficient allocation of resources to small or marginalized communities.
Privacy-Enhancing Technologies is a broad umbrella category that includes many different technical tools. Leading examples of these tools include differential privacy, secure multiparty computation, trusted execution environments, and federated learning. Each one of these technologies is designed to address different privacy threats. For additional information, we suggest the UN Guide on Privacy-Enhancing Technologies for Official Statistics and the ICO’s resources on Privacy-Enhancing Technologies.
NIST has multiple publications related to data privacy, such as the Risk Management Framework for Cybersecurity and the Privacy Framework. The report De-Identifying Government Datasets: Techniques and Governance focuses on responsible data sharing by government organizations, while the Guidelines for Evaluating Differential Privacy Guarantees provides a framework to assess the privacy protection level provided by differential privacy for any organization.
Differential privacy is a framework for controlling the amount of information leaked about individuals during a statistical analysis. Typically, random noise is injected into the results of the analysis to hide individual people’s specific information while maintaining overall statistical patterns in the data. For additional information, we suggest Differential Privacy: A Primer for a Non-technical Audience.
Secure multiparty computation is a technique that allows several actors to jointly aggregate information while protecting each actor’s data from disclosure. In other words, it allows parties to jointly perform computations on their data while ensuring that each party learns only the result of the computation. For additional information, we suggest Secure Multiparty Computation FAQ for Non-Experts.
There are multiple examples of PET deployments at both the federal and local levels both domestically and internationally. We list several examples below, and refer interested readers to the in-depth reports by Advisory Committee on Data for Evidence Building (report 1 and report 2):
- The Census Bureau used differential privacy in their disclosure avoidance system to release results from the 2020 decennial census data. Using differential privacy allowed the bureau to provide formal disclosure avoidance guarantees as well as precise information about the impact of this system on the accuracy of the data.
- The Boston Women’s Workforce Council (BWWC) measures wage disparities among employers in the greater Boston area using secure multiparty computation (MPC).
- The Israeli Ministry of Health publicly released its National Life Birth Registry using differential privacy.
- Privacy-preserving record linkage, a variant of secure multiparty computation, has been used experimentally by both the U.S. Department of Education and the National Center for Health Statistics. Additionally, it has been used at the county level in Allegheny County, PA.
Additional examples can also be found in the UN’s case-study repository of PET deployments.
Data-sharing projects are not new to the government, and pockets of relevant expertise—particularly in statistics, software engineering, subject matter areas, and law—already exist. Deploying PET solutions requires technical computer science expertise for building and integrating PETs into larger systems, as well as sociotechnical expertise in communicating the use of PETs to relevant parties and facilitating decision-making around critical choices.
The Federation of American Scientists supports the National Security Commission on Emerging Biotechnology’s Final Report and the Recommendations contained within it.
The U.S. should create a new non-governmental Innovation Accelerator modeled after the successful In-Q-Tel program to invest in small and mid-cap companies creating technologies that address critical needs of the United States.
To sustain America’s leadership in AI innovation, accelerate adoption across the economy, and guarantee that AI systems remain secure and trustworthy, we offer a set of policy recommendations.
Current scientific understanding shows that so-called “anonymization” methods that have been widely used in the past are inadequate for protecting privacy in the era of big data and artificial intelligence.