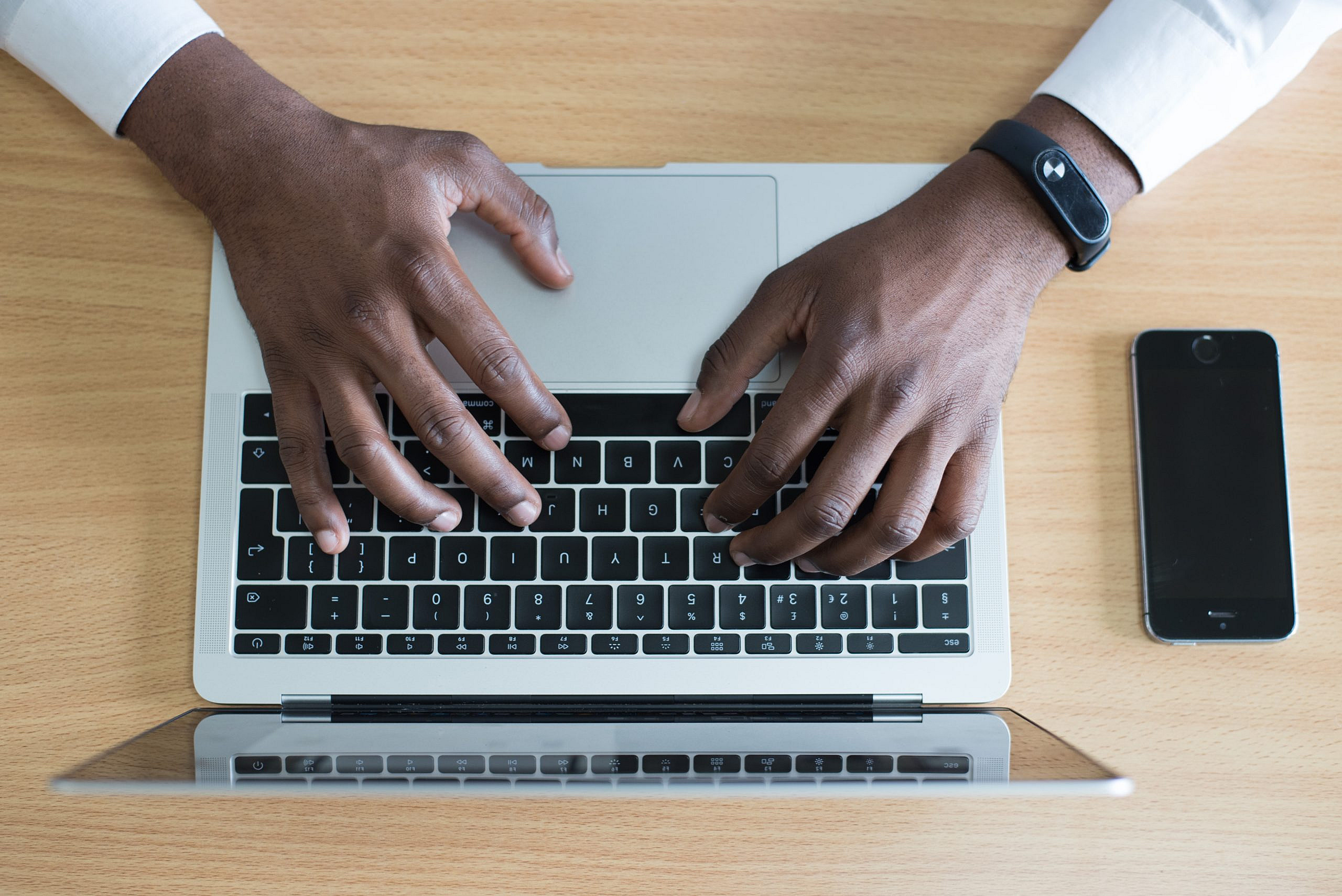
Ensuring Racial Equity in Federal Procurement and Use of Artificial Intelligence
In pursuit of lower costs and improved decision-making, federal agencies have begun to adopt artificial intelligence (AI) to assist in government decision-making and public administration. As AI occupies a growing role within the federal government, algorithmic design and evaluation will increasingly become a key site of policy decisions. Yet a 2020 report found that almost half (47%) of all federal agency use of AI was externally sourced, with a third procured from private companies. In order to ensure that agency use of AI tools is legal, effective, and equitable, the Biden-Harris Administration should establish a Federal Artificial Intelligence Program to govern the procurement of algorithmic technology. Additionally, the AI Program should establish a strict data collection protocol around the collection of race data needed to identify and mitigate discrimination in these technologies.
Researchers who study and conduct algorithmic audits highlight the importance of race data for effective anti-discrimination interventions, the challenges of category misalignment between data sources, and the need for policy interventions to ensure accessible and high-quality data for audit purposes. However, inconsistencies in the collection and reporting of race data significantly limit the extent to which the government can identify and address racial discrimination in technical systems. Moreover, given significant flexibility in how their products are presented during the procurement process, technology companies can manipulate race categories in order to obscure discriminatory practices.
To ensure that the AI Program can evaluate any inequities at the point of procurement, the Office of Science and Technology Policy (OSTP) National Science and Technology Council Subcommittee on Equitable Data should establish guidelines and best practices for the collection and reporting of race data. In particular, the Subcommittee should produce a report that identifies the minimum level of data private companies should be required to collect and in what format they should report such data during the procurement process. These guidelines will facilitate the enforcement of existing anti-discrimination laws and help the Biden-Harris Administration pursue their stated racial equity agenda. Furthermore, these guidelines can help to establish best practices for algorithm development and evaluation in the private sector. As technology plays an increasingly important role in public life and government administration, it is essential not only that government agencies are able to access race data for the purposes of anti-discrimination enforcement—but also that the race categories within this data are not determined on the basis of how favorable they are to the private companies responsible for their collection.
Challenge and Opportunity
Research suggests that governments often have little information about key design choices in the creation and implementation of the algorithmic technologies they procure. Often, these choices are not documented or are recorded by contractors but never provided to government clients during the procurement process. Existing regulation provides specific requirements for the procurement of information technology, for example, security and privacy risks, but these requirements do not account for the specific risks of AI—such as its propensity to encode structural biases. Under the Federal Acquisition Regulation, agencies can only evaluate vendor proposals based on the criteria specified in the associated solicitation. Therefore, written guidance is needed to ensure that these criteria include sufficient information to assess the fairness of AI systems acquired during procurement.
The Office of Management and Budget (OMB) defines minimum standards for collecting race and ethnicity data in federal reporting. Racial and ethnic categories are separated into two questions with five minimum categories for race data (American Indian or Alaska Native, Asian, Black or African American, Native Hawaiian or Other Pacific Islander, and White) and one minimum category for ethnicity data (Hispanic or Latino). Despite these standards, guidelines for the use of racial categories vary across federal agencies and even across specific programs. For example, the Census Bureau classification scheme includes a “Some Other Race” option not used in other agencies’ data collection practices. Moreover, guidelines for collection and reporting of data are not always aligned. For example, the U.S. Department of Education recommends collecting race and ethnicity data separately without a “two or more races” category and allowing respondents to select all race categories that apply. However, during reporting, any individual who is ethnically Hispanic or Latino is reported as only Hispanic or Latino and not any other race. Meanwhile, any respondent who selected multiple race options is reported in a “two or more races” category rather than in any racial group with which they identified.
These inconsistencies are exacerbated in the private sector, where companies are not uniformly constrained by the same OMB standards but rather covered by piecemeal legislation. In the employment context, private companies are required to collect and report on demographic details of their workforce according to the OMB minimum standards. In the consumer lending setting, on the other hand, lenders are typically not allowed to collect data about protected classes such as race and gender. In cases where protected class data can be collected, these data are typically considered privileged information and cannot be accessed by the government. In the case of algorithmic technologies, companies are often able to discriminate on the basis of race without ever explicitly collecting race data by using features or sets of features that act as proxies for protected classes. Facebook’s advertising algorithms, for instance, can be used to target race and ethnicity without access to race data.
Federal leadership can help create consistency in reporting to ensure that the government has sufficient information to evaluate whether privately developed AI is functioning as intended and working equitably. By reducing information asymmetries between private companies and agencies during the procurement process, new standards will bring policymakers back into the algorithmic governance process. This will ensure that democratic and technocratic norms of agency rule-making are respected even as privately developed algorithms take on a growing role in public administration.
Additionally, by establishing a program to oversee the procurement of artificial intelligence, the federal government can ensure that agencies have access to the necessary technical expertise to evaluate complex algorithmic systems. This expertise is crucial not only during the procurement stage but also—given the adaptable nature of AI—for ongoing oversight of algorithmic technologies used within government.
Plan of Action
Recommendation 1. Establish a Federal Artificial Intelligence Program to oversee agency procurement of algorithmic technologies.
The Biden-Harris Administration should create a Federal AI Program to create standards for information disclosure and enable evaluation of AI during the procurement process. Following the two-part test outlined in the AI Bill of Rights, the proposed Federal AI Program would oversee the procurement of any “(1) automated systems that (2) have the potential to meaningfully impact the American public’s rights, opportunities, or access to critical resources or services.”
The goals of this program will be to (1) establish and enforce quality standards for AI used in government, (2) enforce rigorous equity standards for AI used in government, (3) establish transparency practices that enable public participation and political accountability, and (4) provide guidelines for AI program development in the private sector.
Recommendation 2. Produce a report to establish what data are needed in order to evaluate the equity of algorithmic technologies during procurement.
To support the AI Program’s operations, the OSTP National Science and Technology Council Subcommittee on Equitable Data should produce a report to establish guidelines for the collection and reporting of race data that balances three goals: (1) high-quality data for enforcing existing anti-discrimination law, (2) consistency in race categories to reduce administrative burdens and curb possible manipulation, and (3) prioritizing the needs of groups most affected by discrimination. The report should include opportunities and recommendations for integrating its findings into policy. To ensure the recommendations and standards are instituted, the President should direct the General Services Administration (GSA) or OMB to issue guidance and request that agencies document how they will ensure new standards are integrated into future procurement vehicles. The report could also suggest opportunities to update or amend the Federal Acquisition Regulations.
High-Quality Data
The new guidelines should make efforts to ensure the reliability of race data furnished during the procurement process. In particular:
- Self-identification should be used whenever possible to ascertain race. As of 2021, Food and Nutrition Service guidance recommends against the use of visual identification based on reliability, respect for respondents’ dignity, and feedback from Child and Adult Care Food Program) and Summer Food Service Program participants.
- The new guidelines should attempt to reduce missing data. People may be reluctant to share race information for many legitimate reasons, including uncertainty about how personal data will be used, fear of discrimination, and not identifying with predefined race categories. These concerns can severely impact data quality and should be addressed to the extent possible in the OMB guidelines. New York’s state health insurance marketplace saw a 20% increase in response rate for race by making several changes to the way they collect data. These changes included explaining how the data would be used and not allowing respondents to leave the question blank but instead allowing them to select “choose not to answer” or “don’t know.” Similarly, the Census Bureau found that a single combined race and ethnicity question improved data quality and consistency by reducing the rate of “some other race,” missing, and invalid responses as compared with two separate questions (one for race and one for ethnicity).
- The new guidelines should follow best practices established through rigorous research and feedback from a variety of stakeholders. In June 2022, the OMB announced a formal review process to revise Statistical Policy Directive No. 15: Standards for Maintaining, Collecting, and Presenting Federal Data on Race and Ethnicity. While this review process is intended for the revision of federal data requirements, its findings can help inform best practices for collection and reporting requirements for nongovernmental data as well.
Consistency in Data Reporting
Whenever possible and contextually appropriate, the guidelines for data reporting should align with the OMB guidelines for federal data reporting to reduce administrative burdens. However, the report may find that other data is needed that goes beyond the OMB guidelines for the evaluation of privately developed AI.
Prioritizing the Needs of Affected Groups
In their Toolkit for Centering Racial Equity Throughout Data Integration, the Actionable Intelligence for Social Policy group at the University of Pennsylvania identifies best practices for ensuring that data collection serves the groups most affected by discrimination. In particular, this toolkit emphasizes the need for strong privacy protections and stakeholder engagement. In their final report, the Subcommittee on Equitable Data should establish protocols to secure data and for carefully considered role-based access to it.
The final report should also engage community stakeholders in determining which data should be collected and establish a plan for ongoing review that engages with relevant stakeholders, prioritizing affected populations and racial equity advocacy groups. The report should evaluate the appropriate level of transparency in the AI procurement process, in particular, trade-offs between desired levels of transparency and privacy.
Conclusion
Under existing procurement law, the government cannot outsource “inherently governmental functions.” Yet key policy decisions are embedded within the design and implementation of algorithmic technology. Consequently, it is important that policymakers have the necessary resources and information throughout the acquisition and use of procured AI tools. A Federal Artificial Intelligence Program would provide expertise and authority within the federal government to assess these decisions during procurement and to monitor the use of AI in government. In particular, this would strengthen the Biden-Harris Administration’s ongoing efforts to advance racial equity. The proposed program can build on both long-standing and ongoing work within the federal government to develop best practices for data collection and reporting. These best practices will not only ensure that the public use of algorithms is governed by strong equity and transparency standards in the public sector but also provide a powerful avenue for shaping the development of AI in the private sector.
As people become less able to distinguish between what is real and what is fake, it has become easier than ever to be misled by synthetic content, whether by accident or with malicious intent. This makes advancing alternative countermeasures, such as technical solutions, more vital than ever before.
The next administration should establish a Participatory Technology Assessment unit to ensure federal S&T decisions benefit society.
AI is transforming how children learn and live, and policymakers, industry, and educators owe it to the next generation to set in place a responsible policy that embraces this new technology while at the same time ensuring all children’s well-being, privacy, and safety is respected.
A peer support option should be integrated into the 988 Suicide and Crisis Lifeline so that 988 service users can choose to connect with specialists based on a shared lived experience.