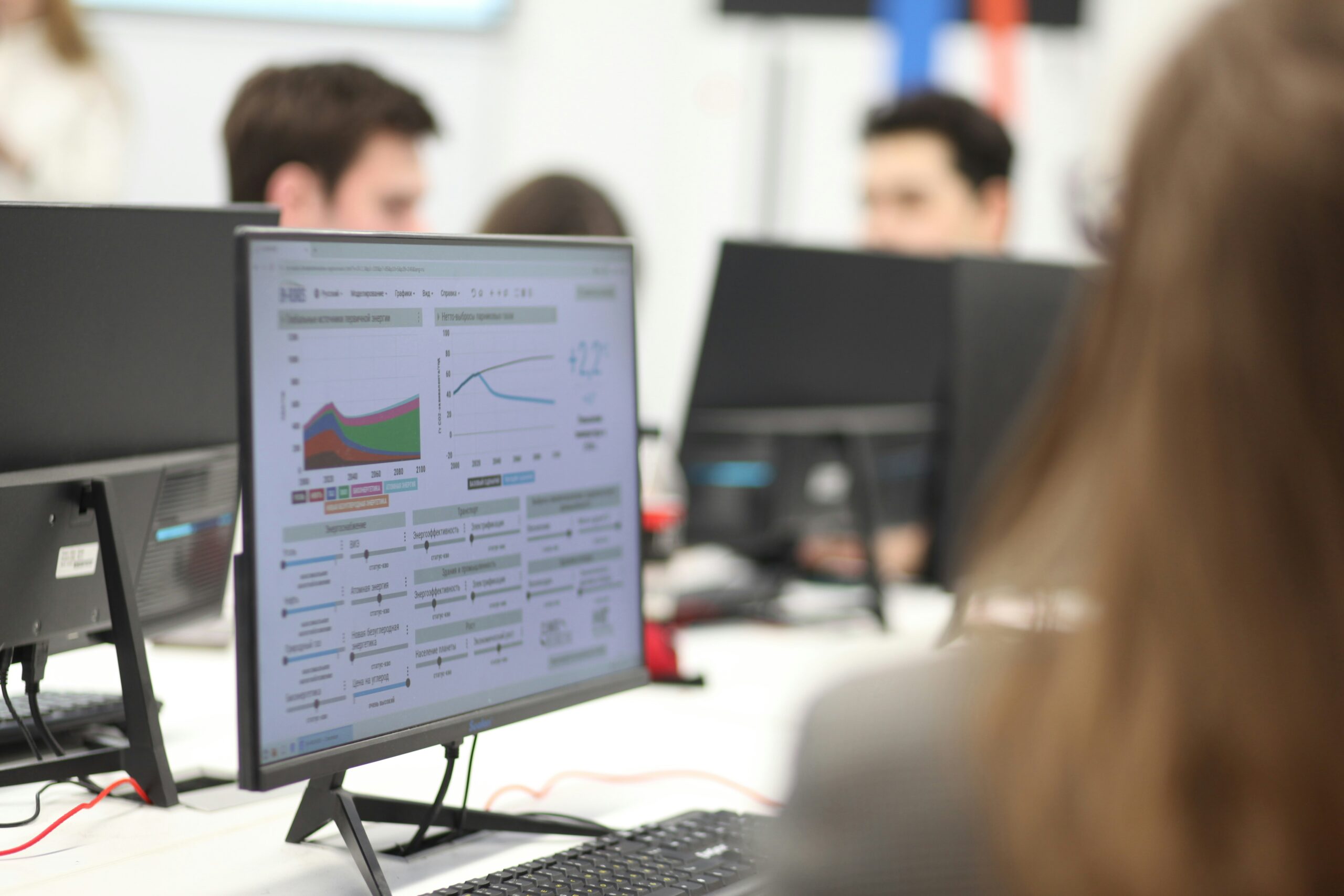
Kickstarting Collaborative, AI-Ready Datasets in the Life Sciences with Government-funded Projects
In the age of Artificial Intelligence (AI), large high-quality datasets are needed to move the field of life science forward. However, the research community lacks strategies to incentivize collaboration on high-quality data acquisition and sharing. The government should fund collaborative roadmapping, certification, collection, and sharing of large, high-quality datasets in life science. In such a system, nonprofit research organizations engage scientific communities to identify key types of data that would be valuable for building predictive models, and define quality control (QC) and open science standards for collection of that data. Projects are designed to develop automated methods for data collection, certify data providers, and facilitate data collection in consultation with researchers throughout various scientific communities. Hosting of the resulting open data is subsidized as well as protected by security measures. This system would provide crucial incentives for the life science community to identify and amass large, high-quality open datasets that will immensely benefit researchers.
Challenge and Opportunity
Life science has left the era of “one scientist, one problem.” It is becoming a field wherein collaboration on large-scale research initiatives is required to make meaningful scientific progress. A salient example is Alphafold2, a machine learning (ML) model that was the first to predict how a protein will fold with an accuracy meeting or exceeding experimental methods. Alphafold2 was trained on the Protein Data Bank (PDB), a public data repository containing standardized and highly curated results of >200,000 experiments collected over 50 years by thousands of researchers.
Though such a sustained effort is laudable, science need not wait another 50 years for the ‘next PDB’. If approached strategically and collaboratively, the data necessary to train ML models can be acquired more quickly, cheaply, and reproducibly than efforts like the PDB through careful problem specification and deliberate management. First, by leveraging organizations that are deeply connected with relevant experts, unified projects taking this approach can account for the needs of both the people producing the data and those consuming it. Second, by centralizing plans and accountability for data and metadata standards, these projects can enable rigorous and scalable multi-site data collection. Finally, by securely hosting the resulting open data, the projects can evaluate biosecurity risk and provide protected access to key scientific data and resources that might otherwise be siloed in industry. This approach is complementary to efforts that collate existing data, such as the Human Cell Atlas and UCSD Genome Browser, and satisfy the need for new data collection that adheres to QC and metadata standards.
In the past, mid-sized grants have allowed multi-investigator scientific centers like the recently funded Science and Technology Center for Quantitative Cell Biology (QCB, $30M in funding 2023) to explore many areas in a given field. Here, we outline how the government can expand upon such schemes to catalyze the creation of impactful open life science data. In the proposed system, supported projects would allow well-positioned nonprofit organizations to facilitate distributed, multidisciplinary collaborations that are necessary for assembling large, AI-ready datasets. This model would align research incentives and enable life science to create the ‘next PDBs’ faster and more cheaply than before.
Plan of Action
Existing initiatives have developed processes for creating open science data and successfully engaged the scientific community to identify targets for the ‘next PDB’ (e.g., Chan Zuckerberg Initiative’s Open Science program, Align’s Open Datasets Initiative). The process generally occurs in five steps:
- A multidisciplinary set of scientific leaders identify target datasets, assessing the scale of data required and the potential for standardization, and defining standards for data collection methods and corresponding QC metrics.
- Collaboratively develop and certify methods for data acquisition to de-risk the cost-per-datapoint and utility of the data.
- Data collection methods are onboarded at automation partner organizations, such as NSF BioFoundries and existing National Labs, and these automation partners are certified to meet the defined data collection standards and QC metrics.
- Scientists throughout the community, including those at universities and for-profit companies, can request data acquisition, which is coordinated, subsidized, and analyzed for quality.
- Data becomes publicly available and is hosted in a stable, robustly maintained database with biosecurity, cybersecurity, and privacy measures in perpetuity for researchers to access.
The U.S. Government should adapt this process for collaborative, AI-ready data collection in the life sciences by implementing the following recommendations:
Recommendation 1. An ARPA-like agency — or agency division — should launch a Collaborative, AI-Ready Datasets program to fund large-scale dataset identification and collection.
This program should be designed to award two types of grants:
- A medium-sized “phase 1” award of $1-$5m to fund new dataset identification and certification. To date, roadmapping dataset concepts (Steps 1-2 above) has been accomplished by small-scale projects of $1-$5M with a community-driven approach. Though selectively successful, these projects have not been as comprehensive or inclusive as they could otherwise be. Government funding could more sustainably and systematically permit iterative roadmapping and certification in areas of strategic importance.
- A large “phase 2” award of $10-$50m to fund the collection of previously identified datasets. Currently, there are no funding mechanisms designed to scale up acquisition (Steps #3-4 above) for dataset concepts that have been deemed valuable and derisked. To fill this gap, the government should leverage existing expertise and collaboration across the nonprofit research ecosystem by awarding grants of $10-50m for the coordination, acquisition, and release of mature dataset concepts. The Human Genome project is a good analogy, wherein a dataset concept was identified and collection was distributed amongst several facilities.
Recommendation 2. The Office of Management and Budget should direct the NSF and NIH to develop plans for funding academics and for-profits traunched on data deposition.
Once an open dataset is established, the government can advance the use and further development of that dataset by providing grants to academics that are traunched on data deposition. This approach would be in direct alignment with the government’s goals for supporting open, shared resources for AI innovation as laid out in section 5.2 of the Executive Order on the Safe, Secure, and Trustworthy Development and Use of Artificial Intelligence.
Agencies’ approaches to meeting this priority could vary. In one scenario, a policy or program could be established in which grantees would use a portion of the funds disbursed to them to pay for open data acquisition at a certified data provider. Analogous structures have enabled scientists to access other types of shared scientific infrastructure, such as the NSF’s ACCESS program. In the same way that ACCESS offers academics access to compute resources, it could be expanded to offer academic access to data acquisition resources at verified facilities. Offering grants in this way would incentivize the scientific community to interact with and expand upon open datasets, as well as encourage compliance through traunching.
Efforts to support use and development of open, certified datasets could also be incorporated into existing programs, including the National AI Research Resource, for which complementary programs could be developed to provide funding for standardized data acquisition and deposition. Similar ideas could also be incorporated into core programs within NSF and NIH, which already disburse funds after completion of annual progress reports. Such programs could mandate checks for data deposition in these reports.
Conclusion
Collaborative, AI-Ready datasets would catalyze progress in many areas of life science, but realizing them requires innovative government funding. By supporting coordinated projects that span dataset roadmapping, methods and standards development, partner certification, distributed collection, and secure release on a large scale, the government can coalesce stakeholders and deliver the next generation of powerful predictive models. To do so, it should combine small-sized, mid-sized, and traunched grants in unified initiatives that are orchestrated by nonprofit research organizations, which are uniquely positioned to execute these initiatives end-to-end. These initiatives should balance intellectual property protection and data availability, and thereby help deliver key datasets upon which new scientific insights depend.
This action-ready policy memo is part of Day One 2025 — our effort to bring forward bold policy ideas, grounded in science and evidence, that can tackle the country’s biggest challenges and bring us closer to the prosperous, equitable and safe future that we all hope for whoever takes office in 2025 and beyond.
PLEASE NOTE (February 2025): Since publication several government websites have been taken offline. We apologize for any broken links to once accessible public data.
Roadmapping dataset opportunities, which can take up to a year, requires convening experts across multiple disciplines, including experimental biology, automation, machine learning, and others. In collaboration, these experts assess both the feasibility and impact of opportunities, as well as necessary QC standards. Roadmapping culminates in determination of dataset value — whether it can be used to train meaningful new machine learning models.
To mitigate single-facility risk and promote site-to-site interoperability, data should be collected across multiple sites. To ensure that standards and organization holds across sites, planning and documentation should be centralized.
Automation partners will be evaluated according to the following criteria:
- Commitment to open science
- Rigor and consistency in methods and QC procedures
- Standardization of data and metadata ontologies
More specifically, certification will depend upon the abilities of partners to accommodate standardized ontologies, capture sufficient metadata, and reliably pass data QC checks. It will also require partners to have demonstrated a commitment to data reusability and replicability, and that they are willing to share methods and data in the open science ecosystem.
Today, scientists have no obligation to publish every piece of data they collect. In an Open Data paradigm, all data must eventually be shared. For some types of data, a short, optional embargo period would enable scientists to participate in open data efforts without compromising their ability to file patents or publish papers. For example, in protein engineering, the patentable product is the sequence of a designed protein, making immediate release of data untenable. An embargo period of one to two years is sufficient to alleviate this concern and may even hasten data sharing by linking it to a fixed length of time after collection, rather than to publication. Whether or not an embargo should be implemented and its length should be determined for each data type, and designed to encourage researchers to participate in acquisition of open data.
Biological data is a strategic resource and requires stewardship and curation to ensure it has maximum impact. Thus, data that is generated through the proposed system should be hosted by high-quality providers that adhere to biosecurity standards and enforce embargo periods. Appropriate biosecurity standards will be specific to different types of data, and should be formulated and periodically reevaluated by a multidisciplinary group of stakeholders. When access to certified, post-embargo data is requested, the same standards will apply as will export controls. In some instances, for some users, restricting access may be reasonable. For offering this suite of valuable services, hosting providers should be subsidized through reimbursements.
A shift toward more circular, transparent systems would not only reduce waste and increase efficiency, but also unlock new business models, strengthen supply chain resilience, and give consumers better, more reliable information about the products they choose.
By better harnessing the power of data, we can build a learning healthcare system where outcomes drive continuous improvement and where healthcare value leads the way.
In this unprecedented inflection point (and time of difficult disruption) for higher education, science funding, and agency structure, we have an opportunity to move beyond incremental changes and advocate for bold, new ideas that envision a future of the scientific research enterprise that looks very different from the current system.
Assigning persistent digital identifiers (Digital Object Identifiers, or DOIs) and using ORCIDs (Open Researcher and Contributor IDs) for key personnel to track outputs for research grants will improve the accountability and transparency of federal investments in research and reduce reporting burden.