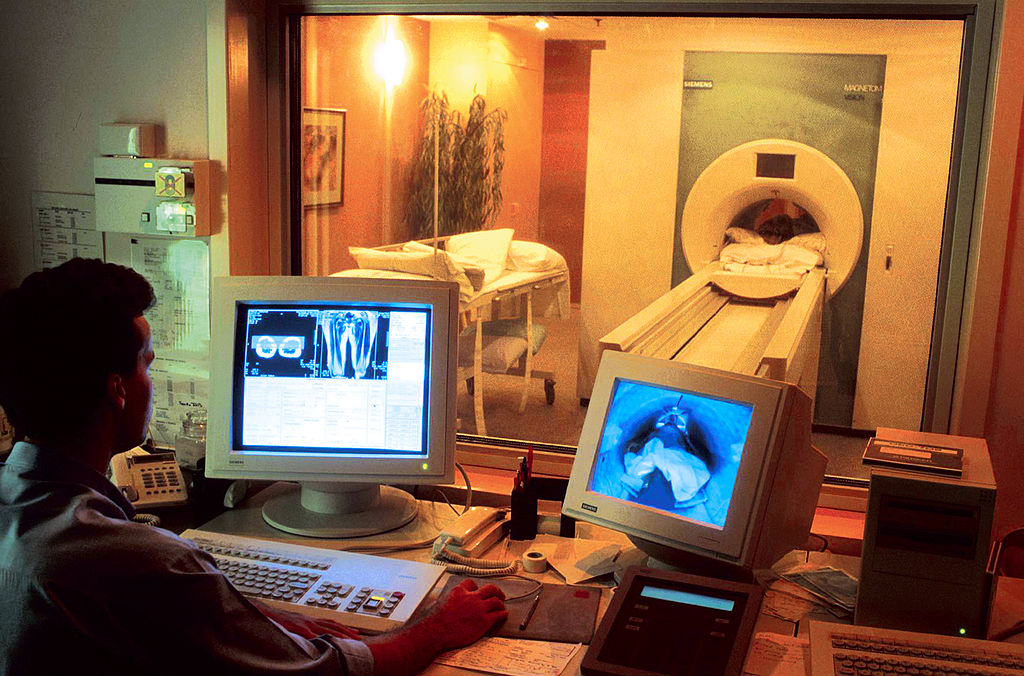
A Quantitative Imaging Infrastructure to Revolutionize AI-Enabled Precision Medicine
Medical imaging, a non-invasive method to detect and characterize disease, stands at a crossroads. With the explosive growth of artificial intelligence (AI), medical imaging offers extraordinary potential for precision medicine yet lacks adequate quality standards to safely and effectively fulfill the promise of AI. Now is the time to create a quantitative imaging (QI) infrastructure to drive the development of precise, data-driven solutions that enhance patient care, reduce costs, and unlock the full potential of AI in modern medicine.
Medical imaging plays a major role in healthcare delivery and is an essential tool in diagnosing numerous health issues and diseases (e.g., oncology, neurology, cardiology, hepatology, nephrology, pulmonary, and musculoskeletal). In 2023, there were more than 607 million imaging procedures in the United States and, per a 2021 study, $66 billion (8.9% of the U.S. healthcare budget) is spent on imaging.
Despite the importance and widespread use of medical imaging like magnetic resonance imaging (MRI), X-ray, ultrasound, computed tomography (CT), it is rarely standardized or quantitative. This leads to unnecessary costs due to repeat scans to achieve adequate image quality, and unharmonized and uncalibrated imaging datasets, which are often unsuitable for AI/machine learning (ML) applications. In the nascent yet exponentially expanding world of AI in medical imaging, a well-defined standards and metrology framework is required to establish robust imaging datasets for true precision medicine, thereby improving patient outcomes and reducing spiraling healthcare costs.
Challenge and Opportunity
The U.S. spends more on healthcare than any other high-income country yet performs worse on measures of health and healthcare. Research has demonstrated that medical imaging could help save money for the health system with every $1 spent on inpatient imaging resulting in approximately $3 total savings in healthcare delivered. However, to generate healthcare savings and improve outcomes, rigorous quality assurance (QA)/quality control(QC) standards are required for true QI and data integrity.
Today, medical imaging suffers two shortcomings inhibiting AI:
- Lack of standardization: Findings or measurements differ based on numerous factors such as system manufacturer, software version, or imaging protocol.
- A reliance on qualitative (subjective) measurements despite the technological capabilities to perform quantitative (objective) measurements.
Both result in variability impacting assessments and reducing the generalizability of, and confidence in, imaging test results and compromise data quality required for AI applications.
The growing field of QI, however, provides accurate and precise (repeatable and reproducible) quantitative-image-based metrics that are consistent across different imaging devices and over time. This benefits patients (fewer scans, biopsies), doctors, researchers, insurers, and hospitals and enables safe, viable development and use of AI/ML tools.
Quantitative imaging metrology and standards are required as a foundation for clinically relevant and useful QI. A change from “this might be a stage 3 tumor” to “this is a stage 3 tumor” will affect how oncologists can treat a patient. Quantitative imaging also has the potential to remove the need for an invasive biopsy and, in some cases, provide valuable and objective information before even the most expert radiologist’s qualitative assessment. This can mean the difference between taking a nonresponding patient off a toxic chemotherapeutic agent or recognizing a strong positive treatment response before a traditional assessment.
Plan of Action
The incoming administration should develop and fund a Quantitative Imaging Infrastructure to provide medical imaging with a foundation of rigorous QA/QC methodologies, metrology, and standards—all essential for AI applications.
Coordinated leadership is essential to achieve such standardization. Numerous medical, radiological, and standards organizations support and recognize the power of QI and the need for rigorous QA/QC and metrology standards (see FAQs). Currently, no single U.S. organization has the oversight capabilities, breadth, mandate, or funding to effectively implement and regulate QI or a standards and metrology framework.
As set forth below, earlier successful approaches to quality and standards in other realms offer inspiration and guidance for medical imaging and this proposal:
- Clinical Laboratory Improvement Amendments of 1988 (CLIA)
- Mammographic Quality Standards Act of 1992 (MQSA)
- Centers for Disease Control’s (CDC) Clinical Standardization Program (CSP)
Recommendation 1. Create a Medical Metrology Center of Excellence for Quantitative Imaging.
Establishing a QI infrastructure would transform all medical imaging modalities and clinical applications. Our recommendation is that an autonomous organization be formed, possibly appended to existing infrastructure, with the mandate and responsibility to develop and operationally support the implementation of quantitative QA/QC methodologies for medical imaging in the age of AI. Specifically this fully integrated QI Metrology Center of Excellence would need federal funding to:
- Define a metrology standards framework, in accord with international metrology standards;
- Implement a standards program to oversee:
- approach to sequence definition
- protocol development
- QA/QC methodology
- QIB profiles
- guidance and standards regarding “digital twins”
- applications related to radiomics
- clinical practice and operations
- vendor-neutral applications (where application is agnostic to manufacturer/machine)
- AI/ML validation
- data standardization
- training and continuing education for doctors and technologists.
Once implemented, the Center could focus on self-sustaining approaches such as testing and services provided for a fee to users.
Similar programs and efforts have resulted in funding (public and private) ranging from $90 million (e.g., Pathogen Genomics Centers of Excellence Network) to $150 million (e.g., Biology and Machine Learning – Broad Institute). Importantly, implementing a QI Center of Excellence would augment and complement federal funding currently being awarded through ARPA-H and the Cancer Moonshot, as neither have an overarching imaging framework for intercomparability between projects.
While this list is by no means exhaustive, any organization would need input and buy-in from:
- National Institutes of Health (NIH) (and related organizations such as National Institute of Biomedical Imaging and Bioengineering (NIBIB))
- National Institute of Standards and Technology (NIST)
- Centers for Disease Control (CDC)
- Centers for Medicare and Medicaid Services (CMS)
- U.S. Department of Defense (DoD)
- Radiological Society of North America (RSNA)
- American Association of Physicists in Medicine (AAPM)
- International Society for Magnetic Resonance in Medicine (ISMRM)
- Society of Nuclear Medicine & Molecular Imaging (SNMMI)
- American Institute of Ultrasound in Medicine (AIUM)
- American College of Radiology (ACR)
- ARPA-H
- HHS
International organizations also have relevant programs, guidance, and insight, including:
- European Society of Radiology (ESR)
- European Association of National Metrology Institutes (EURAMET)
- European Society of Breast Imaging (EUSOBI)
- European Society of Radiology’s Imaging Biomarkers Alliance (EIBALL)
- European Association of Nuclear Medicine (EANM)
- Institute of Physics and Engineering in Medicine (IPEM)
- Japan Quantitative Imaging Biomarker Alliance (JQIBA)
- National Physical Laboratory (NPL)
- National Imaging Facility (NIF)
Recommendation 2. Implement legislation and/or regulation providing incentives for standardizing all medical imaging.
The variability of current standard-of-care medical imaging (whether acquired across different sites or over a period of time) creates different “appearances.” This variability can result in different diagnoses or treatment response measurements, even though the underlying pathology for a given patient is unchanged. Real-world examples abound, such as one study that found 10 MRI studies over three weeks resulted in 10 different reports. This heterogeneity of imaging data can lead to a variable assessment by a radiologist (inter-reader variability), AI interpretation (“garbage-in-garbage-out”), or treatment recommendations from clinicians. Efforts are underway to develop “vendor-neutral sequences” for MRI and other methods (such as quantitative ground truth references, metrological standards, etc.) to improve data quality and ensure intercomparable results across vendors and over time.
To do so, however, requires coordination by all original equipment manufacturers (OEMs) or legislation to incentivize standards. The 1992 Mammography Quality Standards Act (MQSA) provides an analogous roadmap. MQSA’s passage implemented rigorous standards for mammography, and similar legislation focused on quality assurance of quantitative imaging, reducing or eliminating machine bias, and improved standards would reduce the need for repeat scans and improve datasets.
In addition, regulatory initiatives could also advance quantitative imaging. For example, in 2022, the Food and Drug Administration (FDA) issued Technical Performance Assessment of Quantitative Imaging in Radiological Device Premarket Submissions, recognizing the importance of ground truth references with respect to quantitative imaging algorithms. A mandate requiring the use of ground truth reference standards would change standard practice and be a significant step to improving quantitative imaging algorithms.
Recommendation 3. Ensure a funded QA component for federally funded research using medical imaging.
All federal medical research grant or contract awards should contain QA funds and require rigorous QA methodologies. The quality system aspects of such grants would fit the scope of the project; for example, a multiyear, multisite project would have a different scope than single-site, short-term work.
NIH spends the majority of its $48 billion budget on medical research. Projects include multiyear, multisite studies with imaging components. While NIH does have guidelines on research and grant funding (e.g., Guidance: Rigor and Reproducibility in Grant Applications), this guidance falls short in multisite, multiyear projects where clinical scanning is a component of the study.
To the extent NIH-funded programs fail to include ground truth references where clinical imaging is used, the resulting data cannot be accurately compared over time or across sites. Lack of standardization and failure to require rigorous and reproducible methods compromises the long-term use and applicability of the funded research.
By contrast, implementation of rigorous standards regarding QA/QC, standardization, etc. improve research in terms of reproducibility, repeatability, and ultimate outcomes. Further, confidence in imaging datasets enables the use of existing and qualified research in future NIH-funded work and/or imaging dataset repositories that are being leveraged for AI research and development, such as the Medical Imaging and Resource Center (MIDRC). (See also: Open Access Medical Imaging Repositories.)
Recommendation 4. Implement a Clinical Standardization Program (CSP) for quantitative imaging.
While not focused on medical imaging, the CDC’s CSPs have been incredibly successful and “improve the accuracy and reliability of laboratory tests for key chronic biomarkers, such as those for diabetes, cancer, and kidney, bone, heart, and thyroid disease.” By way of example, the CSP for Lipids Standardization has “resulted in an estimated benefit of $338M at a cost of $1.7M.” Given the breadth of use of medical imaging, implementing such a program for QI would have even greater benefits.
Although many people think of the images derived from clinical imaging scans as “pictures,” the pixel and voxel numbers that make up those images contain meaningful biological information. The objective biological information that is extracted by QI is conceptually the same as the biological information that is extracted from tissue or fluids by laboratory assay techniques. Thus, quantitative imaging biomarkers can be understood to be “imaging assays.”
The QA/QC standards that have been developed for laboratory assays can and should be adapted to quantitative imaging. (See also regulations, history, and standards of the Clinical Laboratory Improvement Amendment (CLIA) ensuring quality laboratory testing.)
Recommendation 5. Implement an accreditation program and reimbursement code for quantitative imaging starting with qMRI.
The American College of Radiology currently provides basic accreditation for clinical imaging scanners and concomitant QA for MRI. These requirements, however, have been in place for nearly two decades and do not address many newer quantitative aspects (e.g., relaxometry and ADC) nor account for the impact of image variability in effective AI use. Several new Current Procedural Terminology (CPT) codes have been recently adopted focused on quantitative imaging. An expansion of reimbursement codes for quantitative imaging could drive more widespread clinical adoption.
QI is analogous to the quantitative blood, serum and tissue assays done in clinical laboratories, subject to CLIA, one of the most impactful programs for improving the accuracy and reliability of laboratory assays. This CMS-administered mandatory accreditation program promulgates quality standards for all laboratory testing to ensure the accuracy, reliability, and timeliness of patient test results, regardless of where the test was performed.
Conclusion
These five proposals provide a range of actionable opportunities to modernize the approach to medical imaging to fit the age of AI, data integrity, and precision patient health. A comprehensive, metrology-based quantitative imaging infrastructure will transform medical imaging through:
- Improved clinical care due to an objective, quantitative understanding of a disease state(s)
- Accurate quantitative image datasets for (1) analysis and implementation to establish new imaging and composite biomarkers; and (2) training, evaluating/validating, and optimizing high-accuracy AI/ML applications;
- Reduced healthcare costs due to higher quality (fewer repeat) scans, more accurate diagnoses, and fewer invasive biopsies; and
- Greater accessibility of medical imaging with new to market technologies (e.g., low-field and/or organ-specific MRI) tied to quantitative imaging biomarkers.
With robust metrological underpinnings and a funded infrastructure, the medical community will have confidence in the QI data, unlocking powerful health insights only imaginable until now.
This action-ready policy memo is part of Day One 2025 — our effort to bring forward bold policy ideas, grounded in science and evidence, that can tackle the country’s biggest challenges and bring us closer to the prosperous, equitable and safe future that we all hope for whoever takes office in 2025 and beyond.
Yes. Using MRI as an example, numerous articles, papers, and publications acknowledge qMRI variability in scanner output can vary between manufacturers, over time, and after software or hardware maintenance or upgrades.
With in-vivo metrology, measurements are performed on the “body of living subjects (human or animal) without taking the sample out of the living subject (biopsy).” True in-vivo metrology will enable the diagnosis or understanding of tissue state before a radiologist’s visual inspection. Such measurement capabilities are objective, in contrast to the subjective, qualitative interpretation by a human observer. In-vivo metrology will enhance and support the practice of radiology in addition to reducing unnecessary procedures and associated costs.
Current digital imaging modalities provide the ability to measure a variety of biological and physical quantities with accuracy and reliability, e.g., tissue characterization, physical dimensions, temperature, body mass components, etc. However, consensus standards and corresponding certification or accreditation programs are essential to bring the benefits of these objective QI parameters to patient care. The CSP follows this paradigm as does the earlier CLIA, both of which have been instrumental in improving the accuracy and consistency of laboratory assays. This proposal aims to bring the same rigor to immediately improve the quality, safety and effectiveness of medical imaging in clinical care and to advance the input data needed to create, as well as safely and responsibly use, robust imaging AI tools for the benefit of all patients.
Phantoms are specialized test objects used as ground truth references for quantitative imaging and analysis. NIST plays a central role in measuring and testing solutions for phantoms. Phantoms are used in ultrasound, CT, MRI, and other imaging modalities for routine QA/QC and machine testing. Phantoms are key to harmonizing and standardizing data and improve data quality needed for AI applications.
Precision medicine is a popular term with many definitions/approaches applying to genetics, oncology, pharmacogenetics, oncology, etc. (See, e.g., NCI, FDA, NIH, National Human Genome Research Institute.) Generally, precision (or personalized) medicine focuses on the idea that treatment can be individualized (rather than generalized). While there have been exciting advances in personalized medicine (such as gene testing), the variability of medical imaging is a major limitation in realizing the full potential of precision medicine. Recognizing that medical imaging is a fundamental measurement tool from diagnosis through measurement of treatment response and toxicity assessment, this proposal aims to transition medical imaging practices to quantitative imaging to enable the realization of precision medicine and timely personalized approaches to patient care.
Radiologists need accurate and reliable data to make informed decisions. Improving standardization and advancing QI metrology will support radiologists by improving data quality. To the extent radiologists are relying on AI platforms, data quality is even more essential when it is used to drive AI applications, as the outputs of AI models rely on sound acquisition methods and accurate quantitative datasets.
Standardized data also helps patients by reducing the need for repeat scans, which saves time, money, and unnecessary radiation (for ionizing methods).
Yes! Using MRI as an example, qMRI can advance and support efforts to make MRI more accessible. Historically, MRI systems cost millions of dollars and are located in high-resource hospital settings. Numerous healthcare and policy providers are making efforts to create “accessible” MRI systems, which include portable systems at lower field strengths and to address organ-specific diseases. New low-field systems can reach patient populations historically absent from high-resource hospital settings. However, robust and reliable quantitative data are needed to ensure data collected in rural, nonhospital settings, or in Low and Middle Income Countries, can be objectively compared to data from high-resource hospital settings.
Further, accessibility can be limited by a lack of local expertise. AI could help fill the gap.
However, a QI infrastructure is needed for safe and responsible use of AI tools, ensuring adequate quality of the input imaging data.
The I-SPY 2 Clinical Breast Trials provide a prime example of the need for rigorous QA and scanner standardization. The I-SPY 2 trial is a novel approach to breast cancer treatment that closely monitors treatment response to neoadjuvant therapy. If there is no immediate/early response, the patient is switched to a different drug. MR imaging is acquired at various points during the treatment to determine the initial tumor size and functional characteristics and then to measure any tumor shrinkage/response over the course of treatment. One quantitative MRI tumor characteristic that has shown promise for evaluation of treatment response and is being evaluated in the trial is ADC, a measure of tissue water mobility which is calculated from diffusion-weighted imaging. It is essential for the trial that MR results can be compared over time as well as across sites. To truly know whether a patient is responding, the radiologist must have confidence that any change in the MR reading or measurement is due to a physiological change and not due to a scanner change such as drift, gradient failure, or software upgrade.
For the I-SPY 2 trial, breast MRI phantoms and a standardized imaging protocol are used to test and harmonize scanner performance and evaluate measurement bias over time and across sites. This approach then provides clear data/information on image quality and quantitative measurement (e.g., ADC) for both the trial (comparing data from all sites is possible) as well as for the individual imaging sites.
Nonstandardized imaging results in variation that requires orders of magnitude more data to train an algorithm. More importantly, without reliable and standardized datasets, AI algorithms drift, resulting in degradation of both protocols and performance. Creating and supporting a standards-based framework for medical imaging will mitigate these issues as well as lead to:
- Integrated and coordinated system for establishing QIBs, screening, and treatment planning.
- Cost savings: Standardizing data and implementing quantitative results in superior datasets for clinical use or as part of large datasets for AI applications. Clinical Standardization Programs have focused on standardizing tests and have been shown to save “millions in health care costs.”
- Better health outcomes: Standardization reduces reader error and enables new AI applications to support current radiology practices.
- Support for radiologists’ diagnoses.
- Fewer incorrect diagnoses (false positives and false negatives).
- Elimination of millions of unnecessary invasive biopsies.
- Fewer repeat scans.
- Robust and reliable datasets for AI applications (e.g., preventing model collapse).
It benefits federal organizations such as the National Institutes of Health, Centers for Medicare and Medicaid Services, and Veterans Affairs as well as the private and nonprofit sectors (insurers, hospital systems, pharmaceutical, imaging software, and AI companies). The ultimate beneficiary, however, is the patient, who will receive an objective, reliable quantitative measure of their health—relevant for a point-in-time assessment as well as longitudinal follow-up.
Possible pushback from such a program may come from: (1) radiologists who are unfamiliar with the power of quantitative imaging for precision health and/or the importance and incredible benefits of clean datasets for AI applications; or (2) manufacturers (OEMs) who aim to improve output through differentiation and are focused on customers who are more interested in their qualitative practice.
Radiology practices: Radiology practices’ main objective is to provide the most accurate diagnosis possible in the least amount of time, as cost-effectively as possible. Standardization and calibration are generally perceived as requiring additional time and increased costs; however, these perceptions are often not true, and the variability in imaging introduces more time consumption and challenges. The existing standard of care relies on qualitative assessments of medical images.
While excellent for understanding a patient’s health at a single point in time (though even in these cases subtle abnormalities can be missed), longitudinal monitoring is impossible without robust metrological standards for reproducibility and quantitative assessment of tissue health. While a move from qualitative to quantitative imaging may require additional education, understanding, and time, such an infrastructure will provide radiologists with improved capabilities and an opportunity to supplement and augment the existing standard of care.
Further, AI is undeniably being incorporated into numerous radiology applications, which will require accurate and reliable datasets. As such, it will be important to work with radiology practices to demonstrate a move to standardization will, ultimately, reduce time and increase the ability to accurately diagnose patients.
OEMs: Imaging device manufacturers work diligently to improve their outputs. To the extent differentiation is seen as a business advantage, a move toward vendor-neutral and scanner-agnostic metrics may initially be met with resistance. However, all OEMs are investing resources to improve AI applications and patient health. All benefit from input data that is standard and robust and provides enough transparency to ensure FAIR data principles (findability, accessibility, interoperability, and reusability).
OEMs have plenty of areas for differentiation including improving the patient experience and shortening scan times. We believe OEMs, as part of their move to embrace AI, will find clear metrology and standards-based framework a positive for their own business and the field as a whole.
The first step is to convene a meeting of leaders in the field within three months to establish priorities and timelines for successful implementation and adoption of a Center of Excellence. Any Center must be well-funded with experienced leadership and will need the support and collaboration across the relevant agencies and organizations.
There are numerous potential pilots. The key is to identify an actionable study where results could be achieved within a reasonable time. For example, a pilot study to demonstrate the importance of quantitative MRI and sound datasets for AI could be implemented at the Veterans Administration hospital system. This study could focus on quantifying benefits from standardization and implementation of quantitative diffusion MRI, an “imaging biopsy” modality as well as mirror advances and knowledge identified in the existing I-SPY 2 clinical breast trials.
The timing is right for three reasons: (1) quantitative imaging is doable; (2) AI is upon us; and (3) there is a desire and need to reduce healthcare costs and improve patient outcomes.
There is widespread agreement that QI methodologies have enormous potential benefits, and many government agencies and industry organizations have acknowledged this. Unfortunately, there has been no unifying entity with sufficient resources and professional leadership to coordinate and focus these efforts. Many organizations have been organized and run by volunteers. Finally, some previously funded efforts to support quantitative imaging (e.g., QIN and QIBA) have recently lost dedicated funding.
With rapid advances in technology, including the promise of AI, there is new and shared motivation across communities to revise our approach to data generation and collection at-large—focused on standardization, precision, and transparency. By leveraging the existing widespread support, along with dedicated resources for implementation and enforcement, this proposal will drive the necessary change.
Yes. Human health has no geographical boundaries, so a global approach to quantitative imaging would benefit all. QI is being studied, implemented, and adopted globally.
However, as is the case in the U.S., while standards have been proposed, there is no international body to govern the implementation, coordination, and maturation of this process. The initiatives put forth here could provide a roadmap for global collaboration (ever-more important with AI) and standards that would speed up development and implementation both in the U.S. and abroad.
Some of the organizations that recognize the urgent need for QI standards are National Institute of Standards and Technology (quantitative MR project); National Cancer Institute’s Quantitative Imaging Network; Radiological Society of North America (through its Quantitative Imaging Committee); Quantitative Imaging Biomarker Alliance; International Society of Magnetic Resonance in Medicine; Object Management Group; American Association of Physicists in Medicine; National Institutes of Health; Food and Drug Administration (“guidance” – ground truth references for quantitative imaging algorithms).
As people become less able to distinguish between what is real and what is fake, it has become easier than ever to be misled by synthetic content, whether by accident or with malicious intent. This makes advancing alternative countermeasures, such as technical solutions, more vital than ever before.
The next administration should establish a Participatory Technology Assessment unit to ensure federal S&T decisions benefit society.
AI is transforming how children learn and live, and policymakers, industry, and educators owe it to the next generation to set in place a responsible policy that embraces this new technology while at the same time ensuring all children’s well-being, privacy, and safety is respected.
A peer support option should be integrated into the 988 Suicide and Crisis Lifeline so that 988 service users can choose to connect with specialists based on a shared lived experience.