Addressing Online Harassment and Abuse through a Collaborative Digital Hub
Efforts to monitor and combat online harassment have fallen short due to a lack of cooperation and information-sharing across stakeholders, disproportionately hurting women, people of color, and LGBTQ+ individuals. We propose that the White House Task Force to Address Online Harassment and Abuse convene government actors, civil society organizations, and industry representatives to create an Anti-Online Harassment (AOH) Hub to improve and standardize responses to online harassment and to provide evidence-based recommendations to the Task Force. This Hub will include a data-collection mechanism for research and analysis while also connecting survivors with social media companies, law enforcement, legal support, and other necessary resources. This approach will open pathways for survivors to better access the support and recourse they need and also create standardized record-keeping mechanisms that can provide evidence for and enable long-term policy change.
Challenge and Opportunity
The online world is rife with hate and harassment, disproportionately hurting women, people of color, and LGBTQ+ individuals. A research study by Pew indicated that 47% of women were harassed online for their gender compared to 18% of men, while 54% of Black or Hispanic internet users faced race-based harassment online compared to 17% of White users. Seven in 10 LGBTQ+ adults have experienced online harassment, and 51% faced even more severe forms of abuse. Meanwhile, existing measures to combat online harassment continue to fall short, leaving victims with limited means for recourse or protection.
Numerous factors contribute to these shortcomings. Social media companies are opaque, and when survivors turn to platforms for assistance, they are often met with automated responses and few means to appeal or even contact a human representative who could provide more personalized assistance. Many survivors of harassment face threats that escalate from online to real life, leading them to seek help from law enforcement. While most states have laws against cyberbullying, law enforcement agencies are often ill-trained and ill-equipped to navigate the complex web of laws involved and the available processes through which they could provide assistance. And while there are nongovernmental organizations and companies that develop tools and provide services for survivors of online harassment, the onus continues to lie primarily on the survivor to reach out and navigate what is often both an overwhelming and a traumatic landscape of needs. Although resources exist, finding the correct organizations and reaching out can be difficult and time-consuming. Most often, the burden remains on the victims to manage and monitor their own online presence and safety.
On a larger, systemic scale, the lack of available data to quantitatively analyze the scope and extent of online harassment hinders the ability of researchers and interested stakeholders to develop effective, long-term solutions and to hold social media companies accountable. Lack of large-scale, cross-sector and cross-platform data further hinders efforts to map out the exact scale of the issue, as well as provide evidence-based arguments for changes in policy. As the landscape of online abuse is ever changing and evolving, up-to-date information about the lexicons and phrases that are used in attacks also change.
Forming the AOH Hub will improve the collection and monitoring of online harassment while preserving victims’ privacy; this data can also be used to develop future interventions and regulations. In addition, the Hub will streamline the process of receiving aid for those targeted by online harassment.
Plan of Action
Aim of proposal
The White House Task Force to Address Online Harassment and Abuse should form an Anti-Online Harassment Hub to monitor and combat online harassment. This Hub will center around a database that collects and indexes incidents of online harassment and abuse from technology companies’ self-reporting, through connections civil society groups have with survivors of harassment, and from reporting conducted by the general public and by targets of online abuse. Civil society actors that have conducted past work in providing resources and monitoring harassment incidents, ranging from academics to researchers to nonprofits, will run the AOH Hub in consortium as a steering committee. There are two aims for the creation of this hub.
First, the AOH Hub can promote collaboration within and across sectors, forging bonds among government, the technology sector, civil society, and the general public. This collaboration enables the centralization of connections and resources and brings together diverse resources and expertise to address a multifaceted problem.
Second, the Hub will include a data collection mechanism that can be used to create a record for policy and other structural reform. At present, the lack of data limits the ability of external actors to evaluate whether social media companies have worked adequately to combat harmful behavior on their platforms. An external data collection mechanism enables further accountability and can build the record for Congress and the Federal Trade Commission to take action where social media companies fall short. The allocated federal funding will be used to (1) facilitate the initial convening of experts across government departments and nonprofit organizations; (2) provide support for the engineering structure required to launch the Hub and database; (3) support the steering committee of civil society actors that will maintain this service; and (4) create training units for law enforcement officials on supporting survivors of online harassment.
Recommendation 1. Create a committee for governmental departments.
Survivors of online harassment struggle to find recourse, failed by legal technicalities in patchworks of laws across states and untrained law enforcement. The root of the problem is an outdated understanding of the implications and scale of online harassment and a lack of coordination across branches of government on who should handle online harassment and how to properly address such occurrences. A crucial first step is to examine and address these existing gaps. The Task Force should form a long-term committee of members across governmental departments whose work pertains to online harassment. This would include one person from each of the following organizations, nominated by senior staff:
- Department of Homeland Security
- Department of Justice
- Federal Bureau of Investigation
- Department of Health and Human Services
- Office on Violence Against Women
- Federal Trade Commission
This committee will be responsible for outlining fallibilities in the existing system and detailing the kind of information needed to fill those gaps. Then, the committee will outline a framework clearly establishing the recourse options available to harassment victims and the kinds of data collection required to prove a case of harassment. The framework should be completed within the first 6 months after the committee has been convened. After that, the committee will convene twice a year to determine how well the framework is working and, in the long term, implement reforms and updates to current laws and processes to increase the success rates of victims seeking assistance from governmental agencies.
Recommendation 2: Establish a committee for civil society organizations.
The Task Force shall also convene civil society organizations to help form the AOH Hub steering committee and gather a centralized set of resources. Victims will be able to access a centralized hotline and information page, and Hub personnel will then triage reports and direct victims to resources most helpful for their particular situation. This should reduce the burden on those who are targets of harassment campaigns to find the appropriate organizations that can help address their issues by matching incidents to appropriate resources.
To create the AOH Hub, members of the Task Force can map out civil society stakeholders in the space and solicit applications to achieve comprehensive and equitable representation across sectors. Relevant organizations include organizations/actors working on (but not limited to):
- Combating domestic violence and intimate partner violence
- Addressing technology-facilitated gender based violence (TF-GBV)
- Developing online tools for survivors of harassment to protect themselves
- Conducting policy work to improve policies on harassment
- Providing mental health support for survivors of harassment
- Servicing pro bono or other forms of legal assistance for survivors of harassment
- Connecting tech company representatives with survivors of harassment
- Researching methods to address online harassment and abuse
The Task Force will convene an initial meeting, during which core members will be selected to create an advisory board, act as a liaison across members, and conduct hiring for the personnel needed to redirect victims to needed services. Other secondary members will take part in collaboratively mapping out and sharing available resources, in order to understand where efforts overlap and complement each other. These resources will be consolidated, reviewed, and published as a public database of resources within a year of the group’s formation.
For secondary members, their primary obligation will be to connect with victims who have been recommended to their services. Core members, meanwhile, will meet quarterly to evaluate gaps in services and assistance provided and examine what more needs to be done to continue growing the robustness of services and aid provided.
Recommendation 3: Convene committee for industry.
After its formation, the AOH steering committee will be responsible for conducting outreach with industry partners to identify a designated team from each company best equipped to address issues pertaining to online abuse. After the first year of formation, the industry committee will provide operational reporting on existing measures within each company to address online harassment and examine gaps in existing approaches. Committee dialogue should also aim to create standardized responses to harassment incidents across industry actors and understandings of how to best uphold community guidelines and terms of service. This reporting will also create a framework for standardized best practices for data collection, in terms of the information collected on flagged cases of online harassment.
On a day-to-day basis, industry teams will be available resources for the hub, and cases can be redirected to these teams to provide person-to-person support for handling cases of harassment that require a personalized level of assistance and scale. This committee will aim to increase transparency regarding the reporting process and improve equity in responses to online harassment.
Recommendation 4: Gather committees to provide long-term recommendations for policy change.
On a yearly basis, representatives across the three committees will convene and share insights on existing measures and takeaways. These recommendations will be given to the Task Force and other relevant stakeholders, as well as be accessible by the general public. Three years after the formation of these committees, the groups will publish a report centralizing feedback and takeaway from all committees, and provide recommendations of improvement for moving forward.
Recommendation 5: Create a data-collection mechanism and standard reporting procedures.
The database will be run and maintained by the steering committee with support from the U.S. Digital Service, with funding from the Task Force for its initial development. The data collection mechanism will be informed by the frameworks provided by the committees that compose the Hub to create a trauma-informed and victim-centered framework surrounding the collection, protection, and use of the contained data. The database will be periodically reviewed by the steering committee to ensure that the nature and scope of data collection is necessary and respects the privacy of those whose data it contains. Stakeholders can use this data to analyze and provide evidence of the scale and cross-cutting nature of online harassment and abuse. The database would be populated using a standardized reporting form containing (1) details of the incident; (2) basic demographic data of the victim; (3) platform/means through which the incident occurred; (4) whether it is part of a larger organized campaign; (5) current status of the incident (e.g., whether a message was taken down, an account was suspended, the report is still ongoing); (6) categorization within existing proposed taxonomies indicating the type of abuse. This standardization of data collection would allow advocates to build cases regarding structured campaigns of abuse with well-documented evidence, and the database will archive and collect data across incidents to ensure accountability even if the originals are lost or removed.
The reporting form will be available online through the AOH Hub. Anyone with evidence of online harassment will be able to contribute to the database, including but not limited to victims of abuse, bystanders, researchers, civil society organizations, and platforms. To protect the privacy and safety of targets of harassment, this data will not be publicly available. Access will be limited to: (1) members of the Hub and its committees; (2) affiliates of the aforementioned members; (3) researchers and other stakeholders, after submitting an application stating reasons to access the data, plans for data use, and plans for maintaining data privacy and security. Published reports using data from this database will be nonidentifiable, such as with statistics being published in aggregate, and not be able to be linked back to individuals without express consent.
This database is intended to provide data to inform the committees in and partners of the Hub of the existing landscape of technology-facilitated abuse and violence. The large-scale, cross-domain, and cross-platform nature of the data collected will allow for better understanding and analysis of trends that may not be clear when analyzing specific incidents, and provide evidence regarding disproportionate harms to particular communities (such as women, people of color, LGBTQ+ individuals). Resources permitting, the Hub could also survey those who have been impacted by online abuse and harassment to better understand the needs of victims and survivors. This data aims to provide evidence for and help inform the recommendations made from the committees to the Task Force for policy change and further interventions.
Recommendation 6: Improve law enforcement support.
Law enforcement is often ill-equipped to handle issues of technology-facilitated abuse and violence. To address this, Congress should allocate funding for the Hub to create training materials for law enforcement nationwide. The developed materials will be added to training manuals and modules nationwide, to ensure that 911 operators and officers are aware of how to handle cases of online harassment and how state and federal law can apply to a range of scenarios. As part of the training, operators will also be notified to add records of 911 calls regarding online harassment to the Hub database, with the survivor’s consent.
Conclusion
As technology-facilitated violence and abuse proliferates, we call for funding to create a steering committee in which experts and stakeholders from civil society, academia, industry, and government can collaborate on monitoring and regulating online harassment across sectors and incidents. The resulting Anti-Online Harassment Hub would maintain a data-collection mechanism accessible to researchers to better understand online harassment as well as provide accountability for social media platforms to address the issue. Finally, the Hub would provide accessible resources for targets of harassment in a fashion that would reduce the burden on these individuals. Implementing these measures would create a safer online space where survivors are able to easily access the support they need and establish a basis for evidence-based, longer-term policy change.
Platform policies on hate and harassment differ in the redress and resolution they offer. Twitter’s proactive removal of racist abuse toward members of the England football team after the UEFA Euro 2020 Finals shows that it is technically feasible for abusive content to be proactively detected and removed by the platforms themselves. However, this appears to only be for high-profile situations or for well-known individuals. For the general public, the burden of dealing with abuse usually falls to the targets to report messages themselves, even as they are in the midst of receiving targeted harassment and threats. Indeed, the current processes for reporting incidents of harassment are often opaque and confusing. Once a report is made, targets of harassment have very little control over the resolution of the report or the speed at which it is addressed. Platforms also have different policies on whether and how a user is notified after a moderation decision is made. A lot of these notifications are also conducted through automated systems with no way to appeal, leaving users with limited means for recourse.
Recent years have seen an increase in efforts to combat online harassment. Most notably, in June 2022, Vice President Kamala Harris launched a new White House Task Force to Address Online Harassment and Abuse, co-chaired by the Gender Policy Council and the National Security Council. The Task Force aims to develop policy solutions to enhance accountability of perpetrators of online harm while expanding data collection efforts and increasing access to survivor-centered services. In March 2022, the Biden-Harris Administration also launched the Global Partnership for Action on Gender-Based Online Harassment and Abuse, alongside Australia, Denmark, South Korea, Sweden, and the United Kingdom. The partnership works to advance shared principles and attitudes toward online harassment, improve prevention and response measures to gender-based online harassment, and expand data and access on gender-based online harassment.
Efforts focus on technical interventions, such as tools that increase individuals’ digital safety, automatically blur out slurs, or allow trusted individuals to moderate abusive messages directed towards victims’ accounts. There are also many guides that walk individuals through how to better manage their online presence or what to do in response to being targeted. Other organizations provide support for those who are victims and provide next steps, help with reporting, and information on better security practices. However, due to resource constraints, organizations may only be able to support specific types of targets, such as journalists, victims of intimate partner violence, or targets of gendered disinformation. This increases the burden on victims to find support for their specific needs. Academic institutions and researchers have also been developing tools and interventions that measure and address online abuse or improve content moderation. While there are increasing collaborations between academics and civil society, there are still gaps that prevent such interventions from being deployed to their full efficacy.
While complete privacy and security is extremely different to ensure in a technical sense, we envision a database design that preserves data privacy while maintaining its usability. First, the fields of information required for filing an incident report form would minimize the amount of personally identifiable information collected. As some data can be crowdsourced from the public and external observers, this part of the dataset would consist of existing public data. Nonpublicly available data would be entered by only individuals who are sharing incidents that are targeting them (e.g., direct messages), and individuals would be allowed to choose whether it is visible in the database or only shown in summary statistics. Furthermore, the data collection methods and the database structure will be periodically reviewed by the steering committee of civil society organizations, who will make recommendations for improvement as needed.
Data collection and reporting can be conducted internationally, as we recognize that limiting data collection to the U.S. will also undermine our goals of intersectionality. However, the hotline will likely have more comprehensive support for U.S.-based issues. In the long run, however, efforts can also be expanded internationally, as a cross-collaborative effort across multinational governments.
Creating a Fair Work Ombudsman to Bolster Protections for Gig Workers
To increase protections for fair work, the U.S. Department of Labor (DOL) should create an Office of the Ombudsman for Fair Work. Gig workers are a category of non-employee contract workers who engage in on-demand work, often through online platforms. They have had historic vulnerabilities in the U.S. economy. A large portion of gig workers are people of color, and the nature of their temporary and largely unregulated work can leave them vulnerable to economic instability and workplace abuse. Currently, there is no federal mechanism to protect gig workers, and state-level initiatives have not offered thorough enough policy redress. Establishing an Office of the Ombudsman would provide the Department of Labor with a central entity to investigate worker complaints against gig employers, collect data and evidence about the current gig economy, and provide education to gig workers about their rights. There is strong precedent for this policy solution, since bureaus across the federal government have successfully implemented ombudsmen that are independent and support vulnerable constituents. To ensure its legal and long-lasting status, the Secretary of Labor should establish this Office in an act of internal agency reorganization.
Challenge and Opportunity
The proportion of the U.S. workforce engaging in gig work has risen steadily in the past few decades, from 10.1% in 2005 to 15.8% in 2015 to roughly 20% in 2018. Since the COVID-19 pandemic began, this trend has only accelerated, and a record number of Americans have now joined the gig economy and rely on its income. In a 2021 Pew Research study, over 16% of Americans reported having made money through online platform work alone, such as on apps like Uber and Doordash, which is merely a subset of gig work jobs. Gig workers in particular are more likely to be Black or Latino compared to the overall workforce.
Though millions of Americans rely on gig work, it does not provide critical employee benefits, such as minimum wage guarantees, parental leave, healthcare, overtime, unemployment insurance, or recourse for injuries incurred during work. According to an NPR survey, in 2018 more than half of contract workers received zero benefits through work. Further, the National Labor Relations Act, which protects employees’ rights to unionize and collectively bargain without retaliation, does not protect gig workers. This lack of benefits, rights, and voice leaves millions of workers more vulnerable than full-time employees to predatory employers, financial instability, and health crises, particularly during emergencies—such as the COVID-19 pandemic.
Additionally, in 2022, inflation reached a decades-long high, and though the price of necessities has spiked, wages have not increased correspondingly. Extreme inflation hurts lower-income workers without savings the most and is especially dangerous to gig workers, some of whom make less than the federal minimum hourly wage and whose income and work are subject to constant flux.
State-level measures have as yet failed to create protections for all gig workers. In 2020, California passed AB5, legally reclassifying many gig workers as employees instead of independent contractors and thus entitling them to more benefits and protections. But further bills and Proposition 22 reverted several groups of gig workers, including online platform gig workers like Uber and Doordash drivers, to being independent contractors. Ongoing litigation related to Proposition 22 leaves the future status of online platform gig workers in California unclear. In 2022, Washington State passed ESHB 2076 guaranteeing online platform workers—but not all gig workers—the benefits of full-time employees.
This sparse patchwork of state-level measures, which only supports subgroups of gig workers, could trigger a “race to the bottom” in which employers of gig workers relocate to less strict states. Additionally, inconsistencies between state laws make it harder for gig workers to understand their rights and gain redress for grievances, harder for businesses to determine with certainty their duties and liabilities, and harder for states to enforce penalties when an employer is headquartered in one state and the gig worker lives in another. The status quo is also difficult for businesses that strive to be better employers because it creates downward pressure on the entire landscape of labor market competition. Ultimately, only federal policy action can fully address these inconsistencies and broadly increase protections and benefits for all gig workers.
The federal ombudsman’s office outlined in this proposal can serve as a resource for gig workers to understand the scope of their current rights, provide a voice to amplify their grievances and harms, and collect data and evidence to inform policy proposals. It is the first step toward a sustainable and comprehensive national solution that expands the rights of gig workers.
Specifically, clarifying what rights, benefits, and means of recourse gig workers do and do not have would help gig workers better plan for healthcare and other emergent needs. It would also allow better tracking of trends in the labor market and systemic detection of employee misclassification. Hearing gig workers’ complaints in a centralized office can help the Department of Labor more expeditiously address gig workers’ concerns in situations where they legally do have recourse and can otherwise help the Department of Labor better understand the needs of and harms experienced by all workers. Collecting broad-ranging data on gig workers in particular could help inform federal policy change on their rights and protections. Currently, most datasets are survey based and often leave out people who were not working a gig job at the time the survey was conducted but typically otherwise do. More broadly, because of its informal and dynamic nature, the gig economy is difficult to accurately count and characterize, and an entity that is specifically charged with coordinating and understanding this growing sector of the market is key.
Lastly, employees who are not gig workers are sometimes misclassified as such and thus lose out on benefits and protections they are legally entitled to. Having a centralized ombudsman office dedicated to gig work could expedite support of gig workers seeking to correct their classification status, which the Wage and Hour Division already generally deals with, as well as help the Department of Labor and other agencies collect data to clarify the scope of the problem.
Plan of Action
The Department of Labor should establish an Office of the Ombudsman for Fair Work. This office should be independent of Department of Labor agencies and officials, and it should report directly to the Secretary of Labor. The Office would operate on a federal level with authority over states.
The Secretary of Labor should establish the Office in an act of internal agency reorganization. By establishing the Office such that its powers do not contradict the Department of Labor’s statutory limitations, the Secretary can ensure the Office’s status as legal and long-lasting, due to the discretionary power of the Department to interpret its statutes.
The role of the Office of the Ombudsman for Fair Work would be threefold: to serve as a centralized point of contact for hearing complaints from gig workers; to act as a central resource and conduct outreach to gig workers about their rights and protections; and to collect data such as demographic, wage, and benefit trends on the labor practices of the gig economy. Together, these responsibilities ensure that this Office consolidates and augments the actions of the Department of Labor as they pertain to workers in the gig economy, regardless of their classification status.
The functions of the ombudsman should be as follows:
- Establish a clear and centralized mechanism for hearing, collating, and investigating complaints from workers in the gig economy, such as through a helpline or mobile app.
- Establish and administer an independent, neutral, and confidential process to receive, investigate, resolve, and provide redress for cases in which employers misrepresent to individuals that they are engaged as independent contractors when they’re actually engaged as employees.
- Commence court proceedings to enforce fair work practices and entitlements, as they pertain to workers in the gig economy, in conjunction with other offices in the DOL.
- Represent employees or contractors who are or may become a party to proceedings in court over unfair contracting practices, including but not limited to misclassification as independent contractors. The office would refer matters to interagency partners within the Department of Labor and across other organizations engaged in these proceedings, augmenting existing work where possible.
- Provide education, assistance, and advice to employees, employers, and organizations, including best practice guides to workplace relations or workplace practices and information about rights and protections for workers in the gig economy.
- Conduct outreach in multiple languages to gig economy workers informing them of their rights and protections and of the Office’s role to hear and address their complaints and entitlements.
- Serve as the central data collection and publication office for all gig-work-related data. The Office will publish a yearly report detailing demographic, wage, and benefit trends faced by gig workers. Data could be collected through outreach to gig workers or their employers, or through a new data-sharing agreement with the Internal Revenue Service (IRS). This data report would also summarize anonymized trends based on the complaints collected (as per function 1), including aggregate statistics on wage theft, reports of harassment or discrimination, and misclassification. These trends would also be broken down by demographic group to proactively identify salient inequities. The office may also provide separate data on platform workers, which may be easier to collect and collate, since platform workers are a particular subject of focus in current state legislation and litigation.
Establishing an Office of the Ombudsman for Fair Work within the Department of Labor will require costs of compensation for the ombudsman and staff, other operational costs, and litigation expenses. To reflect the need for a reaction to the rapid ongoing changes in gig economy platforms, a small portion of the Office’s budget should be set aside to support the appointment of a chief innovation officer, aimed at examining how technology can strengthen its operations. Some examples of tasks for this role include investigating and strengthening complaint sorting infrastructure, utilizing artificial intelligence to evaluate contracts for misclassification, and streamlining request for proposal processes.
Due to the continued growth of the gig economy, and the precarious status of gig workers in the onset of an economic recession, this Office should be established in the nearest possible window. Establishing, appointing, and initiating this office will require up to a year of time, and will require budgeting within the DOL.
There are many precedents of ombudsmen in federal office, including the Office of the Ombudsman for the Energy Employees Occupational Illness Compensation Program within the Department of Labor. Additionally, the IRS established the Office of the Taxpayer Advocate, and the Department of Homeland Security has both a Citizenship and Immigration Services Ombudsman and an Immigration Detention Ombudsman. These offices have helped educate constituents about their rights, resolved issues that an individual might have with that federal agency, and served as independent oversight bodies. The Australian Government has a Fair Work Ombudsman that provides resources to differentiate between an independent contractor and employee and investigates employers who may be engaging in sham contracting or other illegal practices. Following these examples, the Office of the Ombudsman for Fair Work should work within the Department of Labor to educate, assist, and provide redress for workers engaged in the gig economy.
Conclusion
How to protect gig workers is a long-standing open question for labor policy and is likely to require more attention as post-pandemic conditions affect labor trends. The federal government needs a solution to the issues of vulnerability and instability experienced by gig workers, and this solution needs to operate independently of legislation that may take longer to gain consensus on. Establishing an office of an ombudsman is the first step to increase federal oversight for gig work. The ombudsman will use data, reporting, and individual worker cases to build a clearer picture for how to create redress for laborers that have been harmed by gig work, which will provide greater visibility into the status and concerns of gig workers. It will additionally serve as a single point of entry for gig workers and businesses to learn about their rights and for gig workers to lodge complaints. If made a reality, this office will be an influential first step in changing the entire policy ecosystem regarding gig work.
There is a current definitional debate about whether gig workers and platform workers are employees or contractors. Until this issue of misclassification can be resolved, there will likely not be a comprehensive state or federal policy governing gig work. However, the office of an ombudsman would be able to serve as the central point within the Department of Labor to handle gig worker issues, and it would be the entity tasked with collecting and publishing data about this class of laborers. This would help elevate the problems gig workers face as well as paint a picture of the extent of the issue for future legislation.
Each ombudsman will be appointed for a six-year period, to ensure insulation from partisan politics.
States often do not have adequate solutions to handle the discrepancies between employees and contractors. There is also the “race to the bottom” issue, where if protections are increased in one state, gig employers will simply relocate to states where the policies are less stringent. Further, there is the issue of gig companies being headquartered in one state while employees work in another. It makes sense for the Department of Labor to house a central, federal mechanism to handle gig work.
The key challenge right now is for the federal government to collect data and solve issues regarding protections for gig work. The office of the ombudsman’s broadly defined mandate is actually an advantage in this still-developing conversation about gig work.
Establishing a new Department of Labor office is no small feat. It requires a clear definition of the goal and allowed activities of the ombudsman. This would require buy-in from key DOL bureaucrats. The office would also have to hire, recruit, and train staff. These tasks may be speed bottlenecks for this proposal to get off the ground. Since DOL plans its budget several years in advance, this proposal would likely be targeted for the 2026 cycle.
Establishing an AI Center of Excellence to Address Maternal Health Disparities
Maternal mortality is a crisis in the United States. Yet more than 60% of maternal deaths are preventable—with the right evidence-based interventions. Data is a powerful tool for uncovering best care practices. While healthcare data, including maternal health data, has been generated at a massive scale by the widespread adoption and use of Electronic Health Records (EHR), much of this data remains unstandardized and unanalyzed. Further, while many federal datasets related to maternal health are openly available through initiatives set forth in the Open Government National Action Plan, there is no central coordinating body charged with analyzing this breadth of data. Advancing data harmonization, research, and analysis are foundational elements of the Biden Administration’s Blueprint for Addressing the Maternal Health Crisis. As a data-driven technology, artificial intelligence (AI) has great potential to support maternal health research efforts. Examples of promising applications of AI include using electronic health data to predict whether expectant mothers are at risk of difficulty during delivery. However, further research is needed to understand how to effectively implement this technology in a way that promotes transparency, safety, and equity. The Biden-Harris Administration should establish an AI Center of Excellence to bring together data sources and then analyze, diagnose, and address maternal health disparities, all while demonstrating trustworthy and responsible AI principles.
Challenge and Opportunity
Maternal deaths currently average around 700 per year, and severe maternal morbidity-related conditions impact upward of 60,000 women annually. Stark maternal health disparities persist in the United States, and pregnancy outcomes are subject to substantial racial/ethnic disparities, including maternal morbidity and mortality. According to the Centers for Disease Control and Prevention (CDC), “Black women are three times more likely to die from a pregnancy-related cause than White women.” Research is ongoing to specifically identify the root causes, which include socioeconomic factors such as insurance status, access to healthcare services, and risks associated with social determinants of health. For example, maternity care deserts exist in counties throughout the country where maternal health services are substantially limited or not available, impacting an estimated 2.2 million women of child-bearing age.
Many federal, public, and private datasets exist to understand the conditions that impact pregnant people, the quality of the care they receive, and ultimate care outcomes. For example, the CDC collects abundant data on maternal health, including the Pregnancy Mortality Surveillance System (PMSS) and the National Vital Statistics System (NVSS). Many of these datasets, however, have yet to be analyzed at scale or linked to other federal or privately held data sources in a comprehensive way. More broadly, an estimated 30% of the data generated globally is produced by the healthcare industry. AI is uniquely designed for data management, including cataloging, classification, and data integration. AI will play a pivotal role in the federal government’s ability to process an unprecedented volume of data to generate evidence-based recommendations to improve maternal health outcomes.
Applications of AI have rapidly proliferated throughout the healthcare sector due to their potential to reduce healthcare expenditures and improve patient outcomes (Figure 1). Several applications of this technology exist across the maternal health continuum and are shown in the figure below. For example, evidence suggests that AI can help clinicians identify more than 70% of at-risk moms during the first trimester by analyzing patient data and identifying patterns associated with poor health outcomes. Based on its findings, AI can provide recommendations for which patients will most likely be at-risk for pregnancy challenges before they occur. Research has also demonstrated the use of AI in fetal health monitoring.
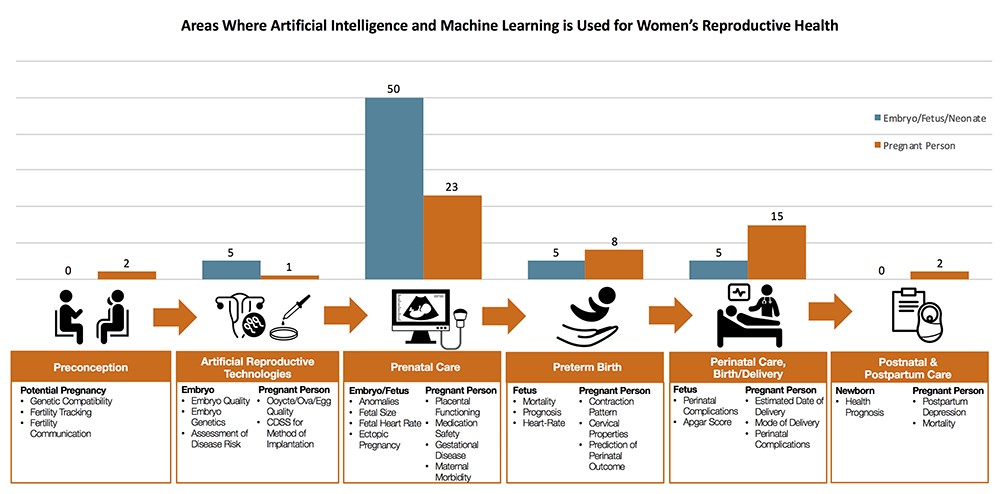
Source: Davidson and Boland (2021).
Yet for all of AI’s potential, there is a significant dearth of consumer and medical provider understanding of how these algorithms work. Policy analysts argue that “algorithmic discrimination” and feedback loops in algorithms—which may exacerbate algorithmic bias—are potential risks of using AI in healthcare outside of the confines of an ethical framework. In response, certain federal entities such as the Department of Defense, the Office of the Director of National Intelligence, the National Institute for Standards and Technology, and the U.S. Department of Health and Human Services have published and adopted guidelines for implementing data privacy practices and building public trust of AI. Further, past Day One authors have proposed the establishment of testbeds for government-procured AI models to provide services to U.S. citizens. This is critical for enhancing the safety and reliability of AI systems while reducing the risk of perpetuating existing structural inequities.
It is vital to demonstrate safe, trustworthy uses of AI and measure the efficacy of these best practices through applications of AI to real-world societal challenges. For example, potential use cases of AI for maternal health include a social determinants of health [SDoH] extractor, which combines AI with clinical notes to more effectively identify SDoH information and analyze its potential role in health inequities. A center dedicated to ethically developing AI for maternal health would allow for the development of evidence-based guidelines for broader AI implementation across healthcare systems throughout the country. Lessons learned from this effort will contribute to the knowledge base around ethical AI and enable development of AI solutions for health disparities more broadly.
Plan of Action
To meet the calls for advancing data collection, standardization, transparency, research, and analysis to address the maternal health crisis, the Biden-Harris Administration should establish an AI Center of Excellence for maternal health. The AI Center of Excellence for Maternal Health will bring together data sources, then analyze, diagnose, and address maternal health disparities, all while demonstrating trustworthy and responsible AI principles. The Center should be created within the Department of Health and Human Services (HHS) and work closely with relevant offices throughout HHS and beyond, including the HHS Office of the Chief Artificial Intelligence Officer (OCAIO), the National Institutes of Health (NIH) IMPROVE initiative, the CDC, the Veterans Health Administration (VHA), and the National Institute for Standards and Technology (NIST). The Center should offer competitive salaries to recruit the best and brightest talent in AI, human-centered design, biostatistics, and human-computer interaction.
The first priority should be to work with all agencies tasked by the White House Blueprint for Addressing the Maternal Health Crisis to collect and evaluate data. This includes privately held EHR data that is made available through the Qualified Health Information Network (QHIN) and federal data from the CDC, Centers for Medicare and Medicaid (CMS), Office of Personnel Management (OPM), Healthcare Resources and Services Agency (HRSA), NIH, United States Department of Agriculture (USDA), Housing and Urban Development (HUD), the Veterans Health Administration, and Environmental Protection Agency (EPA), all of which contain datasets relevant to maternal health at different stages of the reproductive health journey from Figure 1. The Center should serve as a data clearing and cleaning shop, preparing these datasets using best practices for data management, preparation, and labeling.
The second priority should be to evaluate existing datasets to establish high-priority, high-impact applications of AI-enabled research for improving clinical care guidelines and tools for maternal healthcare providers. These AI demonstrations should be aligned with the White House’s Action Plan and be focused on implementing best practices for AI development, such as the AI Risk Management Framework developed by NIST. The following examples demonstrate how AI might help address maternal health disparities, based on priority areas informed by clinicians in the field:
- AI implementation should be explored for analysis of electronic health records from the VHA and QHIN to predict patients who have a higher risk of pregnancy and/or delivery complications.
- Drawing on the robust data collection and patient surveillance capabilities of the VHA and HRSA, AI should be explored for the deployment of digital tools to help monitor patients during pregnancy to ensure adequate and consistent use of prenatal care.
- Using VHA data and QHIN data, AI should be explored in supporting patient monitoring in instances of patient referrals and/or transfers to hospitals that are appropriately equipped to serve high-risk patients, following guidelines provided by the American College of Obstetricians and Gynecologists.
- Data on housing from HUD, rural development from the USDA, environmental health from the EPA, and social determinants of health research from the CDC should be connected to risk factors for maternal mortality in the academic literature to create an AI-powered risk algorithm.
- Understand the power of payment models operated by CMS and OPM for novel strategies to enhance maternal health outcomes and reduce maternal deaths.
The final priority should be direct translation of the findings from AI to federal policymaking around reducing maternal health disparities as well as ethical development of AI tools. Research findings for both aspects of this interdisciplinary initiative should be framed using Living Evidence models that help ensure that research-derived evidence and guidance remain current.
The Center should be able to meet the following objectives within the first year after creation to further the case for future federal funding and creation of more AI Centers of Excellence for healthcare:
- Conduct a study on the use cases uncovered for AI to help address maternal health disparities explored through the various demonstration projects.
- Publish a report of study findings, which should be submitted to Congress with recommendations to help inform funding priorities for subsequent research activities.
- Make study findings available to the public to help build public trust in AI.
Successful piloting of the Center could be made possible by passage of an equivalent bill to S.893 in the current Congress. This is a critical first step in supporting this work. In March 2021, the S.893—Tech to Save Moms Act was introduced in the Senate to fund research conducted by National Academies of Sciences, Engineering, and Medicine to understand the role of AI in maternal care delivery and its impact on bias in maternal health. Passage of an equivalent bill into law would enable the National Academies of Sciences, Engineering, and Medicine to conduct research in parallel with HHS to generate more findings and to broaden potential impact.
Conclusion
The United States has the highest rate of maternal health disparities among all developed countries. Yet more than 60% of pregnancy-related deaths are preventable, highlighting a critical opportunity to uncover the factors impeding more equitable health outcomes for the nation as a whole. Legislative support for research to understand AI’s role in addressing maternal health disparities will affirm the nation’s commitment to ensuring that we are prepared to thrive in a 21st century influenced and shaped by next-generation technologies such as artificial intelligence.
Creating Auditing Tools for AI Equity
The unregulated use of algorithmic decision-making systems (ADS)—systems that crunch large amounts of personal data and derive relationships between data points—has negatively affected millions of Americans. These systems impact equitable access to education, housing, employment, and healthcare, with life-altering effects. For example, commercial algorithms used to guide health decisions for approximately 200 million people in the United States each year were found to systematically discriminate against Black patients, reducing, by more than half, the number of Black patients who were identified as needing extra care.
One way to combat algorithmic harm is by conducting system audits, yet there are currently no standards for auditing AI systems at the scale necessary to ensure that they operate legally, safely, and in the public interest. According to one research study examining the ecosystem of AI audits, only one percent of AI auditors believe that current regulation is sufficient.
To address this problem, the National Institute of Standards and Technology (NIST) should invest in the development of comprehensive AI auditing tools, and federal agencies with the charge of protecting civil rights and liberties should collaborate with NIST to develop these tools and push for comprehensive system audits.
These auditing tools would help the enforcement arms of these federal agencies save time and money while fulfilling their statutory duties. Additionally, there is a pressing need to develop these tools now, with Executive Order 13985 instructing agencies to “focus their civil rights authorities and offices on emerging threats, such as algorithmic discrimination in automated technology.”
Challenge and Opportunity
The use of AI systems across all aspects of life has become commonplace as a way to improve decision-making and automate routine tasks. However, their unchecked use can perpetuate historical inequities, such as discrimination and bias, while also potentially violating American civil rights.
Algorithmic decision-making systems are often used in prioritization, classification, association, and filtering tasks in a way that is heavily automated. ADS become a threat when people uncritically rely on the outputs of a system, use them as a replacement for human decision-making, or use systems with no knowledge of how they were developed. These systems, while extremely useful and cost-saving in many circumstances, must be created in a way that is equitable and secure.
Ensuring the legal and safe use of ADS begins with recognizing the challenges that the federal government faces. On the one hand, the government wants to avoid devoting excessive resources to managing these systems. With new AI system releases happening everyday, it is becoming unreasonable to oversee every system closely. On the other hand, we cannot blindly trust all developers and users to make appropriate choices with ADS.
This is where tools for the AI development lifecycle come into play, offering a third alternative between constant monitoring and blind trust. By implementing auditing tools and signatory practices, AI developers will be able to demonstrate compliance with preexisting and well-defined standards while enhancing the security and equity of their systems.
Due to the extensive scope and diverse applications of AI systems, it would be difficult for the government to create a centralized body to oversee all systems or demand each agency develop solutions on its own. Instead, some responsibility should be shifted to AI developers and users, as they possess the specialized knowledge and motivation to maintain proper functioning systems. This allows the enforcement arms of federal agencies tasked with protecting the public to focus on what they do best, safeguarding citizens’ civil rights and liberties.
Plan of Action
To ensure security and verification throughout the AI development lifecycle, a suite of auditing tools is necessary. These tools should help enable outcomes we care about, fairness, equity, and legality. The results of these audits should be reported (for example, in an immutable ledger that is only accessible by authorized developers and enforcement bodies) or through a verifiable code-signing mechanism. We leave the specifics of the reporting and documenting the process to the stakeholders involved, as each agency may have different reporting structures and needs. Other possible options, such as manual audits or audits conducted without the use of tools, may not provide the same level of efficiency, scalability, transparency, accuracy, or security.
The federal government’s role is to provide the necessary tools and processes for self-regulatory practices. Heavy-handed regulations or excessive government oversight are not well-received in the tech industry, which argues that they tend to stifle innovation and competition. AI developers also have concerns about safeguarding their proprietary information and users’ personal data, particularly in light of data protection laws.
Auditing tools provide a solution to this challenge by enabling AI developers to share and report information in a transparent manner while still protecting sensitive information. This allows for a balance between transparency and privacy, providing the necessary trust for a self-regulating ecosystem.
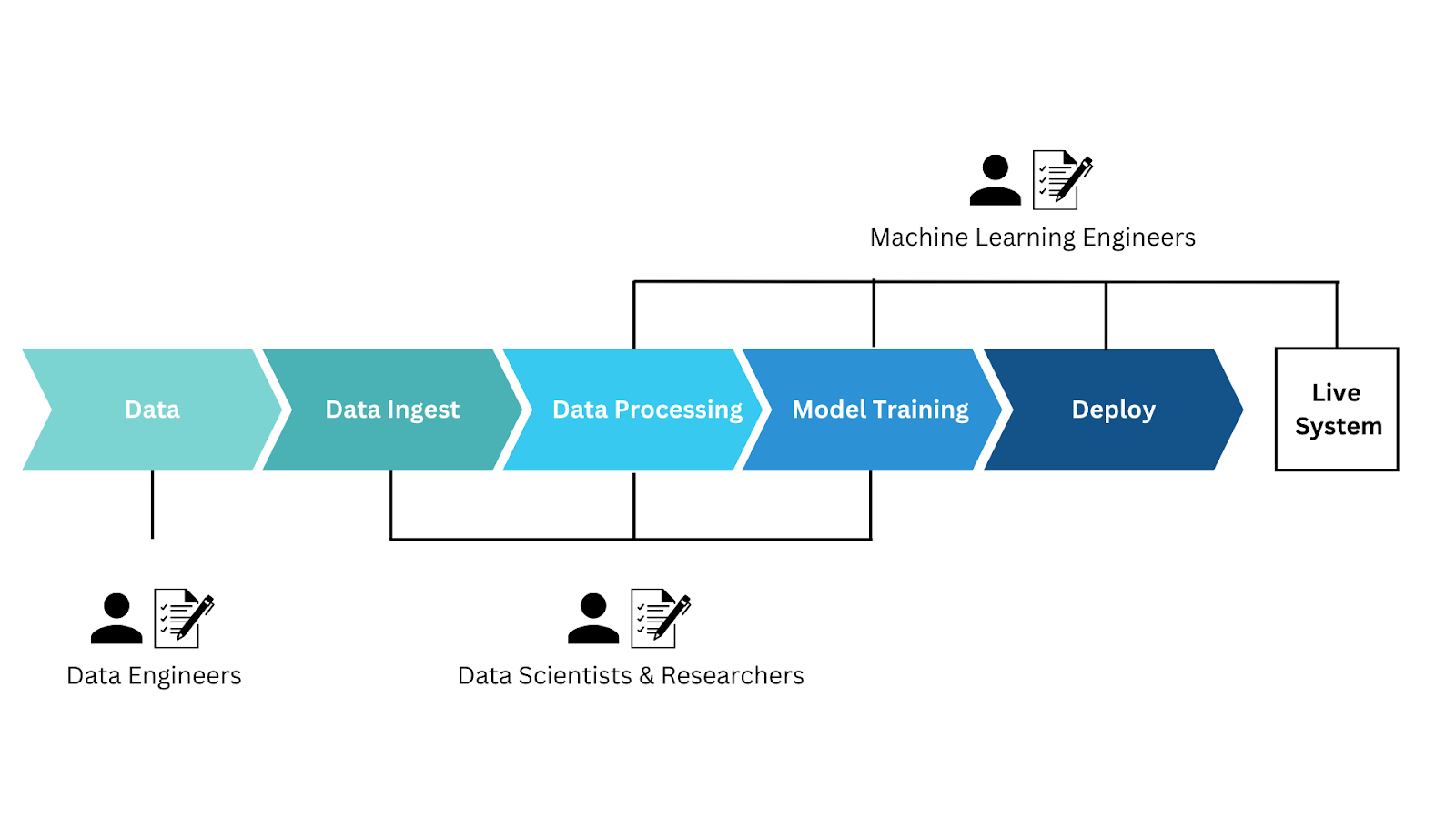
A general machine learning lifecycle. Examples of what system developers at each stage would be responsible for signing off on the use of the security and equity tools in the lifecycle. These developers represent companies, teams, or individuals.
The equity tool and process, funded and developed by government agencies such as NIST, would consist of a combination of (1) AI auditing tools for security and fairness (which could be based on or incorporate open source tools such as AI Fairness 360 and the Adversarial Robustness Toolbox), and (2) a standardized process and guidance for integrating these checks (which could be based on or incorperate guidance such as the U.S. Government Accountability Office’s Artificial Intelligence: An Accountability Framework for Federal Agencies and Other Entities).1
Dioptra, a recent effort between NIST and the National Cybersecurity Center of Excellence (NCCoE) to build machine learning testbeds for security and robustness, is an excellent example of the type of lifecycle management application that would ideally be developed. Failure to protect civil rights and ensure equitable outcomes must be treated as seriously as security flaws, as both impact our national security and quality of life.
Equity considerations should be applied across the entire lifecycle; training data is not the only possible source of problems. Inappropriate data handling, model selection, algorithm design, and deployment, also contribute to unjust outcomes. This is why tools combined with specific guidance is essential.
As some scholars note, “There is currently no available general and comparative guidance on which tool is useful or appropriate for which purpose or audience. This limits the accessibility and usability of the toolkits and results in a risk that a practitioner would select a sub-optimal or inappropriate tool for their use case, or simply use the first one found without being conscious of the approach they are selecting over others.”
Companies utilizing the various packaged tools on their ADS could sign off on the results using code signing. This would create a record that these organizations ran these audits along their development lifecycle and received satisfactory outcomes.
We envision a suite of auditing tools, each tool applying to a specific agency and enforcement task. Precedents for this type of technology already exist. Much like security became a part of the software development lifecycle with guidance developed by NIST, equity and fairness should be integrated into the AI lifecycle as well. NIST could spearhead a government-wide initiative on auditing AI tools, leading guidance, distribution, and maintenance of such tools. NIST is an appropriate choice considering its history of evaluating technology and providing guidance around the development and use of specific AI applications such as the NIST-led Face Recognition Vendor Test (FRVT).
Areas of Impact & Agencies / Departments Involved
Security & Justice
The U.S. Department of Justice, Civil Rights Division, Special Litigation SectionDepartment of Homeland Security U.S. Customs and Border Protection U.S. Marshals Service
Public & Social Sector
The U.S. Department of Housing and Urban Development’s Office of Fair Housing and Equal Opportunity
Education
The U.S. Department of Education
Environment
The U.S. Department of Agriculture, Office of the Assistant Secretary for Civil RightsThe Federal Energy Regulatory CommissionThe Environmental Protection Agency
Crisis Response
Federal Emergency Management Agency
Health & Hunger
The U.S. Department of Health and Human Services, Office for Civil RightsCenter for Disease Control and PreventionThe Food and Drug Administration
Economic
The Equal Employment Opportunity Commission, The U.S. Department of Labor, Office of Federal Contract Compliance Programs
Infrastructure
The U.S. Department of Transportation, Office of Civil RightsThe Federal Aviation AdministrationThe Federal Highway Administration
Information Verification & Validation
The Federal Trade Commission, The Federal Communication Commission, The Securities and Exchange Commission.
Many of these tools are open source and free to the public. A first step could be combining these tools with agency-specific standards and plain language explanations of their implementation process.
Benefits
These tools would provide several benefits to federal agencies and developers alike. First, they allow organizations to protect their data and proprietary information while performing audits. Any audits, whether on the data, model, or overall outcomes, would be run and reported by the developers themselves. Developers of these systems are the best choice for this task since ADS applications vary widely, and the particular audits needed depend on the application.
Second, while many developers may opt to use these tools voluntarily, standardizing and mandating their use would allow an evaluation of any system thought to be in violation of the law to be easily assesed. In this way, the federal government will be able to manage standards more efficiently and effectively.
Third, although this tool would be designed for the AI lifecycle that results in ADS, it can also be applied to traditional auditing processes. Metrics and evaluation criteria will need to be developed based on existing legal standards and evaluation processes; once these metrics are distilled for incorporation into a specific tool, this tool can be applied to non-ADS data as well, such as outcomes or final metrics from traditional audits.
Fourth, we believe that a strong signal from the government that equity considerations in ADS are important and easily enforceable will impact AI applications more broadly, normalizing these considerations.
Example of Opportunity
An agency that might use this tool is the Department of Housing and Urban Development (HUD), whose purpose is to ensure that housing providers do not discriminate based on race, color, religion, national origin, sex, familial status, or disability.
To enforce these standards, HUD, which is responsible for 21,000 audits a year, investigates and audits housing providers to assess compliance with the Fair Housing Act, the Equal Credit Opportunity Act, and other related regulations. During these audits, HUD may review a provider’s policies, procedures, and records, as well as conduct on-site inspections and tests to determine compliance.
Using an AI auditing tool could streamline and enhance HUD’s auditing processes. In cases where ADS were used and suspected of harm, HUD could ask for verification that an auditing process was completed and specific metrics were met, or require that such a process be undergone and reported to them.
Noncompliance with legal standards of nondiscrimination would apply to ADS developers as well, and we envision the enforcement arms of protection agencies would apply the same penalties in these situations as they would in non-ADS cases.
R&D
To make this approach feasible, NIST will require funding and policy support to implement this plan. The recent CHIPS and Science Act has provisions to support NIST’s role in developing “trustworthy artificial intelligence and data science,” including the testbeds mentioned above. Research and development can be partially contracted out to universities and other national laboratories or through partnerships/contracts with private companies and organizations.
The first iterations will need to be developed in partnership with an agency interested in integrating an auditing tool into its processes. The specific tools and guidance developed by NIST must be applicable to each agency’s use case.
The auditing process would include auditing data, models, and other information vital to understanding a system’s impact and use, informed by existing regulations/guidelines. If a system is found to be noncompliant, the enforcement agency has the authority to impose penalties or require changes to be made to the system.
Pilot program
NIST should develop a pilot program to test the feasibility of AI auditing. It should be conducted on a smaller group of systems to test the effectiveness of the AI auditing tools and guidance and to identify any potential issues or areas for improvement. NIST should use the results of the pilot program to inform the development of standards and guidelines for AI auditing moving forward.
Collaborative efforts
Achieving a self-regulating ecosystem requires collaboration. The federal government should work with industry experts and stakeholders to develop the necessary tools and practices for self-regulation.
A multistakeholder team from NIST, federal agency issue experts, and ADS developers should be established during the development and testing of the tools. Collaborative efforts will help delineate responsibilities, with AI creators and users responsible for implementing and maintaining compliance with the standards and guidelines, and agency enforcement arms agency responsible for ensuring continued compliance.
Regular monitoring and updates
The enforcement agencies will continuously monitor and update the standards and guidelines to keep them up to date with the latest advancements and to ensure that AI systems continue to meet the legal and ethical standards set forth by the government.
Transparency and record-keeping
Code-signing technology can be used to provide transparency and record-keeping for ADS. This can be used to store information on the auditing outcomes of the ADS, making reporting easy and verifiable and providing a level of accountability to users of these systems.
Conclusion
Creating auditing tools for ADS presents a significant opportunity to enhance equity, transparency, accountability, and compliance with legal and ethical standards. The federal government can play a crucial role in this effort by investing in the research and development of tools, developing guidelines, gathering stakeholders, and enforcing compliance. By taking these steps, the government can help ensure that ADS are developed and used in a manner that is safe, fair, and equitable.
Code signing is used to establish trust in code that is distributed over the internet or other networks. By digitally signing the code, the code signer is vouching for its identity and taking responsibility for its contents. When users download code that has been signed, their computer or device can verify that the code has not been tampered with and that it comes from a trusted source.
Code signing can be extended to all parts of the AI lifecycle as a means of verifying the authenticity, integrity, and function of a particular piece of code or a larger process. After each step in the auditing process, code signing enables developers to leave a well-documented trail for enforcement bodies/auditors to follow if a system were suspected of unfair discrimination or unsafe operation.
Code signing is not essential for this project’s success, and we believe that the specifics of the auditing process, including documentation, are best left to individual agencies and their needs. However, code signing could be a useful piece of any tools developed.
Additionally, there may be pushback on the tool design. It is important to remember that currently, engineers often use fairness tools at the end of a development process, as a last box to check, instead of as an integrated part of the AI development lifecycle. These concerns can be addressed by emphasizing the comprehensive approach taken and by developing the necessary guidance to accompany these tools—which does not currently exist.
New York regulators are calling on a UnitedHealth Group to either stop using or prove there is no problem with a company-made algorithm that researchers say exhibited significant racial bias. This algorithm, which UnitedHealth Group sells to hospitals for assessing the health risks of patients, assigned similar risk scores to white patients and Black patients despite the Black patients being considerably sicker.
In this case, researchers found that changing just one parameter could generate “an 84% reduction in bias.” If we had specific information on the parameters going into the model and how they are weighted, we would have a record-keeping system to see how certain interventions affected the output of this model.
Bias in AI systems used in healthcare could potentially violate the Constitution’s Equal Protection Clause, which prohibits discrimination on the basis of race. If the algorithm is found to have a disproportionately negative impact on a certain racial group, this could be considered discrimination. It could also potentially violate the Due Process Clause, which protects against arbitrary or unfair treatment by the government or a government actor. If an algorithm used by hospitals, which are often funded by the government or regulated by government agencies, is found to exhibit significant racial bias, this could be considered unfair or arbitrary treatment.
Example #2: Policing
A UN panel on the Elimination of Racial Discrimination has raised concern over the increasing use of technologies like facial recognition in law enforcement and immigration, warning that it can exacerbate racism and xenophobia and potentially lead to human rights violations. The panel noted that while AI can enhance performance in some areas, it can also have the opposite effect as it reduces trust and cooperation from communities exposed to discriminatory law enforcement. Furthermore, the panel highlights the risk that these technologies could draw on biased data, creating a “vicious cycle” of overpolicing in certain areas and more arrests. It recommends more transparency in the design and implementation of algorithms used in profiling and the implementation of independent mechanisms for handling complaints.
A case study on the Chicago Police Department’s Strategic Subject List (SSL) discusses an algorithm-driven technology used by the department to identify individuals at high risk of being involved in gun violence and inform its policing strategies. However, a study by the RAND Corporation on an early version of the SSL found that it was not successful in reducing gun violence or reducing the likelihood of victimization, and that inclusion on the SSL only had a direct effect on arrests. The study also raised significant privacy and civil rights concerns. Additionally, findings reveal that more than one-third of individuals on the SSL, approximately 70% of that cohort, have never been arrested or been a victim of a crime yet received a high-risk score. Furthermore, 56% of Black men under the age of 30 in Chicago have a risk score on the SSL. This demographic has also been disproportionately affected by the CPD’s past discriminatory practices and issues, including torturing Black men between 1972 and 1994, performing unlawful stops and frisks disproportionately on Black residents, engaging in a pattern or practice of unconstitutional use of force, poor data collection, and systemic deficiencies in training and supervision, accountability systems, and conduct disproportionately affecting Black and Latino residents.
Predictive policing, which uses data and algorithms to try to predict where crimes are likely to occur, has been criticized for reproducing and reinforcing biases in the criminal justice system. This can lead to discriminatory practices and violations of the Fourth Amendment’s prohibition on unreasonable searches and seizures, as well as the Fourteenth Amendment’s guarantee of equal protection under the law. Additionally, bias in policing more generally can also violate these constitutional provisions, as well as potentially violating the Fourth Amendment’s prohibition on excessive force.
Example #3: Recruiting
ADS in recruiting crunch large amounts of personal data and, given some objective, derive relationships between data points. The aim is to use systems capable of processing more data than a human ever could to uncover hidden relationships and trends that will then provide insights for people making all types of difficult decisions.
Hiring managers across different industries use ADS every day to aid in the decision-making process. In fact, a 2020 study reported that 55% of human resources leaders in the United States use predictive algorithms across their business practices, including hiring decisions.
For example, employers use ADS to screen and assess candidates during the recruitment process and to identify best-fit candidates based on publicly available information. Some systems even analyze facial expressions during interviews to assess personalities. These systems promise organizations a faster, more efficient hiring process. ADS do theoretically have the potential to create a fairer, qualification-based hiring process that removes the effects of human bias. However, they also possess just as much potential to codify new and existing prejudice across the job application and hiring process.
The use of ADS in recruiting could potentially violate several constitutional laws, including discrimination laws such as Title VII of the Civil Rights Act of 1964 and the Americans with Disabilities Act. These laws prohibit discrimination on the basis of race, gender, and disability, among other protected characteristics, in the workplace. Additionally, the these systems could also potentially violate the right to privacy and the due process rights of job applicants. If the systems are found to be discriminatory or to violate these laws, they could result in legal action against the employers.
Supporting Historically Disadvantaged Workers through a National Bargaining in Good Faith Fund
Black, Indigenous, and other people of color (BIPOC) are underrepresented in labor unions. Further, people working in the gig economy, tech supply chain, and other automation-adjacent roles face a huge barrier to unionizing their workplaces. These roles, which are among the fastest-growing segments of the U.S. economy, are overwhelmingly filled by BIPOC workers. In the absence of safety nets for these workers, the racial wealth gap will continue to grow. The Biden-Harris Administration can promote racial equity and support low-wage BIPOC workers’ unionization efforts by creating a National Bargaining in Good Faith Fund.
As a whole, unions lift up workers to a better standard of living, but historically they have failed to protect workers of color. The emergence of labor unions in the early 20th century was propelled by the passing of the National Labor Relations Act (NLRA), also known as the Wagner Act of 1935. Although the NLRA was a beacon of light for many working Americans, affording them the benefits of union membership such as higher wages, job security, and better working conditions, which allowed many to transition into the middle class, the protections of the law were not applied to all working people equally. Labor unions in the 20th century were often segregated, and BIPOC workers were often excluded from the benefits of unionization. For example, the Wagner Act excluded domestic and agricultural workers and permitted labor unions to discriminate against workers of color in other industries, such as manufacturing.
Today, in the aftermath of the COVID-19 pandemic and amid a renewed interest in a racial reckoning in the United States, BIPOC workers—notably young and women BIPOC workers—are leading efforts to organize their workplaces. In addition to demanding wage equity and fair treatment, they are also fighting for health and safety on the job. Unionized workers earn on average 11.2% more in wages than their nonunionized peers. Unionized Black workers earn 13.7% more and unionized Hispanic workers 20.1% more than their nonunionized peers. But every step of the way, tech giants and multinational corporations are opposing workers’ efforts and their legal right to organize, making organizing a risky undertaking.
A National Bargaining in Good Faith Fund would provide immediate and direct financial assistance to workers who have been retaliated against for attempting to unionize, especially those from historically disadvantaged groups in the United States. This fund offers a simple and effective solution to alleviate financial hardships, allowing affected workers to use the funds for pressing needs such as rent, food, or job training. It is crucial that we advance racial equity, and this fund is one step toward achieving that goal by providing temporary financial support to workers during their time of need. Policymakers should support this initiative as it offers direct payments to workers who have faced illegal retaliation, providing a lifeline for historically disadvantaged workers and promoting greater economic justice in our society.
Challenges and Opportunities
The United States faces several triangulating challenges. First is our rapidly evolving economy, which threatens to displace millions of already vulnerable low-wage workers due to technological advances and automation. The COVID-19 pandemic accelerated automation, which is a long-term strategy for the tech companies that underpin the gig economy. According to a report by an independent research group, self-driving taxis are likely to dominate the ride-hailing market by 2030, potentially displacing 8 million human drivers in the United States alone.
Second, we have a generation of workers who have not reaped the benefits associated with good-paying union jobs due to decades of anti-union activities. As of 2022, union membership has dropped from more than 30% of wage and salary workers in the private sector in the 1950s to just 6.3%. The declining percentage of workers represented by unions is associated with widespread and deep economic inequality, stagnant wages, and a shrinking middle class. Lower union membership rates have contributed to the widening of the pay gap for women and workers of color.
Third, historically disadvantaged groups are overrepresented in nonunionized, low-wage, app-based, and automation-adjacent work. This is due in large part to systemic racism. These structures adversely affect BIPOC workers’ ability to obtain quality education and training, create and pass on generational wealth, or follow through on the steps required to obtain union representation.
Workers face tremendous opposition to unionization efforts from companies that spend hundreds of millions of dollars and use retaliatory actions, disinformation, and other intimidating tactics to stop them from organizing a union. For example, in New York, Black organizer Chris Smalls led the first successful union drive in a U.S. Amazon facility after the company fired him for his activities and made him a target of a smear campaign against the union drive. Smalls’s story is just one illustration of how BIPOC workers are in the middle of the collision between automation and anti-unionization efforts.
The recent surge of support for workers’ rights is a promising development, but BIPOC workers face challenges that extend beyond anti-union tactics. Employer retaliation is also a concern. Workers targeted for retaliation suffer from reduced hours or even job loss. For instance, a survey conducted at the beginning of the COVID-19 pandemic revealed that one in eight workers perceived possible retaliatory actions by their employers against colleagues who raised health and safety concerns. Furthermore, Black workers were more than twice as likely as white workers to experience such possible retaliation. This sobering statistic is a stark reminder of the added layers of discrimination and economic insecurity that BIPOC workers have to navigate when advocating for better working conditions and wages.
The time to enact strong policy supporting historically disadvantaged workers is now. Advancing racial equity and racial justice is a focus for the Biden-Harris Administration, and the political and social will is evident. The day one Biden-Harris Administration Executive Order on Advancing Racial Equity and Support for Underserved Communities Through the Federal Government seeks to develop policies designed to advance equity for all, including people of color and others who have been historically underinvested in, marginalized, and adversely affected by persistent poverty and inequality. Additionally, the establishment of the White House is a significant development. Led by Vice-President Kamala Harris and Secretary of Labor Marty Walsh, the Task Force aims to empower workers to organize and negotiate with their employers through federal government policies, programs, and practices.
A key focus for the Task Force is to increase worker power in underserved communities by examining and addressing the challenges faced by workers in jurisdictions with restrictive labor laws, marginalized workers, and workers in certain industries. The Task Force is well-timed, given the increased support for workers’ rights demonstrated through the record-high number of petitions filed with the National Labor Relations Board and the rise in strikes over the past two years. The Task Force’s approach to empowering workers and supporting their ability to organize and negotiate through federal government policies and programs offers a promising opportunity to address the unique challenges faced by BIPOC workers in unionization efforts.
The National Bargaining in Good Faith Fund is a critical initiative that can help level the playing field by providing financial assistance to workers facing opposition from employers who refuse to engage in good-faith bargaining, thereby expanding access to unions for Black, Indigenous, and other people of color. In addition, the proposed initiative would reinforce Equal Employment Opportunity Commission (EEOC) and National Labor Relations Board (NLRB) policies regarding employer discrimination and retaliation. The Bargaining in Good Faith Fund will provide direct payments to workers whose employers have retaliated against them for engaging in union organizing activities. The initiative also includes monitoring cases where a violation has occurred against workers involved in union organization and connecting their bargaining unit with relevant resources to support their efforts. With the backing of the Task Force, the fund could make a significant difference in the lives of workers facing barriers to organizing.
Plan of Action
While the adoption of a policy like the Bargaining in Good Faith Fund is unprecedented at the federal level, we draw inspiration from successful state-level initiatives aimed at improving worker well-being. Two notable examples are initiatives enacted in California and New York, where state lawmakers provided temporary monetary assistance to workers affected by the COVID-19 pandemic. Taking a cue from these successful programs, we can develop federal policies that better support workers, especially those belonging to historically disadvantaged groups.
The successful implementation of worker-led, union-organized, and community-led strike assistance funds, as well as similar initiatives for low-wage, app-based, and automation-adjacent workers, indicates that the Bargaining in Good Faith Fund has strong potential for success. For example, the Coworker Solidarity Fund provides legal, financial, and strategic support for worker-activists organizing to improve their companies, while the fund invests in ecosystems that increase worker power and improve economic livelihoods and social conditions across the U.S. South.
New York state lawmakers have also set a precedent with their transformative Excluded Workers Fund, which provided direct financial support to workers left out of pandemic relief programs. The $2.1 billion Excluded Workers Fund, passed by the New York state legislature and governor in April 2021, was the first large-scale program of its kind in the country. By examining and building on these successes, we can develop federal policies that better support workers across the country.
A national program requires multiple funding methods, and several mechanisms have been identified to establish the National Bargaining in Good Faith Fund. First, existing policy needs to be strengthened, and companies violating labor laws should face financial consequences. The labor law violation tax, which could be a percentage of a company’s profits or revenue, would be directed to the Bargaining in Good Faith Fund. Additionally, penalties could be imposed on companies that engage in retaliatory behavior, and the funds generated could also be directed to the Bargaining in Good Faith Fund. New legislation from Congress is required to enforce existing federal policy.
Second, as natural allies in the fight to safeguard workers’ rights, labor unions should allocate a portion of their dues toward the fund. By pooling their resources, a portion of union dues could be directed to the federal fund.
Third, a portion of the fees paid into the federal unemployment insurance program should be redirected to Bargaining in Good Faith Fund.
Fourth, existing funding for worker protections, currently siloed in agencies, should be reallocated to support the Bargaining in Good Faith Fund more effectively. To qualify for the fund, workers receiving food assistance and/or Temporary Assistance for Needy Families benefits should be automatically eligible once the NLRB and the EEOC recognize the instance of retaliation. Workers who are not eligible could apply directly to the Fund through a state-appointed agency. This targeted approach aims to support those who face significant barriers to accessing resources and protections that safeguard their rights and well-being due to historical labor exploitation and discrimination.
Several federal agencies could collaborate to oversee the Bargaining in Good Faith Fund, including the Department of Labor, the EEOC, the Department of Justice, and the NLRB. These agencies have the authority to safeguard workers’ welfare, enforce federal laws prohibiting employment discrimination, prosecute corporations that engage in criminal retaliation, and enforce workers’ rights to engage in concerted activities for protection, such as organizing a union.
Conclusion
The federal government has had a policy of supporting worker organizing and collective bargaining since the passage of the National Labor Relations Act in 1935. However, the federal government has not fully implemented its policy over the past 86 years, resulting in negative impacts on BIPOC workers, who face systemic racism in the unionization process and on the job. Additionally, rapid technological advances have resulted in the automation of tasks and changes in the labor market that disproportionately affect workers of color. Consequently, the United States is likely to see an increase in wealth inequality over the next two decades.
The Biden-Harris Administration can act now to promote racial equity by establishing a National Bargaining in Good Faith Fund to support historically disadvantaged workers in unionization efforts. Because this is a pressing issue, a feasible short-term solution is to initiate a pilot program over the next 18 months. It is imperative to establish a policy that acknowledges and addresses the historical disadvantage experienced by these workers and supports their efforts to attain economic equity.
For example, in 2019, the city of Evanston, Illinois, established a fund to provide reparations to Black residents who can demonstrate that they or their ancestors have been affected by discrimination in housing, education, and employment. The fund is financed by a three percent tax on the sale of recreational marijuana and is intended to provide financial assistance for housing, education, and other needs.
Another example is the proposed H.R. 40 bill in the U.S. Congress that aims to establish a commission to study and develop proposals for reparations for African Americans who are descendants of slaves and who have been affected by slavery, discrimination, and exclusion from opportunities. The bill aims to study the impacts of slavery and discrimination and develop proposals for reparations that would address the lingering effects of these injustices, including the denial of education, housing, and other benefits.
The Civil Rights Act of 1964, which banned discrimination on the basis of race, color, religion, sex, or national origin, was challenged in court but upheld by the Supreme Court in 1964.
The Voting Rights Act of 1965, which aimed to eliminate barriers to voting for minorities, was challenged in court several times over the years, with the Supreme Court upholding key provisions in 1966 and 2013, but striking down a key provision in 2013.
The Fair Housing Act of 1968, which banned discrimination in housing, was challenged in court and upheld by the Supreme Court in 1968.
The Affirmative Action policies, which aimed to increase the representation of minorities in education and the workforce, have been challenged in court multiple times over the years, with the Supreme Court upholding the use of race as a factor in college admissions in 2016.
Despite court challenges, policymakers must persist in bringing forth solutions to address racial equity as many complex federal policies aimed at promoting racial equity have been challenged in court over the years, not just on constitutional grounds.
Ensuring Racial Equity in Federal Procurement and Use of Artificial Intelligence
In pursuit of lower costs and improved decision-making, federal agencies have begun to adopt artificial intelligence (AI) to assist in government decision-making and public administration. As AI occupies a growing role within the federal government, algorithmic design and evaluation will increasingly become a key site of policy decisions. Yet a 2020 report found that almost half (47%) of all federal agency use of AI was externally sourced, with a third procured from private companies. In order to ensure that agency use of AI tools is legal, effective, and equitable, the Biden-Harris Administration should establish a Federal Artificial Intelligence Program to govern the procurement of algorithmic technology. Additionally, the AI Program should establish a strict data collection protocol around the collection of race data needed to identify and mitigate discrimination in these technologies.
Researchers who study and conduct algorithmic audits highlight the importance of race data for effective anti-discrimination interventions, the challenges of category misalignment between data sources, and the need for policy interventions to ensure accessible and high-quality data for audit purposes. However, inconsistencies in the collection and reporting of race data significantly limit the extent to which the government can identify and address racial discrimination in technical systems. Moreover, given significant flexibility in how their products are presented during the procurement process, technology companies can manipulate race categories in order to obscure discriminatory practices.
To ensure that the AI Program can evaluate any inequities at the point of procurement, the Office of Science and Technology Policy (OSTP) National Science and Technology Council Subcommittee on Equitable Data should establish guidelines and best practices for the collection and reporting of race data. In particular, the Subcommittee should produce a report that identifies the minimum level of data private companies should be required to collect and in what format they should report such data during the procurement process. These guidelines will facilitate the enforcement of existing anti-discrimination laws and help the Biden-Harris Administration pursue their stated racial equity agenda. Furthermore, these guidelines can help to establish best practices for algorithm development and evaluation in the private sector. As technology plays an increasingly important role in public life and government administration, it is essential not only that government agencies are able to access race data for the purposes of anti-discrimination enforcement—but also that the race categories within this data are not determined on the basis of how favorable they are to the private companies responsible for their collection.
Challenge and Opportunity
Research suggests that governments often have little information about key design choices in the creation and implementation of the algorithmic technologies they procure. Often, these choices are not documented or are recorded by contractors but never provided to government clients during the procurement process. Existing regulation provides specific requirements for the procurement of information technology, for example, security and privacy risks, but these requirements do not account for the specific risks of AI—such as its propensity to encode structural biases. Under the Federal Acquisition Regulation, agencies can only evaluate vendor proposals based on the criteria specified in the associated solicitation. Therefore, written guidance is needed to ensure that these criteria include sufficient information to assess the fairness of AI systems acquired during procurement.
The Office of Management and Budget (OMB) defines minimum standards for collecting race and ethnicity data in federal reporting. Racial and ethnic categories are separated into two questions with five minimum categories for race data (American Indian or Alaska Native, Asian, Black or African American, Native Hawaiian or Other Pacific Islander, and White) and one minimum category for ethnicity data (Hispanic or Latino). Despite these standards, guidelines for the use of racial categories vary across federal agencies and even across specific programs. For example, the Census Bureau classification scheme includes a “Some Other Race” option not used in other agencies’ data collection practices. Moreover, guidelines for collection and reporting of data are not always aligned. For example, the U.S. Department of Education recommends collecting race and ethnicity data separately without a “two or more races” category and allowing respondents to select all race categories that apply. However, during reporting, any individual who is ethnically Hispanic or Latino is reported as only Hispanic or Latino and not any other race. Meanwhile, any respondent who selected multiple race options is reported in a “two or more races” category rather than in any racial group with which they identified.
These inconsistencies are exacerbated in the private sector, where companies are not uniformly constrained by the same OMB standards but rather covered by piecemeal legislation. In the employment context, private companies are required to collect and report on demographic details of their workforce according to the OMB minimum standards. In the consumer lending setting, on the other hand, lenders are typically not allowed to collect data about protected classes such as race and gender. In cases where protected class data can be collected, these data are typically considered privileged information and cannot be accessed by the government. In the case of algorithmic technologies, companies are often able to discriminate on the basis of race without ever explicitly collecting race data by using features or sets of features that act as proxies for protected classes. Facebook’s advertising algorithms, for instance, can be used to target race and ethnicity without access to race data.
Federal leadership can help create consistency in reporting to ensure that the government has sufficient information to evaluate whether privately developed AI is functioning as intended and working equitably. By reducing information asymmetries between private companies and agencies during the procurement process, new standards will bring policymakers back into the algorithmic governance process. This will ensure that democratic and technocratic norms of agency rule-making are respected even as privately developed algorithms take on a growing role in public administration.
Additionally, by establishing a program to oversee the procurement of artificial intelligence, the federal government can ensure that agencies have access to the necessary technical expertise to evaluate complex algorithmic systems. This expertise is crucial not only during the procurement stage but also—given the adaptable nature of AI—for ongoing oversight of algorithmic technologies used within government.
Plan of Action
Recommendation 1. Establish a Federal Artificial Intelligence Program to oversee agency procurement of algorithmic technologies.
The Biden-Harris Administration should create a Federal AI Program to create standards for information disclosure and enable evaluation of AI during the procurement process. Following the two-part test outlined in the AI Bill of Rights, the proposed Federal AI Program would oversee the procurement of any “(1) automated systems that (2) have the potential to meaningfully impact the American public’s rights, opportunities, or access to critical resources or services.”
The goals of this program will be to (1) establish and enforce quality standards for AI used in government, (2) enforce rigorous equity standards for AI used in government, (3) establish transparency practices that enable public participation and political accountability, and (4) provide guidelines for AI program development in the private sector.
Recommendation 2. Produce a report to establish what data are needed in order to evaluate the equity of algorithmic technologies during procurement.
To support the AI Program’s operations, the OSTP National Science and Technology Council Subcommittee on Equitable Data should produce a report to establish guidelines for the collection and reporting of race data that balances three goals: (1) high-quality data for enforcing existing anti-discrimination law, (2) consistency in race categories to reduce administrative burdens and curb possible manipulation, and (3) prioritizing the needs of groups most affected by discrimination. The report should include opportunities and recommendations for integrating its findings into policy. To ensure the recommendations and standards are instituted, the President should direct the General Services Administration (GSA) or OMB to issue guidance and request that agencies document how they will ensure new standards are integrated into future procurement vehicles. The report could also suggest opportunities to update or amend the Federal Acquisition Regulations.
High-Quality Data
The new guidelines should make efforts to ensure the reliability of race data furnished during the procurement process. In particular:
- Self-identification should be used whenever possible to ascertain race. As of 2021, Food and Nutrition Service guidance recommends against the use of visual identification based on reliability, respect for respondents’ dignity, and feedback from Child and Adult Care Food Program) and Summer Food Service Program participants.
- The new guidelines should attempt to reduce missing data. People may be reluctant to share race information for many legitimate reasons, including uncertainty about how personal data will be used, fear of discrimination, and not identifying with predefined race categories. These concerns can severely impact data quality and should be addressed to the extent possible in the OMB guidelines. New York’s state health insurance marketplace saw a 20% increase in response rate for race by making several changes to the way they collect data. These changes included explaining how the data would be used and not allowing respondents to leave the question blank but instead allowing them to select “choose not to answer” or “don’t know.” Similarly, the Census Bureau found that a single combined race and ethnicity question improved data quality and consistency by reducing the rate of “some other race,” missing, and invalid responses as compared with two separate questions (one for race and one for ethnicity).
- The new guidelines should follow best practices established through rigorous research and feedback from a variety of stakeholders. In June 2022, the OMB announced a formal review process to revise Statistical Policy Directive No. 15: Standards for Maintaining, Collecting, and Presenting Federal Data on Race and Ethnicity. While this review process is intended for the revision of federal data requirements, its findings can help inform best practices for collection and reporting requirements for nongovernmental data as well.
Consistency in Data Reporting
Whenever possible and contextually appropriate, the guidelines for data reporting should align with the OMB guidelines for federal data reporting to reduce administrative burdens. However, the report may find that other data is needed that goes beyond the OMB guidelines for the evaluation of privately developed AI.
Prioritizing the Needs of Affected Groups
In their Toolkit for Centering Racial Equity Throughout Data Integration, the Actionable Intelligence for Social Policy group at the University of Pennsylvania identifies best practices for ensuring that data collection serves the groups most affected by discrimination. In particular, this toolkit emphasizes the need for strong privacy protections and stakeholder engagement. In their final report, the Subcommittee on Equitable Data should establish protocols to secure data and for carefully considered role-based access to it.
The final report should also engage community stakeholders in determining which data should be collected and establish a plan for ongoing review that engages with relevant stakeholders, prioritizing affected populations and racial equity advocacy groups. The report should evaluate the appropriate level of transparency in the AI procurement process, in particular, trade-offs between desired levels of transparency and privacy.
Conclusion
Under existing procurement law, the government cannot outsource “inherently governmental functions.” Yet key policy decisions are embedded within the design and implementation of algorithmic technology. Consequently, it is important that policymakers have the necessary resources and information throughout the acquisition and use of procured AI tools. A Federal Artificial Intelligence Program would provide expertise and authority within the federal government to assess these decisions during procurement and to monitor the use of AI in government. In particular, this would strengthen the Biden-Harris Administration’s ongoing efforts to advance racial equity. The proposed program can build on both long-standing and ongoing work within the federal government to develop best practices for data collection and reporting. These best practices will not only ensure that the public use of algorithms is governed by strong equity and transparency standards in the public sector but also provide a powerful avenue for shaping the development of AI in the private sector.
Algorithmic Transparency Requirements for Lending Platforms Using Automated Decision Systems
Now is the time to ensure lending models offered by private companies are fair and transparent. Access to affordable credit greatly impacts quality of life and can potentially impact housing choice. Over the past decade, algorithmic decision-making has increasingly impacted the lives of American consumers. But it is important to ensure all forms of algorithmic underwriting are open to review for fairness and transparency, as inequities may appear in either access to funding or in credit terms. A recent report released by the U.S. Treasury Department speaks to the need for more oversight in the FinTech market.
Challenge and Opportunity
The financial services sector, a historically non-technical industry, has recently and widely adopted automated platforms. Financial technology, known as “FinTech”, offers financial products and services directly to consumers by private companies or in partnership with banks and credit unions. These platforms use algorithms that are non-transparent but directly affect Americans’ ability to obtain affordable financing. Financial institutions (FIs) and mortgage brokers use predictive analytics and artificial intelligence to evaluate candidates for mortgage products, small business loans, and unsecured consumer products. Some lenders underwrite personal loans such as auto loans, personal unsecured loans, credit cards, and lines of credit with artificial intelligence. Although loans that are not government-securitized receive less scrutiny, access to credit for personal purposes impacts the debt-to-income ratios and credit scores necessary to qualify for homeownership or the global cash flow of a small business owner. Historic Home Mortgage Disclosure Act (HMDA) data and studies on small business lending demonstrate that disparate access to mortgages and small business loans occurs. This scenario will not be improved through unaudited decision automation variables, which can create feedback loops that hold the potential to scale inequities.
Forms of discrimination appear in credit approval software and can hinder access to housing. Lorena Rodriguez writes extensively about the current effect of technology on lending laws regulated by the Fair Housing Act of 1968, pointing out that algorithms have incorporated alternative credit scoring models into their decision trees. These newly selected variables have no place in determining someone’s creditworthiness. Inputs include factors like social media activity, retail spending activity, bank account balances, college of attendance, or retail spending habits.
Traditional credit scoring models, although cumbersome, are understandable to the typical consumer who takes the time to understand how to impact their credit score. However, unlike credit scoring models, lending platforms can input a data variable with no requirement to disclose the models that impact decisioning. In other words, a consumer may never understand why their loan was approved or denied, because models are not disclosed. At the same time, it may be unclear which consumers are being solicited for financing opportunities, and lenders may target financially vulnerable consumers for profitable but predatory loans.
Transparency around lending decision models is more necessary now than ever. The COVID-19 pandemic created financial hardship for millions of Americans. The Federal Reserve Bank of New York recently reported all-time highs in American household debt. In a rising interest rate environment, affordable and fair credit access will become even more critical to help households stabilize. Although artificial intelligence has been in use for decades, the general public is only recently beginning to realize the ethical impacts of its uses on daily life. Researchers have noted algorithmic decision-making has bias baked in, which has the potential to exacerbate racial wealth gaps and resegregate communities by race and class. While various agencies—such as the Consumer Financial Protection Bureau (CFPB), Federal Trade Commission (FTC), Financial Crimes Enforcement Network, Securities and Exchange Commission, and state regulators—have some level of authority over FinTech companies, there are oversight gaps. Although FinTechs are subject to fair lending laws, not enough is known about disparate impact or treatment, and regulation of digital financial service providers is still evolving. Modernization of policy and regulation is necessary to keep up with the current digital environment, but new legislation can address gaps in the market that existing policies may not cover.
Plan of Action
Three principles should guide policy implementation around FinTech: (1) research, (2) enforcement, (3) incentives. These principles balance oversight and transparency while encouraging responsible innovation by community development financial institutions (CDFIs) and charitable lenders that may lead to greater access to affordable credit. Interagency cooperation and the development of a new oversight body is critical because FinTech introduces complexity due to technical, trade, and financial services overlap.
Recommendation 1: Research. The FTC should commission a comprehensive, independent research study to understand the scope and impact of disparate treatment in FinTech lending.
To ensure equity, the study should be jointly conducted by a minimum of six research universities, of which at least two must be Historically Black Colleges and Universities, and should be designed to understand the scope and impact of fintech lending. A $3.5 million appropriation will ensure a well-designed, multiyear study. A strong understanding of the landscape of FinTech and its potential for disparate impact is necessary. Many consumers are not adequately equipped to articulate their challenges, except through complaints to agencies such as the Office of the Comptroller of Currency (OCC) and the CFPB. Even in these cases, the burden of responsibility is on the individual to be aware of channels of appeal. Anecdotal evidence suggests BIPOC borrowers and low-to-moderate income (LMI) consumers may be the target of predatory loans. For example, an LMI zip code may be targeted with FinTech ads, while product terms may be at a higher interest rate. Feedback loops in algorithms will continue to identify marginalized communities as higher risk. A consumer with lesser means who also receives a comparative triple-interest rate will remain financially vulnerable due to extractive conditions.
Recommendation 2: Enforcement. A suite of enforcement mechanisms should be implemented.
- FinTechs engaged in mortgage lending should be subject to Home Mortgage Disclosure Act (HMDA) reporting on lending activity and Community Reinvestment Act (CRA) examination. When a bank utilizes a FinTech1, a vendor CRA assessment should be incorporated into the bank’s own examination process. Credit unions should also be required to produce FinTech vendor CRA exams during their examination process. CRA and HMDA requirements would encourage FinTech to make sure they are lending broadly.
- Congress should codify’ FinTechs’ role as the “true lender” whenever a FinTech’s underwriting model is used by an FI partner to clarify FinTech responsibility to all applicable state, local, and federal interest caps, fair lending laws, etc., as well as liability when they do not meet existing standards. Federal regulatory agency guidelines must also be updated to clarify the bank or credit union’s Fintech partner’s shared responsibility when a FinTech model for underwriting violates UDAAP or fair lending guidelines.
- A previously proposed OCC FinTech charter should be adopted but made optional. However, when a FinTech chooses to adopt the OCC charter, the charter should give FinTechs interstate privileges covered under the Reigle-Neal Interstate Banking and Branch Efficiency Act of 1994. This provision should also require FinTechs to fulfill state licensing requirements in each state in which they operate, eliminating their current ability to bypass licensing by partnering with regulated FIs.
- Companies engaged in any financing activity or providing automated lending software to regulated FIs must be required to disclose decision models to the FI’s examiner upon request. FinTech data disclosure must not be limited to federally secured loans such as small business or mortgage loans but include secured and unsecured loan products made to consumers such as auto, personal, and small dollar loans. When consumers obtain a predatory product in these categories, the loans can have a severe impact on debt-to-income/back-end ratios and credit scores of borrowers, preventing them from obtaining homeownership or causing them to receive less favorable terms.
Recommendation 3: Incentives. Develop an ethical FinTech certification that denotes a FinTech as responsible lender, such as modeled by the U.S. Treasury’s CDFI certification.
The certification can sit with the U.S. Treasury and should create incentives for FinTechs demonstrated to be responsible lenders in forms such as grant funding, procurement opportunities, or tax credits. To create this certification, FI regulatory agencies, with input from the FTC and National Telecommunications and Information Administration, should jointly develop an interagency menu of guidelines that dictate acceptable parameters for what criteria may be input into an automated decision model for consumer lending. Guidelines should also dictate what may not be used in a lending model (example: college of attendance). Exceptions to guidelines must be documented, reviewed, and approved by the oversight body after being determined to be a legitimate business necessity.
Conclusion
Now is the time to provide policy guidance that will prevent disparate impact and harm to minority, BIPOC, and other traditionally marginalized communities as a result of algorithmically informed biased lending practices.
Yes, but the CFPB’s general authority to do so is regularly challenged as a result of its independent structure. It is not clear if its authority extends to all forms of algorithmic harm, as its stated authority to regulate FinTech consumer lending is limited to mortgage and payday lending. UDAAP oversight is also less clear, as it pertains to nonregulated lenders. Additionally, the CFPB has the authority to regulate institutions over $10 billion. Many FinTechs operate below this threshold, leaving oversight gaps. Fair lending guidance through financial technology must be codified apart from the CFPB, although some oversight may continue to rest with the CFPB.
Precedent is currently being set for regulation of small business lending data through the CFPB’s enforcement of Section 1071 of the Dodd-Frank Act. Regulation will require financial disclosure of small business lending data. Other government programs, such as the CDFI fund, currently require transaction-level reporting for lending data attached to federal funding. Over time, private company vendors are likely to develop tools to support reporting requirements around lending. Data collection can also be incentivized through mechanisms like certifications or tax credits for responsible lenders that are willing to submit data.
The OCC has proposed a charter for FinTechs that would subject them to regulatory oversight (see policy recommendation). Other FI regulators have adopted various versions of FinTech oversight. Oversight for FinTech-insured depository partnerships should remain with a primary regulatory authority for the depository with support from overarching interagency guidance.
A new regulatory body with enforcement authority and congressional appropriations would be ideal, since FinTech is a unique form of lending that touches issues that impact consumer lending, regulation of private business, and data privacy and security.
This argument is often used by payday lenders that offer products with egregious, predatory interest rates. Not all forms of access to credit are responsible forms of credit. Unless a FinTech operates as a charitable lender, its goal is profit maximization—which does not align well with consumer protection. In fact, research indicates financial inclusion promises in FinTech fall short.
Many private lenders are regulated: Payday lenders are regulated by the CFPB once they reach a certain threshold. Pawn shops and mortgage brokers are subject to state departments for financial regulation. FinTechs also have the unique potential to have a different degree of harm because their techniques of automation and algorithmic evaluation allow for scalability and can create reinforcing feedback loops of disparate impact.
Creating Equitable Outcomes from Government Services through Radical Participation
Government policies, products, and services are created without the true and full design participation and expertise of the people who will use them–the public: citizens, refugees, and immigrants. As a result, the government often replicates private sector anti-patterns1, using or producing oppressive, disempowering, and colonial policies through products and services that embody bias, limit access, create real harm, and discriminate against underutilized communities2 on the basis of various identities violating the President’s Executive Order on Equity. Examples include life-altering police use of racially and sexually biased facial recognition products, racial discrimination in the delivery access of life-saving Medicaid services and SNAP benefits, and racist child welfare service systems.
The Biden-Harris Administration should issue an executive order to embed Radical Participatory Design (RPD) into the design and development of all government policies, products, and services, and to require all federally-funded research to use Radical Participatory Research (RPR). Using an RPD and RPR approach makes the Executive Order on Racial Equity, Executive Order on Transforming the Customer Experience, and the Executive Order on DEIA more likely to succeed. Using RPD and RPR as the implementation strategy is an opportunity to create equitable social outcomes by embodying equity on the policy, product and service design side (Executive Order on Racial Equity), to improve the public’s customer experience of the government (Executive Order on Transforming the Customer Experience, President’s Management Agenda Priority 2), and to lead to a new and more just, equitable, diverse, accessible, and inclusive (JEDAI) future of work for the federal government (Executive Order on DEIA).
Challenge and Opportunity
The technology industry is disproportionately white and male. Compared to private industry overall, whites, men, and Asians are overrepresented while Latinx people, Black people, and women are underrepresented. Only 26% of technology positions in the U.S. are held by women though they represent 57% of the US workforce. Even worse, women of color hold 4% of technology positions even though they are 16% of the population. Similarly, Black Americans are 14% of the population but hold 7% of tech jobs. Latinx Americans only hold 8% of tech jobs while comprising 19% of the population. This representation decreases even more as you look at leadership roles in technology. In FY2020, the federal government spent $392.1 billion contracting services, including services to build products. Latinx, African Americans, Native Americans, and women are underrepresented in the contractor community.
The lack of diversity in designers and developers of the policies, products, and services we use leads to harmful effects like algorithmic bias, automatic bathroom water and soap dispensers that do not recognize darker skin, and racial bias in facial recognition (mis)identification of Black and Brown people.
With a greater expectation of equity from government services, the public experiences greater disappointment when government policies, services, and products are biased, discriminatory, or harmful. Examples include inequitable public school funding services, race and poverty bias in child welfare systems, and discriminatory algorithmic hiring systems used in government.
The federal government has tried to improve the experience of its products and services through methodologies like Human-centered Design (HCD). In HCD, the design process is centered on the community who will use the design, by first conducting research interviews or observations. Beyond the research interactions with community members, designers are supposed to carry empathy for the community all the way through the design, development, and launch process. Unfortunately, given the aforementioned negative outcomes of government products and services for various communities, empathy often is absent. What empathy may be generated does not persist long enough to influence or impact the design process. Ultimately, individual appeals to empathy are inadequate at generating systems level change. Scientific studies show that white people, who make up the majority of technologists and policy-makers, have a reduced capacity for empathy for people of other and similar backgrounds. As a result, the push for equity remains in government services, products, and policies, leading to President Biden’s Executive Order on Advancing Racial Equity and Support for Underserved Communities and, still, again, with the Executive Order on Further Advancing Racial Equity and Support for Underserved Communities.
The federal government lacks processes to embed empathy throughout the lifecycle of policy, product, and service design, reflecting the needs of community groups. Instead of trying to build empathy in designers who have no experiential knowledge, we can create empathetic processes and organizations by embedding lived experience on the team.
Radical Participatory Design (RPD) is an approach to design in which the community members, for whom one is designing, are full-fledged members on the research, design, and development team. In traditional participatory design, designers engage the community at certain times and otherwise work, plan, analyze, or prepare alone before and after those engagements. In RPD, the community members are always there because they are on the team; there are no meetings, phone calls, or planning without them.
RPD has a few important characteristics. First, the community members are always present and leading the process. Second, the community members outnumber the professional designers, researchers, or developers. Third, the community members own the artifacts, outcomes, and narratives around the outcomes of the design process. Fourth, community members are compensated equitably as they are doing the same work as professional designers. Fifth, RPD teams are composed of a qualitatively representative sample (including all the different categories and types of people) of the community.
Embedding RPD in the government connects the government to a larger movement toward participatory democracy. Examples include the Philadelphia Participatory Design Lab, the Participatory City Making Lab, the Center for Lived Experience, the Urban Institute’s participatory Resident Researchers, and Health and Human Service’s “Methods and Emerging Strategies to Engage People with Lived Experience.” Numerous case studies show the power of participatory design to reduce harm and improve design outcomes. RPD can maximize this by infusing equity as people with lived experience both choose, check, and direct the process.
As the adoption of RPD increases across the federal government, the prevalence and incidence of harm, bias, trauma, and discrimination in government products and services will decrease, aiding the implementation of the executive orders on Advancing Racial Equity and Support for Underserved Communities and Further Advancing Racial Equity and Support for Underserved Communities, and ensuring the OSTP AI Bill of Rights for AI products and services. Additionally, RPR aligns with OSTP’s actions to advance open and equitable research. Second, the reduction of harm, discrimination, and trauma improves the customer experience (CX) of government services aiding the implementation of the Executive Order on Transforming the Customer Experience, the President’s Management Agenda Priority 2, and the CAP goal on Customer Experience. An improved CX will increase community adoption, use, and engagement with potentially helpful and life-supporting government services that underutilized people need. RPD highlights the important connection between equity and CX and creates a way to link the two executive orders. You cannot claim excellent CX when the CX is inequitable and entire underutilized segments of the public have a harmful experience.
Third, instead of seeking the intersection of business needs and user needs like in the private sector, RPD will move the country closer to its democratic ideals by equitably aligning the needs of the people with the needs of the government of the people, by the people, and for the people. There are various examples where the government acts like a separate entity completely unaligned to the will of a majority of the public (gun control, abortion). Project by project, RPD helps align the needs of the people and the needs of the government of the people when representative democracy does not function properly.
Fourth, all community members, from all walks of life, brought into government to do participatory research and design will gain or refine skills they can then use to stay in government policy, product, and service design or to get a job outside of government. The workforce outcomes of RPD further diversify policy, product, and service designers and researchers both inside and outside the federal government, aligning with the Executive Order on DEIA in the Federal Workforce.
Plan of Action
The use of RPD and RPR in government is the future of participatory government and a step towards truly embodying a government of the people. RPD must work at the policy level as well, as policy directs the creation of services, products, and research. Equitable product and service design cannot overcome inequitable and discriminatory policy. The following recommended actions are initial steps to embody participatory government in three areas: policy design, the design and development of products and services, and funded research. Because all three areas occur across the federal government, executive action from the White House will facilitate the adoption of RPD.
Policy Design
An executive order from the president should direct agencies to use RPD when designing agency policy. The order should establish a new Radical Participatory Policy Design Lab (RPPDL) for each agency with the following characteristics:
- Embodies a qualitatively representative sample of the public target audience impacted by the agency
- Includes a qualitatively representative sample of agency employees who are also impacted by agency policy
- Designs policy through this radical participatory design team
- Sets budget policy through participatory budgeting (Grand Rapids, NYC, Durham, and HUD examples)
- Assesses agency programs that affect the public through participatory appraisals and participatory evaluations
- Rotates community policy designers in to the lab and out of the lab on six-month renewable terms
- Community policy designers receive equitable compensation for their time
- Community policy designers can be offered jobs to stay in government based on their policy experience, or the office that houses the RPPDL will assist community policy designers in finding roles outside of government based on their experience and desire
- An RPD authorization to allow government policy employees to compensate community members outside of a grant, cooperative agreement, or contract (GSA TTS example)
The executive order should also create a Chief Experience Officer (CXO) for the U.S. as a White House role. The Office of the CXO (OCXO) would coordinate CX work across the government in accordance with the Executive Order on Transforming the CX, the PMA Priority 2, the CX CAP goal, and the OMB Circular A-11 280. The executive order would focus the OCXO on the work of coordinating, approving, advising the RPD work across the federal government, including the following initiatives:
- Improve the public experience of high-impact, trans-agency journeys by managing the Office of Management and Budget (OMB) life experience projects
- Facilitate a CXO council of all federal agency CXOs.
- Advise various agency CXOs and other officials on embedding RPD into their policy, service, and product design and development work.
- Work with agencies to recruit and create a list of civil society organizations who are willing to help recruit community members for RPD and RPR projects.
- Recruit RPD public team members and coordinate the use of RPD in the creation of White House policy.
- Coordinate with the director of OMB and the Equitable Data Working Group to create
- an equity measure of the social outcomes of the government’s products, services, and policies,
- a public CX measurement of the entire federal government.
- Serve as a member of the White House Steering Committee on Equity established by the Executive Order on Further Advancing Equity.
- Serve as a member of the Equitable Data Working Group established by the Executive Order on Advancing Racial Equity.
- Strategically direct the work of the OCXO in order to improve the equity and CX metrics.
- Embed equity measures in CX measurement and data reporting template required by the OMB Circular A-11 280. CX success requires healthy, equitable CX across various subgroups, including underutilized communities, connecting the Executive Order on Transforming the CX to the Executive Order on Advancing Racial Equity.
- Update the OMB Circular A-11 280’s CX Capacity Assessment tool and the Action Plan template to include equity as a central component.
- Evaluate and assess the utilization of RPD in policy, product, and service design by agencies across the government.
Due to the distributed nature of the work, the funding for the various RPPDLs and the OCXO should come from money the director of OMB has identified and added to the budget the President submits to Congress, according to Section 6 of the Executive Order on Advancing Racial Equity. Agencies should also utilize money appropriated by the Agency Equity Teams required by the Executive Order on Further Advancing Racial Equity.
Product and Service Design
The executive order should mandate that all research, design, and delivery of agency products and services for the public be done through RPR and RPD. RPD should be used both for in-house and contracted work through grants, contracts, or cooperative agreements.
On in-house projects, funding for the RPD team should come from the project budget. For grants, contracts, and cooperative agreements, funding for the RPD team should come from the acquisition budget. As a result, the labor costs will increase since there are more designers on the project. The non-labor component of the project budget will be less. A slightly lower non-labor project budget is worth the outcome of improved equity. Agency offices can compensate for this by requesting a slightly higher project budget for in-house or contracted design and development services.
In support of the Executive Order on Transforming the CX, the PMA Priority 2, and the CX CAP goal, OMB should amend the OMB Circular A-11 280 to direct High Impact Service Providers (HISPs) to utilize RPD in their service work.
- HISPS must embed RPD in their product and service research, design, development, and delivery.
- HIPSs must include an equity component in their CX Capacity Assessment and CX Action Plan in line with guidance from the CXO of the U.S.
- Following applicable laws, HISPs should let customers volunteer demographic information during customer experience data collection in order to assess the CX of various subgroups.
- Agency annual plans should include both CX and equity indicator goals.
- Equity assessment data and CX data for various subgroups and underutilized communities must be reported in the OMB-mandated data dashboard.
- Each agency should create an RPD authorization to allow government employees and in-house design teams to compensate community members outside of a grant, cooperative agreement, or contract (GSA TTS example).
OSTP should add RPD and RPD case studies as example practices in OSTP’s AI Bill of Rights. RPD should be listed as a practice that can affect and reinforce all five principles.
Funded Research
The executive order should also mandate that all government-funded, use-inspired research about communities or intended to be used by people or communities, should be done through RPR. In order to determine if a particular intended research project is use-inspired, the following questions should be asked by the government funding agency prior to soliciting researchers:
- For technology research, is the technology readiness level (TRL) 2 or higher?
- Is the research about people or communities?
- Is the research intended to be used by people or communities?
- Is the research intended to create, design, or guide something that will be used by people and communities?
If the answer to any of the questions is yes, the funding agency should require the funded researchers to use an RPR approach.
Funding for the RPR team comes from the research grant or funding. Researchers can use the RPR requirement to estimate how much funding should be requested in the proposal.
OSTP should add RPR and the executive order to their list of actions to advance open and equitable research. RPR should be listed as a key initiative of the year of Open Science.
Conclusion
In order to address inequity, the public’s lived experience should lead the design and development process of government products and services. Because many of those products and services are created to comply with government policy, we also need lived experience to guide the design of government policy. Embedding Radical Participatory Design in government-funded research as well as policy, products, and services reduces harm, creates equity, and improves the public customer experience. Additionally, RPD connects and embeds equity in CX, moves us toward our democratic ideals, and creatively addresses the future of work by diversifying our policy, product, and service design workforce.
Because we do not physically hold digital products, the line between a software product and a software service is thin. Usually, a product is an offering or part of an offering that involves one interaction or touchpoint with a customer. In contrast, a service involves multiple touchpoints both online and offline, or multiple interactions both digital and non-digital.
For example, Google Calendar can be considered a digital product. A product designer for Google Calendar might work on designing its software interface, colors, options, and flows. However, a library is a service. As a library user, you might look for a book on the library website. If you can’t find it, you might call the library. The librarian might ask you to come in. You go in and work with the librarian to find the book. After realizing it is not there, the librarian might then use a software tool to request a new book purchase. Thus, the library service involved multiple touchpoints, both online and offline: a website, a phone line, an in-person service in the physical library, and an online book procurement tool.
Most of the federal government’s offerings are services. Examples like Medicare, Social Security, and veterans benefits involve digital products, in-person services in a physical building, paper forms, phone lines, email services, etc. A service designer designs the service and the mechanics behind the service in order to improve both the customer experience and the employee experience across all touchpoints, offline and online, across all interactions, digital and non-digital.
Participatory design (PD) has many interpretations. Sometimes PD simply means interviewing research participants. Because they are “participants,” by being interviewees, the work is participatory. Sometimes, PD means a specific activity or method that is participatory. Sometimes practitioners use PD to mean a way of doing an activity. For example, we can do a design studio session with just designers, or we can invite some community members to take part for a 90-minute session. PD can also be used to indicate a methodology. A methodology is a collection of methods or activities; or a methodology is a philosophy or guiding philosophy or principles that help you choose a particular method or activity at a particular point in time or in a process.
In all the above ways of interpreting PD, there are times when the community is present and times when they are not. Moreover, the community members are never leading the process.
Radical comes from the Latin word “radix” meaning root. RPD means design in which the community participates “to the root,” fully, completely, from beginning to end. There are no times, planning, meetings, or phone calls where the community is not present because the community is the team.
Peer review is similar to an Institutional Review Board (IRB). A participatory version of this could be called a Community Review Board (CRB). The difficulty is that a CRB can only reject a research plan; a CRB does not create the proposed research plans. Because a CRB does not ensure that great research plans are created and proposed, it can only reduce harm. It cannot create good.
Equality means treating people the same. Equity means treating people differently to achieve equal outcomes. CRBs achieve equality only in approving power, by equally including community members in the approving process. CRBs fail to achieve equity in social outcomes of products and services because community members are missing in the research plan creation process, research plan implementation process, and the development process of policy, products, and services where inequity can enter. To achieve equal outcomes, equity, their lived experiential knowledge is needed throughout the entire process and especially in deciding what to propose to a CRB.
Still a CRB can be a preliminary step before RPR. Unfortunately, IRBs are only required for US government-funded research with human subjects. In practice, it is not interpreted to apply to the approval of design research for policy, products, and services, even when the research usually includes human subjects. The application of participatory CRBs to approve all research–including design research for policy, products, and services–can be an initial step or a pilot.
A good analogy is that of cooking. It is quite helpful for everyone to know how to cook. Most of us cook in some capacity. Yet, there are people who attend culinary school and become chefs or cooks. Has the fact that individual people can and do cook eliminated the need for chefs? No. Chefs and cooks are useful for various situations – eating at a restaurant, catering an event, the creation of cookbooks, lessons, etc.
The main idea is that the chefs have mainstream institutional knowledge learned from books and universities or cooking schools. But that is not the only type of knowledge. There is also lived, experiential knowledge as well as community, embodied, relational, energetic, intuitive, aesthetic, and spiritual knowledge. It is common to meet amazing chefs who have never been to a culinary school but simply learned to cook through lived experience of experimentation and having to cook everyday for X people. Some learned to cook through relational and community knowledge passed down in their culture through parents, mothers, and aunties. Sometimes, famous chefs will go and learn the knowledge of a particular culture from people who did not go to a learning school. The chefs will appropriate the knowledge and then create a cookbook to sell marketing a fusion cuisine, infused with the culture whose culinary knowledge they appropriated.
Similarly, everyone designs. It is not enough to be tech-savvy or an innovation and design expert. The most important knowledge to have is the lived experiential, community, relational, and embodied knowledge of the people for whom we are designing. When lived experience leads, the outcomes are amazing. Putting lived experience alongside professional designers can be powerful as well. Professional designers are still needed, as their knowledge can help improve the design process. Professionals just cannot lead, lead alone, or be the only knowledge base because inequity enters the system more easily.
To realize the ambitions of this policy proposal, full-time teams will be needed. The RPPDLs who are designing policy are full-time roles due to the amount and various levels of policy to design. For products and services, however, some RPD teams may be part-time. For example, improving an existing product or service may be one of many work projects a government team is conducting. So if they are only working on the project 50% of the time, they may only require a group of part-time community members. On the other hand, the work may require full-time work for RPD team members for the design and development of a greenfield or new product or service that does not exist. Full-time projects will need full-time community members. For part-time projects, community members can work on multiple projects to reach full-time capacity.
Team members can receive non-monetary compensation like a gift card, wellness services, or child care. However, it is best practice to allow the community member to choose. Most will choose monetary compensation like grants, stipends, or cash payments.
Ultimately they should be paid at a level equal to that of the mainstream institutional experts (designers and developers) who are being paid to do the same work alongside the community members. Remember to compensate them for travel and child care when needed.
RPD is an opportunity for the government to lead the way. The private sector can make money without equitably serving everyone, so it has no incentive to do so. Nonprofits do not carry the level of influence the federal government carries. The federal government has more money to engage in this work than state or local governments. The federal government has a mandate to be equitable in its products and services and their delivery, and if this goes well, the government can make a law mandating organizations in the private and nonprofit sector to do the same work to transform. The government has a long history of using policy and services to discriminate against various underutilized groups. So the federal government should be the first one to use RPD to move towards equity. Ultimately the federal government has a huge influence on the lives of citizens, immigrant residents, and refugees, and the opportunity is great to move us toward equity.
Embedding RPD in government products and services should also be done at the state and local level. Each level will require different memos due to the different mechanics, budgets, dynamics, and policies. The hope is that RPD work at the federal government can help spark RPD work at various state, local, and county governments.
Possible first steps include:
- Mandate that all use-inspired research, including design research for policy, products, and services, be reviewed by a Community Review Board (CRB) for approval.
- If not approved, the research, design, and development cannot move forward.
- Only mandate all government-funded, use-inspired research be conducted using RPR. Focusing on research funding alone shifts the payment of RPR community teams to the grant recipients, only.
- Mandate all government-funded, use-inspired research use RPR and all contracted research, design, development, and delivery of government products and services uses RPD.
- Focusing on research funding and contracted product and service work shifts the payment of RPR and RPD community team members to the grant recipients, vendors, and contract partners.
- Choose a pilot agency, like NIH, to start.
- Start with all HISPs instead of all federal government agencies.
Use RPD and RPR as the implementation strategy for only implementing the Executive Order on Transforming the Customer Experience, which focuses on the HISPs.
- Start with a high-profile set of projects such as the OMB life experience projects.
Then, later we can advance to an entire pilot agency.
- Focus on embedding equity measures in CX.
After equity is embedded in CX, start by choosing a pilot agency, benchmarking equity and CX, piloting RPD, and measuring the change attributable to RPD.
This allows time to build more evidence.
There are many existing case studies of participatory design.
- Decolonizing Participatory Design: Memory Making in Namibia
- Toward a more just library: Participatory design with Native American students
- Crossing Methodological Borders: Decolonizing Community-Based Participatory Research
- Different eyes/open eyes
- A Case Study Measuring the Impact of a Participatory Design Intervention on System Complexity and Cycle Time in an Assemble-to-Order System
Also there are also case studies of participatory design in the public sector.
In modern product and service development, products and services never convert into an operations and maintenance phase alone. They are continually being researched, designed, and developed due to continuous changes in human expectations, migration patterns, technology, human preferences, globalization, etc. If community members were left out of research, design, and development work after a service or product launches, then the service or product would no longer be designed and developed using an RPD approach. As long as the service or product is active and in service, radical participation in the continuous research, design, and development is needed.
Protecting Civil Rights Organizations and Activists: A Policy Addressing the Government’s Use of Surveillance Tools
In the summer of 2020, some 15 to 26 million people across the country participated in protests against the tragic killings of Black people by law enforcement officers, making it the largest movement in US history. In response, local and state government officials and federal agencies deployed surveillance tools on protestors in an unprecedented way. The Department of Homeland Security used aerial surveillance on protesters across 15 cities, and several law enforcement agencies engaged in social media monitoring of activists. But there is still a lot the public does not know, such as what other surveillance tactics were used during the protests, where this data is being stored, and for what future purpose.
Government agencies have for decades secretly used surveillance tactics on individual activists, such as during the 1950s when the FBI surveilled human rights activists and civil rights organizations. These tactics have had a detrimental effect on political movements, causing people to forgo protesting and activism out of fear of such surveillance. The First Amendment protects freedom of speech and the right to assemble, but allowing government entities to engage in underground surveillance tactics strips people of these rights.
It also damages people’s Fourth Amendment rights. Instead of agencies relying on the court system to get warrants and subpoenas to view an individual’s online activity, today some agencies are entering into partnerships with private companies to obtain this information directly. This means government agencies no longer have to meet the bare minimum of having probable cause before digging into an individual’s private data.
This proposal offers a set of actions that federal agencies and Congress should implement to preserve the public’s constitutional rights.
- Federal agencies should disclose what technologies they are using, how they are using them, and the effect on civil rights. The Department of Justice should use this information to investigate agencies and ensure their practices aren’t violating the public’s civil rights,
- The Office of Science and Technology Policy and the Department of Justice should work with the Office of the Attorney General to revise Attorney General Guidelines for the FBI.
- Congress should pass the Fourth Amendment Is Not For Sale Act.
- Congress should amend the Stored Communications Act of 1986 to compel companies to ensure user data isn’t sold to third parties who will then sell user data to government entities.
- Congress should pass border search exception legislation.
Challenges and Opportunities
Government entities have been surveilling activists and civil rights organizations long before the 2020 protests. Between 1956 and 1971, the FBI engaged in surveillance tactics to disrupt, discredit, and destroy many civil rights organizations, such as the Black Panther Party, American Indian Movement, and the Communist Party. Some of these tactics included illegal wiretaps, infiltration, misinformation campaigns, and bugs. This program was known as COINTELPRO, and the FBI’s goal was to destroy organizations and activists who had political agendas that they viewed as radical and would challenge “the existing social order.” While the FBI didn’t completely achieve this goal, their efforts did have detrimental effects on activist communities, as members were imprisoned or killed for their activist work, and membership in organizations like the Black Panther Party significantly declined and eventually dissolved in 1982.
After COINTELPRO was revealed to the public, reforms were put in place to curtail the FBI’s surveillance tactics against civil rights organizations, but those reforms were soon rolled back after the September 11 attacks. Since 9/11, it has been revealed, mostly through FOIA requests, that the FBI has surveilled the Muslim community, Occupy Wall Street, Standing Rock protesters, murder of Freddie Gray protesters, Black Lives Matter protests, and more. Today, the FBI has more technological tools at their disposal that make mass surveillance and data collection on activist communities incredibly easy.
In 2020, people across the country used social media sites like Facebook to increase engagement and turnout in local Black Lives Matters protests. The FBI’s Joint Terrorism Task Forces responded by visiting people’s homes and workplaces to question them about their organizing, causing people to feel alarmed and terrified. U.S. Customs and Border Protection (CBP) also got involved, deploying a drone over Minneapolis to provide live video to local law enforcement. The Acting Secretary of CBP also tweeted out that CBP was working with law enforcement agencies across the nation during the 2020 Black Lives Matter Protests. CBP involvement in civil rights protests is incredibly concerning given its ability to circumvent the Fourth Amendment and conduct warrantless searches due to the border search exception. (Federal regulations and federal law gives CBP the authority to conduct warrantless searches and seizures within 100 miles of the U.S. border, where approximately two-thirds of the U.S. population resides.)
The longer government agencies are allowed to surveil people who are simply organizing for progressive policies, the more people will be terrified to voice their opinion about the state of affairs in the United States. This has had detrimental effects on people’s First and Fourth Amendment rights and will continue to have even more effects as technology improves and government entities have access to advanced tools. Now is the time for government agencies and Congress to act to prevent further abuse of the public’s rights to protest and assemble. A country that uses tools to watch its residents will ultimately lead to a country with little to no civic engagement and the complete silencing of marginalized communities.
While there is a lot of opportunity to address mass surveillance and protect people’s constitutional rights, government officials have refused to address government surveillance for decades, despite public protest. In the few instances where government officials put up roadblocks to stop surveillance tactics, those roadblocks were later removed or reformed so as to allow the previous surveillance to continue. The lack of political will of Congressmembers to address these issues has been a huge challenge for civil rights organizations and individuals fighting for change.
Plan of Action
Regulations need to be put in place to restrict federal agency use of surveillance tools on the public.
Recommendation 1. Federal agencies must disclose technologies they are using to surveil individuals and organizations, as well as the frequency with which they use them. Agencies should to publish this information on their websites and produce a more comprehensive report for the Department of Justice (DOJ) to review.
Every six months, Google releases the number of requests it receives from government agencies asking for user information. Google informs the public on the number of accounts that were affected by those requests and whether the request was a subpoena, search warrant, or other court order. The FBI also discloses the number of DNA samples it has collected from individuals in each U.S. state and territory and how many of those DNA samples aided in investigations.
Likewise, government agencies should be required to disclose the names of the technologies they are purchasing to surveil people in the United States as well as the number of times they use this technology within the year. Government entities should no longer be able to hide which technologies their departments are using to watch the public. People should be informed on the depth of the government’s use of these tools so they have a chance to voice their objections and concerns.
Federal agencies also need to publish a more comprehensive report for the DOJ to review. This report will include what technologies were used and where, what category of organizations they were used against, racial demographics of the people who were surveilled, and possible threats to civil rights. The DOJ will use this information to run investigate whether agencies are violating the Fourth Amendment or First Amendment in using these technologies against the public.
Agencies may object to releasing this information because of the possibility of it interfering with investigations. However, Google does not release the names of individuals who have had their user information requested, and neither should government agencies release user information. Because government agencies won’t be required to release specific information on individuals to the public, this will not affect their investigations. This disclosure request is aimed at knowing what tools government agencies are using and giving the DOJ the opportunity to investigate whether these tools violate constitutional rights.
Recommendation 2. Attorney General Guidelines should be revised in collaboration with the White House Office of Science and Technology Policy (OSTP) and civil rights organizations that specialize in technology issues.
The FBI has used advanced technology to watch activists and protests with little to no government oversight or input from civil rights organizations. When conducting an investigation or assessment of an individual or organization, FBI agents follow the Attorney General Guidelines, which dictate how investigations should be conducted. Unfortunately, these guidelines do little to protect the public’s civil rights—and in fact contain a few provisions that are quite problematic:
- The FBI is able to conduct assessments, which don’t require factual basis but instead require an authorized purpose, such as obtaining information on an organization or person if it’s believed that they could be involved in activities threatening national security or suspected that they could be the target of an attack.
- Physical surveillance can be used during an assessment for a limited time, but that period has been redacted in the guide so it’s not clear how long they can engage in this practice.
- FBI employees can conduct internet searches of “publicly available information” for an authorized purpose without having a lead, tip, referral, or complaint. FBI employees can also use online services to obtain publicly available information before the employee even decides to open an assessment or formal investigation. FBI employees are not required to seek supervisor approval beforehand.
These provisions are problematic for a few reasons. FBI employees should not be able to conduct assessments on individuals without a factual basis. Giving employees the power to pick and choose who they want to assess provides an opportunity for inherent bias. Instead, all assessments and investigations should have some factual basis behind them and receive approval from a supervisor. Physical surveillance and internet searches, likewise, should not be conducted by FBI agents without probable cause. Allowing these kinds of practices opens the entire public to having their privacy invaded.
These policies should be reviewed and revised to ensure that activists and organizations won’t be subject to surveillance due to internal bias. President Biden should issue an executive order directing OSTP to collaborate with the Office of the Attorney General on the guidelines. OSTP should have a task force dedicated to researching government surveillance and the impact on marginalized groups to guide them on this collaboration.
External organizations that are focused on technology and civil rights should also be brought in to review the final guidelines and voice any concerns. Civil rights organizations are more in tune with the effect that government surveillance has on their communities and the best mechanisms that should be put in place to preserve privacy rights.
Congress also should take steps to protect the public’s civil rights by passing the Fourth Amendment Is Not for Sale Act, revising the Stored Communications Act, and passing border exception legislation.
Recommendation 3. Congress should close the loophole that allows government agencies to circumvent the Fourth Amendment and purchase data from private companies by passing the Fourth Amendment Is Not for Sale Act.
In 2008, it was revealed that AT&T had entered into a voluntary partnership with the National Security Agency (NSA) from 2001 to 2008. AT&T built a room in its headquarters that was dedicated to providing the NSA with a massive quantity of internet traffic, including emails and web searches.
Today, AT&T has eight facilities that intercept internet traffic across the world and provide it to the NSA, allowing them to view people’s emails, phone calls, and online conversations. And the NSA isn’t the only federal agency partnering with private companies to spy on Americans. It was revealed in 2020 that the FBI has an agreement with Dataminr, a company that monitors people’s social media accounts, and Venntel, Inc., a company that purchases bulk location data and maps the movements of millions of people in the United States. These agreements were signed and modified after BLM protests were held across the country.
Allowing government agencies to enter into agreements with private companies to surveil people gives them the ability to bypass the Fourth Amendment and spy on individuals with no restriction. Federal agencies no longer need rely on the courts when seeking private communications and thoughts; they can now purchase sensitive information like a person’s location data and social media activity from a private company. Congress should end this practice and ban federal government agencies from purchasing people’s private data from third parties by passing the Fourth Amendment Is Not For Sale Act. If this bill passed, government agents could no longer purchase location data from a data broker to figure out who was in a certain area during a protest or partner with a company to obtain people’s social media postings without going through the legal process.
Recommendation 4. Congress should amend the Stored Communications Act of 1986 (SCA) to compel electronic communication service companies to prove they are in compliance with the act.
The SCA prohibits companies that provide an electronic communication service from “knowingly” sharing their stored user data with the government. While data brokers are more than likely excluded from this provision, companies that provide direct services to the public such as Facebook, Twitter, and Snapchat are not. Because of this law, direct service companies aren’t partnering with government agencies to sell user information, but they are selling user data to third parties like data brokers.
There should be a responsibility placed on electronic communication service companies to ensure that the companies they sell user information to won’t sell data to government entities. Congress should amend the SCA to include a provision requiring companies to annually disclose who they sold user data to and whether they verified with the third party that the data will not be eventually sold to a government entity. Verification should require at minimum a conversation with the third party about the SCA provision and a signed agreement that the third party will not sell any user information to the government. The DOJ will be tasked with reviewing these disclosures for compliance.
Recommendation 5. Congress should pass legislation revoking the border search exception. As stated earlier, this exception allows federal agents to conduct warrantless searches and seizures within 100 miles of the U.S. border. It also allows federal agents to search and seize digital devices at the border without having any level of suspicion that the traveler has committed a crime. CBP agents have pressured travelers to unlock their devices to look at the content, as well as downloaded the content of the devices and stored the data in a central database for up to 15 years.
While other law enforcement agencies are forced to abide by the Fourth Amendment, federal agents have been able to bypass the Fourth Amendment and conduct warrantless searches and seizures without restriction. If federal agents are allowed to continue operating without the restrictions of the Fourth Amendment, it’s possible we will see more instances of local law enforcement agencies calling on CBP to conduct surveillance operations on the general public during protests. This is an unconscionable amount of power to give to agencies that can and has led to serious abuse of the public’s privacy rights. Congress must roll back this authority and require all law enforcement agencies—local, state, and federal—to have probable cause at a minimum before engaging in searches and seizures.
Conclusion
For too long, government agencies have been able to surveil individuals and civil rights organizations with little to no oversight. With the advancement of technology, their surveillance capabilities have grown tremendously, leading to near 24/7 surveillance. Regulations must be put in place to restrict the use of surveillance technologies by federal agencies, and Congress must pass legislation to protect the public’s constitutional rights.
The FBI operates under the jurisdiction of the DOJ and reports to the Attorney General. The Attorney General has been granted the authority under U.S. Codes and Executive Order 12333 to issue guidelines for the FBI to follow when they conduct domestic investigations. These are the Attorney General Guidelines.
This bill was introduced by Senators Ron Wyden, Rand Paul, and 18 others in 2021 to protect the public from having government entities purchase their personal information, such as location data, from private companies rather than going through the court system. Instead, the government would be required to obtain a court order before they getting an individual’s personal information from a data broker. This is a huge step in protecting people’s private information and stopping mass government surveillance.
Modernizing Enforcement of the Civil Rights Act to Mitigate Algorithmic Harm in Determining Federal Benefits
The Department of Justice should modernize the enforcement of Title VI of the Civil Rights Act to guide effective corrective action for algorithmic systems that produce discriminatory outcomes with regard to federal benefits. To do so, the Department of Justice should clarify the definition of “algorithmic discrimination” in the context of federal benefits, establish systems to identify which federally funded public benefits offices use machine-learning algorithms, and secure the necessary human resources to properly address algorithmic discrimination. This crucial action would leverage a demonstrable, growing interest in regulating algorithms that has bloomed over the past year via policy actions in both the White House and Congress but has yet to concretely establish an appropriate enforcement mechanism for acting on instances of demonstrated algorithmic harm.
Challenge and Opportunity
Algorithmic systems are inescapable in modern life. They have become core elements of everyday activities, like surfing the web, driving to work, and applying for a job. It is virtually impossible to go through life without encountering an algorithmic system multiple times per day.
As machine-learning technologies have become more pervasive, they have also become gatekeepers for crucial resources, like accessing credit, receiving healthcare, securing housing, and getting a mortgage. Both local and federal governments have embraced algorithmic decision-making to determine which constituents are able to access key services, often with little transparency, if any, for those who are subject to such decision-making.
When it comes to federal benefits, imperfections in these systems scale significantly. For example, the deployment of flawed algorithmic tools led to the wrongful termination of Medicaid for 19% of beneficiaries in Arkansas, the wrongful termination of Social Security income for thousands in New York, wrongful termination of $78 million worth of Medicaid and Supplemental Nutrition Assistance Program benefits in Indiana, and erroneous unemployment fraud charges for 40,000 people in Michigan. These errors are particularly harmful to low-income Americans for whom access to credit, housing, job opportunities, and healthcare are especially important.
Over the past year, momentum for regulating algorithmic systems has grown, resulting in several key policy actions. In February 2022, Senators Ron Wyden and Cory Booker and Representative Yvette Clarke introduced the Algorithmic Accountability Act. Endorsed by AI experts, this bill would have required deployers of algorithmic systems to conduct and publicly share impact assessments of their systems. In October 2022, the White House released its Blueprint for an AI Bill of Rights. Although not legally enforceable, this robust rights-based framework for algorithmic systems was developed with a broad coalition of support through an intensive, yearlong public consultation process with community members, private sector representatives, tech workers, and policymakers. Also in October 2022, the AI Training Act was passed into law. The legislation requires the development of a training curriculum covering core concepts in artificial intelligence for federal employees in a limited range of roles, primarily those involved in procurement. Finally, January 2023 saw the introduction of the NIST AI Risk Management Framework to guide how organizations and individuals design, develop, deploy, or use artificial intelligence to manage risk and promote responsible use.
Collectively, these actions demonstrate clear interest in preventing harm caused by algorithmic systems, but none of them provide clear enforcement mechanisms for federal agencies to pursue corrective action in the wake of demonstrated algorithmic harm.
However, Title VI of the Civil Rights Act offers a viable and legally enforceable mechanism to aid anti-discrimination efforts in the algorithmic age. At its core, Title VI bans the use of federal funding to support programs (including state and local governments, educational institutions, and private companies) that discriminate on the basis of race, color, or national origin. Modernizing the enforcement of Title VI, specifically in the context of federal benefits, offers a clear opportunity for developing and refining a modern enforcement approach to civil rights law that can respond appropriately and effectively to algorithmic discrimination.
Plan of Action
Fundamentally, this plan of action seeks to:
- Clarify how “algorithmic bias” is defined, specifically in the context of federal benefits.
- Identify where and when public benefits systems use machine-learning algorithms.
- Equip federal agencies with authority and skill sets to address algorithmic discrimination.
Clarify the Framework for Algorithmic Bias in Federal Benefits
Recommendation 1. Fund the Department of Justice (DOJ) to develop a new working group focused specifically on civil rights concerns around artificial intelligence.
The DOJ has already requested funding for and justified the existence of this unit in its FY2023 Performance Budget. In that budget, the DOJ requested $4.45 million to support 24 staff.
Clear precedents for this type of cross-sectional working group already exist within the Department of Justice (e.g., the Indian Working Group and LGBTQI+ Working Group). Both of these groups contain members of the 11 sections of the Civil Rights Division to ensure a comprehensive strategy for protecting the civil rights of Indigenous peoples and the LGBTQ+ community, respectively. The pervasiveness of algorithmic systems in modern life suggests a similarly broad scope is appropriate for this issue.
Recommendation 2. Direct the working group to develop a framework that defines algorithmic discrimination and appropriate corrective action specifically in the context of public benefits.
A clear framework or rubric for assessing when algorithmic discrimination has occurred is a prerequisite for appropriate corrective action. Despite having a specific technical definition, the term “algorithmic bias” can vary widely in its interpretation depending on the specific context in which an automated decision is being made. Even if algorithmic bias does exist, researchers and legal scholars have made the case that biased algorithms may be preferable to biased human decision-makers on the basis of consistency and the relative ease of behavior change. Consequently, the DOJ should develop a context-specific framework for determining when algorithmic bias leads to harmful discriminatory outcomes in federal benefits systems, starting with major federal systems like Social Security and Medicare/Medicaid.
As an example, the Brookings Institution has produced a helpful report that illustrates what it means to define algorithmic bias in a specific context. Cross-walking this blueprint with existing Title VI procedures can yield guidelines for how the Department of Justice can notify relevant offices of algorithmic discrimination and steer corrective action.
Identify Federal Benefits Systems that Use Algorithmic Tools
Recommendation 3. Establish a federal register or database for offices that administer federally funded public benefits to document when they use machine-learning algorithms.
This system should specifically detail the developer of the algorithmic system and the office using said system. If possible, descriptions of relevant training data should be included as well, especially if these data are federal property. Consider working with the Office of Federal Contract Compliance Programs to secure this information from current and future government contractors within the federal benefits domain.
In terms of cost, previous budget requests for databases of this type have ranged from $2 million to $5 million.
Recommendation 4. Provide public access to the federal register.
Making the federal register public would provide baseline transparency regarding the federal funding of algorithmic systems. This would facilitate external investigative efforts to identify possible instances of algorithmic discrimination in public benefits, which would complement internal efforts by directing limited federal staff bandwidth towards cases that have already been identified. The public-facing portion of this registry should be structured to respecting appropriate privacy and trade secrecy restrictions
Recommendation 5. Link the public-facing register to a public-facing form for submitting claims of algorithmic discrimination in the context of federal benefits.
This step would help channel public feedback regarding claims of algorithmic discrimination with a sufficiently high threshold to minimize frivolous claims. A well-designed system will ask for evidence and data to justify any claim of algorithmic discrimination, allowing federal employees to prioritize which claims to pursue.
Equip Agencies with Necessary Resources for Addressing Algorithmic Discrimination
Recommendation 6. Authorize funding for technical hires in enforcement arms of federal regulatory agencies, including but not limited to the Department of Justice.
Effective enforcement of anti-discrimination statutes today requires technical fluency in machine-learning techniques. In addition to the DOJ’s Civil Rights Division (see Recommendation 1), consider directing funds to hire or train technical experts within the enforcement arms of other federal agencies with explicit anti-discrimination enforcement authority, including the Federal Trade Commission, Federal Communications Commission, and Department of Education.
Recommendation 7. Pass the Stopping Unlawful Negative Machine Impacts through National Evaluation Act.
This act was introduced with bipartisan support in the Senate at the very end of the 2021–2022 legislative session by Senator Rob Portman. The short bill seeks to clarify that civil rights legislation applies to artificial intelligence systems and decisions made by these systems will be liable to claims of discrimination under said legislation, including the Civil Rights Act, the Americans with Disabilities Act, and the Age Discrimination Act of 1975, among others. Passing the bill is a simple but effective way to indicate to federal regulatory agencies (and those they regulate) that artificial intelligence systems must comply with civil rights law and affirms the federal government’s authority to ensure they do so.
Conclusion
On his first day in office, President Biden signed an executive order to address the entrenched denial of equal opportunities for underserved communities in the United States. Ensuring that federal benefits are not systematically denied via algorithmic discrimination to low-income Americans and Americans of color is crucial to successfully meeting the goals of that order and the rising chorus of voices who want meaningful regulation for algorithmic systems. The authority for such regulation in the context of federal benefits already exists. To ensure that authority can be effectively enforced in the modern age, the federal government needs to clearly define algorithmic discrimination in the context of federal benefits, identify where federal funding is supporting algorithmic determination of federal benefits, and recruit the necessary talent to verify instances of algorithmic discrimination.
An algorithm is a structured set of steps for doing something. In the context of this memo, an algorithm usually means computer code that is written to do something in a structured, repeatable way, such as determining if someone is eligible for Medicare, identifying someone’s face using a facial recognition tool, or matching someone’s demographic profile to a certain kind of advertisement.
Machine-learning techniques are a specific set of algorithms that train a computer to do different tasks by taking in a massive amount of data and looking for patterns. Artificial intelligence generally refers to technical systems that have been trained to perform tasks with minimal human oversight. Machine learning and artificial intelligence are similar and often used as interchangeable terms.
We can identify algorithmic bias by comparing the expected outputs of an algorithm to the actual outputs for an algorithm. For example, if we find that an algorithm uses race as a decisive factor in determining whether someone is eligible for federal benefits that should be race-neutral, that would be an example of algorithmic bias. In practice, these assessments often take the form of statistical tests that are run over multiple outputs of the same algorithmic system.
Although many algorithms are biased, not all biases are equally harmful. This is due to the highly contextual nature in which an algorithm is used. For example, a false positive in a criminal-sentencing algorithm arguably causes more harm than a false positive in a federal benefits determination. Algorithmic bias is not inherently a bad thing and, in some cases, can actually advance equity and inclusion efforts depending on the specific contexts (consider a hiring algorithm for higher-level management that weights non-male gender or non-white race more heavily for selection).
Using a Digital Justice Framework To Improve Disaster Preparation and Response
Social justice, environmental justice, and climate justice are all digital justice. Digital injustice arises from the fact that 21 million Americans are not connected to the internet, and seven percent of Americans do not use it, even if they have access to it. This lack of connectivity can lead to the loss of life, disrupted communities, and frayed social cohesion during natural disasters, as people are unable to access life-saving information and preventive tools found online.
Digital injustice primarily affects poor rural communities and African American, Indigenous, and other communities of color. These communities are also overexposed to climate risk, economic fragility, and negative public health outcomes. Digital access is a pathway out of this overexposure. It is a crucial aspect of the digital justice conversation, alongside racial equity and climate resilience.
Addressing this issue requires a long-term commitment to reimagining frameworks, but we can start by helping communities and policymakers understand the problem. Congress and the Biden-Harris Administration should embrace and support the creation of a Digital Justice Policy Framework that includes:
- training and access to information for divested communities
- within-government climate and digital literacy efforts
- a public climate and digital literacy campaign
Challenges and Opportunities
The internet has become a crucial tool in preparing for and recovering from ecological emergencies, building wealth, and promoting community connections. However, the digital divide has created barriers to accessing these resources for millions of people, particularly low-income individuals and people of color. The lack of access to the internet and technology during emergencies deepens existing vulnerabilities and creates preventable losses of life, displacement, and disrupted lives.

Digital divestment, disasters, and poverty overlap in dangerous ways that reveal “inequities and deepen existing vulnerability… In the United States, roughly 21% of children live in poverty and without consistent access to food. Cascading onto poverty and vulnerability to large-scale events like pandemics and other disasters is the lack of access to the Internet and the education and opportunity that comes with it.”
A recent report about digital divestment in rural communities shows that access to internet infrastructure, devices, and information is critical to economic development. Yet rural communities are more likely to have no device in the home—26.4% versus 20% of the broader United States. Access to broadband is even lower, as most rural counties have just one or no provider. Geography often challenges access to public services.
To tackle this issue, we must reimagine the use of data to ensure that all communities have access to information that reduces vulnerability and strengthens resilience. One pathway to reimagining data in a meaningful way is laid out in a National Academies of Science consensus study report, “Communities need information that they can effectively use in making decisions and investments that reduce the vulnerability and strengthen the resilience of their residents, economy, and environment. Assembling and using that information requires three things. First, data, while often abundantly available to communities, can be challenging for local communities and users to navigate, access, understand, and evaluate relative to local needs and questions. Second, climate data needs to be vetted and translated into information that is useful at a local level. Finally, information that communities receive from other sources needs to reflect the challenges and opportunities of those communities to not just be useful but also used.” Once communities are effectively connected and skilled up, they can use the information to make effective decisions.
The Government Accountability Office (GAO) looked into the intersection of information and justice, releasing a study on the fragmented and overlapping broadband plan and funding. It recommended a national strategy to help scale these efforts across communities and focus agency efforts on communities in need that includes recommendations for education, workforce training, and evidence-based policymaking.
Communities can be empowered to take a data-driven journey from lack of access to resources to using innovative concepts like regenerative finance to build resiliency. With the right help, divested communities can co-create sustainable solutions and work toward digital justice. The federal government should leverage initiatives like the Justice 40 initiative, aimed at undoing past injustices and divestment, to create opportunities for communities to gain access to the tools they need and understand how to use them.
Plan of Action
Executive branch agencies and Congress should initiate a series of actions to establish a digital justice framework. The first step is to provide education and training for divested communities as a pathway to participate in digital and green economies.
- Funding from recent legislation and agency earmarks should be leveraged to initiate education and training targeted at addressing historical inequities in the localization, quality, and information provided by digital infrastructure:
- The Infrastructure Investment and Jobs Act (IIJA) allocates $65 billion to expand the availability of broadband Internet access. The bulk of that funding is dedicated to access and infrastructure. Under the National Telecommunications and Information Administration’s (NTIA) Broadband Equity, Access, and Deployment (BEAD) Program, there is both funding and broad program language that allows for upskilling and training. Community leaders and organizations need support to advocate for funding at the state and local levels.
- The Environmental Protection Agency’s (EPA)1 environmental education fund, which traditionally has $2 million to $3.5 million in grant support to communities, is being shaped right now. Its offerings and parameters can be leveraged and extended without significant structural change. The fund’s parameters should include elements of the framework, including digital justice concepts like climate, digital, and other kinds of literacy programs in the notices of funding opportunities. This would enable community organizations that are already doing outreach and education to include more offerings in their portfolios.
To further advance a digital justice framework, agencies receiving funding from IIJA and other recent legislative actions should look to embed education initiatives within technical assistance requests for proposals and funding announcements. Communities often lack access to and support in how to identify and use public resources and information related to digital and climate change challenges. One way to overcome this challenge is to include education initiatives as key components of technical assistance programs. In its role of ensuring the execution of budget proposals and legislation, the Office of Budget and Management (OMB) can issue guidance or memoranda to agencies directing them to include education elements in notices of funding, requests for proposals, and other public resources related to IIJA, IRA and Justice 40.
One example can be found in the Building Resilient Infrastructure and Communities (BRIC) program. In addition to helping communities navigate the federal funding landscape, OMB could require that new rounds of the program include climate or resilience education and digital literacy. The BRIC program can also increase its technical assistance offerings from 20% of applicants to 40%, for example. This would empower recipients to navigate the fuller landscape of using science to develop solutions and then successfully navigate the funding process.
Another program that is being designed at the time of this writing is the Environmental and Climate Justice Grant Program, which contains $3 billion in funding from the IRA. There is a unique opportunity to draft requests for information, collaboration, or proposals to include ideas for education and access programs to democratize critical information by teaching communities how to access and use it.
An accompanying public education campaign can make these ideas sustainable. Agencies should engage with the Ad Council on a public education campaign about digital justice or digital citizenship, social mobility, and climate resilience. As an example, in 2022 FEMA funded a preparation initiative directed at Black Americans and disasters with the Ad Council that discussed protecting people and property from disasters across multiple topics and media. The campaign was successful because the information was accessible and demonstrated its value.
Climate literacy and digital citizenship training are as necessary for those designing programs as they are for communities. The federal agencies that disburse this funding should be tasked with creating programs to offer climate literacy and digital citizenship training for their workforce. Program leaders and policy staff should also be briefed and trained in understanding and detecting data collection, aggregation, and use biases. Federal program officers can be stymied by the lack of baseline standards for federal workforce training and curricula development. For example, FEMA has a goal to create a “climate literate” workforce and to “embed equity” into all of its work—yet there is no evidence-based definition nor standard upon which to build training that will yield consistent outcomes. Similar challenges surface in discussions about digital literacy and understanding how to leverage data for results.2 Within the EPA, the challenge is helping the workforce understand how to manage the data it generates, use it to inform programs, and provide it to communities in meaningful ways. Those charged with delivering justice-driven programs must be provided with the necessary education and tools to do so.
FEMA, like the EPA and other agencies, will need help from Congress. Congress should do more to support scientific research and development for the purpose of upskilling the federal workforce. Where necessary, Congress must allocate funding, or adjust current funding mechanisms, to provide necessary resources. There is $369 billion for “Energy Security and Climate Change” in the Inflation Reduction Act of 2022 that broadly covers the aforementioned ideas. Adjusting language to reference programs that address education and access to information would make it clear that agencies can use some of that funding. In the House, this could take the form of a suspension bill or addition as technical correction language in a report. In the Senate, these additions could be added as amendments during “vote-o-rama.”
For legislative changes involving the workforce or communities, it is possible to justify language changes by looking at the legal intent of complementary initiatives in the Biden-Harris Administration. In addition to IIJA provisions, policy writers can use parts of the Inflation Reduction Act and the Justice 40 initiative, as well as the climate change and environmental justice executive orders, to justify changes that will provide agencies with direction and resources. Because this project is at the intersection of climate and digital justice, the jurisdictional alignments would mainly be with the United States Department of Commerce, the National Telecommunications and Information Administration, the United States Department of Agriculture, EPA and FEMA.
Recommendations for federal agencies:
- Make public literacy about digital and climate justice a national priority. (This includes government agency personnel as well as residents and citizens.)
- Train agency program officers charged with administering programs on the impacts and solutions for digital justice.
- To empower rural and BIPOC communities to access programs consistently, require plain language drafts or section-by-section explainers for scientific and financial information related to digital justice.
- Create and require a set of “accessible research” guidelines for research institutions that receive federal funding to ensure their work is usable in communities.
Recommendations for Congress:
- Provide research dollars to help agencies develop evidence-based benchmarks for climate, data, and digital literacy programs.
- Set aside federal workforce development funds to build government-wide capacity in these areas.
- Make technical assistance for small municipalities and small community-based organizations a required part of any new digital justice-related statutes and funding mechanisms.
Conclusion
Digital justice is about a deeper understanding of the generational challenges we must confront in the next few years: the digital divide, climate risk, racial injustice, and rural poverty. Each of these connects back to our increasingly digital world and efforts to make sure all communities can access its benefits. A new policy framework for digital justice should be our ultimate goal. However, there are present opportunities to leverage existing programs and policy concepts to create tangible outcomes for communities now. Those include digital and climate literacy training, public education, and better education of government program leaders as well as providing communities and organizations with more transparent access to capital and information.
Digital divestment refers to the intentional exclusion of certain communities and groups from the social, intellectual, and economic benefits of the internet, as well as technologies that leverage the internet.
Climate resilience is about successfully coping with and managing the impacts of climate change while preventing those impacts from growing worse. This does not mean only thinking about severe weather. It also includes economic shocks and public health emergencies that come with climate change. During the COVID-19 pandemic, women disproportionately passed away and in one Maryland city, survivors’ social mobility decreased by 1%. However, the introduction of community WIFI began to change these outcomes.
Communities (municipalities, states) that are left out of access to internet infrastructure not only miss out on educational, economic, and social mobility opportunities; they also miss out on critical information about severe weather and climate change. Scientists and researchers depend on an internet connection to conduct research to target solutions. No high-quality internet means no access to information about cascading risk.
While the IIJA broadband infrastructure funding is a once-in-a-generation effort, the reality is that in many rural areas broadband is either not cost-effective nor a feasible solution due to geography or other contexts.
By opening funding to different kinds of internet infrastructures (community Wi-Fi, satellite, fixed access), communities can increase their risk awareness and make their own solutions.
The federal government is already creating executive orders and legislation in this space. What is needed is a more cohesive plan. In some cases that may entail partnering with the private sector or finding creative ways to partner with communities.
The first step is briefings and socializing this policy work because looking at equity, tech, and climate change from this perspective is still new and unfamiliar to many.