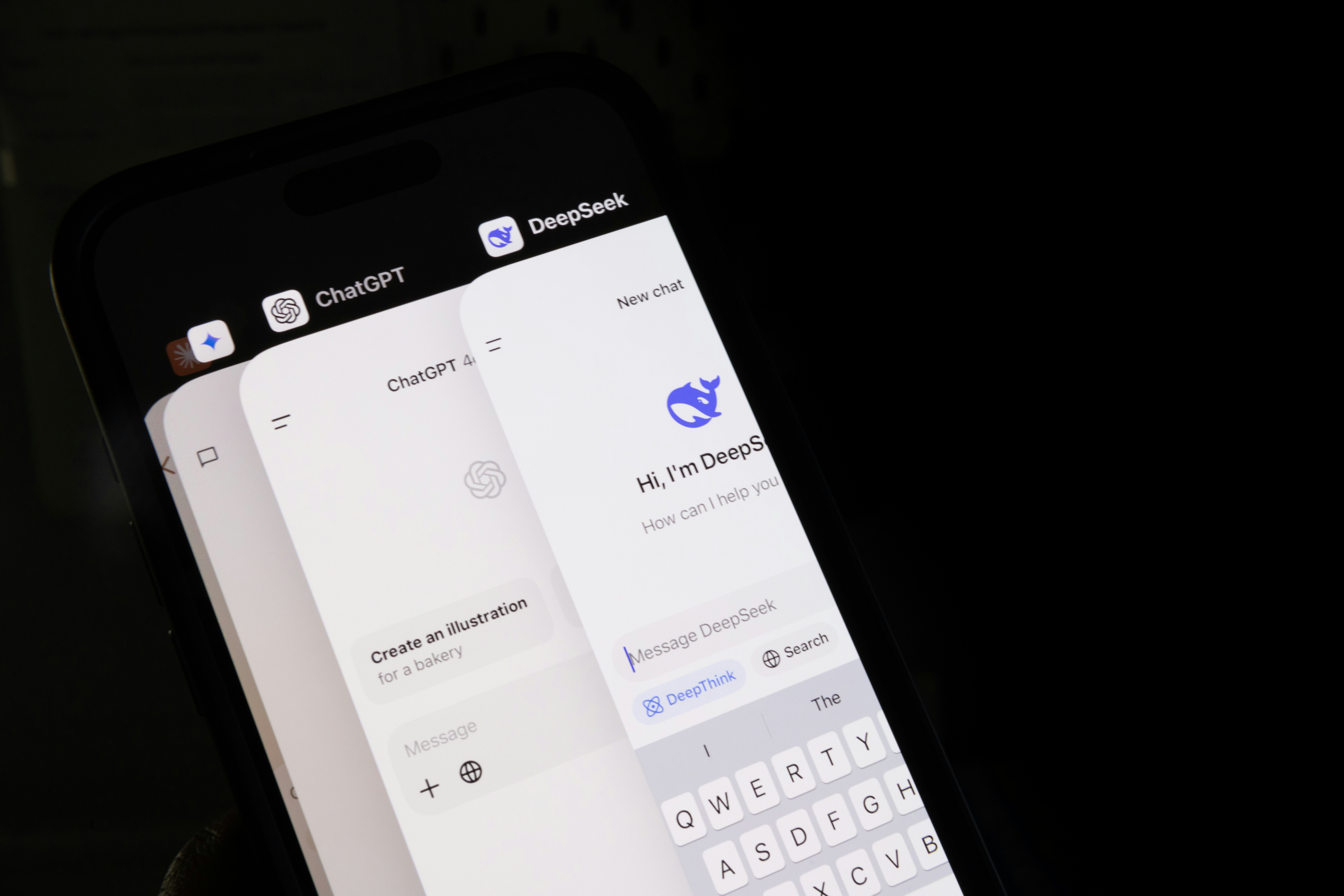
Strengthening Information Integrity with Provenance for AI-Generated Text Using ‘Fuzzy Provenance’ Solutions
Synthetic text generated by artificial intelligence (AI) can pose significant threats to information integrity. When users accept deceptive AI-generated content—such as large-scale false social media posts by malign foreign actors—as factual, national security is put at risk. One way to help mitigate this danger is by giving users a clear understanding of the provenance of the information they encounter online.
Here, provenance refers to any verifiable indication of whether text was generated by a human or by AI, for example by using a watermark. However, given the limitations of watermarking AI-generated text, this memo also introduces the concept of fuzzy provenance, which involves identifying exact text matches that appear elsewhere on the internet. As these matches will not always be available, the descriptor “fuzzy” is used. While this information will not always establish authenticity with certainty, it offers users additional clues about the origins of a piece of text.
To ensure platforms can effectively provide this information to users, the National Institute of Standards and Technology (NIST)’s AI Safety Institute should develop guidance on how to display to users both provenance and fuzzy provenance—where available—within no more than one click. To expand the utility of fuzzy provenance, NIST could also issue guidance on how generative AI companies could allow the records of their free AI models to be crawled and indexed by search engines, thereby making potential matches to AI-generated text easier to discover. Tradeoffs surrounding this approach are explored further in the FAQ section.
By creating a reliable, user-friendly framework for surfacing these details, NIST would empower readers to better discern the trustworthiness of the text they encounter, thereby helping to counteract the risks posed by deceptive AI-generated content.
Challenge and Opportunity
Synthetic Text and Information Integrity
In the past two years, generative AI models have become widely accessible, allowing users to produce customized text simply by providing prompts. As a result, there has been a rapid proliferation of “synthetic” text—AI-generated content—across the internet. As NIST’s Generative Artificial Intelligence Profile notes, this means that there is a “[l]owered barrier of entry to generated text that may not distinguish fact from opinion or fiction or acknowledge uncertainties, or could be leveraged for large scale dis- and mis-information campaigns.”
Information integrity risks stemming from synthetic text—particularly when generated for non-creative purposes—can pose a serious threat to national security. For example, in July 2024 the Justice Department disrupted Russian generative-AI-enabled disinformation bot farms. These Russian bots produced synthetic text, including in the form of social media posts by fake personas, meant to promote messages aligned with the interests of the Russian government.
Provenance Methods For Reducing Information Integrity Risks
NIST has an opportunity to provide community guidance to reduce the information integrity risks posed by all types of synthetic content. The main solution currently being considered by NIST for reducing the risks of synthetic content in general is provenance, which refers to whether a piece of content was generated by AI or a human. As described by NIST, provenance is often ascertained by creating a non-fungible watermark, or a cryptographic signature for a piece of content. The watermark is permanently associated with the piece of content. Where available, provenance information is helpful because knowing the origin of text can help a user know whether to rely on the facts it contains. For example, an AI-generated news report may currently be less trustworthy than a human news report because the former is more prone to fabrications.
However, there are currently no methods widely accepted as effective for determining the provenance of synthetic text. As NIST’s report, Reducing Risks Posed by Synthetic Content, details, “[t]he effectiveness of synthetic text detection is subject to ongoing debate” (Sec. 3.2.2.4). Even if a piece of text is originally AI-generated with a watermark (e.g., by generating words with a unique statistical pattern), people can easily copy a piece of text by paraphrasing (especially via AI), without transferring the original watermark. Text watermarks are also vulnerable to adversarial attacks, with malicious actors able to mimic the watermark signature and make text appear watermarked when it is not.
Plan of Action
To capture the benefits of provenance, while mitigating some of its weaknesses, NIST should issue guidance on how platforms can make available to users both provenance and “fuzzy provenance” of text. Fuzzy provenance is coined here to refer to exact text matches on the internet, which can sometimes reflect provenance but not necessarily (thus “fuzzy”). Optionally, NIST could also consider issuing guidance on how generative AI companies can make their free models’ records available to be crawled and indexed by search engines, so that fuzzy provenance information would show text matches with generative AI model records. There are tradeoffs to this recommendation, which is why it is optional; see FAQs for further discussion. Making both provenance and fuzzy provenance information available (in no more than one click) will give users more information to help them evaluate how trustworthy a piece of text is and reduce information integrity risks.
Combined Provenance and Fuzzy Provenance Approach
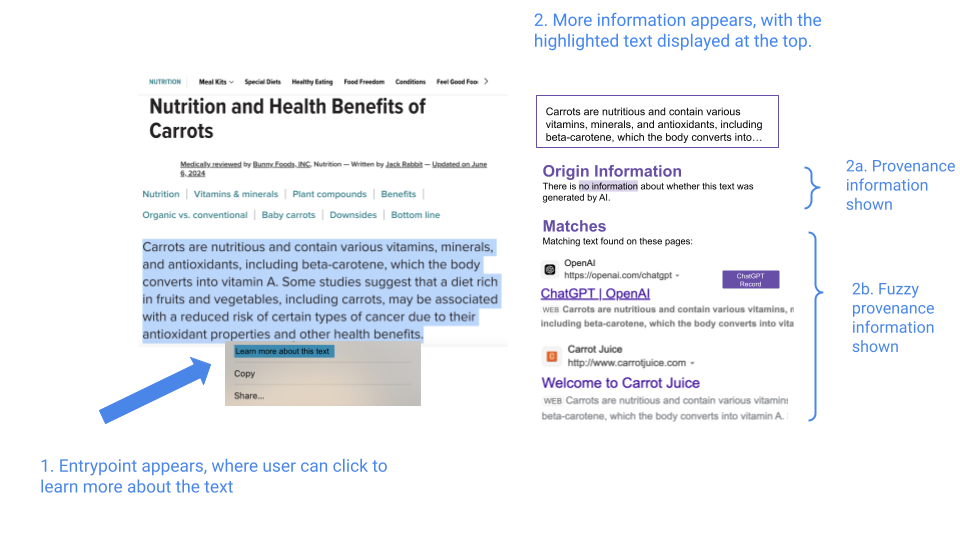
Figure 1. Mock implementation of combined provenance and fuzzy provenance
The above image captures what an implementation of the combined provenance and fuzzy provenance guidance might include. When a user highlights a piece of text that is sufficiently long, they can click “learn more about this text” to find more information.
There are ways to communicate provenance and fuzzy provenance so that it is both useful and easy-to-understand. In this concept showing the provenance of text, for example:
- Provenance information is shown under the heading “Origin Information”. This includes whether there is conclusive metadata (like watermarks) showing the text was generated by AI, according to C2PA standards, where available.
- Fuzzy provenance information is shown under the heading “Matches,” and includes other websites that have an exact text match to the highlighted text, similar to the results that come up when using a search engine. Furthermore, depending on NIST guidance, generative AI companies could have their free models’ records available to be crawled and indexed by search engines. This would enable these records to appear in the results if they contain an exact text match. These records would be clearly labeled (e.g., “ChatGPT Record”) and ranked at the top.
- See the following video for what this might look like for a user: user journeys.
Benefits of the Combined Approach
Showing both provenance and fuzzy provenance information provides users with critical context to evaluate the trustworthiness of a piece of text. Between provenance and fuzzy provenance, users would have access to information about many pieces of high-impact text, especially claims that could be particularly harmful for individuals, groups, or society at large. Making all this information immediately available also reduces friction for users so that they can get this information right where they encounter text.
Provenance information can be helpful to provide to users when it is available. For instance, knowing that a tech support company’s website description was AI-generated may encourage users to check other sources (like reviews) to see if the company is a real entity (and AI was used just to generate the description) or a fake entity entirely, before giving a deposit to hire the company (see user journey 1 in this video for an example).
Where clear provenance information is not available, fuzzy provenance can help fill the gap by providing valuable context to users in several ways:
- First, if there is no exact text match on the internet, it may indicate that the content is original—whether AI-generated or human-created—which can be especially relevant for assessing the trustworthiness of certain documents (see user journey 2 in this video for an example).
- Second, when presented with a misleading claim like “ginger is 10000x more effective than chemotherapy”, seeing that the text matches are from fact-check sites and unreliable sources such as other social media posts can encourage users to further investigate that claim (see user journey 3 in this video for an example).
- Third, absent any text matches from reliable sources, knowing that a claim (e.g., about carrots and cancer) matched an AI model’s record may cause a user to be skeptical, as they may realize that the text has the potential to be false content generated by AI (see user journey 4 in this video for an example).
Fuzzy provenance is also effective because it shows context and gives users autonomy to decide how to interpret that context. Academic studies have found that users tend to be more receptive when presented with further information they can use for their own critical thinking compared to being shown a conclusion directly (like a label), which can even backfire or be misinterpreted. This is why users may trust contextual methods like crowdsourced information more than provenance labels.
Finally, fuzzy provenance methods are generally feasible at scale, since they can be easily implemented with existing search engine capabilities (via an exact text match search). Furthermore, since fuzzy provenance only relies on exact text matching with other sources on the internet, it works without needing coordination among text-producers or compliance from bad actors.
Conclusion
To reduce the information integrity risks posed by synthetic text in a scalable and effective way, the National Institute for Standards and Technology (NIST) should develop community guidance on how platforms hosting text-based digital content can make accessible (in no more than one click) the provenance and “fuzzy provenance” of the piece of text, when available. NIST should also consider issuing guidance on how AI companies could make their free generative AI records available to be crawled by search engines, to amplify the effectiveness of “fuzzy provenance”.
This action-ready policy memo is part of Day One 2025 — our effort to bring forward bold policy ideas, grounded in science and evidence, that can tackle the country’s biggest challenges and bring us closer to the prosperous, equitable and safe future that we all hope for whoever takes office in 2025 and beyond.
PLEASE NOTE (February 2025): Since publication several government websites have been taken offline. We apologize for any broken links to once accessible public data.
Making free generative AI records available to be crawled by search engines includes tradeoffs, which is why it is an optional recommendation to consider. Below are some questions regarding implementation guidance, and trade offs including privacy and proprietary information.
Guidance could instruct AI model companies how to make their free generative AI conversation records available to be crawled and indexed by search engines. Similar to ChatGPT logs or Perplexity threads, a unique URL would be created for each conversation, capturing the date it occurred. The key difference is that all free model conversation records would be made available, but only with the AI outputs of the conversation, after removing personally identifiable information (PII) (see “Privacy considerations” section below). Because users can already choose to share conversations with each other (meaning the conversation logs are retained), and conversation logs for major model providers do not currently appear to have an expiration date, this requirement shouldn’t impose an additional storage burden for AI model companies.
Guidance could instruct search engines how to crawl and index these model logs so that queries with exact text matches to the AI outputs would surface the appropriate model logs. This would not be very different from search engines crawling/indexing other types of new URLs and should be well-within existing search engine capabilities. In terms of storage, since only free model logs would be crawled and indexed, and most free models rate-limit the number of user messages allowed, storage should also not be a concern. For instance, even with 200 million weekly active users for ChatGPT, the number of conversations in a year would only be on the order of billions, which is well-within the current scale that existing search engines have to operate to enable users to “search the web”.
- Output filtering on the AI outputs should be done to remove any personal identifiable information (PII) present in the model’s responses. However, it might still be possible to extrapolate who the original user was just by looking at the AI outputs taken together and inferring some of the user prompts. This is a privacy concern that should be further investigated. Some possible mitigations include additionally removing any location references of a certain granularity (i.e. removing mentions of neighborhoods, but retaining mentions of states) and presenting AI responses in the conversation in a randomized order.
- Removals should be made possible by a user-initiated process demonstrating privacy concerns, similar to existing search engine removal protocols.
- User consent would also be an important consideration here. NIST could propose that free model users must “opt-in”, or that free model record crawling/indexing be “opt-out” by default for users, though this may greatly compromise the reliability of fuzzy provenance.
- Training on AI-generated text: AI companies are concerned about accidentally picking up on too much AI-generated text on the web and training on that instead of higher human-generated text, thus degrading the quality of their own generative models. However, because they would have identifiable domain prefixes (ie chatgpt.com, perplexity.ai), it would be easy to exclude these AI-generated conversation logs if desired during training. Indeed, provenance and fuzzy provenance may help AI companies avoid unintentionally training on AI-generated text.
- Sharing model outputs: On the flipside, AI companies might be concerned that making so many AI-generated model outputs available for competitors to access may result in helping competitors improve their own models. This is a fair concern, though partially mitigated by a) specific user inputs are not available, and only the AI outputs; and b) only free model outputs would be logged, rather than any premium models, thus providing some proprietary protection. However, it is still possible that competitors may be able to enhance their own responses by training on the structure of AI outputs from other models at scale.
It’s paramount to balance both innovation capabilities and risk as we work towards ensuring that the U.S. bioeconomy is a priority area for both the Nation and for National Security.
The Federation of American Scientists supports the National Security Commission on Emerging Biotechnology’s Final Report and the Recommendations contained within it.
The U.S. should create a new non-governmental Innovation Accelerator modeled after the successful In-Q-Tel program to invest in small and mid-cap companies creating technologies that address critical needs of the United States.
To sustain America’s leadership in AI innovation, accelerate adoption across the economy, and guarantee that AI systems remain secure and trustworthy, we offer a set of policy recommendations.