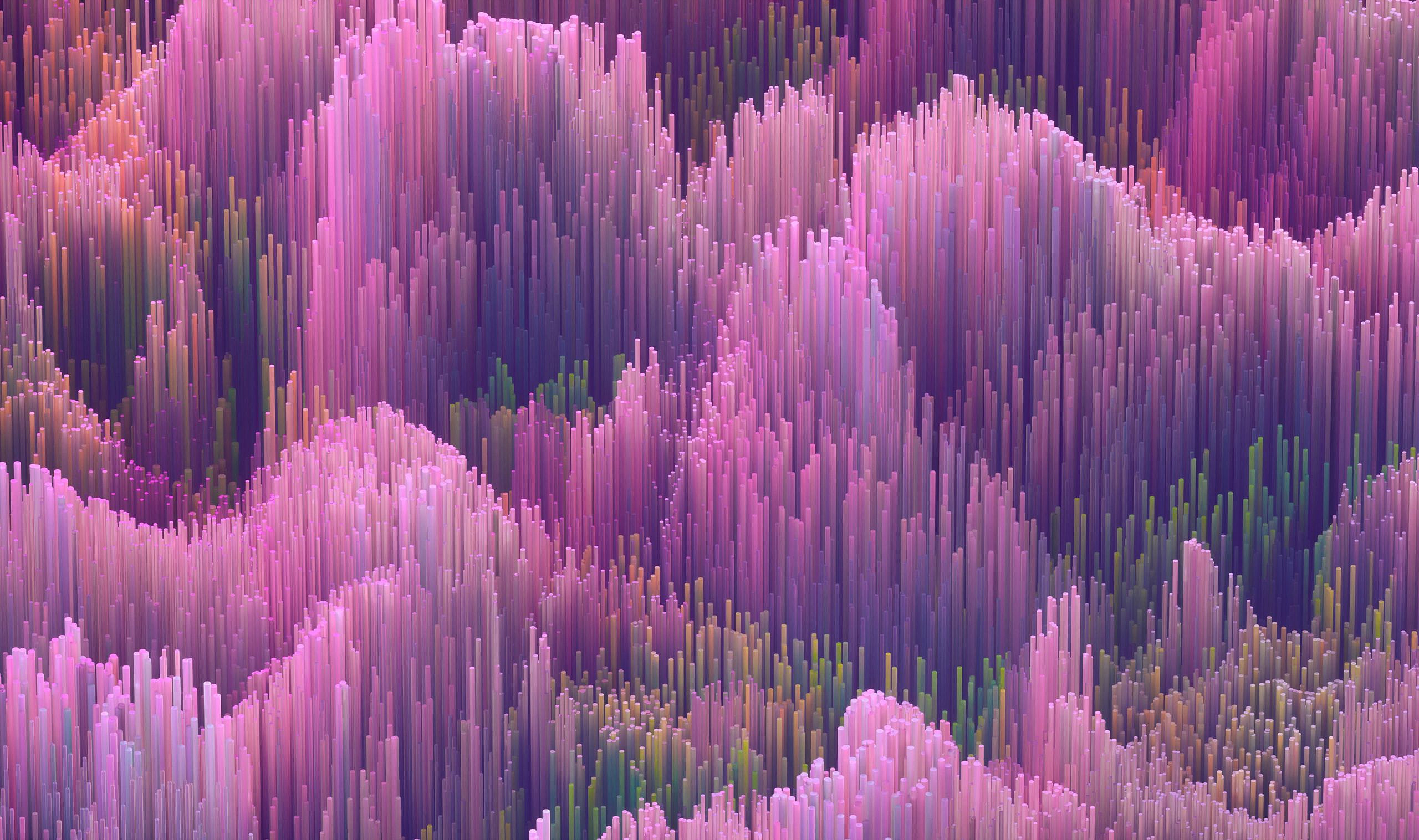
Unlocking Federal Grant Data To Inform Evidence-Based Science Funding
Summary
Federal science-funding agencies spend tens of billions of dollars each year on extramural research. There is growing concern that this funding may be inefficiently awarded (e.g., by under-allocating grants to early-career researchers or to high-risk, high-reward projects). But because there is a dearth of empirical evidence on best practices for funding research, much of this concern is anecdotal or speculative at best.
The National Institutes of Health (NIH) and the National Science Foundation (NSF), as the two largest funders of basic science in the United States, should therefore develop a platform to provide researchers with structured access to historical federal data on grant review, scoring, and funding. This action would build on momentum from both the legislative and executive branches surrounding evidence-based policymaking, as well as on ample support from the research community. And though grantmaking data are often sensitive, there are numerous successful models from other sectors for sharing sensitive data responsibly. Applying these models to grantmaking data would strengthen the incorporation of evidence into grantmaking policy while also guiding future research (such as larger-scale randomized controlled trials) on efficient science funding.
Challenge and Opportunity
The NIH and NSF together disburse tens of billions of dollars each year in the form of competitive research grants. At a high level, the funding process typically works like this: researchers submit detailed proposals for scientific studies, often to particular program areas or topics that have designated funding. Then, expert panels assembled by the funding agency read and score the proposals. These scores are used to decide which proposals will or will not receive funding. (The FAQ provides more details on how the NIH and NSF review competitive research grants.)
A growing number of scholars have advocated for reforming this process to address perceived inefficiencies and biases. Citing evidence that the NIH has become increasingly incremental in its funding decisions, for instance, commentators have called on federal funding agencies to explicitly fund riskier science. These calls grew louder following the success of mRNA vaccines against COVID-19, a technology that struggled for years to receive federal funding due to its high-risk profile.
Others are concerned that the average NIH grant-winner has become too old, especially in light of research suggesting that some scientists do their best work before turning 40. Still others lament the “crippling demands” that grant applications exert on scientists’ time, and argue that a better approach could be to replace or supplement conventional peer-review evaluations with lottery-based mechanisms.
These hypotheses are all reasonable and thought-provoking. Yet there exists surprisingly little empirical evidence to support these theories. If we want to effectively reimagine—or even just tweak—the way the United States funds science, we need better data on how well various funding policies work.
Academics and policymakers interested in the science of science have rightly called for increased experimentation with grantmaking policies in order to build this evidence base. But, realistically, such experiments would likely need to be conducted hand-in-hand with the institutions that fund and support science, investigating how changes in policies and practices shape outcomes. While there is progress in such experimentation becoming a reality, the knowledge gap about how best to support science would ideally be filled sooner rather than later.
Fortunately, we need not wait that long for new insights. The NIH and NSF have a powerful resource at their disposal: decades of historical data on grant proposals, scores, funding status, and eventual research outcomes. These data hold immense value for those investigating the comparative benefits of various science-funding strategies. Indeed, these data have already supported excellent and policy-relevant research. Examples include Ginther et. al (2011) which studies how race and ethnicity affect the probability of receiving an NIH award, and Myers (2020), which studies whether scientists are willing to change the direction of their research in response to increased resources. And there is potential for more. While randomized control trials (RCTs) remain the gold standard for assessing causal inference, economists have for decades been developing methods for drawing causal conclusions from observational data. Applying these methods to federal grantmaking data could quickly and cheaply yield evidence-based recommendations for optimizing federal science funding.
Opening up federal grantmaking data by providing a structured and streamlined access protocol would increase the supply of valuable studies such as those cited above. It would also build on growing governmental interest in evidence-based policymaking. Since its first week in office, the Biden-Harris administration has emphasized the importance of ensuring that “policy and program decisions are informed by the best-available facts, data and research-backed information.” Landmark guidance issued in August 2022 by the White House Office of Science and Technology Policy directs agencies to ensure that federally funded research—and underlying research data—are freely available to the public (i.e., not paywalled) at the time of publication.
On the legislative side, the 2018 Foundations for Evidence-based Policymaking Act (popularly known as the Evidence Act) calls on federal agencies to develop a “systematic plan for identifying and addressing policy questions” relevant to their missions. The Evidence Act specifies that the general public and researchers should be included in developing these plans. The Evidence Act also calls on agencies to “engage the public in using public data assets [and] providing the public with the opportunity to request specific data assets to be prioritized for disclosure.” The recently proposed Secure Research Data Network Act calls for building exactly the type of infrastructure that would be necessary to share federal grantmaking data in a secure and structured way.
Plan of Action
There is clearly appetite to expand access to and use of federally held evidence assets. Below, we recommend four actions for unlocking the insights contained in NIH- and NSF-held grantmaking data—and applying those insights to improve how federal agencies fund science.
Recommendation 1. Review legal and regulatory frameworks applicable to federally held grantmaking data.
The White House Office of Management and Budget (OMB)’s Evidence Team, working with the NIH’s Office of Data Science Strategy and the NSF’s Evaluation and Assessment Capability, should review existing statutory and regulatory frameworks to see whether there are any legal obstacles to sharing federal grantmaking data. If the review team finds that the NIH and NSF face significant legal constraints when it comes to sharing these data, then the White House should work with Congress to amend prevailing law. Otherwise, OMB—in a possible joint capacity with the White House Office of Science and Technology Policy (OSTP)—should issue a memo clarifying that agencies are generally permitted to share federal grantmaking data in a secure, structured way, and stating any categorical exceptions.
Recommendation 2. Build the infrastructure to provide external stakeholders with secure, structured access to federally held grantmaking data for research.
Federal grantmaking data are inherently sensitive, containing information that could jeopardize personal privacy or compromise the integrity of review processes. But even sensitive data can be responsibly shared. The NIH has previously shared historical grantmaking data with some researchers, but the next step is for the NIH and NSF to develop a system that enables broader and easier researcher access. Other federal agencies have developed strategies for handling highly sensitive data in a systematic fashion, which can provide helpful precedent and lessons. Examples include:
- The U.S. Census Bureau (USCB)’s Longitudinal Employer-Household Data. These data link individual workers to their respective firms, and provide information on salary, job characteristics, and worker and firm location. Approved researchers have relied on these data to better understand labor-market trends.
- The Department of Transportation (DOT)’s Secure Data Commons. The Secure Data Commons allows third-party firms (such as Uber, Lyft, and Waze) to provide individual-level mobility data on trips taken. Approved researchers have used these data to understand mobility patterns in cities.
In both cases, the data in question are available to external researchers contingent on agency approval of a research request that clearly explains the purpose of a proposed study, why the requested data are needed, and how those data will be managed. Federal agencies managing access to sensitive data have also implemented additional security and privacy-preserving measures, such as:
- Only allowing researchers to access data via a remote server, or in some cases, inside a Federal Statistical Research Data Center. In other words, the data are never copied onto a researcher’s personal computer.
- Replacing any personal identifiers with random number identifiers once any data merges that require personal identifiers are complete.
- Reviewing any tables or figures prior to circulating or publishing results, to ensure that all results are appropriately aggregated and that no individual-level information can be inferred.
Building on these precedents, the NIH and NSF should (ideally jointly) develop secure repositories to house grantmaking data. This action aligns closely with recommendations from the U.S. Commission on Evidence-Based Policymaking, as well as with the above-referenced Secure Research Data Network Act (SRDNA). Both the Commission recommendations and the SRDNA advocate for secure ways to share data between agencies. Creating one or more repositories for federal grantmaking data would be an action that is simultaneously narrower and broader in scope (narrower in terms of the types of data included, broader in terms of the parties eligible for access). As such, this action could be considered either a precursor to or an expansion of the SRDNA, and could be logically pursued alongside SRDNA passage.
Once a secure repository is created, the NIH and NSF should (again, ideally jointly) develop protocols for researchers seeking access. These protocols should clearly specify who is eligible to submit a data-access request, the types of requests that are likely to be granted, and technical capabilities that the requester will need in order to access and use the data. Data requests should be evaluated by a small committee at the NIH and/or NSF (depending on the precise data being requested). In reviewing the requests, the committee should consider questions such as:
- How important and policy-relevant is the question that the researcher is seeking to answer? If policymakers knew the answer, what would they do with that information? Would it inform policy in a meaningful way?
- How well can the researcher answer the question using the data they are requesting? Can they establish a clear causal relationship? Would we be comfortable relying on their conclusions to inform policy?
Finally, NIH and NSF should consider including right-to-review clauses in agreements governing sharing of grantmaking data. Such clauses are typical when using personally identifiable data, as they give the data provider (here, the NIH and NSF) the chance to ensure that all data presented in the final research product has been properly aggregated and no individuals are identifiable. The Census Bureau’s Disclosure Review Board can provide some helpful guidance for NIH and NSF to follow on this front.
Recommendation 3. Encourage researchers to utilize these newly available data, and draw on the resulting research to inform possible improvements to grant funding.
The NIH and NSF frequently face questions and trade-offs when deciding if and how to change existing grantmaking processes. Examples include:
- How can we identify promising early-career researchers if they have less of a track record? What signals should we look for?
- Should we cap the amount of federal funding that individual scientists can receive, or should we let star researchers take on more grants? In general, is it better to spread funding across more researchers or concentrate it among star researchers?
- Is it better to let new grantmaking agencies operate independently, or to embed them within larger, existing agencies?
Typically, these agencies have very little academic or empirical evidence to draw on for answers. A large part of the problem has been the lack of access to data that researchers need to conduct relevant studies. Expanding access, per Recommendations 1 and 2 above, is a necessary part of but not a sufficient solution. Agencies must also invest in attracting researchers to use the data in a socially useful way.
Broadly advertising the new data will be critical. Announcing a new request for proposals (RFP) through the NIH and/or the NSF for projects explicitly using the data could also help. These RFPs could guide researchers toward the highest-impact and most policy-relevant questions, such as those above. The NSF’s “Science of Science: Discovery, Communication and Impact” program would be a natural fit to take the lead on encouraging researchers to use these data.
The goal is to create funding opportunities and programs that give academics clarity on the key issues and questions that federal grantmaking agencies need guidance on, and in turn the evidence academics build should help inform grantmaking policy.
Conclusion
Basic science is a critical input into innovation, which in turn fuels economic growth, health, prosperity, and national security. The NIH and NSF were founded with these critical missions in mind. To fully realize their missions, the NIH and NSF must understand how to maximize scientific return on federal research spending. And to help, researchers need to be able to analyze federal grantmaking data. Thoughtfully expanding access to this key evidence resource is a straightforward, low-cost way to grow the efficiency—and hence impact—of our federally backed national scientific enterprise.
For an excellent discussion of this question, see Li (2017). Briefly, the NIH is organized around 27 “Institutes or Centers” (ICs) which typically correspond to disease areas or body systems. ICs have budgets each year that are set by Congress. Research proposals are first evaluated by around 180 different “study sections”, which are committees organized by scientific areas or methods. After being evaluated by the study sections, proposals are returned to their respective ICs. The highest-scoring proposals in each IC are funded, up to budget limits.
Research proposals are typically submitted in response to announced funding opportunities, which are organized around different programs (topics). Each proposal is sent by the Program Officer to at least three independent reviewers who do not work at the NSF. These reviewers judge the proposal on its Intellectual Merit and Broader Impacts. The Program Officer then uses the independent reviews to make a funding recommendation to the Division Director, who makes the final award/decline decision. More details can be found on the NSF’s webpage.
The NIH and NSF both provide data on approved proposals. These data can be found on the RePORTER site for the NIH and award search site for the NSF. However, these data do not provide any information on the rejected applications, nor do they provide information on the underlying scores of approved proposals.
To understand the range of governmental priorities for the bioeconomy, we spoke with key agencies represented on the National Bioeconomy Board to collect their perspectives.
Congress should foster a more responsive and evidence-based ecosystem for GenAI-powered educational tools, ensuring that they are equitable, effective, and safe for all students.
Without independent research, we do not know if the AI systems that are being deployed today are safe or if they pose widespread risks that have yet to be discovered, including risks to U.S. national security.
Companies that store children’s voice recordings and use them for profit-driven applications without parental consent pose serious privacy threats to children and families.