Social Media Conversations in Support of Herd Immunity are Driven by Bots
Key highlights
- Approximately half of the profiles pushing the case for herd immunity are artificial accounts. These bot or bot-like accounts are generally characterized as engaging in abnormally high levels of retweets and low content diversity.
- The high level of bot-like behavior attributed to support for the Great Barrington Declaration on social media indicates the conversation is manipulated and inorganic in comparison to the scientific consensus-based conversation opposing herd immunity theories. A consequence of high frequency of inorganic activity is the creation of a majority illusion (when certain members within a social network give the appearance that an idea or opinion is more popular than it is).
Online debate surrounding herd immunity shows one-sided automation
For months, debates about so-called herd immunity have occurred internationally. The idea behind this herd immunity strategy is to shield vulnerable populations while allowing COVID-19 transmission to go uncontrolled among less vulnerable populations. This strategy has been dismissed by many scientists for several ethical and practical reasons, such as the large size of vulnerable populations in countries like the US, and a lack of full understanding of longer-term impacts of SARS-CoV-2 on lower-risk groups. However, the conversation has increased in support, partially due to the embrace of the concept by a senior advisor to President Trump, Dr. Scott Atlas, who has called for the US to attempt the strategy. Support for the strategy has been outlined by a small group of public health scientists in the Great Barrington Declaration.
On the other side of the debate, prominent scientists like Dr. Fauci have called herd immunity through natural infection “dangerous” and “nonsense”. Infectious disease experts speculate this strategy could result in two million unnecessary deaths. Another group of scientists have recently published a correspondence in the Lancet, as well as an accompanying John Snow Memorandum, as an alternative to the Great Barrington Declaration.
About Topic Modeling
In our study of hundreds of thousands of tweets, we found a robust conversation related to the issues of herd immunity and the Great Barrington Declaration. To better understand the conversation we applied a technique known as topic modeling. A topic model is a frequently-used text-mining approach that applies statistical analysis of words and phrases across a particular conversation to discover patterns and common themes pertaining to the discussion. We first extracted public social data on text surrounding The Great Barrington conversation, searching for tweets with the terms such as “Barrington”, “Barrington Declaration”, and “focused protection”. We then applied a topic modeling method known as Latent Dirichlet Allocation (LDA) to discover how the conversation clusters into distinct topics.
Results
Through LDA Topic Modeling, we identified three distinct clusters surrounding the pro-herd immunity conversation and three distinct conversations surrounding opposition to herd immunity. The three cluster or distinct topics of conversation surrounding the pro-herd immunity conversation are: 1) Ending lockdowns; 2) political opposition to epidemiological social controls; and 3) support for the Great Barrington Declaration and the scientists who signed the document. The sentiment surrounding the Great Barrington Declaration conversation is largely positive, highlighting the support for this herd immunity strategy within online conversation. However, the conversation demonstrates evidence of significant bot behavior, specifically in the timing of tweets and topic clustering with low content diversity. In fact, when conducting bot-detection analysis, we discovered an estimated 45% of messages surrounding the Great Barrington Declaration are likely bot-driven. Meanwhile, the conversation opposing herd immunity has much greater diversity, is generally organic and not heavily bot-driven. The topic clusters consisted of: 1) Criticism of senior U.S. officials pushing herd immunity, 2) the high potential mortality required to attempt herd immunity, and 3) the ongoing mask debate, and vaccine development timelines. This more organic conversation is highly negative towards the concept of herd immunity.
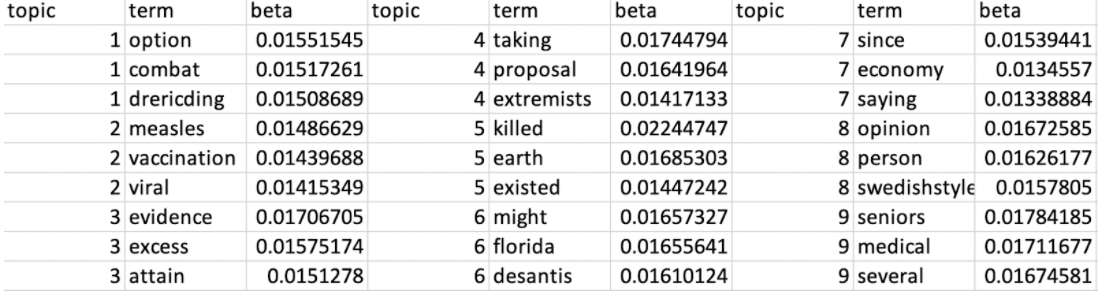
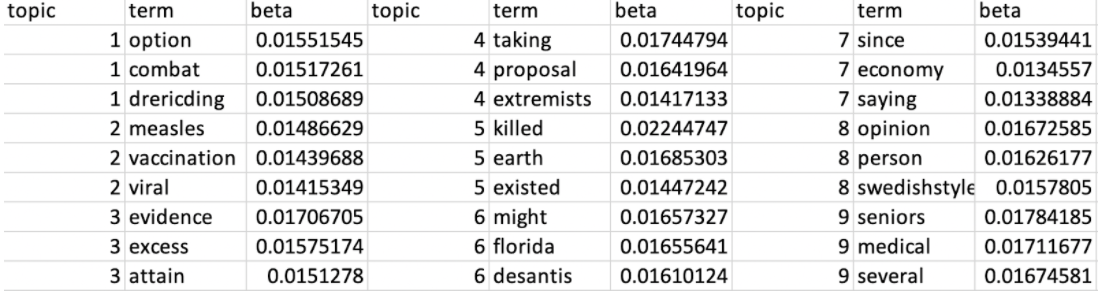
Detection of Bot-Activity
We assessed the conversation for signs of automation and other artificial signatures (i.e. bot activity). Given the volume of the conversation and the limitations placed on commercial bot detection systems, we took a functional approach to this analysis and focused on identifying periods of the conversation in which a large volume of similar content was posted at the same time. Using one-second breaks, each tweet was plotted by timestamp. Next, anomaly detection was run against a subset of these data that captured the number of tweets per second and anomalous times were identified as one second periods that were statistical outliers with high levels of tweet activity. The red labeled dots in Figure 3 represent anomalous tweets within these identified periods. These tweets consist of retweeted messages, repeated hashtags signifying inorganic activity. We subset tweets labeled as taking place in anomalous periods, whether they contained original or repeated content, labeled them as originating from either the Barrington or herd immunity conversation, and extracted the user data from the tweet. Finally, user data was analyzed for indications of account automation, or bot behavior. We find that an estimated 45% of tweets in the Great Barrington Declaration conversion, with content supporting the idea of herd immunity, were posted by bots. Meanwhile, 22% of the herd immunity conversation is bot driven, less than half the levels of the Great Barrington conversation, and closer to the approximately 15% automation levels found on average in twitter conversation.
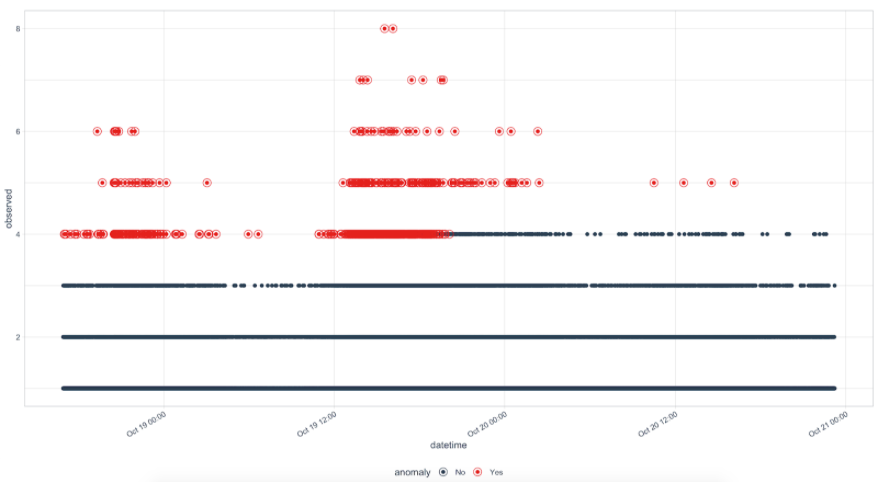
We find the conversation related to herd immunity in general to be driven by real accounts and maintaining both conversational diversity with an overall negative sentiment, while breakout analysis of the Great Barrington Declaration conversation consists of nearly half artificial accounts with high levels of retweets, low content diversity, and an overall positive sentiment related to the document. This suggests the conversation supporting herd immunity on Twitter is artificially driven while real accounts engaged in the conversation maintain a negative view of the issue.
The 45% artificiality rate for the Great Barrington is high compared to the 9-15% rate of bots broadly on Twitter. Because bot saturation is relative to a given conversation, it is difficult to have a universal statistical baseline for what level of artificial behavior is abnormal. Instead, the comparison of the percentage of artificial behavior between corpuses is more meaningful.
Artificial promotion of the Great Barrington Declaration suggests that sponsors of the herd immunity strategy are seeking to foster non-expert support over the support of scientists, doctors, and public health officials. While reasons must be explored further, it can be speculated that generating popular support for the strategy against the advice for experts is the fastest way to promote ending closures, partial and full lockdowns, and perhaps other public health measures such as the wearing of face masks as many are growing inpatient with restricted social activity, and other containment strategies.
Methodology
Using open-source investigation techniques, we performed a combination of social network analysis, and anomalous behavior discovery regarding herd immunity. We analyzed a total of 180,578 tweets in the herd immunity conversation, using key terms like “herd immunity”, “Barrington”, and “focused protection.” Out of the 180,578 tweets we sampled, there were 41,870 unique tweets attributed to and shared by 83,765 users.
A breakdown of the tweets is below:
Tweets analyzed:
75,557 tweets with search term “Barrington Declaration”
105,021 tweets with search term “herd immunity”
Unique tweets:
14,507 tweets with the search term “Barrington”
Herd immunity 41,870
Unique users:
73,931 tweets with the search term “Herd”
9,834 tweets with the search term “Barrington”
For more about the FAS Disinformation Research Group and to see previous reports, visit the project page here.
____________________________
* Above are the terms comprising characterizing the clusters 1-3 of pro-herd immunity conversation (left to right). The beta is an indicator of the topic-word density, measured by word frequency or repeating pattern of co-occurring terms. The higher the beta, the higher the frequency of words or phrases.
** Above are the terms comprising characterizing the clusters 1-3 on opposition to herd immunity (left to right). The beta is an indicator of the topic-word density, measured by word frequency or repeating pattern of co-occurring terms. The higher the beta, the higher the frequency of words or phrases.
*** The image above displays frequency of messaging across the herd immunity conversation. The red dots display higher unusual frequency of messaging based on either high frequency in tweeting the same message or high frequency of tweets emanating from the same account. The horizontal axis represents tweets over time.
The Federation of American Scientists applauds the United States for declassifying the number of nuclear warheads in its military stockpile and the number of retired and dismantled warheads.
North Korea may have produced enough fissile material to build up to 90 nuclear warheads.
Secretary Austin’s likely certification of the Sentinel program should be open to public interrogation, and Congress must thoroughly examine whether every requirement is met before allowing the program to continue.
Researchers have many questions about the modernization of Pakistan’s nuclear-capable aircraft and associated air-launched cruise missiles.