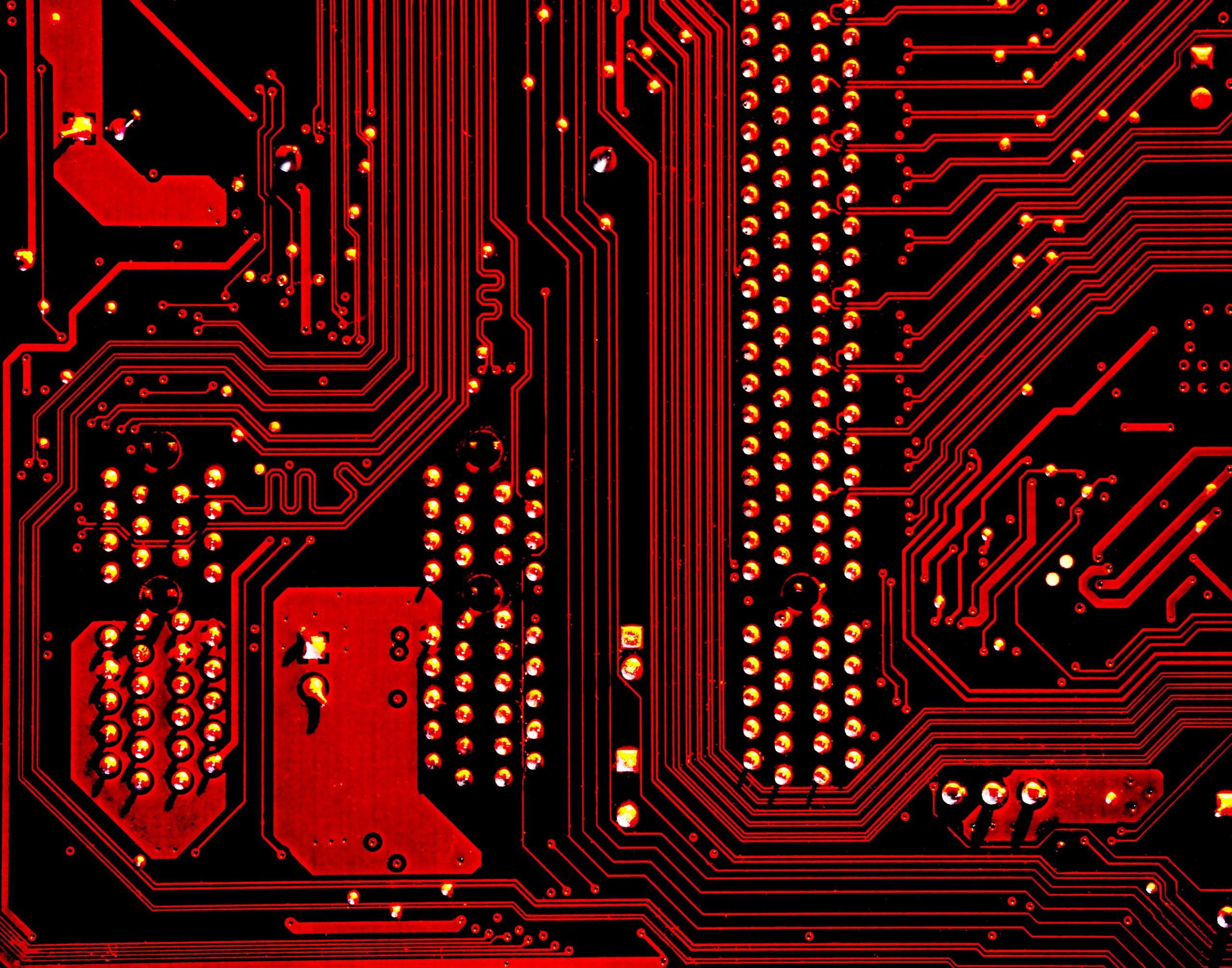
Leveraging Machine Learning To Reduce Cost & Burden of Reviewing Research Proposals at S&T Agencies
Summary
With about $130 billion USD, the United States leads the world in federal research and development spending. Most of this spending is distributed by science and technology (S&T) agencies that use internal reviews to identify the best proposals submitted in response to competitive funding opportunities. As stewards of quality scientific research, part of each funding agency’s mission is to ensure fairness, transparency, and integrity in the proposal-review process. Manual proposal review is time-consuming and expensive, costing an estimated $300 million annually at the National Science Foundation alone. Yet at current proposal-success rates (between 5% and 20% for most funding opportunities), a substantial fraction of proposals reviewed are simply not competitive.
The next administration should initiate and execute a plan to advance machine learning to triage scientific proposals. This proposal presents a set of actions and a vision to diffuse machine-learning across science and technology agencies to ultimately become a standard component of proposal review, while improving the efficiency of the funding process without compromising the quality of funded research.
To understand the range of governmental priorities for the bioeconomy, we spoke with key agencies represented on the National Bioeconomy Board to collect their perspectives.
Congress should foster a more responsive and evidence-based ecosystem for GenAI-powered educational tools, ensuring that they are equitable, effective, and safe for all students.
Without independent research, we do not know if the AI systems that are being deployed today are safe or if they pose widespread risks that have yet to be discovered, including risks to U.S. national security.
Companies that store children’s voice recordings and use them for profit-driven applications without parental consent pose serious privacy threats to children and families.