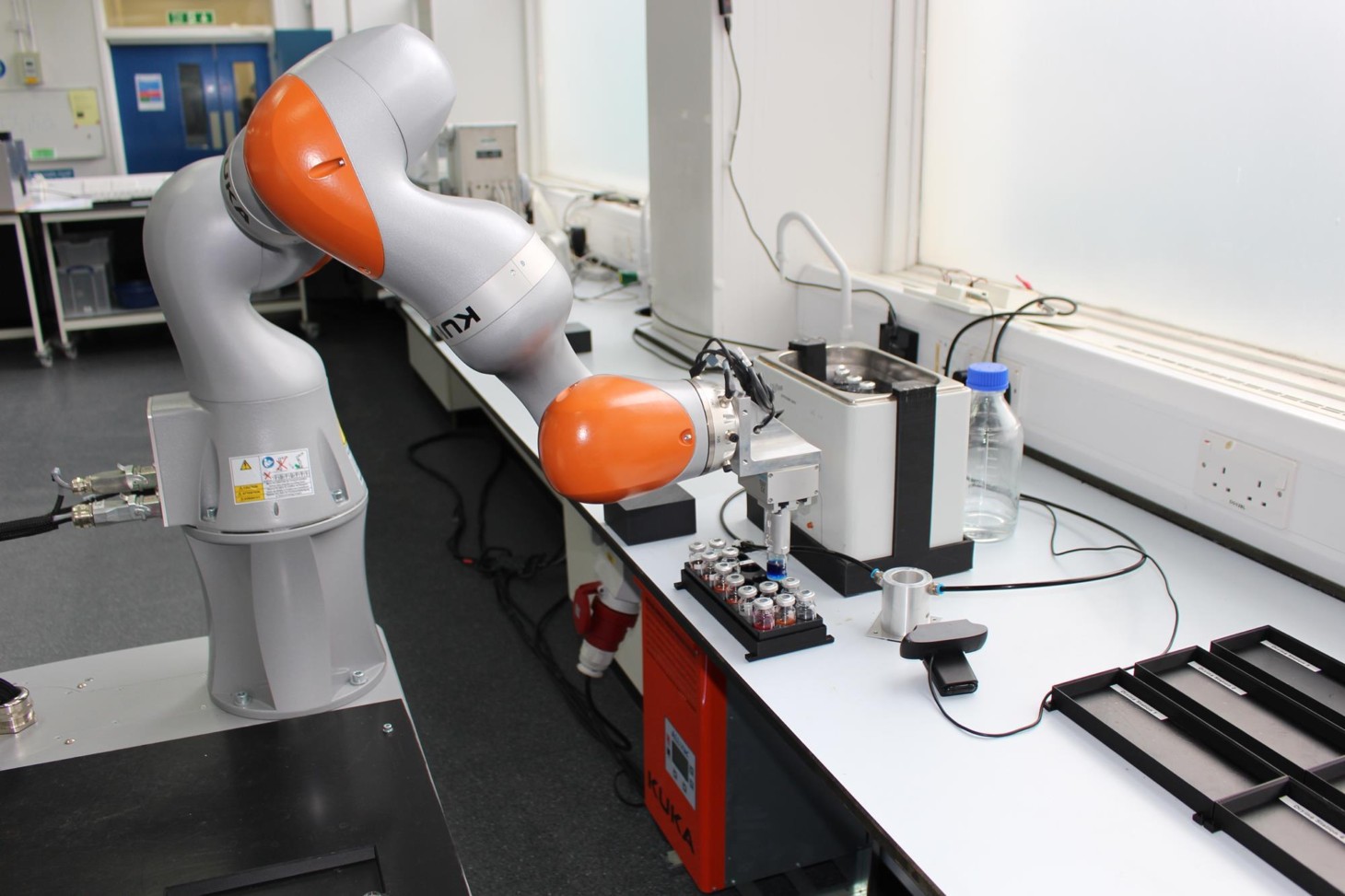
Automating Scientific Discovery: A Research Agenda for Advancing Self-Driving Labs
Despite significant advances in scientific tools and methods, the traditional, labor-intensive model of scientific research in materials discovery has seen little innovation. The reliance on highly skilled but underpaid graduate students as labor to run experiments hinders the labor productivity of our scientific ecosystem. An emerging technology platform known as Self-Driving Labs (SDLs), which use commoditized robotics and artificial intelligence for automated experimentation, presents a potential solution to these challenges.
SDLs are not just theoretical constructs but have already been implemented at small scales in a few labs. An ARPA-E-funded Grand Challenge could drive funding, innovation, and development of SDLs, accelerating their integration into the scientific process. A Focused Research Organization (FRO) can also help create more modular and open-source components for SDLs and can be funded by philanthropies or the Department of Energy’s (DOE) new foundation. With additional funding, DOE national labs can also establish user facilities for scientists across the country to gain more experience working with autonomous scientific discovery platforms. In an era of strategic competition, funding emerging technology platforms like SDLs is all the more important to help the United States maintain its lead in materials innovation.
Challenge and Opportunity
New scientific ideas are critical for technological progress. These ideas often form the seed insight to creating new technologies: lighter cars that are more energy efficient, stronger submarines to support national security, and more efficient clean energy like solar panels and offshore wind. While the past several centuries have seen incredible progress in scientific understanding, the fundamental labor structure of how we do science has not changed. Our microscopes have become far more sophisticated, yet the actual synthesizing and testing of new materials is still laboriously done in university laboratories by highly knowledgeable graduate students. The lack of innovation in how we historically use scientific labor pools may account for stagnation of research labor productivity, a primary cause of concerns about the slowing of scientific progress. Indeed, analysis of scientific literature suggests that scientific papers are becoming less disruptive over time and that new ideas are getting harder to find. The slowing rate of new scientific ideas, particularly in the discovery of new materials or advances in materials efficiency, poses a substantial risk, potentially costing billions of dollars in economic value and jeopardizing global competitiveness. However, incredible advances in artificial intelligence (AI) coupled with the rise of cheap but robust robot arms are leading to a promising new paradigm of material discovery and innovation: Self-Driving Labs. An SDL is a platform where material synthesis and characterization is done by robots, with AI models intelligently selecting new material designs to test based on previous experimental results. These platforms enable researchers to rapidly explore and optimize designs within otherwise unfeasibly large search spaces.
Today, most material science labs are organized around a faculty member or principal investigator (PI), who manages a team of graduate students. Each graduate student designs experiments and hypotheses in collaboration with a PI, and then executes the experiment, synthesizing the material and characterizing its property. Unfortunately, that last step is often laborious and the most time-consuming. This sequential method to material discovery, where highly knowledgeable graduate students spend large portions of their time doing manual wet lab work, rate limits the amount of experiments and potential discoveries by a given lab group. SDLs can significantly improve the labor productivity of our scientific enterprise, freeing highly skilled graduate students from menial experimental labor to craft new theories or distill novel insights from autonomously collected data. Additionally, they yield more reproducible outcomes as experiments are run by code-driven motors, rather than by humans who may forget to include certain experimental details or have natural variations between procedures.
Self-Driving Labs are not a pipe dream. The biotech industry has spent decades developing advanced high-throughput synthesis and automation. For instance, while in the 1970s statins (one of the most successful cholesterol-lowering drug families) were discovered in part by a researcher testing 3800 cultures manually over a year, today, companies like AstraZeneca invest millions of dollars in automation and high-throughput research equipment (see figure 1). While drug and material discovery share some characteristics (e.g., combinatorially large search spaces and high impact of discovery), materials R&D has historically seen fewer capital investments in automation, primarily because it sits further upstream from where private investments anticipate predictable returns. There are, however, a few notable examples of SDLs being developed today. For instance, researchers at Boston University used a robot arm to test 3D-printed designs for uniaxial compression energy adsorption, an important mechanical property for designing stronger structures in civil engineering and aerospace. A Bayesian optimizer was then used to iterate over 25,000 designs in a search space with trillions of possible candidates, which led to an optimized structure with the highest recorded mechanical energy adsorption to date. Researchers at North Carolina State University used a microfluidic platform to autonomously synthesize >100 quantum dots, discovering formulations that were better than the previous state of the art in that material family.
These first-of-a-kind SDLs have shown exciting initial results demonstrating their ability to discover new material designs in a haystack of thousands to trillions of possible designs, which would be too large for any human researcher to grasp. However, SDLs are still an emerging technology platform. In order to scale up and realize their full potential, the federal government will need to make significant and coordinated research investments to derisk this materials innovation platform and demonstrate the return on capital before the private sector is willing to invest it.
Other nations are beginning to recognize the importance of a structured approach to funding SDLs: University of Toronto’s Alan Aspuru-Guzik, a former Harvard professor who left the United States in 2018, has created an Acceleration Consortium to deploy these SDLs and recently received $200 million in research funding, Canada’s largest ever research grant. In an era of strategic competition and climate challenges, maintaining U.S. competitiveness in materials innovation is more important than ever. Building a strong research program to fund, build, and deploy SDLs in research labs should be a part of the U.S. innovation portfolio.
Plan of Action
While several labs in the United States are working on SDLs, they have all received small, ad-hoc grants that are not coordinated in any way. A federal government funding program dedicated to self-driving labs does not currently exist. As a result, the SDLs are constrained to low-hanging material systems (e.g., microfluidics), with the lack of patient capital hindering labs’ ability to scale these systems and realize their true potential. A coordinated U.S. research program for Self-Driving Labs should:
Initiate an ARPA-E SDL Grand Challenge: Drawing inspiration from DARPA’s previous grand challenges that have catalyzed advancements in self-driving vehicles, ARPA-E should establish a Grand Challenge to catalyze state-of-the-art advancements in SDLs for scientific research. This challenge would involve an open call for teams to submit proposals for SDL projects, with a transparent set of performance metrics and benchmarks. Successful applicants would then receive funding to develop SDLs that demonstrate breakthroughs in automated scientific research. A projected budget for this initiative is $30 million1, divided among six selected teams, each receiving $5 million over a four-year period to build and validate their SDL concepts. While ARPA-E is best positioned in terms of authority and funding flexibility, other institutions like National Science Foundation (NSF) or DARPA itself could also fund similar programs.
Establish a Focused Research Organization to open-source SDL components: This FRO would be responsible for developing modular, open-source hardware and software specifically designed for SDL applications. Creating common standards for both the hardware and software needed for SDLs will make such technology more accessible and encourage wider adoption. The FRO would also conduct research on how automation via SDLs is likely to reshape labor roles within scientific research and provide best practices on how to incorporate SDLs into scientific workflows. A proposed operational timeframe for this organization is five years, with an estimated budget of $18 million over that time period. The organization would work on prototyping SDL-specific hardware solutions and make them available on an open-source basis to foster wider community participation and iterative improvement. A FRO could be spun out of the DOE’s new Foundation for Energy Security (FESI), which would continue to establish the DOE’s role as an innovative science funder and be an exciting opportunity for FESI to work with nontraditional technical organizations. Using FESI would not require any new authorities and could leverage philanthropic funding, rather than requiring congressional appropriations.
Provide dedicated funding for the DOE national labs to build self-driving lab user facilities, so the United States can build institutional expertise in SDL operations and allow other U.S. scientists to familiarize themselves with these platforms. This funding can be specifically set aside by the DOE Office of Science or through line-item appropriations from Congress. Existing prototype SDLs, like the Argonne National Lab Rapid Prototyping Lab or Berkeley Lab’s A-Lab, that have emerged in the past several years lack sustained DOE funding but could be scaled up and supported with only $50 million in total funding over the next five years. SDLs are also one of the primary applications identified by the national labs in the “AI for Science, Energy, and Security” report, demonstrating willingness to build out this infrastructure and underscoring the recognized strategic importance of SDLs by the scientific research community.
As with any new laboratory technique, SDLs are not necessarily an appropriate tool for everything. Given that their main benefit lies in automation and the ability to rapidly iterate through designs experimentally, SDLs are likely best suited for:
- Material families with combinatorially large design spaces that lack clear design theories or numerical models (e.g., metal organic frameworks, perovskites)
- Experiments where synthesis and characterization are either relatively quick or cheap and are amenable to automated handling (e.g., UV-vis spectroscopy is relatively simple, in-situ characterization technique)
- Scientific fields where numerical models are not accurate enough to use for training surrogate models or where there is a lack of experimental data repositories (e.g., the challenges of using density functional theory in material science as a reliable surrogate model)
While these heuristics are suggested as guidelines, it will take a full-fledged program with actual results to determine what systems are most amenable to SDL disruption.
When it comes to exciting new technologies, there can be incentives to misuse terms. Self-Driving Labs can be precisely defined as the automation of both material synthesis and characterization that includes some degree of intelligent, automated decision-making in-the-loop. Based on this definition, here are common classes of experiments that are not SDLs:
- High-throughput synthesis, where synthesis automation allows for the rapid synthesis of many different material formulations in parallel (lacks characterization and AI-in-the-loop)
- Using AI as a surrogate trained over numerical models, which is based on software-only results. Using an AI surrogate model to make material predictions and then synthesizing an optimal material is also not a SDL, though certainly still quite the accomplishment for AI in science (lacks discovery of synthesis procedures and requires numerical models or prior existing data, neither of which are always readily available in the material sciences).
SDLs, like every other technology that we have adopted over the years, eliminate routine tasks that scientists must currently spend their time on. They will allow scientists to spend more time understanding scientific data, validating theories, and developing models for further experiments. They can automate routine tasks but not the job of being a scientist.
However, because SDLs require more firmware and software, they may favor larger facilities that can maintain long-term technicians and engineers who maintain and customize SDL platforms for various applications. An FRO could help address this asymmetry by developing open-source and modular software that smaller labs can adopt more easily upfront.
To understand the range of governmental priorities for the bioeconomy, we spoke with key agencies represented on the National Bioeconomy Board to collect their perspectives.
Congress should foster a more responsive and evidence-based ecosystem for GenAI-powered educational tools, ensuring that they are equitable, effective, and safe for all students.
Without independent research, we do not know if the AI systems that are being deployed today are safe or if they pose widespread risks that have yet to be discovered, including risks to U.S. national security.
Companies that store children’s voice recordings and use them for profit-driven applications without parental consent pose serious privacy threats to children and families.