Three Artificial Intelligence Bills Endorsed by Federation of American Scientists Advance from the House Committee
Proposed bills advance research ecosystems, economic development, and education access and move now to the U.S. House of Representatives for a vote
Washington, D.C. – September 12, 2024 – Three proposed artificial intelligence bills endorsed by the Federation of American Scientists (FAS), a nonpartisan science think tank, advance forward from a House Science, Space, and Technology Committee markup held on September 11th, 2024. These bills received bipartisan support and will now be reported to the full chamber. The three bills are: H.R. 9403, the Expanding AI Voices Act, co-sponsored by Rep. Vince Fong (CA-20) and Rep. Andrea Salinas (OR-06); H.R. 9197, the Small Business AI Act, co-sponsored by Rep. Mike Collins (GA-10) and Rep. Haley Stevens (MI-11), and H.R. 9403, the Expand AI Act, co-sponsored by Rep. Valerie Foushee (NC-04) and Rep. Frank Lucas (OK-03).
“FAS endorsed these bills based on the evaluation of their strengths. Among these are the development of infrastructure to develop AI safely and responsibly; the deployment of resources to ensure development benefits more equitably across our economy; and investment in the talent pool necessary for this consequential, emerging technology,” says Dan Correa, CEO of FAS.
“These three bills pave a vision for the equitable and safe use of AI in the U.S. Both the Expanding AI Voices Act and the NSF AI Education Act will create opportunities for underrepresented voices to have a say in how AI is developed and deployed. Additionally, the Small Business AI Act will ensure that an important sector of our society feels empowered to use AI safely and securely,” says Clara Langevin, FAS AI Policy Specialist.
Expanding AI Voices Act
The Expanding AI Voices Act will support a broad and diverse interdisciplinary research community for the advancement of artificial intelligence and AI-powered innovation through partnerships and capacity building at certain institutions of higher education to expand AI capacity in populations historically underrepresented in STEM.
Specifically, the Expanding AI Voices Act of 2024 will:
- Codify and expand the ExpandAI program at the National Science Foundation (NSF), which supports artificial intelligence (AI) capacity-building projects for eligible entities including Minority Serving Institutions (MSIs), Historically Black Colleges and Universities (HBCUs), and Tribal Colleges and Universities (TCUs).
- Broaden the ExpandAI program in scope and types of activities it supports to further build and enhance partnerships between eligible entities and awardees of the National AI Research Institutes ecosystem to broaden AI research and development.
- Direct the National Science Foundation to engage in outreach to increase their pool of applications and address common barriers preventing these organizations from submitting an application.
Small Business AI Act
Emerging science is central to new and established small businesses, across industries and around the country. This bill will require the Director of the National Institute of Standards and Technology (NIST) to develop resources for small businesses in utilizing artificial intelligence, and for other purposes.
- This bill amends the NIST Organic Act, as amended by the National AI Initiative Act, and directs NIST, in coordination with the Small Business Administration, to consider the needs of America’s small businesses and develop AI resources for best practices, case studies, benchmarks, methodologies, procedures, and processes for small businesses to understand, apply, and integrate AI systems.
- It will connect Small Businesses with existing Federal educational resources, such as the risk management framework and activities from the national cybersecurity awareness and education program under the Cybersecurity Enhancement Act of 2014.
- This bill aligns with FAS’s mission to broaden AI use and access as a catalyst for economic development.
National Science Foundation Artificial Intelligence Education Act of 2024 (NSF AI Education Act).
The National Artificial Intelligence Initiative Act of 2020 (15 U.S.C. 9451) will bolster educational skills in AI through new learning initiatives and workforce training programs. Specifically, the bill will:
- Allow NSF to award AI scholarships in critical sectors such as education, agriculture and advanced manufacturing.
- Authorize the NSF to conduct outreach and encourage applications from rural institutions, Tribal Colleges and Universities, and institutions located in Established Program to Stimulate Competitive Research (EPSCoR) jurisdictions to promote research competitiveness.
- Award fellowships for teachers, school counselors, and other school professionals for professional development programs, providing skills and training in collaboration with industry partners on the teaching and application of artificial intelligence in K-12 settings
- This bill aligns with FAS’s commitment to STEM education and equity as powerful levers for our nation to compete on the global stage.
###
ABOUT FAS
The Federation of American Scientists (FAS) works to advance progress on a broad suite of contemporary issues where science, technology, and innovation policy can deliver dramatic progress, and seeks to ensure that scientific and technical expertise have a seat at the policymaking table. Established in 1945 by scientists in response to the atomic bomb, FAS continues to work on behalf of a safer, more equitable, and more peaceful world. More information at fas.org.
GenAI in Education Research Accelerator (GenAiRA)
The United States faces a critical challenge in addressing the persistent learning opportunity gaps in math and reading, particularly among disadvantaged student subgroups. According to the 2022 National Assessment of Educational Progress (NAEP) data, only 37% of fourth-grade students performed at or above the proficient level in math, and 33% in reading. The rapid advancement of generative AI (GenAI) technologies presents an unprecedented opportunity to bridge these gaps by providing personalized learning experiences and targeted support. However, the current mismatch between the speed of GenAI innovation and the lengthy traditional research pathways hinders the thorough evaluation of these technologies before widespread adoption, potentially leading to unintended negative consequences.
Failure to adapt our research and regulatory processes to keep pace with the development of GenAI technologies could expose students to ineffective or harmful educational tools, exacerbate existing inequities, and hinder our ability to prepare all students for success in an increasingly complex and technology-driven world. The education sector must act with urgency to establish the necessary infrastructure, expertise, and collaborative partnerships to ensure that GenAI-powered tools are rigorously evaluated, continuously improved, and equitably implemented to benefit all students.
To address this challenge, we propose three key recommendations for congressional action:
- Establish the GenAI in Education Research Accelerator Program (GenAiRA) within the Institute of Education Sciences (IES) to support and expedite efficacy research on GenAI-powered educational tools.
- Adapt IES research and evaluation processes to create a framework for the rapid assessment of GenAI-enabled educational technology, including alternative research designs and evidence standards.
- Support the establishment of a GenAI Education Research and Innovation Consortium, bringing together schools, researchers, and education technology (EdTech) developers to participate in rapid cycle studies and continuous improvement of GenAI tools.
By implementing these recommendations, Congress can foster a more responsive and evidence-based ecosystem for GenAI-powered educational tools, ensuring that they are equitable, effective, and safe for all students. This comprehensive approach will help unlock the transformative potential of GenAI to address persistent learning opportunity gaps and improve outcomes for all learners, while maintaining scientific rigor and prioritizing student well-being.
During the preparation of this work, the authors used the tool Claude 3 Opus (by Anthropic) to help clarify and synthesize, and add accessible language around concepts and ideas generated by members of the team. The authors reviewed and edited the content as needed and take full responsibility for the content of this publication.
Challenge and Opportunity
Widening Learning Opportunity Gap
NAEP data reveals that many U.S. students, especially those from disadvantaged subgroups, are not achieving proficiency in math and reading. In 2022, only 37% of fourth-graders performed at or above the NAEP proficient level in math, and 33% in reading—the lowest levels in over a decade. Disparities are more profound when disaggregated by race, ethnicity, and socioeconomic status; for example, only 17% of Black students and 21% of Hispanic students reached reading proficiency, compared to 42% of white students.
Rapid AI Evolution
GenAI is a transformative technology that enables rapid development and personalization of educational content and tools, addressing unmet needs in education such as lack of resources, 1:1 teaching time, and teacher quality. However, that rapid pace also raises concerns about premature adoption of unvetted tools, which could negatively impact students’ educational achievement. Unvetted GenAI tools may introduce misconceptions, provide incorrect guidance, or be misaligned with curriculum standards, leading to gaps in students’ understanding of foundational concepts. If used for an extended period, particularly with vulnerable learners, these tools could have a long-term impact on learning foundations that may be difficult to remedy.
On the other hand, carefully designed, trained, and vetted GenAI models that have undergone rapid cycle studies and design iterations based on data have the potential to effectively address students’ misconceptions, build solid learning foundations, and provide personalized, adaptive support to learners. These tools could accelerate progress and close learning opportunity gaps at an unprecedented scale.
Slow Vetting Processes
The rapid pace of AI development poses significant challenges for traditional research and evaluation processes in education. Efficacy research, particularly studies sponsored by the IES or other Department of Education entities, is a lengthy, resource-intensive, and often onerous process that can take years to complete. Randomized controlled trials and longitudinal studies struggle to keep up with the speed of AI innovation: by the time a study is completed, the AI-powered tool may have already undergone multiple iterations or been replaced.
It can be difficult to recruit and sustain school and teacher participation in efficacy research due to the significant time and effort required from educators. Moreover, obtaining certifications and approvals for research can be complex and time-consuming, as researchers must navigate institutional review boards, data privacy regulations, and ethical guidelines, which can delay the start of a study by months or even years.
Many EdTech developers find themselves in a catch-22 situation, where their products are already being adopted by schools and educators, yet they are simultaneously expected to participate in lengthy and expensive research studies to prove efficacy. The time and resources required to engage in such research can be a significant burden for EdTech companies, especially start-ups and small businesses, which may prefer to focus on iterating and improving their products based on real-world feedback. As a result, many EdTech developers may be reluctant to participate in traditional efficacy research, further exacerbating the disconnect between the rapid pace of AI innovation and the slow process of evaluating the effectiveness of these tools in educational settings.
Gaps in Existing Efforts and Programs
While federal initiatives like SEERNet and ExpandAI have made strides in supporting AI and education research and development, they may not be fully equipped to address the specific challenges and opportunities presented by GenAI for several reasons:
- GenAI has the ability to generate novel content and interact with users in unpredictable and personalized ways.
- GenAI-powered educational technologies involve unique considerations in terms of data training, prompt engineering, and output evaluation, especially when considering the developmental stages of PreK-12 students.
- GenAI raises specific ethical concerns, such as the potential for biased or inappropriate content generation, ensuring the accuracy and quality of generated responses, and protecting student privacy and agency.
- GenAI is evolving at an unprecedented pace.
Traditional approaches to efficacy research and evaluation may not be well-suited to evaluating the potential benefits and outcomes associated with GenAI-powered tools in the short term, particularly when assessing whether a program shows enough promise to warrant wider deployment with students.
A New Approach
To address these challenges and bridge the gap between GenAI innovation and efficacy research, we need a new approach to streamline the research process, reduce the burden on educators and schools, and provide timely and actionable insights into the effectiveness of GenAI-powered tools. This may involve alternative study designs, such as rapid cycle evaluations or single-case research, and developing new incentive structures and support systems to encourage and facilitate the participation of teachers, schools, and product developers in research studies.
GenAiRA aims to tackle these challenges by providing resources, guidance, and infrastructure to support more agile and responsive efficacy research in the education sciences. By fostering collaboration among researchers, developers, and educators, and promoting innovative approaches to evaluation, this program can help ensure that the development and adoption of AI-powered tools in education are guided by rigorous, timely, and actionable evidence—while simultaneously mitigating risks to students.
Learning from Other Sectors
Valuable lessons can be drawn from other fields that have faced similar balancing acts between innovation, research, and safety. Two notable examples are the U.S. Food and Drug Administration’s (FDA) expedited review pathways for drug development and the National Institutes of Health’s (NIH) Clinical and Translational Science Awards (CTSA) program for accelerating medical research.
Example 1: The FDA Model
The FDA’s expedited review programs, such as Fast Track, Breakthrough Therapy, Accelerated Approval, and Priority Review, are designed to speed up the development and approval of drugs that address unmet medical needs or provide significant improvements over existing treatments. These pathways recognize that, in certain cases, the benefits of bringing a potentially life-saving drug to market quickly may outweigh the risks associated with a more limited evidence base at the time of approval.
Key features include:
- Early and frequent communication between the FDA and drug developers to provide guidance and feedback throughout the development process.
- Flexibility in clinical trial design and evidence requirements, such as allowing the use of surrogate endpoints or single-arm studies in certain cases.
- Rolling review of application materials, allowing drug developers to submit portions of their application as they become available rather than waiting for the entire package to be complete.
- Shortened review timelines, with the FDA committing to reviewing and making a decision on an application within a specified timeframe (e.g., six months for Priority Review).
These features can accelerate the development and approval process while still ensuring that drugs meet standards for safety and effectiveness. They also acknowledge that the evidence base for a drug may evolve over time, with post-approval studies and monitoring playing a crucial role in confirming the drug’s benefits and identifying any rare or long-term side effects.
Example 2: The CTSA Program
The NIH’s CTSA program established a national network of academic medical centers, research institutions, and community partners to accelerate the translation of research findings into clinical practice and improve patient outcomes.
Key features include:
- Collaborative research infrastructure, consisting of a network of institutions and partners that work together to conduct translational research, share resources and expertise, and disseminate best practices.
- Streamlined research processes with standardized protocols, templates, and tools to facilitate the rapid design, approval, and implementation of research studies across the network.
- Training and development of researchers and clinicians to build a workforce equipped to conduct innovative and rigorous translational research.
- Community engagement in the research process to ensure that studies are responsive to real-world needs and priorities.
By learning from the successes and principles of the FDA’s expedited review pathways and the NIH’s CTSA program, the education sector can develop its own innovative approach to accelerating the responsible development, evaluation, and deployment of GenAI-powered tools, as outlined in the following plan of action.
Plan of Action
To address the challenges and opportunities presented by GenAI in education, we propose the following three key recommendations for congressional action and the evolution of existing programs.
Recommendation 1. Establish the GenAI in Education Research Accelerator Program (GenAiRA).
Congress should establish the GenAiRA, housed in the IES, to support and expedite efficacy research on products and tools utilizing AI-powered educational tools and programs. This program will:
- Provide funding and resources to researchers and educators to conduct rigorous, timely, and cost-effective efficacy studies on promising AI-based solutions that address achievement gaps.
- Create guidelines and offer webinars and technical assistance to researchers, educators, and developers to build expertise in the responsible design, implementation, and evaluation of GenAI-powered tools in education.
- Foster collaboration and knowledge-sharing among researchers, educators, and GenAI developers to facilitate the rapid translation of research findings into practice and continuously improve GenAI-powered tools.
- Develop and disseminate best practices, guidelines, and ethical frameworks for responsible development and deployment of GenAI-enabled educational technology tools in educational settings, focusing on addressing bias, accuracy, privacy, and student agency issues.
Recommendation 2. Under the auspices of GenAiRA, adapt IES research and evaluation processes to create a framework to evaluate GenAI-enabled educational technology.
In consultation with experts in educational research and AI, IES will develop a framework that:
- Identifies existing research designs and creates alternative research designs (e.g., quasi-experimental studies, rapid short evaluations) suitable for generating credible evidence of effectiveness while being more responsive to the rapid pace of AI innovation.
- Establish evidence-quality guidelines for rapid evaluation, including minimum sample sizes, study duration, effect size, and targeted population.
- Funds replication studies and expansion studies to determine impact in different contexts or with different populations (e.g., students with IEPs and English learners).
- Provides guidance to districts on how to interpret and apply evidence from different types of studies to inform decision-making around adopting and using AI technologies in education.
Recommendation 3. Establish a GenAI Education Research and Innovation Consortium.
Congress should provide funding and incentives for IES to establish a GenAI Education Research and Innovation Consortium that brings together a network of “innovation schools,” research institutions, and EdTech developers committed to participating in rapid cycle studies and continuous improvement of GenAI tools in education. This approach will ensure that AI tools are developed and implemented in a way that is responsive to the needs and values of educators, students, and communities.
To support this consortium, Congress should:
- Allocate funds for the IES to provide grants and resources to schools, research institutions, and EdTech developers that meet established criteria for participation in the consortium, such as demonstrated commitment to innovation, research capacity, and ethical standards.
- Direct IES to work with programs like SEERNet and ExpandAI to identify and match potential consortium members, provide guidance and oversight to ensure that research studies meet rigorous standards for quality and ethics, and disseminate findings and best practices to the broader education community.
- Encourage the development of standardized protocols and templates for data sharing, privacy protection, and informed consent within the consortium, to reduce the time and effort required for each individual study and streamline administrative processes.
- Incentivize participation in the consortium by offering resources and support for schools, researchers, and developers, such as access to funding opportunities, technical assistance, and professional development resources.
- Require the establishment of a central repository of research findings and best practices generated through rapid cycle evaluations conducted within the consortium, to facilitate the broader dissemination and adoption of effective GenAI-powered tools.
Conclusion
Persistent learning opportunity gaps in math and reading, particularly among disadvantaged students, are a systemic challenge requiring innovative solutions. GenAI-powered educational tools offer potential for personalizing learning, identifying misconceptions, and providing tailored support. However, the mismatch between the pace of GenAI innovation and lengthy traditional research pathways impedes thorough vetting of these technologies to ensure they are equitable, effective, and safe before widespread adoption.
GenAiRA and development of alternative research frameworks provide a comprehensive approach to bridge the divide between GenAI’s rapid progress and the need for thorough evaluation in education. Leveraging existing partnerships, research infrastructure, and data sources can expedite the research process while maintaining scientific rigor and prioritizing student well-being.
The plan of action creates a roadmap for responsibly harnessing GenAI’s potential in education. Identifying appropriate congressional mechanisms for establishing the accelerator program, such as creating a new bill or incorporating language into upcoming legislation, can ensure this critical initiative receives necessary funding and oversight.
This comprehensive strategy charts a path toward equitable, personalized learning facilitated by GenAI while upholding the highest standards of evidence. Aligning GenAI innovation with rigorous research and prioritizing the needs of underserved student populations can unlock the transformative potential of these technologies to address persistent achievement gaps and improve outcomes for all learners.
This idea is part of our AI Legislation Policy Sprint. To see all of the policy ideas spanning innovation, education, healthcare, and trust, safety, and privacy, head to our sprint landing page.
Implementing AI and GenAI-powered educational tools without sufficient evidence of their effectiveness or safety could lead to the widespread use of ineffective interventions. If these tools fail to improve student outcomes or even hinder learning progress, they can have long-lasting negative consequences for students’ academic attainment and self-perception as learners.
When students are exposed to ineffective educational tools, they may struggle to grasp key concepts, leading to gaps in their knowledge and skills. Over time, these gaps can compound, leaving students ill-prepared for future learning challenges and limiting their academic and career opportunities. Moreover, repeated experiences of frustration and failure with educational technologies can erode students’ confidence, motivation, and engagement with learning.
This erosion of learner identity can be particularly damaging for students from disadvantaged backgrounds, who may already face additional barriers to academic success. If AI-powered tools fail to provide effective support and personalization, these students may fall even further behind their peers, exacerbating existing educational inequities.
A Safe Harbor for AI Researchers: Promoting Safety and Trustworthiness Through Good-Faith Research
Artificial intelligence (AI) companies disincentivize safety research by implicitly threatening to ban independent researchers that demonstrate safety flaws in their systems. While Congress encourages companies to provide bug bounties and protections for security research, this is not yet the case for AI safety research. Without independent research, we do not know if the AI systems that are being deployed today are safe or if they pose widespread risks that have yet to be discovered, including risks to U.S. national security. While companies conduct adversarial testing in advance of deploying generative AI models, they fail to adequately test their models after they are deployed as part of an evolving product or service. Therefore, Congress should promote the safety and trustworthiness of AI systems by establishing bug bounties for AI safety via the Chief Digital and Artificial Intelligence Office and creating a safe harbor for research on generative AI platforms as part of the Platform Accountability and Transparency Act.
Challenge and Opportunity
In July 2023, the world’s top AI companies signed voluntary commitments at the White House, pledging to “incent third-party discovery and reporting of issues and vulnerabilities.” Almost a year later, few of the signatories have lived up to this commitment. While some companies do reward researchers for finding security flaws in their AI systems, few companies strongly encourage research on safety or provide concrete protections for good-faith research practices. Instead, leading generative AI companies’ Terms of Service legally prohibit safety and trustworthiness research, in effect threatening anyone who conducts such research with bans from their platforms or even legal action.
In March 2024, over 350 leading AI researchers and advocates signed an open letter calling for “a safe harbor for independent AI evaluation.” The researchers noted that generative AI companies offer no legal protections for independent safety researchers, even though this research is critical to identifying safety issues in AI models and systems. The letter stated: “whereas security research on traditional software has established voluntary protections from companies (‘safe harbors’), clear norms from vulnerability disclosure policies, and legal protections from the DOJ, trustworthiness and safety research on AI systems has few such protections.”
In the months since the letter was released, companies have continued to be opaque about key aspects of their most powerful AI systems, such as the data used to build their models. If a researcher wants to test whether AI systems like ChatGPT, Claude, or Gemini can be jailbroken such that they pose a threat to U.S. national security, they are not allowed to do so as companies proscribe such research. Developers of generative AI models tout the safety of their systems based on internal red-teaming, but there is no way for the federal government or independent researchers to validate these results, as companies do not release reproducible evaluations.
Generative AI companies also impose barriers on their platforms that limit good-faith research. Unlike much of the web, the content on generative AI platforms is not publicly available, meaning that users need accounts to access AI-generated content and these accounts can be restricted by the company that owns the platform. In addition, companies like Google, Amazon, Microsoft, and OpenAI block certain requests that users might make of their AI models and limit the functionality of their models to prevent researchers from unearthing issues related to safety or trustworthiness.
Similar issues plague social media, as companies take steps to prevent researchers and journalists from conducting investigations on their platforms. Social media researchers face liability under the Computer Fraud and Abuse Act and Section 1201 of the Digital Millennium Copyright Act among other laws, which has had a chilling effect on such research and worsened the spread of misinformation online. The stakes are even higher for AI, which has the potential not only to turbocharge misinformation but also to provide U.S. adversaries like China and Russia with material strategic advantages. While legislation like the Platform Accountability and Transparency Act would enable research on recommendation algorithms, proposals that grant researchers access to platform data do not consider generative AI platforms to be in scope.
Congress can safeguard U.S. national security by promoting independent AI safety research. Conducting pre-deployment risk assessments is insufficient in a world where tens of millions of Americans are using generative AI—we need real-time assessments of the risks posed by AI systems after they are deployed as well. Big Tech should not be taken at its word when it says that its AI systems cannot be used by malicious actors to generate malware or spy on Americans. The best way to ensure the safety of generative AI systems is to empower the thousands of cutting-edge researchers at U.S. universities who are eager to stress test these systems. Especially for general-purpose technologies, small corporate safety teams are not sufficient to evaluate the full range of potential risks, whereas the independent research community can do so thoroughly.
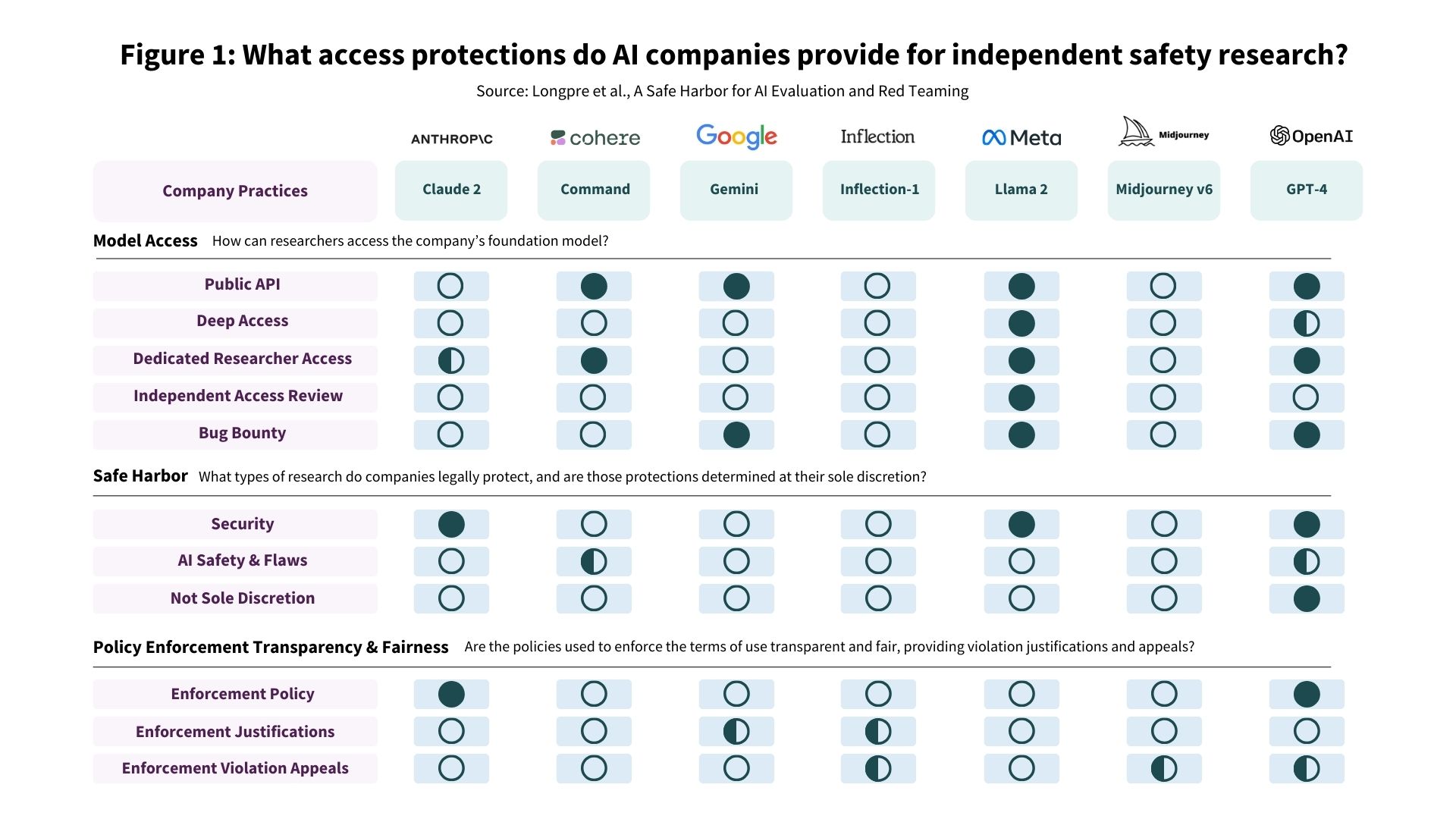
Figure 1. What access protections do AI companies provide for independent safety research? Source: Longpre et al., “A Safe Harbor for AI Evaluation and Red Teaming.”
Plan of Action
Congress should enable independent AI safety and trustworthiness researchers by adopting two new policies. First, Congress should incentivize AI safety research by creating algorithmic bug bounties for this kind of work. AI companies often do not incentivize research that could reveal safety flaws in their systems, even though the government will be a major client for these systems. Even small incentives can go a long way, as there are thousands of AI researchers capable of demonstrating such flaws. This would also entail establishing mechanisms through which safety flaws or vulnerabilities in AI models can be disclosed, or a kind of help-line for AI systems.
Second, Congress should require AI platform companies, such as Google, Amazon, Microsoft, and OpenAI to share data with researchers regarding their AI systems. As with social media platforms, generative AI platforms mediate the behavior of millions of people through the algorithms they produce and the decisions they enable. Companies that operate application programming interfaces used by tens of thousands of enterprises should share basic information about their platforms with researchers to facilitate external oversight of these consequential technologies.
Taken together, vulnerability disclosure incentivized through algorithmic bug bounties and protections for researchers enabled by safe harbors would substantially improve the safety and trustworthiness of generative AI systems. Congress should prioritize mitigating the risks of generative AI systems and protecting the researchers who expose them.
Recommendation 1. Establish algorithmic bug bounties for AI safety.
As part of the FY2024 National Defense Authorization Act (NDAA), Congress established “Artificial Intelligence Bug Bounty Programs” requiring that within 180 days “the Chief Digital and Artificial Intelligence Officer of the Department of Defense shall develop a bug bounty program for foundational artificial intelligence models being integrated into the missions and operations of the Department of Defense.” However, these bug bounties extend only to security vulnerabilities. In the FY2025 NDAA, this bug bounty program should be expanded to include AI safety. See below for draft legislative language to this effect.
Recommendation 2. Create legal protections for AI researchers.
Section 9 of the proposed Platform Accountability and Transparency Act (PATA) would establish a “safe harbor for research on social media platforms.” This likely excludes major generative AI platforms such as Google Cloud, Amazon Web Services, Microsoft Azure, and OpenAI’s API, meaning that researchers have no legal protections when conducting safety research on generative AI models via these platforms. PATA and other legislative proposals related to AI should incorporate a safe harbor for research on generative AI platforms.
Conclusion
The need for independent AI evaluation has garnered significant support from academics, journalists, and civil society. Safe harbor for AI safety and trustworthiness researchers is a minimum fundamental protection against the risks posed by generative AI systems, including related to national security. Congress has an important opportunity to act before it’s too late.
This idea is part of our AI Legislation Policy Sprint. To see all of the policy ideas spanning innovation, education, healthcare, and trust, safety, and privacy, head to our sprint landing page.
The authors of this memorandum as well as the academic paper underlying it submitted a comment to the Copyright Office in support of an exemption to DMCA for AI safety and trustworthiness research. The Computer Crime and Intellectual Property Section of the U.S. Department of Justice’s Criminal Division and Senator Mark Warner have also endorsed such an exemption. However, a DMCA exemption regarding research on AI bias, trustworthiness, and safety alone would not be sufficient to assuage the concerns of AI researchers, as they may still face liability under other statutes such as the Computer Fraud and Abuse Act.
Much of this research is currently conducted by research labs with direct connections to the AI companies they are assessing. Researchers who are less well connected, of which there are thousands, may be unwilling to take the legal or personal risk of violating companies’ Terms of Service. See our academic paper on this topic for further details on this and other questions.
See draft legislative language below, building on Sec. 1542 of the FY2024 NDAA:
SEC. X. EXPANSION OF ARTIFICIAL INTELLIGENCE BUG BOUNTY PROGRAMS.
(a) Update to Program for Foundational Artificial Intelligence Products Being Integrated Within Department of Defense.—
(1) Development required.—Not later than 180 days after the date of the enactment of this Act and subject to the availability of appropriations, the Chief Digital and Artificial Intelligence Officer of the Department of Defense shall expand its bug bounty program for foundational artificial intelligence models being integrated into the missions and operations of the Department of Defense to include unsafe model behaviors in addition to security vulnerabilities.
(2) Collaboration.—In expanding the program under paragraph (1), the Chief Digital and Artificial Intelligence Officer may collaborate with the heads of other Federal departments and agencies with expertise in cybersecurity and artificial intelligence.
(3) Implementation authorized.—The Chief Digital and Artificial Intelligence Officer may carry out the program In subsection (a).
(4) Contracts.—The Secretary of Defense shall ensure, as may be appropriate, that whenever the Secretary enters into any contract, such contract allows for participation in the bug bounty program under paragraph (1).
(5) Rule of construction.—Nothing in this subsection shall be construed to require—
(A) the use of any foundational artificial intelligence model; or
(B) the implementation of the program developed under paragraph (1) for the purpose of the integration of a foundational artificial intelligence model into the missions or operations of the Department of Defense.
Update COPPA 2.0 to Strengthen Children’s Online Voice Privacy in the AI Era
Emerging technologies like artificial intelligence (AI) are changing the way humans interact with machines. As AI technology has made huge progress over the last decade, the processing of modalities such as text, voice, image, and video data has been replaced with data-driven large AI models. These models were primarily aimed for machines to comprehend various data and perform tasks without human intervention. Now, with the emergence of generative AI like ChatGPT, these models are capable of generating data such as text, voice, image, or video. Policymakers across the globe are struggling to draft to govern ethical use of data as well as regulate the creation of safe, secure, and trustworthy AI models.
Data privacy is a major concern with the advent of AI technology. Actions by the US Congress such as the proposed American Privacy Rights Act aim to enforce strict data privacy rights. With emerging AI applications for children, the privacy of children and the safekeeping of their personal information is also a legislative challenge.
Congress must act to protect children’s voice privacy before it’s too late. Companies that store children’s voice recordings and use them for profit-driven applications (or advertising) without parental consent pose serious privacy threats to children and families. The proposed revisions to the Children’s Online Privacy Protection Act (COPPA) aim to restrict companies’ capacity to profit from children’s data and transfer the responsibility of compliance from parents to companies. However, several measures in the proposed legislation need more clarity and additional guidelines.
Challenge and Opportunity
Human voice1 is one of the most popular modalities for AI technology. Advancements in voice AI technology such as voice AI assistants (Siri, Google, Bixby, Alexa, etc.) in smartphones have made many day-to-day activities easier; however, there are also emerging threats from voice AI and a lack of regulations governing voice data and voice AI applications. One example is AI voice impersonation scams. Using the latest voice AI technology,2 a high-quality personalized voice recording can be generated with as little as 15 seconds of the speaker’s recorded voice. A technology rat race among Big Tech has begun, as companies are trying to achieve this using voice recordings that are less than a few seconds. Scammers have increasingly been using this technology for their benefit. OpenAI, the creator of ChatGPT, recently developed a product called Voice Engine—but refrained from commercializing it by acknowledging that this technology poses “serious risks,” especially in an election year.
A voice recording contains very personal information about a speaker, and that gives the ability to identify a target speaker from recordings of multiple speakers. Emerging research in voice AI technology has potential implications for medical and health-related applications from voice recordings, plus identification of age, height, and much more. When using cloud-based applications, privacy concerns also arise during voice data transfer and from data storage leaks, due to noncompliance with data collection and storage. Therefore, the threats from misuse of voice data and voice AI technology are enormous.
Social media services, educational technology, online games, and smart toys are just a few services for children that have started adopting voice technology (e.g., Alexa for Kids). Any service operator (or company) collecting and using children’s personal information, including their voice, is bound by the Children’s Online Privacy Protection Act (COPPA). The Federal Trade Commission (FTC) is the enforcing federal agency for COPPA. However, several companies have recently violated COPPA by collecting personal information from children without parental consent and used it for advertising and maximizing their platform profits. “Amazon’s history of misleading parents, keeping children’s recordings indefinitely, and flouting parents’ deletion requests violated COPPA and sacrificed privacy for profits,” said Samuel Levine of the FTC’s Bureau of Consumer Protection. The FTC alleges that Amazon maintained records of children’s data, disregarding parents’ deletion requests, and trained its Voice AI algorithms on that data.
Children’s spoken characteristics are different from those of adults; thus, developing voice AI technology for children is more challenging. Most commercial voice-AI-enabled services work smoothly for adults, but their accuracy in understanding children’s voices is often limited. Another challenge is the relatively sparse availability of children’s voice data to train AI models. Therefore, Big Tech is looking to leverage ways to acquire as much children’s voice data as possible to train AI voice models. This challenge is prevalent not only in industry but also in academic research on the subject due to very limited data availability and varying spoken skills. However, misuse of acquired data, especially without consent, is not a solution, and operators must be penalized for such actions.
Considering the recent violations of COPPA by operators, and with a goal to strengthen the compliance of safeguarding and avoid misuse of personal information such as voice, Congress is updating COPPA with new legislation. The COPPA updates propose to extend and update the definition of “operator,” “personal information” including voice prints, “consent,” “website/service/application” including devices connected to the internet, and guidelines for “collection, use, disclosure, and deletion of personal information.” These updates are especially critical when the personal information of users (or consumers) can serve as valuable data for operators for profit-driven applications and misuse without any federal regulation. The FTC acknowledges that the current version of COPPA is insufficient; therefore, these updates would also enable the FTC to act on operators and take strict action.
Plan of Action
The Children and Teens’ Online Privacy Protection Act (COPPA 2.0) has been proposed in both the Senate and House to update COPPA for the modern internet age, with a renewed focus on limiting misuse of children’s personal data (including voice recordings). This proposed legislation has gained momentum and bipartisan support. However, the text in this legislation could still be updated to ensure consumer privacy and support future innovation.
Recommendation 1. Clarify the exclusion clause for audio files.
An exclusion clause has been added in this legislation particularly for audio files containing a child’s voice, declaring that the collected audio file is not considered personal information if it meets certain criteria. This was added to adopt a more expansive audio file exception, particularly to allow operators to provide some features to their users (or consumers).
While just having the text “only uses the voice within the audio file solely as a replacement for written words”3 might be overly restrictive for voice-based applications, the text “to perform a task” might open the use of audio files for any task that could be beneficial to operators. The task should only be related to performing a request or providing a service to the user, and that needs to be clarified in the text. Potential misuse of this text could be (1) to train AI models for tasks that might help operators provide a service to the user—especially for personalization, or (2) to extract and store “audio features”4 (most voice AI models are trained using audio features instead of the raw audio itself). Operators might argue that extracting audio features is necessary as part of the algorithm that assists in providing a service to the user. Therefore, the phrasing “to perform a task” in this exclusion might be open-ended and should be modified as suggested:
Current text: “(iii) only uses the voice within the audio file solely as a replacement for written words, to perform a task, or engage with a website, online service, online application, or mobile application, such as to perform a search or fulfill a verbal instruction or request; and”
Suggestion text: “(iii) only uses the voice within the audio file solely as a replacement for written words, to only perform a task to engage with a website, online service, online application, or mobile application, such as to perform a search or fulfill a verbal instruction or request; and”
On a similar note, legislators should consider adding the term “audio features.” Audio features are enough to train voice AI models and develop any voice-related application, even if the original audio file is deleted. Therefore, the deletion argument in the exclusion clause should be modified as suggested:
Current text: “(iv) only maintains the audio file long enough to complete the stated purpose and then immediately deletes the audio file and does not make any other use of the audio file prior to deletion.”
Suggestion text: “(iv) only maintains the audio file long enough to complete the stated purpose and then immediately deletes the audio file and any extracted audio-based features and does not make any other use of the audio file (or extracted audio-features) prior to deletion.”
Adding more clarity to the exclusion will help avoid misuse of children’s voices for any task that companies might still find beneficial and also ensure that operators delete all forms of the audio which could be used to train AI models.
Recommendation 2. Add guidelines on the deidentification of audio files to enhance innovation.
A deidentified audio file is one that cannot be used to identify the speaker whose voice is recorded in that file. The legislative text of COPPA 2.0 does not mention or have any guidelines on how to deidentify an audio file. These guidelines would not only protect the privacy of users but also allow operators to use deidentified audio files to add features and improve their products. The guidelines could include steps to be followed by operators as well as additional commitment from operators.
The steps include:
- Each audio file collected by an application should be stored with an anonymous identifier.
- Each audio file collected from the same user account (a child) or a device (e.g., smartphone, tablets/iPad, laptop/computer) should be treated as an individual file and stored with anonymous identifiers. This will avoid linking multiple audio files from the same user or device.
- If any audio file contains any personally identifiable information, that audio file must be deleted instantly, and the user (parent of the child) must be informed. There is no guarantee that an audio recording will not contain any personally identifiable information, and therefore this step is critical.
The commitments include:
- An operator should commit not to reidentify the speaker from any given audio file (both anonymized and non-anonymous) (this task is often called “speaker identification”).
- An operator should seek consent (or approval) from the parent of the user (child) to allow the use of the deidentified audio files for product development, and only then use it or else immediately delete the audio file following the completion of the task for which it was collected.
Following these guidelines might be expensive for operators; however, it is crucial to take as many precautions as possible. Current deidentification steps of audio files followed by operators are not sufficient, and there have been numerous instances in which anonymized data had been reidentified, according to a statement released by a group of State Attorneys General. These proposed guidelines could allow operators to deidentify audio files and use those files for product development. This will allow the innovation of voice AI technology for children to flourish.
Recommendation 3. Add AI-generated avatars in the definition of personal information.
With the emerging applications of generative AI and growing virtual reality use for education (in classrooms) and for leisure (in online games), “AI-based avatar generation from a child’s image, audio, or video” should be added to the legislative definition of “personal information.” Virtual reality is a growing space, and digital representations of the human user (an avatar) are increasingly used to allow the user to see and interact with virtual reality environments and other users.
Conclusion
As new applications of AI emerge, operators must ensure compliance in the collection and use of consumers’ personal information and safety in the design of their products using that data, especially when dealing with vulnerable populations like children. Since the original passage of COPPA in 1998, how consumers use online services for day-to-day activities, including educational technology and amusement for children, has changed dramatically. This ever-changing scope and reach of online services require strong legislative action to bring online privacy standards into the 21st century. Without a doubt, COPPA 2.0 will lead this regulatory drive not only to protect children’s personal information collected by online services and operators from misuse but also to ensure that the burden of compliance rests on the operators rather than on parents. These recommendations will help strengthen the protections of COPPA 2.0 even further while leaving open avenues for innovation in voice AI technology for children.
This idea is part of our AI Legislation Policy Sprint. To see all of the policy ideas spanning innovation, education, healthcare, and trust, safety, and privacy, head to our sprint landing page.
A National Training Program for AI-Ready Students
In crafting future legislation on artificial intelligence (AI), Congress should introduce a Digital Frontier and AI Readiness Act of 2025 to create educator training sites in emerging technology to ensure our students can graduate AI-ready. Computing, data, and AI basics will be critical for every student, yet our education system does not have the capacity to impart them. A national mobilization for the education workforce would ensure U.S. leadership in the global AI talent race, address mounting challenges in teacher shortages and retention, and fill critical workforce preparedness gaps not addressed by the CHIPS and Science Act. The legislation would include three components: (1) a prestigious national fellowship program for classroom educators with extended summer pay; (2) an evidence-based national network of training sites for peer-based learning; and (3) a modernization competition for teacher college programs to sustain long-term improvement in our education workforce.
Investing in effective educators has a significant impact: one high-quality teacher can significantly boost lifetime incomes, degree attainment, and other life satisfaction measures for many classrooms of students. These programs would be facilitated through the National Science Foundation (NSF), including through simplified application procedures, expanded eligibility, and evaluation approaches.
Challenge and Opportunity
If AI is positioned to dramatically transform our economy, from the production line to the c-suite, then everyone must be prepared to leverage its power. AI alone may add between an estimated $2.6 trillion to $4.4 trillion annually to the global economy and may automate between 60% to 70% of task-time within existing jobs, rather than full replacement. Earlier studies estimated that emerging technologies will increase the technology intensity of existing careers across all sectors. A report by the Burning Glass Institute found that 22% of all current open jobs in the U.S. economy include at least one “data science skill,” with the highest share of data-skill job postings in utilities, manufacturing, and agriculture. Not every worker will build the next AI algorithm or become a data scientist, but nearly every American will need to leverage data and AI to maintain a competitive edge in their sector or risk losing entire industries to other countries who do the same. This unprecedented economic growth will only be captured by the countries whose workers are prepared in data and AI basics.
U.S. educators are mostly unsupported to teach students about AI and other emerging technologies. An analysis of math educators nationally found that teachers are least confident to teach about data and statistics, as well as technology integration, compared to other content categories. Computer science was the least popular credential for K-12 educators to pursue as recently as the 2018–2019 school year. These challenges translate to student opportunities and outcomes. As of 2023, only 5.8% of our high school students are enrolled in foundational computer science courses. Introductory basics in data or AI are typically not covered even if they exist in some state standards. Nationally, students’ foundational data literacy has declined between one and three grade levels steadily over the past decade, varying disproportionately by race and geography, with losses only accelerated by the pandemic.
Moreover, our teacher workforce capacity is declining. Teacher entry, preparation, and retention rates remain at historical lows across the country and have not meaningfully recovered since the pandemic. Over the past decade, the number of individuals completing a teacher preparation program has fallen 25%, with only modest recovery since the pandemic, shortages of at least 55,000 unfilled positions this year, and long-term forecasts reaching at least 100,000 shortages annually. Factors including low pay, low prestige, and difficult environments create a perception challenge for the profession: less than 1 in 5 Americans would encourage a young person to become a teacher. These challenges compound over time, as more graduate schools of education close or cut their programming. In 2022, Harvard discontinued its Undergraduate Teacher Program completely, citing low interest and enrollment numbers, one among many.
What if the concurrent challenges of digital upskilling and teacher shortages could help solve one another? The teaching profession is facing a perception problem just as AI has made education more important than ever before. In the global information age, U.S. worker skills and talent are our greatest weapons. The expectations of teachers and teaching must change. Major U.S. economic peers, including Canada, Germany, China, India, New Zealand, and the United Kingdom, have all announced similar national efforts to make robust investments in teacher upskilling in high-value technology areas. In our new AI era, U.S. policymakers now have the opportunity to develop the infrastructure, 21st-century training, and prestigious social recognition to properly value education as an economic and national security priority. A recent report from Goldman Sachs identified “a narrow window of opportunity – what we call the inter-AI years,” in which policymaker “decisions made today will determine what is possible in the future. A generative world order will emerge.” Inaction today risks the United States falling quickly behind tomorrow.
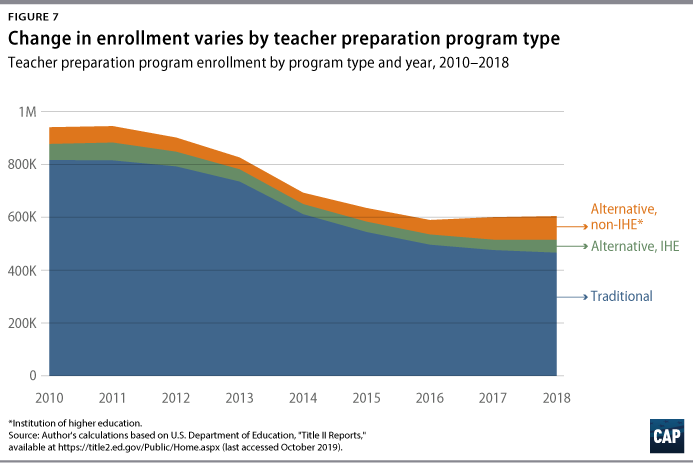
Teacher preparation program enrollment by program and year, 2010–2018 via CAP, 2019
Plan of Action
A Digital Frontier Teaching Corps (DFT Corps) would mobilize a new generation of teachers who are fluent in, adaptive to, and resilient to fast-changing technology, equipped to help our students become the same. The DFT Corps would re-norm the job of teaching to become a full-year profession, making the summer months an essential part of the job of adaptive 21st-century teaching with regular training intensives. Currently, educators only work and are paid for nine months of the year.
Upon acceptance by application, selected teachers would enter a three-year fellowship program to participate in training intensives facilitated at local institutes of higher education, nonprofits, educational service agencies, or industry partners. Scholarships facilitated through the National Science Foundation would extend educator pay and hours from nine months to a full annualized salary. DFT Corps members would also be eligible for substantial federal loan forgiveness in return for their additional time investment.
After three rotations, members would become eligible to serve as DFT Corps site leaders, responsible for program design at new or existing training sites. These opportunities would lend greater compensation, prestige, and retention through leadership opportunities, concurrently addressing systemic talent challenges in education at their root and creating an adaptive mechanism for faster upskilling. Additional program components, including licensure incentives and teacher college innovation grants, would further sustain long-term impacts. By year three of the program, 50,000 educators would be on the path to preparing our students for the future of work, 500 inaugural Corps members would become state or local site leaders to expand the mobilization, and the perception of teaching would further shift from childcare to a critical and respected national service.
To accomplish this vision, Congress should authorize the National Science Foundation to create:
1. A national Digital Frontier Teaching Corps, a three-year “talent surge” fellowship opportunity covering summertime pay for high-potential educators to conduct intensive study in AI, data science, and computing foundations. The DFT Corps would be a prestigious and materially meaningful program to both impart digital technical skills and transform the social perception of the teaching profession. The DFT Corps would include:
- Educator scholarships for full summertime pay
- A low-barrier digital application process for individuals to apply for funding directly from the NSF or an intermediary third-party organization
- Automatic enrollment in local site-based training sites, including college-level coursework (computer science, data science, artificial intelligence, quantum computing) and peer-based pedagogical training
- Tax-based verification for program completion status
- Federal loan forgiveness upon successful program completion
- Federal recognition awards of schools employing DFT Corps educators
- Eligibility to apply for DFT Corps training site leadership upon successful program completion
2. DFT Corps training sites, a national network of university-based, locally led professional development sites in collaboration with local education agencies, based on the evidence-based model of the National Writing Project. Competitive five-year grants would support the creation of Corps sites, one per state, with the opportunity for renewal. DFT Corps training sites would:
- Provide college-level coursework (computer science, data science, artificial intelligence, quantum computing) and peer-based pedagogy training to 100 educators per summer
- Be co-designed with local faculty, researchers, or industry experts, along with prior DFT Corps members
- Be evaluated on an annual basis, with criteria determined by the NSF, with all evaluation results published publicly and submitted to the NSF
- Expand with matching funds from state, industry, or philanthropic support as resources allow
3. Teacher College Innovation Grants, a competitive NSF grant program for modernizing teacher preparation programs and teacher licensure models. Teacher College Innovation Grants would provide research funding and capacity to evaluate DFT Corps training sites and ensure lessons learned are quickly integrated back into teacher preparation programs. Competitive priorities would be made for:
- Proposals that develop and/or scale new methods-style courses for teaching digital technology (i.e., computational thinking, data literacy, AI literacy) to students across traditional school subjects
- Proposals that develop new models for teacher preparation, leveraging online learning or other digital training systems that result in lower program costs
- Proposals that focus on building or modernizing teacher preparation capacity in majority-rural or majority-Indigenous communities
The DFT Corps program is intended to be catalytic. Should the program find success in early scaling, state and local funding could support further adoption of the model over time, so that teaching transforms to an annualized profession across subject areas and grade-levels.
Conclusion
In the new era of AI, education is a national security issue. Advancing our population’s ability to effectively deploy AI and other emerging technology will uniquely determine U.S. leadership and economic competitiveness in the coming years and decades. Education investments made by states within the next few years will all but determine local long-term economic trajectories.
In the 1950s and 1960s, education and competitiveness were one and the same. One year after the Soviets launched Sputnik, Congress took action and passed the National Defense Education Act, a $1 billion spending package to advance teaching and learning in science, mathematics, and foreign languages. At one time, we respected teachers as critical to the national mission, leading the charge to prepare our next generation to lead, and we took swift action to support their mission. We must take the same bold action now.
This idea is part of our AI Legislation Policy Sprint. To see all of the policy ideas spanning innovation, education, healthcare, and trust, safety, and privacy, head to our sprint landing page.
The scale of this national challenge requires meaningful appropriations to raise teacher pay, ensure high-quality training opportunities with sufficient expertise, and sustain a long-term strategy to address deeply-rooted sector challenges. A short-term, one-shot approach will simply waste money and generate minimal impact.
Moreover, the program’s creation necessitates a significant simplification of National Science Foundation application processes to reduce grant application length, burden, and paperwork. It also creates a targeted exception for the NSF to support broader nonresearch activities that are otherwise sector-critical for national scientific and educational endeavors. If enacted, this legislation could help reduce overhead for program administration and redirect more resources to supporting quality state and local implementation vs. program compliance.
Once scaled to all 50 states, the recurring annual costs of the proposed legislation would be $250 million:
- $150 million for DFT Corps member scholarships (5,000 teachers per year)
- $50 million for DFT Corps training sites (one site per state at $1 million each)
- $50 million for Teacher College Innovation Grants (one site per state at $1 million each)
In the first five years, the cost would slowly increase to the total amount, starting at a base $25 million for five states ($15 million for 500 scholarships, $5 million for training sites, $5 million for Innovation grants).
Creating an AI-ready workforce is a critical national priority to maintain U.S. economic competitiveness, mitigate risk of AI primacy, and ensure our citizenry can successfully navigate a complex technology landscape they will graduate into. McKinsey projects that successful integration of AI across more than 63 business use cases would add between $13.6 trillion and $22.1 trillion to the global economy. A recent National Institutes of Health analysis suggests that, for any country to successfully specialize in AI, there must be general preexisting technological capabilities and a strong scientific knowledge base. AI-readiness must be a population-wide goal. Given that 60% of Americans do not complete a bachelor’s degree, AI readiness must begin early in K-12 education and in community colleges.
Estimated return on investment: $250 million represents less than 1.5% of the Every Student Succeeds Act’s last annual appropriation level in 2020, the nation’s primary national education funding mechanism. If this legislation increases the share of economic growth forecasted by effectively harnessing AI by only five percent, we would conservatively add $171 billion to the U.S. economy each year.
The majority of educator training programs are too short, only given during the busy school year, and do not have the opportunity to improve over multiple years within a given school. Early iterations of the National Writing Project, on which this program is based, determined that “although schools may see results from C3WP in a single school year, a longer-term investment may produce a greater impact.” Even if sustained during a school year, researchers have found that “absent a surrounding context that is highly supportive of teacher learning and change, 1 year of PD cannot sufficiently alter instructional practices enough to impact student outcomes.” While earlier evaluation studies saw no impact on student achievement, the National Writing Project is now one of the most lauded and effective educator training models trialed in the United States, made possible by a long-term and consistent investment in professional learning.
A three-year program will allow educators to advance from novice (year 1) to intermediate (year 2) to mentor or facilitator (year 3).By year 4, graduating educators would be prepared to serve as site leaders, dramatically increasing the available talent pool for sustaining and growing DFT Corps sites nationally. Additional time will also enable a local site to improve its own programming and align tightly with multi-year school and district planning.
The DFT Corps is an accelerated investment in the creation of locally led professional development sites, uniquely designed with (1) direct support for current classroom educators to participate; (2) a replicated network model for summer-based, in-service training; and (3) innovation grants to research aligned training improvements and best practices. No current federal program does all three at once for current classroom educators.
Existing teacher training grant programs, such as the Teacher Quality Partnerships or Supporting Effective Educator Development carry strong evidence requirements or incompatible competitive preferences. Given AI is new and little research exists on effective teaching practices, these requirements serve to significantly limit proposals on emerging topics. Grants also vary widely by institution.
Existing educator scholarship programs, such as the Robert Noyce Teacher Scholarship Program, focus mostly on recruitment of new teachers, and only provide small support for existing teachers pursuing or having previously obtained a master’s degree. 40% of U.S. teachers do not have a master’s degree. A targeted national focus on AI readiness would also require several higher-education institutions across states to organically propose training programs to the Noyce program at the same time, with the same model.
AI technology development is moving faster than the education sector can respond. In order to accelerate site creation, reduce application burden, and modernize grant distribution, the DFT Corps program would direct the NSF to:
- Allow nonresearch activities to be funded under the program, including educator salary support
- Remove and centralize all program evaluation requirements away from individual grantees, reallocating evaluation activities to external researchers across sites
- Centrally manage disbursement of DFT salary supplements, potentially via tax credits
- Modernize required data management plan requirements for present-day technology
- Limit total grant application length to 10 pages or less. In other fields, NSF grant applications take investigators over 171 hours to prepare, despite little relation between time invested and actual funding outcomes in some cases. Another study found that 42% of investigators time is spent on administrative and reporting tasks to support the execution of an NSF grant.
Yes, with appropriations. Under new 2023 guidance, the Robert Noyce Teacher Scholarship Program has expanded salary supplement options and enabled two-summer support. An executive action version of this proposal would expand the Robert Noyce Teacher Scholarship program via (1) increasing support for Track 3 with lower degree requirements (i.e. Bachelors instead of Masters); (2) stipulating a competitive priority for AI readiness and emerging technology education (defined as: computer science, computational thinking, data science, artificial intelligence literacy across the curriculum); and (3) direct the White House Office of Science & Technology Policy to launch a multi-agency, public-facing communications and recruitment effort for the DFT Corps program, in collaboration with the 50 largest teacher colleges and other participating Noyce program institutions.
The proposed DFT Corps mirrors a long-running evidence-based model, the National Writing Project (NWP), which has trained over 95,000 teachers in high-quality writing instruction across 2,000 school districts since 1974. Three independent evaluation studies over multiple years across 20 states found “positive and statistically significant effects on student achievement” across all measured components of writing. The evidence base supporting NWP is “unusually robust” for education research, employing randomized-controlled trials and meeting ESSA Tier 1 evidence criteria. A recent replication study in 2023 focusing on rural schools found positive results on “on all attributes measured,” a similar priority for the proposed DFT Corps program.
Similar to the Robert Noyce Scholarship program, the DFT Corps program would waive tuition costs and provide scholarship funds in exchange for a multi-year teaching commitment. Each year’s participation in the program would extend an educator’s teaching commitment by two additional years. A 2013 evaluation of the Noyce program found this model worked, with longer retention rates compared to new teachers graduating from the same institutions.
Recodes a nine-month profession to annual pay, and annual expectations: A primary change advanced by the DFT Corps is converting the typical teacher job from a nine-month term to an annual salary, similar to lawyers, doctors, and other high-prestige professions. In a recent RAND report on why teachers wanted to leave the profession, salary was the #2 reason, hours worked outside the school day the #3, and total hours worked was the #4. Teachers are promised a flexible and part-year job on paper, when the reality is very different. Nine-month pay challenges are so extreme that several U.S. banks host articles on “surviving the summer paycheck gap.” Many teachers take second (non-academic) jobs. And the popular #NoSummersOff hashtag gained a significant following amongst educators pre-pandemic. Concurrently, the rate of technology and curriculum changes demand more professional learning time than is typically given by schools and districts. Summer professional learning is often optional and highly variable across states. Our expectations are far too low for one of our most critical knowledge jobs. DFT Corps members would be paid during the summer for intensive study to update curriculum, plan content, and incorporate new education research on how students learn. Full-time summer work would remove pressure for administrators to “squeeze in” short, one-day professional development sessions during the school year, which study after study has demonstrated are a waste of time and money. Many current classroom educators to the former U.S. Secretary of Education continue to question these existing PD approaches.
Creates a leadership ladder: Leadership opportunities for classroom educators are few and far between. Teaching is often described as a “flat” profession, and nearly half of educators leaving the field point to a perceived lack of leadership or decision-making opportunities as contributing factors. Concurrently, new teachers who have the opportunity to collaborate with teacher-leaders within their own school generate stronger academic gains for their students. The DFT Corps would create state-wide leadership opportunities at Corps summer sites that do not disrupt school-year teaching, allowing educators to remain in the classroom during the other nine months of the year but still access visible leadership and mentor roles during the summer.
Leverages peer-based learning: Beyond the opportunity to positively impact students and student learning, 63% of educators report that strong relationships with other teachers are a top reason for staying in the classroom. The DFT Corps would leverage peer-based professional development over multiple years, reallocating the summer months to joint study and creating stronger educator networks statewide. One of the DFT Corp’s precedent peer-based models, the National Writing Project, “has a legacy as being the best professional development model for K-12 teachers” precisely due to a targeted focus on peer exchange. In post-training interviews, researchers found that educators “immediately changed several of their teaching practices and felt a renewed sense of enthusiasm towards the teaching of writing after participating in the NWP… a renewed sense of authority that quickly transferred to agency, these teachers possessed the self-efficacy to share what they knew and had learned with other teachers, administrators, district leaders, fellow graduate students, and most importantly, the students who would enter their classrooms in the fall.”
Builds needed prestige for the profession: The DFT Corps program forwards a reinvigorated national prioritization of the education field. In the information economy, educators are one of our most critical professions, and a greater determinant of gross domestic product than any individual semiconductor or algorithm. Under a DFT Corps communications rollout, teaching would be separated from any prior stereotypes of “caretakers,” positioned instead as essential to the economic, technology, and security fabric that advance societal progress. Research consistently suggests that low prestige of the profession pushes high-achievers away from teaching, is closely correlated with both falling preparation and retention, and may even directly affect student achievement. In China, where educators have long enjoyed high prestige for their profession, researchers found that an expansion of the country’s Free Teacher Education program helped to increase application competitiveness, extend retention rates, and enhance self-identity for program participants in a pre-publication evaluation study. In a 2018 “Global Teacher Status Index,” China was the only country to score 100 while the United States scored under 40 points. The United States is falling behind in our education culture, and we have little time to make up for lost ground.
The long-term vision for this proposal also extends beyond the NSF AI Education Act and suggests a new mechanism for federal education support in the Every Student Succeeds Act.
National Security AI Entrepreneur Visa: Creating a New Pathway for Elite Dual-Use Technology Founders to Build in America
NVIDIA, Anthropic, OpenAI, HuggingFace, and scores of other American startups helping cement America’s leadership in the race for artificial intelligence (AI) dominance all have one thing in common: they have at least one immigrant co-founder. In fact, in 2023, the National Foundation for American Policy released a policy analysis on the role of immigrants in the top American AI companies. According to their research, 65% of the companies appearing on the Forbes AI 50 list were founded or co-founded by at least one immigrant. Immigrant entrepreneurs are critical to America’s economic success, and as the private sector takes an increasing role in developing critical dual-use technologies like AI, they will be critical to America’s defense.
According to a Brookings Foundation report, “China sees talent as central to its technological advancement; President Xi Jinping has repeatedly called talent ‘the first resource’ in China’s push for ‘independent innovation.’” It’s easy to understand why the CCP sees talent as critical in its efforts to dominate key dual-use technologies relevant to national and economic security – in today’s knowledge economy, those who can innovate faster win. A company like SpaceX, which almost single-handedly reinvigorated America’s spacefaring economy, would likely not exist without Elon Musk. The lists of companies and dual-use technologies critical to American national and economic security that are unlikely to have been created successfully without the right personalities behind them are innumerable. America needs these entrepreneurs more than ever as competition with China for global leadership in key fields like AI heats up.
Given increased competition for talent – from allies like the United Kingdom to competitors and adversaries like China – in critical technology areas like AI, Congress must act to support high-skilled entrepreneurs by creating a National Security Startup Visa specifically targeted at founders of AI firms whose technology is inherently dual-use and critical for America’s economic leadership and national security. To maximize the potential economic benefits of such a visa for all Americans, it can be narrowly tailored, focusing only on entrepreneurs who (1) have raised significant capital from accredited American investors and venture capitalists (VCs), (2) are willing to physically reside and start their business in an Opportunity Zone, and (3) will hire at least five Americans within the first year of operation. Immigration may be a complex issue, but there is no doubt that immigrant founders are the not-so-secret ingredient that have helped to fuel America’s rise as a tech superpower. Developing a narrowly scoped visa targeted at a critical technology segment means that America can ensure its continued dominance in AI, a technology that the CEO of Google has said may be as profound as fire or electricity.
Challenge and Opportunity
While the United States has long been the preferred destination for immigrant entrepreneurs, America has never had more competition for global talent. Countries like Canada, Germany, and Estonia have created visas to attract entrepreneurs, and they appear to be working. After the introduction of a Canadian startup visa in 2013, the program increased the likelihood of previously U.S.-based immigrants creating a startup in Canada by 69%. These are immigrants who were already in America to study or work, and it should have been an obvious choice for them to stay and build their company in the United States. This means that the United States is losing out to hundreds of new companies and likely thousands of high-paying jobs that would come along with them. The fact that Canada, thanks to a streamlined immigration process for founders, was able to attract so many who were already in the United States should serve as a serious warning as to how the competition for talent is heating up.
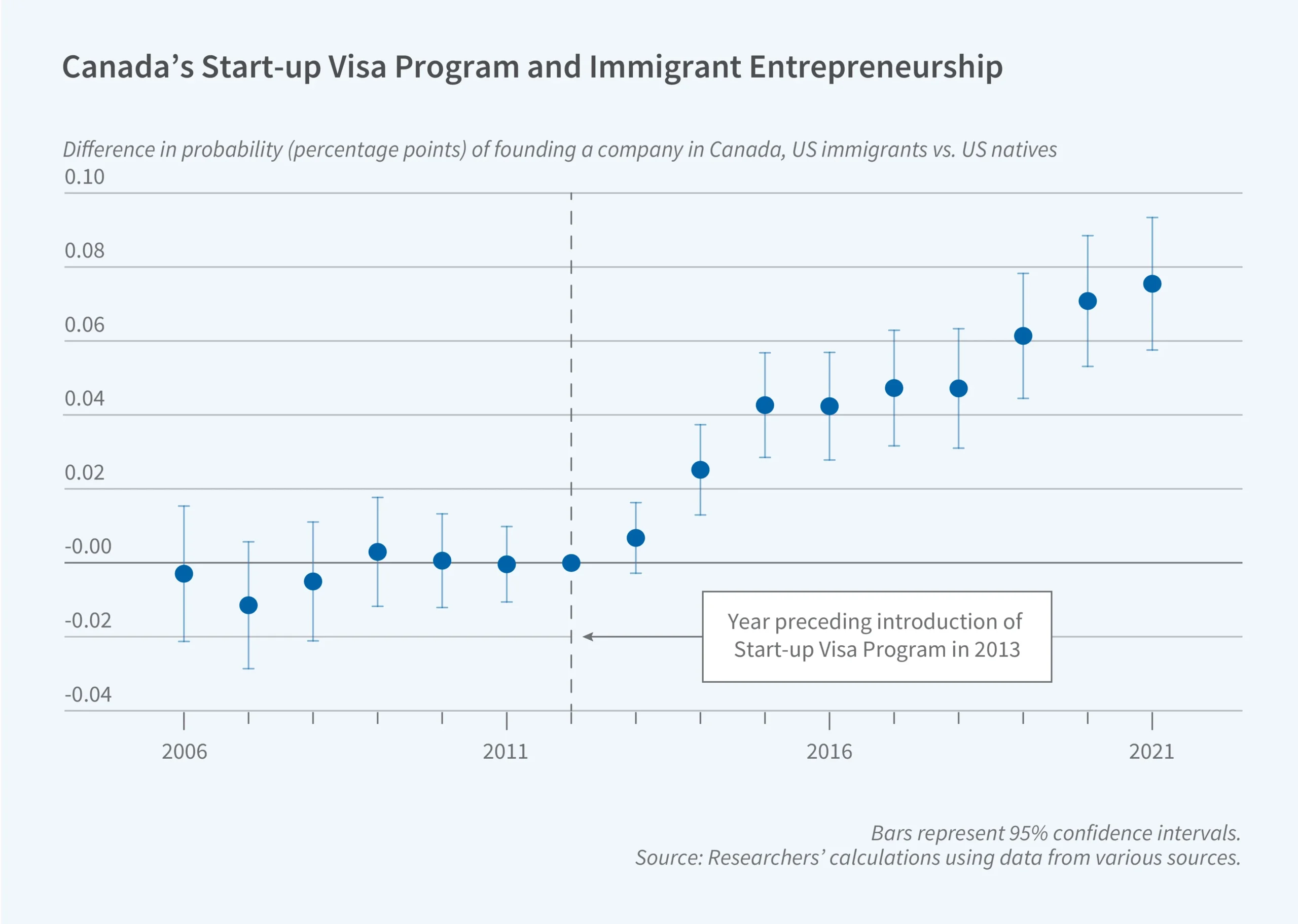
Canada demonstrates how a start-up visa enhances immigrant entrepreneurship via National Bureau of Economic Research
Historically, the United States—and Silicon Valley in particular—was the undisputed leader for venture capital fundraising and the place to start a potential unicorn (a company valued at over $1 billion). However, America’s dominance has shrunk, and VC dollars along with unicorns are increasingly found across the world in tech hotspots from China to India to the United Kingdom, showing it is increasingly easy for entrepreneurs to build a successful startup elsewhere. This is critical, because when America was the only place to build a leading company, entrepreneurs had little choice but to wade through the labyrinth that is the American immigration system. Now, top talent have many choices, and the United States must compete to become not just the premier destination to build a company and raise capital but one that is accessible to startup founders who can’t afford high-priced immigration lawyers or to wait for years until their visa is granted.
While America’s largest geopolitical competitor may suffer from extreme difficulties in attracting foreign entrepreneurs to its shores, China has a massive population advantage. This can be seen directly in the STEM space and AI in particular. According to a CSIS report, “By 2025, Chinese universities are projected to produce more than 77,000 STEM PhD graduates per year, more than double the 2010 level of about 34,000 STEM PhD graduates. In comparison, the United States is projected to graduate only approximately 40,000 STEM PhD students in 2025, a figure that includes over 16,000 international students.”
China has already outpaced the United States in the number of AI-related research articles published, and its domestic tech champions are global leaders in AI-enabled technology like facial recognition. Given the strong domestic showing in AI from Chinese researchers and entrepreneurs, with local AI startups raising billions of dollars in 2023 despite a slowdown in VC funding in China, China presents a strategic threat to America’s leadership in the AI space. America is on the cusp of losing its leadership in AI to China, but this policy creates clear opportunities to expeditiously regain lost ground by bringing in AI entrepreneurs who have already raised venture funding and are able to immediately hire American workers.
However daunting the challenge China presents, America has long had a superpower: attracting the best and brightest to our shores to build innovative global businesses. And while many leading American AI startups have an immigrant co-founder, for every entrepreneur coming to the United States today, many more are turned away or dissuaded from applying. Take Erdal Arikan, a Turkish MIT and CalTech graduate who had difficulty staying in America to continue his research and returned to Turkey. According to Graham Allison and Eric Schmidt, “It turned out that Arikan’s insight was the breakthrough needed to leap from 4G telecommunications networks to much faster 5G mobile internet services. Four years later, China’s national telecommunications champion, Huawei, was using Arikan’s discovery to invent some of the first 5G technologies. Today, Huawei holds over two-thirds of the patents related to Arikan’s solution… Had the United States been able to retain Arikan—simply by allowing him to stay in the country instead of making his visa contingent on immediately finding a sponsor for his work—this history might well have been different.”
By creating a narrowly tailored AI National Security Entrepreneur Visa, the United States has a unique opportunity to recruit founders in a field deemed “critical and emerging” by the White House and help the nation maintain both its economic and national security competitiveness. And while many are concerned about the potential economic dislocation from AI, one way to mitigate such a risk is by helping entrepreneurship flourish in the United States, especially in underserved communities like those found in Opportunity Zones across every state. With hundreds or thousands of new businesses creating high-paid jobs in rural and underserved communities, Americans outside existing tech hubs of New York City and San Francisco could finally see real economic benefits of the tech boom.
The economic potential for such a visa is tremendous. According to a 2024 report from the Center for Growth and Opportunity at Utah State University, a startup visa could have a significant impact: “Data collected at the state level suggests that when the population’s share of immigrant college graduates increases by 1 percent, patents per capita increase by 9 to 18 percent” with the report going on to say that (depending on the number of entrepreneurs brought in) “Census and industrial data predict an increase of 500,000 to 1.6 million new jobs from young start-up visa companies in the United States after 10 years of operation.”
The time for an AI startup visa is now. It will help create American jobs and revitalize local economies, cement American global leadership, and ensure that we beat China in the AI race.
Plan of Action
Create a 10-year pilot AI Entrepreneur Visa program for a select group of countries to demonstrate the potential efficacy of the visa.
The AI National Security Entrepreneur Visa will be narrowly tailored to founders from friendly nations, who have already raised significant capital for their companies from accredited American investors and are willing to physically reside in an Opportunity Zone. This will minimize risks of visa overstays and espionage while maximizing the potential economic benefits by bringing companies that have capital ready to deploy to the United States.
Visa Characteristics
- Initially available for nationals of Israel, the European Union, Japan, South Korea, Australia, New Zealand, United Kingdom, Canada, Ukraine, Armenia, Colombia, India, and Nigeria based on these country’s close ties to the U.S. and/or high rates of entrepreneurship.
- A two-year visa with an opportunity for a single three-year extension, plus a pathway to citizenship after the third year of residence in the United States.
- Also applies to immediate family members only (children and spouse).
- Allows for physical residency within any designated Opportunity Zone for the visa holder and, if applicable, their spouse and children.
- Rapid issuance by default with a maximum timeline for issuing a visa of 30 days from the date of a completed application being submitted.
- Allows for multiple foreign cofounders of a company to submit an application for a visa.
Initial Visa Application Requirements
- Must demonstrate that the company has raised at least $500,000 from an accredited American venture investor or firm.
- Have a startup focused on developing an AI or AI-enabled solution.
- To be considered eligible, each applicant must own at least 20% of the startup.
- Applicants must have demonstrated AI-related technology skills including software engineering, AI research, applied AI research, or tech product management OR have a bachelor’s or graduate degree in software engineering, physics, or mathematics from an accredited college or university.
Visa Extension Requirements
- The applicant must demonstrate that the visa holder’s company has created a minimum of five jobs employing American citizens within the first year of operation.
- The applicant must demonstrate that the company has grown revenues by at least 10% month over month on average during the period in which the visa was held OR have raised at least an additional $1.5 million from accredited American investors.
- The applicant must own 10% or more of the company at time of application for the visa extension.
Recommended Timeline
- Congress should mandate that the U.S. Citizenship and Immigration Services (USCIS) begin accepting applications for the pilot program within 180 days of the bill’s passage.
- Congress may consider adding a sunset provision 10 years after the program formally begins accepting applications in order to assess the program’s efficacy and determine whether it should be continued as is, scaled up, scaled down, or deprecated.
Miscellaneous Recommendations
- Consider creating a volunteer board of current and former American investors and entrepreneurs who can provide training and recommendations to the Department of Homeland Security/USCIS while guidelines and rules are being created for the new program.
- Example: An immigration officer may have difficulty understanding how prestigious an accelerator program such as Y Combinator or StartX is, leading them to downgrade an applicant who actually has a high likelihood of building a competitive startup.
- Require an independent economic impact assessment to be conducted every three years to understand the current and projected future impact of the program over time.
- Make the program fee-based, so that applicant fees pay for USCIS’s operating costs and no additional costs are passed on to taxpayers.
- Consider creating a cap of under $10,000 for total federal fees to make it accessible to early-stage startups.
- Ensure that it is possible for existing visa holders (student, work) to easily switch to the AI Entrepreneur Visa assuming they meet the relevant criteria.
- Consider allowing governors to opt in to the program, so that only states that would like to participate in the program do so.
Conclusion
America is in a race for global talent, especially when it comes to AI. The data shows that the majority of leading AI companies in America were created with at least one immigrant founder—but our immigration system makes it incredibly difficult for experts to come and build their companies in America, a serious strategic disadvantage compared to China, which produces dramatically more STEM graduates. By creating an AI National Security Entrepreneur Visa targeting high-skill founders who have already raised funds, Congress can quickly close the gap with China, bringing the best and brightest from around the world to America to build their companies. Not only will this help create jobs across the United States, it will make America the undisputed superpower in AI, allowing us to set standards and control the development of a technology whose impact may surpass those of all other innovations in recent decades.
This idea is part of our AI Legislation Policy Sprint. To see all of the policy ideas spanning innovation, education, healthcare, and trust, safety, and privacy, head to our sprint landing page.
Yes. Take it from the founder of Yahoo and naturalized American citizen, Jerry Yang, who said “If I had to worry about a visa, maybe Yahoo wouldn’t have gotten started,” and that “There are more places around the world where entrepreneurship has taken off… so founders have more choices. And to the extent that our immigration policies are not so welcoming, people don’t want to come.”
Created under President Trump’s Tax Cuts and Jobs Act, Opportunity Zones are designated areas across all 50 states deemed economically distressed by the Internal Revenue Service. Many previous technology booms have created outsized benefits to existing wealthy tech hubs like San Francisco and New York City thanks to positive agglomeration and network effects. By pushing entrepreneurs to found their business in a Opportunity Zone, which by its nature is an economically distressed area, the visa will help bring new jobs and opportunities to areas that previously had a difficult time attracting tech entrepreneurs and high-growth startups.
The Economic Innovation Group has written extensively about the concept of a “heartland visa,” which would allow counties to decide on specific new immigration pathways based on their distinct needs. The AI Entrepreneur Visa could be structured similarly, with states or localities opting in to the program and deciding the number and type of AI entrepreneurs they would like to bring to their communities.
Yes. Some options to further narrow the visa:
- Decrease the number of countries eligible for the pilot visa program.
- Create a cap for the number of potential founders per year (recommended minimum of 10,000 to create a sample size large enough for an economic impact assessment).
- Create a mandatory sunset for the program, requiring it to be renewed after five or 10 years.
- Increase equity ownership requirements or implement a maximum number of applicants per company.
- Allow individual states or counties to opt in to the program rather than it being available for the entire nation’s Opportunity Zones at the start.
Yes. Some options to further expand the visa:
- Increase the number of countries eligible to apply for the visa.
- Expand the technologies/industries eligible for the visa.
- Decrease or eliminate the threshold for the amount of funds raised to be eligible.
- Decrease or eliminate equity ownership requirements.
- The company’s primary physical place of business must be shown to be within an Opportunity Zone.
Improving Health Equity Through AI
Clinical decision support (CDS) artificial intelligence (AI) refers to systems and tools that utilize AI to assist healthcare professionals in making more informed clinical decisions. These systems can alert clinicians to potential drug interactions, suggest preventive measures, and recommend diagnostic tests based on patient data. Inequities in CDS AI pose a significant challenge to healthcare systems and individuals, potentially exacerbating health disparities and perpetuating an already inequitable healthcare system. However, efforts to establish equitable AI in healthcare are gaining momentum, with support from various governmental agencies and organizations. These efforts include substantial investments, regulatory initiatives, and proposed revisions to existing laws to ensure fairness, transparency, and inclusivity in AI development and deployment.
Policymakers have a critical opportunity to enact change through legislation, implementing standards in AI governance, auditing, and regulation. We need regulatory frameworks, investment in AI accessibility, incentives for data collection and collaboration, and regulations for auditing and governance of AI systems used in CDS systems/tools. By addressing these challenges and implementing proactive measures, policymakers can harness AI’s potential to enhance healthcare delivery and reduce disparities, ultimately promoting equitable access to quality care for everyone.
Challenge and Opportunity
AI has the potential to revolutionize healthcare, but its misuse and unequal access can lead to unintended dire consequences. For instance, algorithms may inadvertently favor certain demographic groups, allocating resources disproportionately and deepening disparities. Efforts to establish equitable AI in healthcare have seen significant momentum and support from various governmental agencies and organizations, specifically regarding medical devices. The White House recently announced substantial investments, including $140 million for the National Science Foundation (NSF) to establish institutes dedicated to assessing existing generative AI (GenAI) systems. While not specific to healthcare, President Biden’s blueprint for an “AI Bill of Rights” outlines principles to guide AI design, use, and deployment, aiming to protect individuals from its potential harms. The Food and Drug Administration (FDA) has also taken steps by releasing a beta version of its regulatory framework for medical device AI used in healthcare. The Department of Health and Human Services (DHHS) has proposed revisions to Section 1557 of the Patient Protection and Affordable Care Act, which would explicitly prohibit discrimination in the use of clinical algorithms to support decision-making in covered entities.
How Inequities in CDS AI Hurt Healthcare Delivery
Exacerbate and Perpetuate Health Disparities
The inequitable use of AI has the potential to exacerbate health disparities. Studies have revealed how population health management algorithms, which proxy healthcare needs with costs, allocate more care to white patients than to Black patients, even when health needs are accounted for. This disparity arises because the proxy target, correlated with access to and use of healthcare services, tends to identify frequent users of healthcare services, who are disproportionately less likely to be Black patients due to existing inequities in healthcare access. Inequitable AI perpetuates data bias when trained on skewed or incomplete datasets, inheriting and reinforcing the biases through algorithmic decisions, thereby deepening existing disparities and hindering efforts to achieve fairness and equity in healthcare delivery.
Increased Costs
Algorithms trained on biased datasets may exacerbate disparities by misdiagnosing or overlooking conditions prevalent in marginalized communities, leading to unnecessary tests, treatments, and hospitalizations and driving up costs. Health disparities, estimated to contribute $320 billion in excess healthcare spending, are compounded by the uneven adoption of AI in healthcare. The unequal access to AI-driven services widens gaps in healthcare spending, with affluent communities and resource-rich health systems often pioneering AI technologies, leaving underserved areas behind. Consequently, delayed diagnoses and suboptimal treatments escalate healthcare spending due to preventable complications and advanced disease stages.
Decreased Trust
The unequal distribution of AI-driven healthcare services breeds skepticism within marginalized communities. For instance, in one study, an algorithm demonstrated statistical fairness in predicting healthcare costs for Black and white patients, but disparities emerged in service allocation, with more white patients receiving referrals despite similar sickness levels. This disparity undermines trust in AI-driven decision-making processes, ultimately adding to mistrust in healthcare systems and providers.
How Bias Infiltrates CDS AI
Lack of Data Diversity and Inclusion
The datasets used to train AI models often mirror societal and healthcare inequities, propagating biases present in the data. For instance, if a model is trained on data from a healthcare system where certain demographic groups receive inferior care, it will internalize and perpetuate those biases. Compounding the issue, limited access to healthcare data leads AI researchers to rely on a handful of public databases, contributing to dataset homogeneity and lacking diversity. Additionally, while many clinical factors have evidence-based definitions and data collection standards, attributes that often account for variance in healthcare outcomes are less defined and more sparsely collected. As such, efforts to define and collect these attributes and promote diversity in training datasets are crucial to ensure the effectiveness and fairness of AI-driven healthcare interventions.
Lack of Transparency and Accountability
While AI systems are designed to streamline processes and enhance decision-making across healthcare, they also run the risk of inadvertently inheriting discrimination from their human creators and the environments from which they draw data. Many AI decision support technologies also struggle with a lack of transparency, making it challenging to fully comprehend and appropriately use their insights in a complex, clinical setting. By gaining clear visibility into how AI systems reach conclusions and establishing accountability measures for their decisions, the potential for harm can be mitigated and fairness promoted in their application. Transparency allows for the identification and remedy of any inherited biases, while accountability incentivizes careful consideration of how these systems may negatively or disproportionately impact certain groups. Both are necessary to build public trust that AI is developed and used responsibly.
Algorithmic Biases
The potential for algorithmic bias to permeate healthcare AI is significant and multifaceted. Algorithms and heuristics used in AI models can inadvertently encode biases that further disadvantage marginalized groups. For instance, an algorithm that assigns greater importance to variables like income or education levels may systematically disadvantage individuals from socioeconomically disadvantaged backgrounds.
Data scientists can adjust algorithms to reduce AI bias by tuning hyperparameters that optimize decision thresholds. These thresholds for flagging high-risk patients may need adjustment for specific groups to balance accuracy. Regular monitoring ensures thresholds address emerging biases over time. In addition, fairness-aware algorithms can apply statistical parity, where protected attributes like race or gender do not predict outcomes.
Unequal Access
Unequal access to AI technology exacerbates existing disparities and subjects the entire healthcare system to heightened bias. Even if an AI model itself is developed without inherent bias, the unequal distribution of access to its insights and recommendations can perpetuate inequities. When only healthcare organizations that can afford advanced AI for CDS leverage these tools, their patients enjoy the advantages of improved care that remain inaccessible to disadvantaged groups. Federal policy initiatives must prioritize equitable access to AI by implementing targeted investments, incentives, and partnerships for underserved populations. By ensuring that all healthcare entities, regardless of financial resources, have access to AI technologies, policymakers can help mitigate biases and promote fairness in healthcare delivery.
Misuse
The potential for bias in healthcare through the misuse of AI extends beyond the composition of training datasets to encompass the broader context of AI application and utilization. Ensuring the generalizability of AI predictions across diverse healthcare settings is as imperative as equity in the development of algorithms. It necessitates a comprehensive understanding of how AI applications will be deployed and whether the predictions derived from training data will effectively translate to various healthcare contexts. Failure to consider these factors may lead to improper use or abuse of AI insights.
Opportunity
Urgent policy action is essential to address bias, promote diversity, increase transparency, and enforce accountability in CDS AI systems. By implementing responsible oversight and governance, policymakers can harness the potential of AI to enhance healthcare delivery and reduce costs, while also ensuring fairness and inclusion. Regulations mandating the auditing of AI systems for bias and requiring explainability, auditing, and validation processes can hold organizations accountable for the ethical development and deployment of healthcare technologies. Furthermore, policymakers can establish guidelines and allocate funding to maximize the benefits of AI technology while safeguarding vulnerable groups. With lives at stake, eliminating bias and ensuring equitable access must be a top priority, and policymakers must seize this opportunity to enact meaningful change. The time for action is now.
Plan of Action
The federal government should establish and implement standards in AI governance and auditing for algorithms directly influencing diagnosis, treatment, and access to care of patients. These efforts should address and measure issues such as bias, transparency, accountability, and fairness. They should be flexible enough to accommodate advancements in AI technology while ensuring that ethical considerations remain paramount.
Regulate Auditing and Governance of AI
The federal government should implement a detailed auditing framework for AI in healthcare, beginning with stringent pre-deployment evaluations that require rigorous testing and validation against established industry benchmarks. These evaluations should thoroughly examine data privacy protocols to ensure patient information is securely handled and protected. Algorithmic transparency must be prioritized, requiring developers to provide clear documentation of AI decision-making processes to facilitate understanding and accountability. Bias mitigation strategies should be scrutinized to ensure AI systems do not perpetuate or exacerbate existing healthcare disparities. Performance reliability should be continuously monitored through real-time data analysis and periodic reviews, ensuring AI systems maintain accuracy and effectiveness over time. Regular audits should be mandated to verify ongoing compliance, with a focus on adapting to evolving standards and incorporating feedback from healthcare professionals and patients. AI algorithms evolve due to shifts in the underlying data, model degradation, and changes to application protocols. Therefore, routine auditing should occur at a minimum of annually.
With nearly 40% of Americans receiving benefits under a Medicare or Medicaid program, and the tremendous growth and focus on value-based care, the Centers for Medicare & Medicaid Services (CMS) is positioned to provide the catalyst to measure and govern equitable AI. Since many health systems and payers leverage models across multiple other populations, this could positively affect the majority of patient care. Both the companies making critical decisions and those developing the technology should be obliged to assess the impact of decision processes and submit select impact-assessment documentation to CMS.
For healthcare facilities participating in CMS programs, this mandate should be included as a Condition of Participation. Through this same auditing process, the federal government can capture insight into the performance and responsibility of AI systems. These insights should be made available to healthcare organizations throughout the country to increase transparency and quality between AI partners and decision-makers. This will help the Department of Health and Human Services (HHS) meet the “Promote Trustworthy AI Use and Development” pillar of its AI strategy (Figure 1).
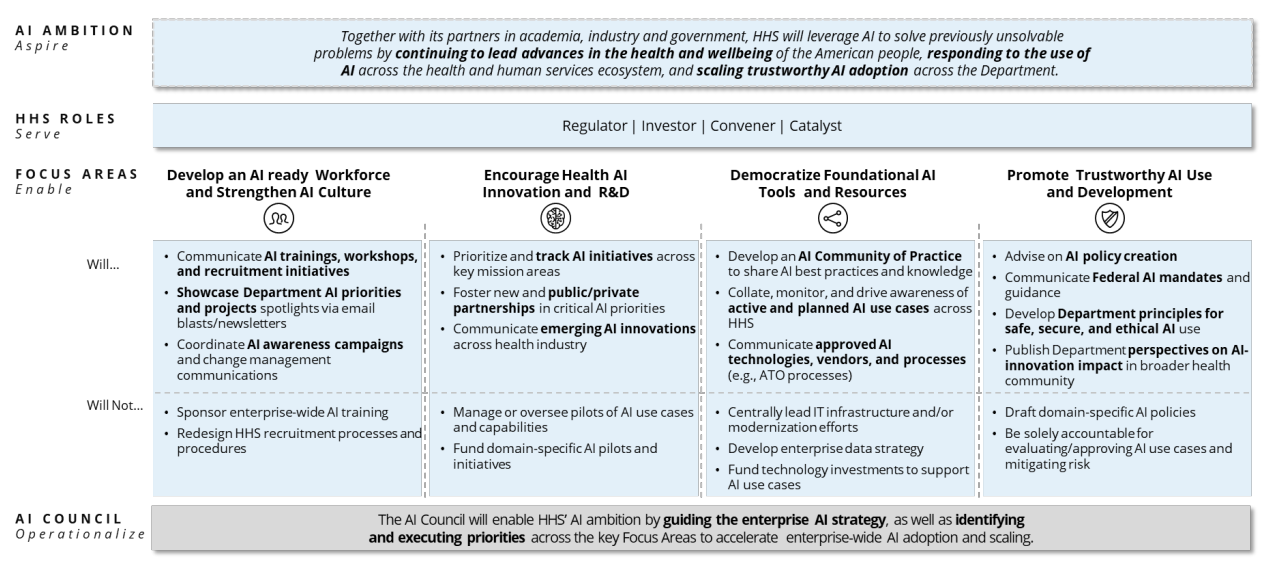
Congress must enforce these systems of accountability for advanced algorithms. Such work could be done by amending and passing the 2023 Algorithmic Accountability Act. This proposal mandates that companies evaluate the effects of automating critical decision-making processes, including those already automated. However, it fails to make these results visible to the organizations that leveraging these tools. An extension should be added to make results available to governing bodies and member organizations, such as the American Hospital Association (AHA).
Invest in AI Accessibility and Improvement
AI that integrates the social and clinical risk factors that influence preventive care could be beneficial in managing health outcomes and resource allocation, specifically for facilities providing care to mostly rural areas and patients. While organizations serving large proportions of marginalized patients may have access to nascent AI tools, it is very likely they are inadequate given they weren’t trained with data adequately representing this population. Therefore, the federal government should allocate funding to support AI access for healthcare organizations serving higher percentages of vulnerable populations. Initial support should stem from subsidies to AI service providers that support safety net and rural health providers.
The Health Resources and Services Administration should deploy strategic innovation funding to federally qualified health centers and rural health providers to contribute to and consume equitable AI. This could include funding for academic institutions, research organizations, and private-sector partnerships focused on developing AI algorithms that are fair, transparent, and unbiased specific for these populations.
Large language models (LLM) and GenAI solutions are being rapidly adopted in CDS tooling, providing clinicians with an instant second opinion in diagnostic and treatment scenarios. While these tools are powerful, they are not infallible and pose a risk without the ability to evolve. Therefore, research regarding AI self-correction should be a focus of future policy. Self-correction is the ability for an LLM or GenAI to identify and rectify errors without external or human intervention. Mastering the ability for these complex engines to recognize possible life-threatening errors would be crucial in their adoption and application. Healthcare agencies, such as the Agency for Healthcare Research and Quality (AHRQ) and the Office of the National Coordinator for Health Information Technology, should fund and oversee research for AI self-correction specifically leveraging clinical and administrative claims data. This should be an extension of either of the following efforts:
- 45 CFR Parts 170, 171 as it “promotes the responsible development and use of artificial intelligence through transparency and improves patient care through policies…which are central to the Department of Health and Human Services’ efforts to enhance and protect the health and well-being of all Americans”
- AHRQ’s funding opportunity from May 2024 (NOT-HS-24-014), Examining the Impact of AI on Healthcare Safety (R18)
Much like the Breakthrough Device Program, AI that can prove it decreases health disparities and/or increases accessibility can be fast-tracked through the audit process and highlighted as “best-in-class.”
Incentivize Data Collection and Collaboration
The newly released “Driving U.S. Innovation in Artificial Intelligence” roadmap considers healthcare a high-impact area for AI and makes specific recommendations for future “legislation that supports further deployment of AI in health care and implements appropriate guardrails and safety measures to protect patients,… and promoting the usage of accurate and representative data.” While auditing and enabling accessibility in healthcare AI, the government must ensure that the path to build equity into AI solutions does not remain an obstacle. This entails improved data collection and data sharing to ensure that AI algorithms are trained on diverse and representative datasets. As the roadmap declares, there must be “support the NIH in the development and improvement of AI technologies…with an emphasis on making health care and biomedical data available for machine learning and data science research while carefully addressing the privacy issues raised by the use of AI in this area.”
These data exist across the healthcare ecosystem, and therefore decentralized collaboration can enable a more diverse corpus of data to be available to train AI. This may involve incentivizing healthcare organizations to share anonymized patient data for research purposes while ensuring patient privacy and data security. This incentive could come in the form of increased reimbursement from CMS for particular services or conditions that involve collaborating parties.
To ensure that diverse perspectives are considered during the design and implementation of AI systems, any regulation handed down from the federal government should not only encourage but evaluate the diversity and inclusivity in AI development teams. This can help mitigate biases and ensure that AI algorithms are more representative of the diverse patient populations they serve. This should be evaluated by accrediting parties such as The Joint Commission (a CMS-approved accrediting organization) and their Healthcare Equity Certification.
Conclusion
Achieving health equity through AI in CDS requires concerted efforts from policymakers, healthcare organizations, researchers, and technology developers. AI’s immense potential to transform healthcare delivery and improving outcomes can only be realized if accompanied by measures to address biases, ensure transparency, and promote inclusivity. As we navigate the evolving landscape of healthcare technology, we must remain vigilant in our commitment to fairness and equity so that AI can serve as a tool for empowerment rather than perpetuating disparities. Through collective action and awareness, we can build a healthcare system that truly leaves no one behind.
This idea is part of our AI Legislation Policy Sprint. To see all of the policy ideas spanning innovation, education, healthcare, and trust, safety, and privacy, head to our sprint landing page.
Supporting States in Balanced Approaches to AI in K-12 Education
Congress must ensure that state education agencies (SEAs) and local education agencies (LEAs) are provided a gold-standard policy framework, critical funding, and federal technical assistance that supports how they govern, map, measure, and manage the deployment of accessible and inclusive artificial intelligence (AI) in educational technology across all K-12 educational settings. Legislation designed to promote access to an industry-designed and accepted policy framework will help guide SEAs and LEAs in their selection and use of innovative and accessible AI designed to align with the National Educational Technology Plan’s (NETP) goals and reduce current and potential divides in AI.
Although the AI revolution is definitively underway across all sectors of U.S. society, questions still remain about AI’s accuracy, accessibility, how its broad application can influence how students are represented within datasets, and how educators use AI in K-12 classrooms. There is both need and capacity for policymakers to support and promote thoughtful and ethical integration of AI in education and to ensure that its use complements and enhances inclusive teaching and learning while also protecting student privacy and preventing bias and discrimination. Because no federal legislation currently exists that aligns with and accomplishes these goals, Congress should develop a bill that targets grant funds and technical assistance to states and districts so they can create policy that is backed by industry and designed by educators and community stakeholders.
Challenge and Opportunity
With direction provided by Congress, the U.S. Department of Commerce, through the National Institute of Standards and Technology (NIST), has developed the Artificial Intelligence Risk Management Framework (NIST Framework). Given that some states and school districts are in the early stages of determining what type of policy is needed to comprehensively integrate AI into education while also addressing both known and potential risks, the hallmark guidance can serve as the impetus for developing legislation and directed-funding designed to help.
A new bill focused on applying the NIST Framework to K-12 education could create both a new federally funded grant program and a technical assistance center designed to help states and districts infuse AI into accessible education systems and technology, and also prevent discrimination and/or data security breaches in teaching and learning. As noted in the NIST Framework:
AI risk management is a key component of responsible development and use of AI systems. Responsible AI practices can help align the decisions about AI system design, development, and uses with intended aim and values. Core concepts in responsible AI emphasize human centricity, social responsibility, and sustainability. AI risk management can drive responsible uses and practices by prompting organizations and their internal teams who design, develop, and deploy AI to think more critically about context and potential or unexpected negative and positive impacts. Understanding and managing the risks of AI systems will help to enhance trustworthiness, and in turn, cultivate public trust.
In a recent national convening hosted by the U.S. Department of Education, Office of Special Education Programs, national leaders in education technology and special education discussed several key themes and questions, including:
- How does AI work and who are the experts in the field?
- What types of professional development are needed to support educators’ effective and inclusive use of AI?
- How can AI be responsive to all learners, including those with disabilities?
Participants emphasized the importance of addressing the digital divide associated with AI and leveraging AI to help improve accessibility for students, addressing AI design principles to help educators use AI as a tool to improve student engagement and performance, and assuring guidelines and policies are in use to protect student confidentiality and privacy. Stakeholders also specifically and consistently noted “the need for policy and guidance on the use of AI in education and, overall, the convening emphasized the need for thoughtful and ethical integration of AI in education, ensuring that it complements and enhances the learning experience,” according to notes from participants.”
Given the rapid advancement of innovation in education tools, states and districts are urgently looking for ways to invest in AI that can support teaching and learning. As reported in fall 2023,
Just two states—California and Oregon—have offered official guidance to school districts on using AI [in Fall 2023]. Another 11 states are in the process of developing guidance, and the other 21 states who have provided details on their approach do not plan to provide guidance on AI for the foreseeable future. The remaining states—17, or one-third—did not respond [to requests for information] and do not have official guidance publicly available.
While states and school districts are in various stages of developing policies around the use of AI in K-12 classrooms, to date there is no federally supported option that would help them make cohesive plans to invest in and use AI in evidence-based teaching and to support the administrative and other tasks educators have outside of instructional time. A major investment for education could leverage the expertise of state and local experts and encourage collaboration around breakthrough innovations to address both the opportunities and challenges. There is general agreement that investments in and support for AI within K-12 classrooms will spur educators, students, parents, and policymakers to come together to consider what skills both educators and students need to navigate and thrive in a changing educational landscape and changing economy. Federal investments in AI – through the application and use of the NIST Framework – can help ensure that educators have the tools to teach and support the learning of all U.S. learners. To that end, any federal policy initiative must also ensure that state, federal, and local investments in AI do not overlook the lessons learned by leading researchers who have spent years studying ways to infuse AI into America’s classrooms. As noted by Satya Nitta, former head researcher at IBM,
To be sure, AI can do sophisticated things such as generating quizzes from a class reading and editing student writing. But the idea that a machine or a chatbot can actually teach as a human can represents a profound misunderstanding of what AI is actually capable of… We missed something important. At the heart of education, at the heart of any learning, is [human] engagement.
Additionally, while current work led by Kristen DiCerbo at Khan Academy shows promise in the use of ChatGPT in Khanmingo, DiCerbo admits that their online 30-minute tutoring program, which utilizes AI, “is a tool in your toolbox” and is “not a solution to replacing humans” in the classroom. “In one-to-one teaching, there is an element of humanity that we have not been able to replicate—and probably should not try to replicate—in artificial intelligence. AI cannot respond to emotion or become your friend.”
With these data in mind, there is a great need and timely opportunity to support states and districts in developing flexible standards based on quality evidence. The NIST Framework – which was designed as a voluntary guide – is also “intended to be practical and adaptable.” State and district educators would benefit from targeted federal legislation that would elevate the Framework’s availability and applicability to current and future investments in AI in K-12 educational settings and to help ensure AI is used in a way that is equitable, fair, safe, and supportive of educators as they seek to improve student outcomes. Educators need access to industry-approved guidance, targeted grant funding, and technical assistance to support their efforts, especially as AI technologies continue to develop. Such state- and district-led guidance will help AI be operationalized in flexible ways to support thoughtful development of policies and best practices that will ensure school communities can benefit from AI, while also protecting students from potential harms.
Plan of Action
Federal legislation would provide funding for grants and technical assistance to states and districts in planning and implementing comprehensive AI policy-to-practice plans utilizing the NIST Framework to build a locally designed plan to support and promote thoughtful and ethical integration of AI in education and to ensure that its use complements and enhances inclusive teaching, accessible learning, and an innovation-driven future for all.
- Agency: U.S. Department of Education
- Cost: $500 Million
- Budget Line: ESEA: Title IV: Part A: Student Support and Academic Enrichment (SSAE) grant program, which supports well-rounded education, safe and healthy students, and the effective use of education technology.
Legislative Specifications
Sec. I: Grant Program to States
Purposes:
(A) To provide grants to State Education Agencies (SEA/State) to guide and support local education agencies (LEA/district) in the planning, development, and investment in AI in K-12 educational settings; ensuring AI is used in a way that is equitable, fair, safe, and can support educators and help improve student outcomes.
(B) To provide federal technical assistance (TA) to States and districts in the planning, development, and investments in AI in K-12 education and to evaluate State use of funds.
- SEAs apply for and lead the planning and implementation. Required partners in planning and implementation are:
- A minimum of three LEA/district teams, each of which includes
- A district leader.
- An expert in teacher professional development.
- A systems and or education technology expert.
- A minimum of three LEA/district teams, each of which includes
Each LEA/district must be representative of the students and the school communities across the state in size, demographics, geographic locations, etc.
Other requirements for state/district planning are:
- A minimum of one accredited state university providing undergraduate and graduate level personnel preparation to teachers, counselors, administrators, and/or college faculty
- A minimum of one state-based nonprofit organization or consortia of practitioners from the state with expertise in AI in education
- A minimum of one nonprofit organization with expertise in accessible education materials, technology/assistive technology
- SEAs may also include any partners the State or district(s) deems necessary to successfully conduct planning and carry out district implementation in support of K-12 students and to increase district access to reliable guidance on investments in and use of AI.
- SEAs must develop a plan that will be carried out by the LEA/district partners [and other LEAs] within the timeframe indicated.
- SEAs must utilize the NIST Framework, National Education Technology Plan, and recommendations included in the Office of Education Technology report on AI in developing the plan. Such planning and implementation must also:
- Focus on the state’s population of K-12 students including rural and urban school communities.
- Protect against bias and discrimination of all students, with specificity for student subgroups (i.e., economically disadvantaged students; students from each major racial/ethnic group; children with disabilities as defined under IDEA; and English learners) as defined by Elementary and Secondary Education Act.
- Support educators in the use of accessible AI in UDL-enriched and inclusive classrooms/schools/districts.
- Build in metrics essential to understanding district implementation impacts on both educators and students [by subgroup as indicated above].
- SEAs may also utilize any other resources they deem appropriate to develop a plan.
Timeline
- 12 months to plan
- 12 months to begin implementation, to be carried out over 24–36 months
- SEAs must set aside funds to:
- Conduct planning activities with partners as required/included over 12 months
- Support LEA implementation over 24 months.
- Support SEA/LEA participation in federal TA center evaluation—with the expectation such funding will not exceed 8–10% of the overall grant.
- Evaluation of SEA and LEA planning and implementation required
- Option to renew grant (within the 24-month period). Such renewal(s) are contingent on projected need across the state/LEA uptake and other reliable outcomes supporting an expanded roll-out across the state.
Sec. 2: Federal TA Center: To assist states in planning and implementing state-designed standards for AI in education.
Cost: 6% set-aside of overall appropriated annual funding
The TA center must achieve, at a minimum, the following expected outcomes:
(a) Increased capacity of SEAs to develop useful guidance via the NIST Framework, the National Education Technology Plan of 2024 and recommendations via the Office of Education Technology in the use of artificial intelligence (AI) in schools to support the use of AI for K-12 educators and for K-12 students in the State and the LEAs of the State;
(b) Increased capacity of SEAs, and LEAs to use new State and LEA-led guidance that ensures AI is used in a way that is equitable, fair, safe, protects against bias and discrimination of all students, and can support educators and help improve student outcomes.
(c) Improved capacity of SEAs to assist LEAs, as needed, in using data to drive decisions related to the use of K-12 funds to AI is used in a way that is equitable, fair, safe, and can support educators and help improve student outcomes.
(d) Collect data on these and other areas as outlined by the Secretary.
Timeline: TA Center is funded by the Secretary upon congressional action to fund the grant opportunity.
Conclusion
State and local education agencies need essential tools to support their use of accessible and inclusive AI in educational technology across all K-12 educational settings. Educators need access to industry-approved guidance, targeted grant funding, and technical assistance to support their efforts. It is essential that AI is operationalized in varying degrees and capacities to support thoughtful development of policies and best practices that ensure school communities can benefit from AI–while also being protected from its potential harms—now and in the future.
This idea is part of our AI Legislation Policy Sprint. To see all of the policy ideas spanning innovation, education, healthcare, and trust, safety, and privacy, head to our sprint landing page.
An Early Warning System for AI-Powered Threats to National Security and Public Safety
In just a few years, state-of-the-art artificial intelligence (AI) models have gone from not reliably counting to 10 to writing software, generating photorealistic videos on demand, combining language and image processing to guide robots, and even advising heads of state in wartime. If responsibly developed and deployed, AI systems could benefit society enormously. However, emerging AI capabilities could also pose severe threats to public safety and national security. AI companies are already evaluating their most advanced models to identify dual-use capabilities, such as the capacity to conduct offensive cyber operations, enable the development of biological or chemical weapons, and autonomously replicate and spread. These capabilities can arise unpredictably and undetected during development and after deployment.
To better manage these risks, Congress should set up an early warning system for novel AI-enabled threats to provide defenders maximal time to respond to a given capability before information about it is disclosed or leaked to the public. This system should also be used to share information about defensive AI capabilities. To develop this system, we recommend:
- Congress should assign and fund the Bureau of Industry and Security (BIS) to act as an information clearinghouse to receive, triage, and distribute reports on dual-use AI capabilities. In parallel, Congress should require developers of advanced models to report dual-use capability evaluations results and other safety critical information to BIS.
- Congress should task specific agencies to lead working groups of government agencies, private companies, and civil society to take coordinated action to mitigate risks from novel threats.
Challenge and Opportunity
In just the past few years, advanced AI has surpassed human capabilities across a range of tasks. Rapid progress in AI systems will likely continue for several years, as leading model developers like OpenAI and Google DeepMind plan to spend tens of billions of dollars to train more powerful models. As models gain more sophisticated capabilities, some of these could be dual-use, meaning they will “pose a serious risk to security, national economic security, national public health or safety, or any combination of those matters”—but in some cases may also be applied to defend against serious risks in those domains.
New AI capabilities can emerge unexpectedly. AI companies are already evaluating models to check for dual-use capabilities, such as the capacity to enhance cyber operations, enable the development of biological or chemical weapons, and autonomously replicate and spread. These capabilities could be weaponized by malicious actors to threaten national security or could lead to brittle, uncontrollable systems that cause severe accidents. Despite the use of evaluations, it is not clear what should happen when a dual-use capability is discovered.
An early-warning system would allow the relevant actors to access evaluation results and other details of dual-use capability reports to strengthen responses to novel AI-powered threats. Various actors could take concrete actions to respond to risks posed by dual-use AI capabilities, but they need lead time to coordinate and develop countermeasures. For example, model developers could mitigate immediate risks by restricting access to models. Governments could work with private-sector actors to use new capabilities defensively or employ enhanced, targeted export controls to deny foreign adversaries from accessing strategically relevant capabilities.
A warning system should ensure secure information flow between three types of actors:
- Finders: the parties that can initially identify dual-use capabilities in models. These include AI company staff, government evaluators such as the U.S. AI Safety Institute (USAISI), contracted evaluators and red-teamers, and independent security researchers.
- Coordinators: the parties that provide the infrastructure for collecting, triaging, and directing dual AI capability reports.
- Defenders: the parties that could take concrete actions to mitigate threats from dual-use capabilities or leverage them for defensive purposes, such as advanced AI companies and various government agencies.
While this system should cover a variety of finders, defenders, and capability domains, one example of early warning and response in practice might look like the following:
- Discovery: An AI company identifies a novel capability in one of its latest models during the development process. They find the model is able to autonomously detect, identify, and exploit cyber vulnerabilities in simulated IT systems.
- Reporting to coordinator: The company is concerned that this capability could be used to attack critical infrastructure systems, so they report the relevant information to a government coordinator.
- Triage and reporting to working groups: The coordinator processes this report and passes it along to the Cybersecurity and Infrastructure Security Agency (CISA), the lead agency for handling AI-enabled cyber threats to critical infrastructure.
- Verification and response: CISA verifies that this system can identify specific types of vulnerabilities in some legacy systems and creates a priority contract with the developer and critical infrastructure providers to use the model to proactively and regularly identify vulnerabilities across these systems for patching.
The current environment has some parts of a functional early-warning system, such as reporting requirements for AI developers described in Executive Order 14110, and existing interagency mechanisms for information-sharing and coordination like the National Security Council and the Vulnerabilities Equities Process.
However, gaps exist across the current system:
- There is a lack of clear intake channels and standards for capability reporting to the government outside of mandatory reporting under EO14110. Also, parts of the Executive Order that mandate reporting may be overturned in the next administration, or this specific use of the Defense Production Act (DPA) could be successfully struck down in the courts.
- Various legal and operational barriers mean that premature public disclosure, or no disclosure at all, is likely to happen. This might look like an independent researcher publishing details about a dangerous offensive cyber capability online, or an AI company failing to alert appropriate authorities due to concerns about trade secret leakage or regulatory liability.
- BIS intakes mandatory dual-use capability reports, but it is not tasked to be a coordinator and is not adequately resourced for that role, and information-sharing from BIS to other parts of government is limited.
- There is also a lack of clear, proactive ownership of response around specific types of AI-powered threats. Unless these issues are resolved, AI-powered threats to national security and public safety are likely to arise unexpectedly without giving defenders enough lead time to prepare countermeasures.
Plan of Action
Improving the U.S. government’s ability to rapidly respond to threats from novel dual-use AI capabilities requires actions from across government, industry, and civil society. The early warning system detailed below draws inspiration from “coordinated vulnerability disclosure” (CVD) and other information-sharing arrangements used in cybersecurity, as well as the federated Sector Risk Management Agency (SRMA) approach used to organize protections around critical infrastructure. The following recommended actions are designed to address the issues with the current disclosure system raised in the previous section.
First, Congress should assign and fund an agency office within the BIS to act as a coordinator–an information clearinghouse for receiving, triaging, and distributing reports on dual-use AI capabilities. In parallel, Congress should require developers of advanced models to report dual-use capability evaluations results and other safety critical information to BIS (more detail can be found in the FAQ). This creates a clear structure for finders looking to report to the government and provides capacity to triage reports and figure out what information should be sent to which working groups.
This coordinating office should establish operational and legal clarity to encourage voluntary reporting and facilitate mandatory reporting. This should include the following:
- Set up a reporting protocol where finders can report dual-use capability-related information: a full accounting of dual-use capability evaluations run on the model, details on mitigation measures, and information about the compute used to train affected models.
- Outline criteria for Freedom of Information Act (FOIA) disclosure exemptions for reported information in order to manage concerns from companies and other parties around potential trade secret leakage.1
- Adapt relevant protections for whistleblowers from their employers or contracting parties.
- If the relevant legal mechanism is not fit for purpose, Congress should include equivalent mechanisms in legislation. This can draw from similar legislation in the cybersecurity domain, such as the Cybersecurity Information Sharing Act 2015’s provisions to protect reporting organizations from antitrust and specific kinds of regulatory liability.
BIS is suited to house this function because it already receives reports on dual-use capabilities from companies via DPA authority under EO14110. Additionally, it has in-house expertise on AI and hardware from administering export controls on critical emerging technology, and it has relationships with key industry stakeholders, such as compute providers. (There are other candidates that could house this function as well. See the FAQ.)
To fulfill its role as a coordinator, this office would need an initial annual budget of $8 million to handle triaging and compliance work for an annual volume of between 100 and 1,000 dual-use capability reports.2 We provide a budget estimate below:
The office should leverage the direct hire authority outlined by Office of Personnel Management (OPM) and associated flexible pay and benefits arrangements to attract staff with appropriate AI expertise. We expect most of the initial reports would come from 5 to 10 companies developing the most advanced models. Later, if there’s more evidence that near-term systems have capabilities with national security implications, then this office could be scaled up adaptively to allow for more fine-grained monitoring (see FAQ for more detail).
Second, Congress should task specific agencies to lead working groups of government agencies, private companies, and civil society to take coordinated action to mitigate risks from novel threats. These working groups would be responsible for responding to threats arising from reported dual-use AI capabilities. They would also work to verify and validate potential threats from reported dual-use capabilities and develop incident response plans. Each working group would be risk-specific and correspond to different risk areas associated with dual-use AI capabilities:
- Chemical weapons research, development, and acquisition
- Biological weapons research, development, and acquisition
- Cyber-offense research, development, and acquisition
- Radiological and nuclear weapons research, development, and acquisition
- Deception, persuasion, manipulation, and political strategy
- Model autonomy and loss of control3
- For dual-use capabilities that fall into a category not covered by other lead agencies, USAISI acts as the interim lead until a more appropriate owner is identified.
This working group structure enables interagency and public-private coordination in the style of SRMAs and Government Coordination Councils (GCCs) used for critical infrastructure protection. This approach distributes responsibilities for AI-powered threats across federal agencies, allowing each lead agency to be appointed based on the expertise they can leverage to deal with specific risk areas. For example, the Department of Energy (specifically the National Nuclear Security Administration) would be an appropriate lead when it comes to the intersection of AI and nuclear weapons development. In cases of very severe and pressing risks, such as threats of hundreds or thousands of fatalities, the responsibility for coordinating an interagency response should be escalated to the President and the National Security Council system.
Conclusion
Dual-use AI capabilities can amplify threats to national security and public safety but can also be harnessed to safeguard American lives and infrastructure. An early-warning system should be established to ensure that the U.S. government, along with its industry and civil society partners, has maximal time to prepare for AI-powered threats before they occur. Congress, working together with the executive branch, can lay the foundation for a secure future by establishing a government coordinating office to manage the sharing of safety-critical across the ecosystem and tasking various agencies to lead working groups of defenders focused on specific AI-powered threats.
The longer research report this memo is based on can be accessed here.
This idea is part of our AI Legislation Policy Sprint. To see all of the policy ideas spanning innovation, education, healthcare, and trust, safety, and privacy, head to our sprint landing page.
This plan recommends that companies developing and deploying dual-use foundation models be mandated to report safety-critical information to specific government offices. However, we expect these requirements to only apply to a few large tech companies that would be working with models that fulfill specific technical conditions. A vast majority of businesses and models would not be subject to mandatory reporting requirements, though they are free to report relevant information voluntarily.
The few companies that are required to report should have the resources to comply. An important consideration behind our plan is to, where possible and reasonable, reduce the legal and operational friction around reporting critical information for safety. This can be seen in our recommendation that relevant parties from industry and civil society work together to develop reporting standards for dual-use capabilities. Also, we suggest that the coordinating office should establish operational and legal clarity to encourage voluntary reporting and facilitate mandatory reporting, which is done with industry and other finder concerns in mind.
This plan does not place restrictions on how companies conduct their activities. Instead, it aims to ensure that all parties that have equities and expertise in AI development have the information needed to work together to respond to serious safety and security concerns. Instead of expecting companies to shoulder the responsibility of responding to novel dangers, the early-warning system distributes this responsibility to a broader set of capable actors.
Bureau of Industry and Security (BIS) already intakes reports on dual-use capabilities via DPA authority under EO 14110
Department of Commerce
- USAISI will have significant AI safety-related expertise and also sits under Commerce
- Internal expertise on AI and hardware from administering export controls
US AI Safety Institute (USAISI), Department of Commerce
- USAISI will have significant AI safety-related expertise
- Part of NIST, which is not a regulator, so there may be fewer concerns on the part of companies when reporting
- Experience coordinating relevant civil society and industry groups as head of the AI Safety Consortium
Cybersecurity and Infrastructure Security Agency (CISA), Department of Homeland Security
- Experience managing info-sharing regime for cyber threats that involve most relevant government agencies, including SRMAs for critical infrastructure
- Experience coordinating with private sector
- Located within DHS, which has responsibilities covering counterterrorism, cyber and infrastructure protection, domestic chemical, biological, radiological, and nuclear protection, and disaster preparedness and response. That portfolio seems like a good fit for work handling information related to dual-use capabilities.
- Option of Federal Advisory Committee Act exemption for DHS Federal Advisory Committees would mean working group meetings can be nonpublic and meetings do not require representation from all industry representatives
Office of Critical and Emerging Technologies, Department of Energy (DOE)
- Access to DOE expertise and tools on AI, including evaluations and other safety and security-relevant work (e.g., classified testbeds in DOE National Labs)
- Links to relevant defenders within DOE, such as the National Nuclear Security Administration
- Partnerships with industry and academia on AI
- This office is much smaller than the alternatives, so would require careful planning and management to add this function.
Based on dual-use capability evaluations conducted on today’s most advanced models, there is no immediate concern that these models can meaningfully enhance the ability of malicious actors to threaten national security or cause severe accidents. However, as outlined in earlier sections of the memo, model capabilities have evolved rapidly in the past, and new capabilities have emerged unintentionally and unpredictably.
This memo recommends initially putting in place a lean and flexible system to support responses to potential AI-powered threats. This would serve a “fire alarm” function if dual-use capabilities emerge and would be better at reacting to larger, more discontinuous jumps in dual-use capabilities. This also lays the foundation for reporting standards, relationships between key actors, and expertise needed in the future. Once there is more concrete evidence that models have major national security implications, Congress and the president can scale up this system as needed and allocate additional resources to the coordinating office and also to lead agencies. If we expect a large volume of safety-critical reports to pass through the coordinating office and a larger set of defensive actions to be taken, then the “fire alarm” system can be shifted into something involving more fine-grained, continuous monitoring. More continuous and proactive monitoring would tighten the Observe, Orient, Decide, and Act (OODA) loop between working group agencies and model developers, by allowing agencies to track gradual improvements, including from post-training enhancements.
While incident reporting is also valuable, an early-warning system focused on capabilities aims to provide a critical function not addressed by incident reporting: preventing or mitigating the most serious AI incidents before they even occur. Essentially, an ounce of prevention is worth a pound of cure.
Sharing information on vulnerabilities to AI systems and infrastructure and threat information (e.g., information on threat actors and their tactics, techniques, and practices) is also important, but distinct. We think there should be processes established for this as well, which could be based on Information Sharing and Analysis Centers, but it is possible that this could happen via existing infrastructure for sharing this type of information. Information sharing around dual-use capabilities though is distinct to the AI context and requires special attention to build out the appropriate processes.
While this memo focuses on the role of Congress, an executive branch that is interested in setting up or supporting an early warning system for AI-powered threats could consider the following actions.
Our second recommendation—tasking specific agencies to lead working groups to take coordinated action to mitigate risks from advanced AI systems—could be implemented by the president via Executive Order or a Presidential Directive.
Also, the National Institute of Standards and Technology could work with other organizations in industry and academia, such as advanced AI developers, the Frontier Model Forum, and security researchers in different risk domains, to standardize dual-use capability reports, making it easier to process reports coming from diverse types of finders. A common language around reporting would make it less likely that reported information is inconsistent across reports or is missing key decision-relevant elements; standardization may also reduce the burden of producing and processing reports. One example of standardization is narrowing down thresholds for sending reports to the government and taking mitigating actions. One product that could be generated from this multi-party process is an AI equivalent to the Stakeholder-Specific Vulnerability Categorization system used by CISA to prioritize decision-making on cyber vulnerabilities. A similar system could be used by the relevant parties to process reports coming from diverse types of finders and by defenders to prioritize responses and resources according to the nature and severity of the threat.
The government has a responsibility to protect national security and public safety – hence their central role in this scheme. Also, many specific agencies have relevant expertise and authorities on risk areas like biological weapons development and cybersecurity that are difficult to access outside of government.
However, it is true that the private sector and civil society have a large portion of the expertise on dual-use foundation models and their risks. The U.S. government is working to develop its in-house expertise, but this is likely to take time.
Ideally, relevant government agencies would play central roles as coordinators and defenders. However, our plan recognizes the important role that civil society and industry play in responding to emerging AI-powered threats as well. Industry and civil society can take a number of actions to move this plan forward:
- An entity like the Frontier Model Forum can convene other organizations in industry and academia, such as advanced AI developers and security researchers in different risk domains, to standardize dual-use capability reports independent of NIST.
- Dual-use foundation model (DUFM) developers should establish clear policies and intake procedures for independent researchers reporting dual-use capabilities.
- DUFM developers should work to identify capabilities that could help working groups to develop countermeasures to AI threats, which can be shared via the aforementioned information-sharing infrastructure or other channels (e.g., pre-print publication).
- In the event that a government coordinating office cannot be created, there could be an independent coordinator that fulfills a role as an information clearinghouse for dual-use AI capabilities reports. This could be housed in organizations with experience operating federally funded research and development centers like MITRE or Carnegie Mellon University’s Software Engineering Institute.
- If it is responsible for sharing information between AI companies, this independent coordinator may need to be coupled with a safe harbor provision around antitrust litigation specifically pertaining to safety-related information. This safe harbor could be created via legislation, like a similar provision used in CISA 2015 or via a no-action letter from the Federal Trade Commission.
We suggest that reporting requirements should apply to any model trained using computing power greater than 1026 floating-point operations. These requirements would only apply to a few companies working with models that fulfill specific technical conditions. However, it will be important to establish an appropriate authority within law to dynamically update this threshold as needed. For example, revising the threshold downwards (e.g., to 1025) may be needed if algorithmic improvements allow developers to train more capable models with less compute or other developers devise new “scaffolding” that enables them to elicit dangerous behavior from already-released models. Alternatively, revising the threshold upwards (e.g., to 1027) may be desirable due to societal adaptation or if it becomes clear that models at this threshold are not sufficiently dangerous. The following information should be included in dual-use AI capability reports, though the specific format and level of detail will need to be worked out in the standardization process outlined in the memo:
- Name and address of model developer
- Model ID information (ideally standardized)
- Indicator of sensitivity of information
- A full accounting of the dual-use capabilities evaluations run on the model at the training and pre-deployment stages, their results, and details of the size and scope of safety-testing efforts, including parties involved
- Details on current and planned mitigation measures, including up-to-date incident response plans
- Information about compute used to train models that have triggered reporting (e.g., amount of compute and training time required, quantity and variety of chips used and networking of compute infrastructure, and the location and provider of the compute)
Some elements would not need to be shared beyond the coordinating office or working group lead (e.g., personal identifying information about parties involved in safety testing or specific details about incident response plans) but would be useful for the coordinating office in triaging reports.
The following information should not be included in reports in the first place since it is commercially sensitive and could plausibly be targeted for theft by malicious actors seeking to develop competing AI systems:
- Information on model architecture
- Datasets used in training
- Training techniques
- Fine-tuning techniques
Shared Classified Commercial Coworking Spaces
The legislation would establish a pilot program for the Department of Defense (DoD) to establish classified commercial shared spaces (think WeWork or hotels but for cleared small businesses and universities), professionalize industrial security protections, and accelerate the integration of new artificial intelligence (AI) technologies into actual warfighting capabilities. While the impact of this pilot program would be felt across the National Security Innovation Base, this issue is particularly pertinent to the small business and start-up community, for whom access to secure facilities is a major impediment to performing and competing for government contracts.
Challenge and Opportunity
The process of obtaining and maintaining a facility clearance and the appropriate industrial security protections is a major burden on nontraditional defense contractors, and as a result they are often disadvantaged when it comes to performing on and competing for classified work. Over the past decade, small businesses, nontraditional defense contractors, and academic institutions have all successfully transitioned commercial solutions for unclassified government contracts. However, the barriers to entry (cost, complexity, administrative burden, timeline) to engage in classified contracts has prevented similar successes. There have been significant and deliberate policy revisions and strategic pivots by the U.S. government to ignite and accelerate commercial technologies and solutions for government use cases, but similar reforms have not reduced the significant burden these organizations face when trying to secure follow-on classified work.
For small, nontraditional defense companies and universities, creating their own classified facility is a multiyear endeavor, is often cost-prohibitive, and includes coordination among several government organizations. This makes the prospect of building their own classified infrastructure a high-risk investment with an unknown return, thus deterring many of these organizations from competing in the classified marketplace and preventing the most capable technology solutions from rapid integration into classified programs. Similarly, many government contracting officers, in an effort to satisfy urgent operational requirements, only select from vendors with existing access to classified infrastructure due to knowing the long timelines involved for new entrants getting their own facilities accredited, thus further limiting the available vendor pool and restricting what commercial technologies are available to the government.
In January 2024, the Texas National Security Review published the results of a survey of over 800 companies from the defense industrial base as well as commercial businesses, ranging from small businesses to large corporations. 44 percent ranked “accessing classified environments as the greatest barrier to working with the government.” This was amplified in March 2024 during a House Armed Services Committee hearing on “Outpacing China in Defense Innovation,” where Under Secretary for Acquisition and Sustainment William LaPlante, Under Secretary for Research and Engineering Heidi Shyu, and Defense Innovation Unit Director Doug Beck all acknowledged the seriousness of this issue.
The current government method of approving and accrediting commercial classified facilities is based on individual customers and contracts. This creates significant costs, time delays, and inefficiencies within the system. Reforming the system to allow for a “shared” commercial model will professionalize industrial security protections and accelerate the integration of new AI technologies into actual national security capabilities. While Congress has expressed support for this concept in both the Fiscal Year 2018 National Defense Authorization Act and the Fiscal Year 2022 Intelligence Authorization Act, there has been little measurable progress with implementation.
Plan of Action
Congress should pass legislation to create a pilot program under the Department of Defense (DoD) to expand access to shared commercial classified spaces and infrastructure. The DoD will incur no cost for the establishment of the pilot program as there is a viable commercial market for this model. Legislative text has been provided and will be socialized with the committees of jurisdiction and relevant congressional members offices for support.
- Agency: U.S. Department of Defense
- Cost: $0
- Budget Line: N/A
Legislative Specifications
SEC XXX – ESTABLISHMENT OF PILOT PROGRAM FOR ACCESS TO SHARED CLASSIFIED COMMERCIAL INFRASTRUCTURE
(a) ESTABLISHMENT. – Not later than 180 days after the date of enactment of this act, the Secretary of Defense shall establish a pilot program to streamline access to shared classified commercial infrastructure in order to:
- (1) expand access by a small business concern, nontraditional defense contractors, and institutions of higher learning to secret/collateral accredited facilities and sensitive compartmented information facilities for the purpose of providing such contractors with a facility to securely perform work under existing classified contracts;
- (2) reduce the cost and administrative requirements on small businesses concern, nontraditional defense contractors, and institutions of higher learning to maintain access to sensitive compartmented information facilities;
- (3) increase opportunities for small businesses concerns, nontraditional defense contractors, and institutions of higher learning that have been issued a Facility Clearance to apply for federal government funding opportunities;
- (4) identify policy barriers that prevent broader use of shared classified commercial infrastructure, including access to required information technology systems, accreditation, and timelines;
(b) DESIGNATION. – The Secretary of Defense shall designate a principal civilian official responsible for overseeing the pilot program authorized in subsection (a)(1) and shall directly report to the Deputy Secretary of Defense.
- (1) RESPONSIBILITIES – The principal civilian official designated under subsection (b) shall:
- (A) seek to enter into a contract or other agreement with a private-sector entity or entities for access to shared classified commercial infrastructure and to facilitate utilization by covered small businesses and institutions of higher learning.
- (B) coordinate with the Directors of the Defense Counterintelligence and Security Agency, the Defense Intelligence Agency, and the Defense Information Systems Agency to prescribe policies and regulations governing the process and timelines pertaining to how shared commercial classified infrastructure may obtain relevant facility authorizations and access to secure information technology networks from the Department.
- (C) make recommendations to the Secretary in order to modernize, streamline, and accelerate the Department’s approval process of contracts, subcontracts, and co-use or joint use agreements for shared classified commercial infrastructure.
- (D) develop and maintain metrics tracking the outcomes of active and open facility accreditation requests from shared commercial classified infrastructure under the pilot program.
- (E) provide a report to the congressional defense committees, not later than 270 days after the enactment of this act, on the establishment of this pilot program.
(c) REQUIREMENTS.
- (1) As part of the pilot in subsection (a) the Directors of the Defense Counterintelligence and Security Agency, the Defense Intelligence Agency, and the Defense Information Systems Agency shall prescribe policies and regulations governing the process and timelines pertaining to how shared commercial classified infrastructure and facilities may obtain relevant facility authorizations and access to relevant secure information technology (IT) networks from the Department of Defense.
- (2) The pilot program shall include efforts to modernize, streamline, and accelerate the Department’s approval process of shared, co-use, and joint use agreements to facilitate the department’s access for small business concerns, nontraditional defense contractors and institutions of higher learning in classified environments.
(d) DEFINITION. – In this section:
- (1) The term “small business concern” has the meaning given such term under section 3 of the Small Business Act (15 U.S.C. 632).
- (2) the term “nontraditional defense contractor” has the meaning given in section 3014 of title 10, United States Code.
- (3) the term “institutions of higher learning” has the meaning given in section 3452(f) of title 38, United States Code.
- (4) the term “shared commercial classified infrastructure” means fully managed, shared, classified infrastructure, (facilities and networks), and associated services that are operated by an independent third-party, for the benefit of appropriated cleared government and commercial personnel that have limited or constrained access to secret collateral and sensitive compartmented information facilities.
(d) ANNUAL REPORT. – Not later than 270 days after the date of the enactment of this Act and annual thereafter until 2028, the Secretary of Defense shall provide to the congressional defense committees a report on establishment of this pilot program pursuant to this section, to include:
- (1) a list of all active and open facility accreditation requests from entities covered in subsection (a)(1), including the date the request was made to the Department and to the relevant facility accreditation agency.
- (2) a list of the total number of personnel authorized to conduct facility certification inspections under the pilot program.
- (3) actions taken to streamline the Department’s approval process for approval of co-use and joint use agreements to facilitate the department’s access to small business concerns, nontraditional defense contractors, and institutions of higher learning in classified environments.
(e) TERMINATION. – The authority to carry out this pilot program under subsection (a) shall terminate on the date that is five years after the date of enactment of this Act.
Conclusion
Congress must ensure that the nonfinancial barriers that prevent novel commercially developed AI capabilities and emerging technologies from transitioning into DoD and government use are reduced. Access to classified facilities and infrastructure continues to be a major obstacle for small businesses, research institutions, and nontraditional defense contractors working with the government. This pilot program will ensure reforms are initiated that reduce these barriers, professionalize industrial security protections, and accelerate the integration of new AI technologies into actual national security capabilities.
A National Center for AI in Education
There are immense opportunities associated with artificial intelligence (AI), yet it is important to vet the tools, establish threat monitoring, and implement appropriate regulations to guide the integration of AI into an equitable education system. Generative AI in particular is already being used in education, through human resource talent acquisition, predictive systems, personalized learning systems to promote students’ learning, automated assessment systems to support teachers in evaluating what students know, and facial recognition systems to provide insights about learners’ behaviors, just to name a few. Continuous research of AI’s use by teachers and schools is important to ensure AI’s positive integration into education systems worldwide is crucial for improved outcomes for all.
Congress should establish a National Center for AI in Education to build the capacity of education agencies to undertake evidence-based continuous improvement in AI in education. It will increase the body of rigorous research and proven solutions in AI use by teachers and students in education. Teachers will use testing and research to develop guidance for AI in education.
Challenge and Opportunity
It should not fall to one single person, group, industry, or country to decide what role AI’s deep learning should play in education—especially when that utility function will play a major role in creating new learning environments and more equitable opportunities for students.
Teachers need appropriate professional development on using AI not only so they can implement AI tools in their teaching but also so they can impart those skills and knowledge to their students. Survey research from EdWeek Research Center affirms that teachers, principals, and district leaders view the importance of teaching AI. Most disturbing is the lack of support and guidance around AI that teachers are receiving: 87% of teachers reported receiving zero hours of professional development related to incorporating AI into their work.
A National Center for AI in Education would transform the current model of how education technology is developed and monitored from a “supply creates the demand system” to a “demand creates the supply” system. Often, education technology resources are developed in isolation from the actual end users, meaning the teachers and students, and this exacerbates inequity. The Center will help to bridge the gap between tech innovators and the classroom, driving innovation and ensuring AI aligns with educational goals.
The collection and use of data in education settings has expanded dramatically in recent decades, thanks to advancements in student information systems, statistical software, and analytic methods, as well as policy frameworks that incentivize evidence generation and use in decision-making. However, this growing body of research all too frequently ignores the effective use of AI in education. The challenges, assets, and context of AI in education vary greatly within states and across the nation. As such, evidence that is generated in real time within school settings should begin to uncover the needs of education related to AI.
Educators need research, regulation, and policies that are understood in the context of educational settings to effectively inform practice and policy. Students’ preparedness for and transition into college or the workforce is of particular concern, given spatial inequities in the distribution of workforce and higher-education opportunities and the dual imperatives of strengthening student outcomes while ensuring future community vitality. The teaching and use of AI all play into this endeavor.
An analog for this proposal is the National Center for Rural Education Research Networks (NCRERN), an Institute of Education Sciences research and development center that has demonstrated the potential of research networks for generating rigorous, causal evidence in rural settings through multi-site randomized controlled trials. NCRERN’s work leading over 60 rural districts through continuous improvement cycles to improve student postsecondary readiness and facilitate postsecondary transitions generated key insights about how to effectively conduct studies, generate evidence, influence district practice, and improve student outcomes. NCRERN research is used to inform best practices with teachers, counselors, and administrators in school districts, as well as inform and provide guidance for policymaking on state, local, and federal levels.
Another analog is Indiana’s AI-Powered Platform Pilot created by the Indiana Department of Education. The pilot launched during the 2023–2024 school year with 2,500 teachers from 112 schools in 36 school corporations across Indiana using approved AI platforms in their classrooms. More than 45,000 students are impacted by this pilot. A recent survey of teachers in the pilot indicated that 53% rated the overall impact of the AI platform on their students’ learning and their teaching practice as positive or very positive.
In the pilot, a competitive grant opportunity funds the subscription fees and professional development support for student high dosage tutoring and reducing teacher workload using an AI platform. The vision for this opportunity is to focus on a cohort of teachers and students in the integration of an AI platform. It might be used to support a specific building, grade level, subject area, or student population. Schools are encouraged to focus on student needs in response to academic impact data.
Plan of Action
Congress should authorize the establishment of a National Center for AI in Education whose purpose is to research and develop guidance for Congress regarding policy and regulations for the use of AI in educational settings.
Through a competitive grant process, a university should be chosen to house the Center. This Center should be established within three years of enactment by Congress. The winning institution will be selected and overseen by either the Institute of Education Sciences or another office within the Department of Education. The Department of Education and National Science Foundation will be jointly responsible for supporting professional development along with the Center awardee.
The Center should begin as a pilot with teachers selected from five participating states. These PK-12 teachers will be chosen via a selection process developed by the Center. Selected teachers will have expertise in AI technology and education as evidenced by effective classroom use and academic impact data. Additional criteria could include innovation mindset, willingness to collaborate with knowledge of AI technologies, innovative teaching methods, commitment to professional development, and a passion for improving student learning outcomes. Stakeholders such as students, parents, and policymakers should be involved in the selection process to ensure diverse perspectives are considered.
The National Center for AI in Education’s duties should include but not be limited to:
- Conducting research on the use of AI in education and its impact on student learning. This research would then inform guidance pertaining to development, use, policy, and regulation development. Potential research areas include:
- Assessing learning: Process vs. Product. Plagiarism because of the Large Language Model’s (LLM) ability to produce text.
- Use of generative AI as the first draft and beginning for creativity, thus raising the bar for student learning.
- AI hallucinations.
- Recommending AI technology for educational purposes, with a focus on outcomes.
- Establishing best practices for educators to address the pitfalls of privacy, replication, and bias.
- Providing resources and training for teachers about and use of AI in education.
Congress should authorize funding for the National Center for AI in Education. Funding should be provided by the federal government to support its research and operations. Plans should be made for a 3–5-year pilot grant as well as a continuation/expansion grant after the first 3–5-year funding cycle. Additional funding may be obtained through grants, donations, and partnerships with private organizations.
Reporting on progress to monitor and evaluate the Center’s pursuits. The National Center for AI in Education would submit an annual report to Congress detailing its research findings, advising and providing regulatory guidance, and impact on education. There will need to be a plan for the National Center for AI in Education to be subject to regular evaluation and oversight to ensure its compliance with legislation and regulations.
To begin this work of the National Center for AI in Education will:
- Research and develop courses of action for improvement of AI algorithms to mitigate bias and privacy issues: Regularly reassess AI algorithms used in samples from the Center’s pilot states and school districts and make all necessary adjustments to address those issues.
- Incorporate AI technology developers into the feedback loop by establishing partnerships and collaborations. Invite developers to participate in research projects, workshops, and conferences related to AI in education. Research and highlight promising practices in teaching responsible AI use for students: Teaching about AI is as important, if not more important, as teaching with AI. Therefore, extensive curriculum research should be done for teaching students how to ethically and effective use AI to enhance their learning. Incorporate real-world application of AI into coursework so students are ready to use AI effectively and ethically in the next chapter of their postsecondary journey.
- Develop an AI diagnostic toolkit: This toolkit, which should be made publicly available for state agencies and district leaders, will analyze teacher efficacy, students’ grade level mastery, and students’ postsecondary readiness and success.
- Provide professional development for teachers on effective and ethical AI use: Training should include responsible use of generative AI and AI for learning enhancement.
- Monitor systems for bias and discrimination: Test tools to identify unintended bias to ensure that they do not perpetuate gender, racial, or social discrimination. Study and recommend best practices and policies.
- Develop best practices for ensuring privacy: Ensure that student, family, and staff privacy are not compromised by the use of facial recognition or recommender systems. Protect students’ privacy, data security, and informed consent. Research and recommend policies and IT solutions to ensure privacy compliance.
- Curate proven algorithms that protect student and staff autonomy: Predictive systems can limit a person’s ability to act on their own interest and values. The Center will identify and highlight algorithms that are proven to not jeopardize our students or teachers’ self-freedom.
In addition, the National Center for AI in Education will conduct five types of studies:
- Descriptive quantitative studies exploring patterns and predictors of teachers’ and students’ use of AI. Diagnostic studies will draw on district administrative, publicly available, and student survey data.
- Mixed methods case studies describing the context of teachers/schools participating in the Center and how stakeholders within these communities conceptualize students’ postsecondary readiness and success. One case study per pilot state will be used, drawing on survey, focus group, observational, and publicly available data.
- Development evaluations of intervention materials developed by educators and content experts. AI sites/software will be evaluated through district prototyping and user feedback from students and staff.
- Block cluster randomized field trials of at least two AI interventions. The Center will use school-level randomization, blocked on state and other relevant variables, to generate impact estimates on students’ postsecondary readiness and success. The Center will use the ingredients methods to additionally estimate cost-effectiveness estimates.
- Mixed methods implementation studies of at least two AI interventions implemented in real-world conditions. The Center will use intervention artifacts (including notes from participating teachers) as well as surveys, focus groups, and observational data.
Findings will be disseminated through briefs targeted at a policy and practitioner audience, academic publications, conference presentations, and convenings with district partners.
A publicly available AI diagnostic toolkit will be developed for state agencies and district leaders to use to analyze teacher efficacy, students on grade level mastery, and students’ postsecondary readiness and success. This toolkit will also serve as a resource for legislators to keep up to date on AI in education.
Professional development, ongoing coaching, and support to district staff will also be made available to expand capacity for data and evidence use. This multifaceted approach will allow the National Center for AI in Education to expand capacity in research related to AI use in education while having practical impacts on educator practice, district decision-making, and the national field of rural education research.
Conclusion
The National Center for AI in Education would be valuable for United States education for several reasons. First, it could serve as a hub for research and development in the field, helping to advance our understanding of how AI can be effectively used in educational settings. Second, it could provide resources and support for educators looking to incorporate AI tools into their teaching practices. Third, it could help to inform future policies, as well as standards and best practices for the use of AI education, ensuring that students are receiving high-quality, ethically sound educational experiences. A National Center for AI in Education could help to drive innovation and improvement in the field, ultimately benefiting students and educators alike.
This idea is part of our AI Legislation Policy Sprint. To see all of the policy ideas spanning innovation, education, healthcare, and trust, safety, and privacy, head to our sprint landing page.
Message Incoming: Establish an AI Incident Reporting System
What if an artificial intelligence (AI) lab found their model had a novel dangerous capability? Or a susceptibility to manipulation? Or a security vulnerability? Would they tell the world, confidentially notify the government, or quietly patch it up before release? What if a whistleblower wanted to come forward – where would they go?
Congress has the opportunity to proactively establish a voluntary national AI Incident Reporting Hub (AIIRH) to identify and share information about AI system failures, accidents, security breaches, and other potentially hazardous incidents with the federal government. This reporting system would be managed by a designated federal agency—likely the National Institute of Standards and Technology (NIST). It would be modeled after successful incident reporting and info-sharing systems operated by the National Cybersecurity FFRDC (funded by the Cybersecurity and Infrastructure Security Agency (CISA)), the Federal Aviation Administration (FAA), and the Food and Drug Administration (FDA). This system would encourage reporting by allowing for confidentiality and guaranteeing only government agencies could access sensitive AI systems specifications.
AIIRH would provide a standardized and systematic way for companies, researchers, civil society, and the public to provide the federal government with key information on AI incidents, enabling analysis and response. It would also provide the public with some access to these data in a reliable way, due to its statutory mandate – albeit often with less granularity than the government will have access to. Nongovernmental and international organizations, including the Responsible AI Collaborative (RAIC) and the Organisation for Economic Co-operation and Development (OECD), already maintain incident reporting systems, cataloging incidents such as facial recognition systems identifying the wrong person for arrest and trading algorithms causing market dislocations. However, these two systems have a number of limitations in their scope and reliability that make them more suitable for public accountability than government use.
By establishing this system, Congress can enable better identification of critical AI risk areas before widespread harm occurs. This proposal would help both build public trust and, if implemented successfully, would help relevant agencies recognize emerging patterns and take preemptive actions through standards, guidance, notifications, or rulemaking.
Challenge and Opportunity
While AI systems have the potential to produce significant benefits across industries like healthcare, education, environmental protection, finance, and defense, they are also potentially capable of serious harm to individuals and groups. It is crucial that the federal government understand the risks posed by AI systems and develop standards, best practices, and legislation around its use.
AI risks and harms can take many forms, from representational (such as women CEOs being underrepresented in image searches), to financial (such as automated trading systems or AI agents crashing markets), to possibly existential (such as through the misuse of AI to advance chemical, biological, radiological, and nuclear (CBRN) threats). As these systems become more powerful and interact with more aspects of the physical and digital worlds, a material increase in risk is all but inevitable in the absence of a sensible governance framework. However, in order to craft public policy that maximizes the benefits of AI and ameliorates harms, government agencies and lawmakers must understand the risks these systems pose.
There have been notable efforts by agencies to catalog types of risks, such as NIST’s 2023 AI Risk Management Framework, and to combat the worst of them, such as the Department of Homeland Security’s (DHS) efforts to mitigate AI CBRN threats. However, the U.S. government does not yet have an adequate resource to track and understand specific harmful AI incidents that have occurred or are likely to occur in the real world. While entities like the RAIC and the OECD manage AI incident reporting efforts, these systems primarily collect publicly reported incidents from the media, which are likely a small fraction of the total. These databases serve more as a source of public accountability for developers of problematic systems than a comprehensive repository suitable for government use and analysis. The OECD system lacks a proper taxonomy for different incident types and contexts, and while the RAIC database applies two external taxonomies to their data, it only does so at an aggregated level. Additionally, the OECD and RAIC systems depend on their organizations’ continued support, whereas AIIRH would be statutorily guaranteed.
The U.S. government should do all it can to facilitate as comprehensive reporting of AI incidents and risks as possible, enabling policymakers to make informed decisions and respond flexibly as the technology develops. As it has done in the cybersecurity space, it is appropriate for the federal government to act as a focal point for collection, analysis, and dissemination of data that is nationally distributed, is multi-sectoral, and has national impacts. Many federal agencies are also equipped to appropriately handle sensitive and valuable data, as is the case with AI system specifications. Compiling this kind of comprehensive dataset would constitute a national public good.
Plan of Action
We propose a framework for a voluntary Artificial Intelligence Incident Reporting Hub, inspired by existing public initiatives in cybersecurity, like the list of Common Vulnerabilities and Exploits (CVE)1 funded by CISA, and in aviation, like the FAA’s confidential Aviation Safety Reporting System (ASRS).
AIIRH should cover a broad swath of what could be considered an AI incident in order to give agencies maximal data for setting standards, establishing best practices, and exploring future safeguards. Since there is no universally agreed-upon definition of an AI safety “incident,” AIIRH would (at least initially) utilize the OECD definitions of “AI incident” and “AI hazard,” as follows:
- An AI incident is an event, circumstance or series of events where the development, use or malfunction of one or more AI systems directly or indirectly leads to any of the following harms:
- (a) injury or harm to the health of a person or groups of people;
- (b) disruption of the management and operation of critical infrastructure;
- (c) violations of human rights or a breach of obligations under the applicable law intended to protect fundamental, labour and intellectual property rights;
- (d) harm to property, communities or the environment.
- An AI hazard is an event, circumstance or series of events where the development, use or malfunction of one or more AI systems could plausibly lead to an AI incident, i.e., any of the following harms:
- (a) injury or harm to the health of a person or groups of people;
- (b) disruption of the management and operation of critical infrastructure;
- (c) violations to human rights or a breach of obligations under the applicable law intended to protect fundamental, labour and intellectual property rights;
- (d) harm to property, communities or the environment.
With this scope, the system would cover a wide range of confirmed harms and situations likely to cause harm, including dangerous capabilities like CBRN threats. Having an expansive repository of incidents also sets up organizations like NIST to create and iterate on future taxonomies of the space, unifying language for developers, researchers, and civil society. This broad approach does introduce overlap on voluntary cybersecurity incident reporting with the expanded CVE and National Vulnerability Database (NVD) systems proposed by Senators Warner and Tillis in their Secure AI Act. However, the CVE provides no analysis of incidents, so it should be viewed instead as a starting point to be fed into the AIIRH2, and the NVD only applies traditional cybersecurity metrics, whereas the AIIRH could accommodate a much broader holistic analysis.
Reporting submitted to AIIRH should highlight key issues, including whether the incident occurred organically or as the result of intentional misuse. Details of harm either caused or deemed plausible should also be provided. Importantly, reporting forms should allow maximum information but require as little as possible in order to encourage industry reporting without fear of leaking sensitive information and lower the implied transaction costs of reporting. While as much data on these incidents as possible should be broadly shared to build public trust, there should be guarantees that any confidential information and sensitive system details shared remain secure. Contributors should also have the option to reveal their identity only to AIIRH staff and otherwise maintain anonymity.
NIST is the natural candidate to function as the reporting agency, as it has taken a larger role in AI standards setting since the release of the Biden Administration’s Executive Order on the Safe, Secure, and Trustworthy Development and Use of Artificial Intelligence. NIST also has experience with incident reporting through their NVD, which contains agency experts’ analysis of CVE incidents. Finally, similar to how the National Aeronautics and Space Administration (NASA) operates the FAA’s confidential reporting system, ASRS, as a neutral third party, NIST is a non-enforcing agency with excellent industry relationships due to its collaborations on standards and practices. CISA is another option, as it funds and manages several incident reporting systems, including over AI security if the Warner-Tillis bill passes, but there is no reason to believe CISA has the expertise to address harms caused by things like algorithmic discrimination or CBRN threats.
While NIST might be a trusted party to maintain a confidential system, employees reporting credible threats to AIIRH should have additional guarantees against retaliation from their current/former employers in the form of whistleblower protections. These are particularly relevant in light of reports that OpenAI, an AI industry leader, is allegedly neglecting safety and preventing employee disclosure through restrictive nondisparagement agreements. A potential model could be whistleblower protections introduced in California SB1047, where employers are forbidden from preventing, or retaliating based upon, the disclosure of an AI incident to an appropriate government agent.
In order to further incentivize reporting, contributors may be granted advanced, real-time, or more complete access to the AIIRH reporting data. While the goal is to encourage the active exchange of threat vectors, in acknowledgment of the aforementioned confidentiality issues, reporters could opt out from having their data shared in this way, forgoing their own advanced access. If they allow a redacted version of their incident to be shared anonymously with other contributors, they could still maintain access to the reporting data.
Key stakeholders include:
- NIST’s Information Services Office
- NIST’s Artificial Intelligence Safety Institute
- DHS/CISA Stakeholder Engagement Division and Cybersecurity Division
- Congressional and Legislative Affairs Office and Office of Information Systems Management
Related proposed bills include:
- Secure AI Act – Senators Warner and Tillis
- California State Bill 1047 – Senators Wiener, Roth, Rubio, Stern
The proposal is likely to require congressional action to appropriate funds for the creation and implementation of the AIIRH. It would require an estimated $10–25 million annually to create and maintain AIIRH, pay-for to be determined.3
Conclusion
An AI Incident Reporting System would enable informed policymaking as the risks of AI continue to develop. By allowing organizations to report information on serious risks that their systems may pose in areas like CBRN, illegal discrimination, and cyber threats, this proposal would enable the U.S. government to collect and analyze high-quality data and, if needed, promulgate standards to prevent the proliferation of dangerous capabilities to non-state actors. By incentivizing voluntary reporting, we can preserve innovative and high-value uses of AI for society and the economy, while staying up-to-date with the quickly evolving frontier in cases where regulatory oversight is paramount.
This idea is part of our AI Legislation Policy Sprint. To see all of the policy ideas spanning innovation, education, healthcare, and trust, safety, and privacy, head to our sprint landing page.
NIST has institutional expertise with incident reporting, having maintained the National Vulnerability Database and Disaster Data Portal. NIST’s role as a standard-setting body leaves it ideally placed to keep pace with developments in new areas of technology. This role as a standard-setting body that frequently collaborates with companies, while not regulating them, allows them to act as a trusted home for cross-industry collaboration on sensitive issues. In the Biden Administration’s Executive Order on AI, NIST was given authority over establishing testbeds and guidance for testing and red-teaming of AI systems, making it a natural home for the closely-related work here.
AIIRH staff shall be empowered to conduct follow-ups on credible threat reports, and to share information with Department of Commerce, Department of Homeland Security, Department of Defense, and other agency leadership on those reports.
AIIRH staff could work with others at NIST to build a taxonomy of AI incidents, which would provide a helpful shared language for standards and regulations. Additionally, staff might share incidents as relevant with interested offices like CISA, Department of Justice, and the Federal Trade Commission, although steps should be taken to minimize retribution against organizations who voluntarily disclosed incidents (in contrast to whistleblower cases).
Similar to the logic of companies disclosing cybersecurity vulnerabilities and incidents, voluntary reporting builds public trust, earns companies favor with enforcement agencies, and increases safety broadly across the community. The confidentiality guarantees provided by AIIRH should make the prospect more appealing as well. Separately, individuals at organizations like OpenAI and Google have demonstrated a propensity towards disclosure through whistleblower complaints when they believe their employers are acting unsafely.